Uc Berkeley Hiv Report Re Blacks in Prison, 2005
Download original document:
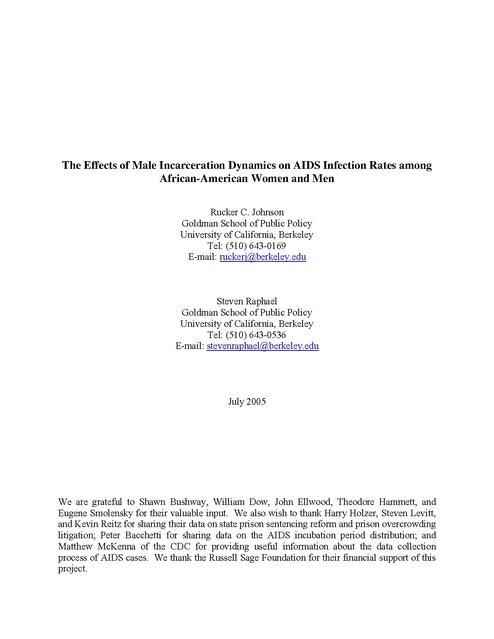
Document text
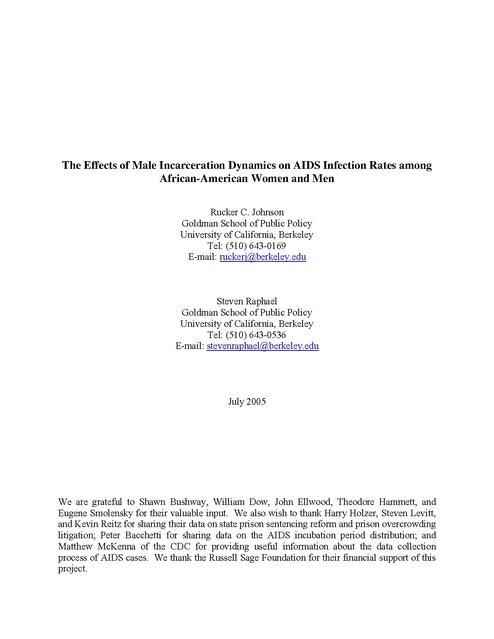
Document text
This text is machine-read, and may contain errors. Check the original document to verify accuracy.
The Effects of Male Incarceration Dynamics on AIDS Infection Rates among African-American Women and Men Rucker C. Johnson Goldman School of Public Policy University of California, Berkeley Tel: (510) 643-0169 E-mail: ruckerj@berkeley.edu Steven Raphael Goldman School of Public Policy University of California, Berkeley Tel: (510) 643-0536 E-mail: stevenraphael@berkeley.edu July 2005 We are grateful to Shawn Bushway, William Dow, John Ellwood, Theodore Hammett, and Eugene Smolensky for their valuable input. We also wish to thank Harry Holzer, Steven Levitt, and Kevin Reitz for sharing their data on state prison sentencing reform and prison overcrowding litigation; Peter Bacchetti for sharing data on the AIDS incubation period distribution; and Matthew McKenna of the CDC for providing useful information about the data collection process of AIDS cases. We thank the Russell Sage Foundation for their financial support of this project. The Effects of Male Incarceration Dynamics on AIDS Infection Rates among African-American Women and Men Abstract In this paper, we investigate the potential connection between incarceration dynamics and AIDS infection rates, with a particular emphasis on the black-white AIDS rate disparity. Using caselevel data from the U.S. Centers for Disease Control and Prevention, we construct a panel data set of AIDS infection rates covering the period 1982 to 2001 that vary by year of onset, mode of transmission, state of residence, age, gender, and race/ethnicity. Using data from the U.S. Census, we construct a conforming panel of male and female incarceration rates. We use this panel data to model the dynamic relationship between the male and female AIDS infection rates and the proportion of men in the age/state/race-matched cohort that are incarcerated. We find very strong effects of male incarceration rates on both male and female AIDS infection rates. The dynamic structure of this relationship—i.e., the lagged effects of the proportion of incarcerated males—parallels the distribution of the incubation time between HIV infection and the onset of full-blown AIDS documented in the medical and epidemiological literature. These results are robust to explicit controls for (race-specific) year fixed effects and a fully interacted set of age/race/state fixed effects. Our results reveal that the higher incarceration rates among black males over this period explain a substantial share of the racial disparity in AIDS infection between black women and women of other racial and ethnic groups. In a separate analysis, we estimate a two-stage-least-squares (TSLS) model of AIDS infection rates employing a set of variables describing intra-state changes in sentencing regimes as instruments for variation in incarceration rates. We find TSLS effects of incarceration rates on AIDS infection rates that are significant and comparable in magnitude to the corresponding OLS estimates. 1 I. Introduction Coincident with the large increase in black male incarceration rates is a pronounced increase in the AIDS infection rate among African-American women and men. Between 1970 and 2000, the proportion of black men incarcerated on any given day increased from 0.03 to 0.08, with a much larger increase in the proportion that has ever been to prison. There is no comparable increase among non-Hispanic white men. Concurrently, the HIV/AIDS infection rate among African-American women went from zero during the pre-epidemic period to an annual rate of 55 per 100,000 between 2000 and 2003 (an infection rate nearly nineteen times higher than that for non-Hispanic white women). For African-American men, this rate exceeds 100 per 100,000 (in contrast to less than 15 per 100,000 among non-Hispanic white men). Moreover, African-Americans accounted for half of the AIDS cases reported in 2002, despite accounting for only 12 percent of the overall population. The sources of racial differences in HIV/AIDS infection rates are not well understood. In this paper, we investigate the potential connection between incarceration dynamics and AIDS infection rates. Our analysis considers the role of the relatively high levels of black male incarceration as a potential explanation for the black-white AIDS rate disparity. This research represents the first systematic analysis of the relationship between incarceration and AIDS infection rates using nationally representative population data from the U.S.. Has the trend of increased incarceration, most pronounced among African-Americans, produced the deleterious effects of accelerated AIDS infection rates among affected communities? Our task is to sort out how much, if any, of the observed correlations in these aggregate trends represent causal relationships of incarceration on subsequent AIDS infection rate trajectories. An increase in male incarceration rates may affect HIV/AIDS infection rates among inmates and members of the community at large through several channels. First, the relatively high concentration of HIV-positive people in prison (Hammett et. al. 2002) coupled with risky behavior among inmates (Krebs 2002, Swartz et. al. 2004) may accelerate the transmission rate of the disease among the incarcerated and among non-incarcerated members of the sexual networks of former inmates. Second, the temporal dynamics of incarceration, characterized by brief incarceration spells and the cycling in and out of institutions, may increase the degree of concurrent sexual relationships (sexual relationships that overlap in time) among inmates and their non-institutionalized partners (Adimora and Schoenbach 2005). This is a factor known to augment the risk of contracting and spreading sexually transmitted diseases. In addition, spells of incarceration may hasten the dissolution of sexual relationships, enlarging the total lifetime number of sex partners among inmates and their partners. An increase in incarceration rates may be viewed as an exogenous shock to an affected individual or group’s sexual-relationship market (in much the same way economists traditionally conceive of marriage markets (Becker 1981)). In particular, male incarceration lowers the sex ratio (male-to-female), abruptly disrupts the continuity of heterosexual relationships, and increases exposure to homosexual activity for incarcerated males—all of which may have farreaching implications for an individual or group’s AIDS infection risk. Given the relatively high rate of incarceration among black men, all these avenues of HIV/AIDS transmission are likely to have disproportionate effects on the AIDS infection rates of black women and men. An alternative explanation for an apparent relationship between a community’s AIDS prevalence and the proportion that has ever served time in prison is that it is spurious and stems from rates of participation in risky behaviors that affect both AIDS and incarceration rates, such as drug use. We attempt to distinguish between these competing explanations, which is the principle challenge of the empirical work. Our empirical strategy exploits the fact that the overwhelming majority of sexual relationships as well as marriages occur between women and men in relationship markets defined by the interaction of race, age, and state of residence (Charles and Luoh 2005, Laumann 2 et. al. 1994). We exploit this stratification of sexual relationships and the tremendous variation in the incarceration trends over the past two decades within these groups, to identify the effect of incarceration on AIDS infection rates. Accordingly, we define sexual relationship markets by age/state/race groupings, since sexually-transmitted AIDS infection is the hypothesized chief mechanism linking incarceration and AIDS. Using case-level data from the U.S. Centers for Disease Control and Prevention, we construct a panel data set of AIDS infection rates covering the period 1982 to 2001. Our tabulated infection rates vary by year of onset, mode of transmission, state of residence, age, gender, and race/ethnicity. Using data from the U.S. Census, we construct a conforming panel of male and female incarceration rates. We use this panel data to model the dynamic relationship between the male and female AIDS infection rates and the proportion of men in the age/state/race-matched cohort that are incarcerated. The impact of incarceration is identified from variation within sexual relationship markets over time. We estimate 13-year (constrained) distributed lag models separately by gender and mode of transmission to gain greater understanding of the underlying pathways through which incarceration dynamics may affect HIV/AIDS infection rates. To preview the results, we find very strong effects of male incarceration rates on both male and female AIDS infection rates. The dynamic structure of this relationship—i.e., the lagged effects of the proportion of incarcerated males—parallels the distribution of the incubation time between HIV infection and the onset of full-blown AIDS documented in the medical and epidemiological literature. These results are robust to explicit controls for (racespecific) year fixed effects and a fully interacted set of age/race/state fixed effects. Our results reveal that the higher incarceration rates among black males over this period explain a large share of the racial disparity in AIDS infection between black women and women of other racial and ethnic groups. During the decade of the 1990s, the largest component of the growth in the 3 racial disparity in female AIDS infection rates resulted from infection occurring through heterosexual sex (as opposed to intravenous drug use), while homosexually-contracted AIDS was a growing component of the black-white AIDS gap among men. These results taken together suggest that high black male incarceration rates is a principal explanation for the relatively high rate of infection among black women. The strong link between incarceration and AIDS is further evidenced in our findings that the black-white gap in homosexually-contracted AIDS infection rates among males can be fully accounted for by black’s higher incarceration rates. In a separate analysis, we assess whether the effect of incarceration on AIDS infection rates are causal by re-estimating a modified model specification using instrumental variables. Specifically, we estimate a two-stage-least-squares (TSLS) model employing a set of variables describing intra-state changes in sentencing regimes as instruments for variation in incarceration rates. We find TSLS effects of incarceration rates on AIDS infection rates that are significant and comparable in magnitude to the corresponding OLS estimates. The remainder of the paper is organized as follows. Section II provides a brief overview of the conceptual framework of plausible mechanisms linking incarceration and AIDS infection rates. This section also provides a literature review of the state of knowledge on HIV transmission risk (and AIDS prevalence) in and out of prison, related characteristics and behaviors of individuals who serve time in prison, and a brief discussion of factors that affect the spread of the AIDS epidemic in a community more generally. identification strategy and econometric model. Section III lays out our Section IV describes the data sources and provides descriptive analysis. Sections V and VI present the results from the dynamic regression models and the instrumental variables approach, respectively. Section VII concludes with discussion of the implications of the results for public health and criminal justice policy. 4 II. Incarceration and HIV/AIDS Transmission Among Inmates and the Community Our paper builds upon and extends two strands of literature. The first is the analysis of previously unexplored dimensions of the intended and unintended consequences of incarceration policy. The second contributes to our understanding of the sources of racial/ethnic differences in HIV/AIDS infection rates and the divergent patterns that have emerged over the past two decades. At the nexus of these two literatures, this paper explores the connection between AIDS and the number of men relative to women in the sexual-relationship market and, of these men, the proportion who have ever served time in prison or who are at high risk of imprisonment in the future. The general epidemiological assumption that behavior is exogenous to environment can lead to erroneous conclusions about the cause of disease patterns, and consequently, to misguided public health policy decisions. This was emphasized as a cautionary note in Kremer’s (1996) work on AIDS. For example, an economic model of the spread of infectious diseases hypothesizes that individuals will alter their demand for risky activities as risk increases, holding all else constant (Philipson and Posner 1993). Ahituv, Hotz, and Philipson’s (1996) results suggest there has been an increase in the demand for safer sex, such as the use of condoms, in response to the increase in the prevalence of AIDS. They find that while there was no difference in condom demand among U.S. census regions in 1984 (before AIDS cases were very prevalent), the incidence of condom usage became geographically heterogeneous as the AIDS epidemic progressed, with higher rates of utilization in states with higher AIDS prevalence rates, and more rapid growth in condom utilization among black men, single men, those in urban areas, and those who are more sexually active. Based on their estimates, they conclude that more than half of the rise in condom use among young adults that occurred during the second half of the 1980s can be explained solely by the increases in local prevalence of AIDS cases that occurred during this decade. However, it must be borne in mind that the imperfect information of transmission risk 5 possessed by an individual, due to delays in onset and awareness of HIV prevalence, is likely to significantly limit the efficacy of this behavioral response in slowing the speed of the AIDS epidemic in a community. How, then, would a large exogenous increase in incarceration rates affect the rate at which HIV/AIDS propagates through a given population? Perhaps the most mechanical effect may occur through the incapacitation of a group of individuals who are likely to engage in risky behaviors while not incarcerated. To the extent that prisons remove from society individuals whose behavior accelerate the spread of infectious diseases, an increase in incarceration may actually reduce the overall incidence of HIV/AIDS. Even if offenders are eventually returned to society, their time in prison reduces the total exposure of the non-incarcerated public to high-risk individuals, holding all else constant. However, there are likely to be countervailing effects of incarceration that may accelerate the spread of HIV/AIDS. For example, the concentration of high-risk individuals behind bars coupled with the behavioral responses (sexual and otherwise) to being incarcerated may elevate the rate at which inmates transmit the disease to each other. Thus, while reducing the aggregate exposure time of the non-incarcerated to the incarcerated, imprisonment may raise the AIDS incidence among those who are currently serving or have served time. Furthermore, as most inmates are returned to society after a relatively short prison spell, an accelerated transmission rate among inmates may spillover to the non-institutionalized population post-release. A less obvious transmission mechanism may occur through the effect of incarceration on the process by which sexual partners match with another. Given that inmates are overwhelmingly male and minority, a disproportionately large increase in incarceration among minority men differentially reduces the ratio of minority men to minority women. This relative scarcity of minority men improves the bargaining position of non-institutionalized minority men in negotiating personal relationships. The relative shortage of men may translate into men 6 having to display less commitment or loyalty in seeking sexual relations, a factor that is likely to increase the average lifetime number of partners among both sexes and perhaps even the incidence of concurrent sexual relationships (both known risk factors for the spread of sexually transmitted diseases). Moreover, independently of these effects, the disruptive effects of men cycling in and out of institutions may further increase the risk of HIV transmission through the disruption of existing heterosexual relationships (and by extension, the creation of new relationships) and the increase in the average lifetime number of sex partners. The net effect of an increase in incarceration rates on AIDS transmission is thus theoretically ambiguous.1 While this net effect is essentially the main empirical question that we address below, here we discuss existing research pertaining to these questions. Evidence of incapacitation and enhanced transmission behind bars The relatively high prevalence of various infectious diseases among the imprisoned is well documented in the U.S. and abroad. Between 2 and 3 percent of prison inmates in the U.S. have HIV/AIDS, a figure that is nearly 5 times the infection rates for the general population (Hammett et. al. 2002). The World Health Organization documents comparably high infection rates among prisoners in Western and Eastern Europe (WHO 2001). U.S. prisoners also account for disproportionately large shares of those infected with the hepatitis B virus (Macalino et. al. 2004) and the hepatitis C virus (Hammett et. al. 2002, Macalino et. al. 2004), and have been linked to several tuberculosis outbreaks within prisons and within communities receiving released inmates (Freudenberg 2001). The relatively high HIV/AIDS infection rates among U.S. prison inmates may reflect either a selected population at high risk of infection regardless of incarceration, an elevated risk of infection while incarcerated, or some combination of the two. To the extent that the high rate simply reflects pre-incarceration behavior, imprisonment may simply isolate a high risk 1 In future work, we plan to develop a formal model of the supply and demand dynamics of sexual behavior, with emphasis on the role of incarceration and implications for HIV epidemics, which nest these ideas. 7 population from the general public and thus reduce transmission, holding all else equal. To be sure, the profile of the average inmate clearly indicates that the incarcerated population is drawn from a sub-population at high risk of having HIV/AIDS. The typical inmate in the United States is relatively young, poor, minority, with very low levels of educational attainment (Raphael and Stoll 2005), and is likely to engage in risky sexual activity and drug abuse prior to becoming incarcerated (Swartz et. al. 2004). Thus, part of the higher infection rate among inmates is certainly attributable to the pre-prison behaviors and characteristics of inmates themselves. Whether the incarceration of these high-risk individuals epidemiologically incapacitates them and reduces HIV/AIDS transmission is an unanswered question. However, there is ample evidence of an incapacitation effect of incarceration on crime (Levitt 1996, Raphael and Stoll 2005), and thus the proposition that similar effects exist for the transmission of infectious diseases is not implausible. Any incapacitation effect of incarceration on overall transmission rates, however, may be offset by an instrumentally higher transmission rate while incarcerated. Concentrating high-risk individuals behind bars may accelerate the transmission of HIV/AIDS among inmates (and ultimately the general community) due to behaviors that are specific to prison as well as risks that are faced specifically by inmates. Researchers have identified a number of behaviors that are common in correctional settings that may facilitate transmission of blood-borne illnesses, such as tattooing, drug use, and high-risk sexual activity. Tattooing is a common practice in prison culture, and prisoners often receive tattoos while incarcerated under unsanitary conditions (Krebs 2002). While intravenous drug use is likely to be suppressed while incarcerated, some prisoners do indeed abuse drugs, and many argue that intravenous drug use in prison is more likely to involve risky sharing of needles among users (Hammett 2004). Research pertaining to consensual sexual activity among inmates provides a fairly imprecise portrait of the extent to which prisoners have sex behind bars. Most research indicates 8 that between 20 percent (Tewksbury 1989) and 65 percent (Wooden and Parker 1982) engage in sexual activity while incarcerated (although at least one study cited in Krebs (2002) provides an estimate as low as 2 percent). There is even greater uncertainty regarding the likelihood of being sexually assaulted while incarcerated, although there is consensus that the risk for males of being sexually assaulted while incarcerated exceeds the comparable risk while not incarcerated.2 Nonetheless, it seems safe to assume that sex among inmates is not infrequent and that the sexual activity that occurs is particularly high risk. The risk of transmission is greatest for men who have sex with other men, and the overwhelming majority of prisons and jails (95 percent) in the United States do not provide condoms to inmates (Hammett 2004). The results from inmate surveys suggest that many of these high-risk behaviors are associated with prison sub-culture and the material and emotional deprivation of being institutionalized. Krebs’ (2004) survey of prison inmates in a southern state of the U.S. reveals that many prisoners believe that tattooing is an activity that often commences behind bars and occurs with increasing frequency while incarcerated. Inmates also indicated that they believed that at least half of inmates engage in homosexual sex while incarcerated, and that most who do had no prior homosexual intercourse before entering prison. Thus, while prisoners in general are likely to be drawn from sub-segments of the population with high HIV/AIDS infection rates, behaviors that are common behind bars are likely to independently elevate the rate of transmission. There are a handful of studies that attempt to measure the rate at which HIV is transmitted within prisons. This research typically follows one of two strategies: (1) estimating 2 The Bureau of Justice Statistics recently completed the first-ever national survey of administrative records on sexual violence in adult and juvenile correctional facilities. This data collection effort to estimate the incidence and prevalence of sexual violence in prison was mandated by the Prison Rape Elimination Act of 2003. Administrative records alone, however, cannot provide reliable estimates of sexual violence because victims are often reluctant to report incidents to correctional authorities due to fear of retaliation from perpetrators. During 2004, an estimated 8,210 allegations of sexual violence were reported by correctional authorities—the equivalent of 3.2 allegations per 1,000 inmates and youths incarcerated in 2004. See http://www.ojp.usdoj.gov/bjs/abstract/svrca04.htm for details of the BJS report, the survey instrument and methods for data collection. 9 the rate at which new inmates seroconvert (test negative upon entering prison and positive at a follow up date) while incarcerated (Brewer et. al. 1988, Horsburgh et. al. 1990, Castro et. al. 1994, Macalino et. al. 2004), or (2) assessing the degree to which long-term prisoners that had been incarcerated since before the start of the AIDS epidemic become infected with the HIV virus (Mutter et. al. 1994, Krebs and Simmons 2002). While not all of these studies tabulate annual transmission rates (thus limiting comparability),3 those that do suggest transmission rates per year served on the order of 0.1 to 0.5 percent. By comparison, the CDC estimates that there were 40,000 new HIV cases in 2001, giving an overall transmission rate for the general population of 0.014 percent. Thus, the extant research suggests that the rate of transmission in prison is between 7 and 35 times the rate of transmission for the nation overall. However, this relatively high transmission rate behind bars may occur at a similarly high rate among the comparable non-incarcerated population. This evidence does not address the relevant questions pertaining to the group’s counterfactual HIV transmission rate that would have prevailed in the absence of their imprisonment, nor does it resolve the related question of what the community’s HIV incidence rate would have been in the short- and long-run had these individuals not been incarcerated. It is possible for the short- and long-run impacts of an increase in incarceration on a community’s HIV infection rates to move in opposite directions. For example, an increase in incarceration may result in a short-run decline in HIV incidence due to an incapacitation effect followed by a subsequent long-run increase post-release due to inmates’ elevated risks of acquiring HIV while previously incarcerated. The attempt to construct appropriate counterfactual estimates of HIV/AIDS rates under different scenarios, and identifying exogenous sources of variation in incarceration rates to do so, is the chief challenge empirically. 3 For example, Krebs and Simmon (2002) tabulate the proportion of long term inmates in a southern state that test positive before leaving prison but do not normalize for time served. See Hammett (2004) for a thorough discussion of this research. 10 The effect of incarceration on concurrency and the lifetime number of sexual partners The rate at which a sexually transmitted disease spreads through a population depends critically on the initial prevalence of the disease in the population, the rates at which new sexual relationships form and dissolve, the riskiness of the sexual activity involved, and the degree to which members of the population engage in concurrent sexual relationships. An increase in incarceration rates may alter the sexual behaviors of the non-incarcerated in ways that increase the risk of transmission. Of particular importance are the effects of incarceration on the total lifetime number of sex partners and the likelihood of concurrent sexual relationships. The rates at which new relationships form and dissolve impacts the lifetime number of sexual partners at any given age, which affects the risk of sexual contact with an infected person. Concurrent sexual relationships increase transmission rates through a number of channels. Morris and Kretzchmar (1995, 1996) note that when an individual who is engaged in concurrent sexual relationships becomes infected, a subsequent transmission is likely to occur more rapidly. Subsequent transmissions do not depend on the dissolution of the relationship generating the initial infection. In addition, for serially monogamous relationships, prior partners are protected from a newly infected current partner. For concurrent relationships, however, there is no comparable sequential break between the sexual networks of an individual’s various sex partners. Incarceration may impact the number of sex partners and the likelihood of concurrency through two specific avenues: through a destabilizing effect on existing relationships and through a general equilibrium effect on sexual relationship markets. The dynamics of prison entry and exit, coupled with a large increase in incarceration rates for men, are likely to impact the rate at which existing sexual relationships dissolve and form. The majority of men that enter U.S. prisons will serve relatively short spells (a median of 2 years) followed by even shorter spells (for roughly two thirds of releases) usually triggered by a parole violation (Raphael and Stoll 11 2005).4 In addition to time actually served in prison, spells outside of prison are likely to be punctuated by jail time while awaiting trial or while attempting to make bail. These periodic absences from non-incarcerated partners are likely to result in the formation of new relationships by the partners left behind, as well as new sexual relationships formed by the inmate while incarcerated, thus increasing the total lifetime number of partners. To the extent that these ancillary relationships continue after an inmate is released and returns to previous partners, the churning in and out of prison may augment the extent of concurrency. A more subtle pathway through which a large increase in incarceration may affect the formation of new relationships and concurrency operates through the impact of incarceration on the ratio of non-incarcerated men-to-women. The model of social exchange applied to the formation of sexual relationships in Baumeister and Vohs (2005) serves to illustrate this point. In this model, the social interactions at the beginning of heterosexual relationships are akin to a bargaining process, where the relationship will form if both parties can agree on the terms. Factors that will influence whether the relationship forms may include the degree of commitment and loyalty displayed by members of either sex, the perceived trustworthiness of the potential partner, potential promises of economic security, etc. The value of what is gained and exchanged in a relationship market is determined in part by preferences and in part by broader market conditions. Market forces tend to stabilize the rate of exchange within a community (but not necessarily across communities). For example, a decline in the relative supply of men (driven by an increase in incarceration) may lead women to lower their standards and match with less reliable and less stable men. Accordingly, with a lower sex ratio, non-incarcerated men may lower the degree to which they make perceived costly commitments to ensure the formation of a new sexual relationship, and may display less loyalty. 4 In an analysis of 18 to 25 year olds entering the California state prison system in 1990, Raphael (2005) finds that over the subsequent decade, the typical inmate served 2.8 years behind bars with roughly 5 years elapsing between the date of the initial admission and the final release. For black inmates, the comparable figures were 3 years served over a 6.2 year period. 12 In the aggregate, the degree of concurrency may increase, as well as the total number of sex partners at a given age. While there is little direct evidence of an effect of incarceration on relationship formations and concurrency operating through this channel, Charles and Louh (2005) show that higher male imprisonment has lowered the likelihood that women marry, reduced the quality of their spouses when they do, and caused a shift in the gains from marriage away from women and towards men. In addition, low sex ratios have been shown to be associated with higher rates of teen pregnancy (Sampson 1995), syphilis (Kilmarx et. al. 1997) and gonorrhea (Thomas et. al. 2003). Incarceration trends and racial differences in AIDS infection rates The mechanisms noted above – incapacitation effects operating through the temporary isolation of high-risk individuals, elevated transmission rates while incarcerated, and effects of incarceration dynamics on the formation of new sexual relationships and concurrency – should disproportionately impact the African-American community in the United States. Roughly onefifth of black adult males in the U.S. have served time (Raphael 2005), and many of these men have cycled in and out of correctional institutions for fairly long periods of their early adult lives. The ratio of men to women among the non-institutionalized is markedly lower for non-Hispanic blacks than for non-Hispanic whites (Adimora and Schoenbach 2005). Moreover, black women are nearly twice as likely to have recently had concurrent partnerships relative to white women (Adimora and Schoenbach 2005), and, on average, they have higher lifetime numbers of partners holding age constant (all factors that may result theoretically from high black male incarceration rates).5 Whether these factors translate into greater AIDS infection rates among African- Americans is the question to which we now turn. 5 Our own tabulations of the 2001-2002 National Health and Nutrition Examination Survey Sexual Behavior Component revealed that black women on average have greater lifetime numbers of sexual partners relative to white women. These differences are on the order of 20 percent and disappear for women over 50. It is notable that these race differences in the lifetime number of sexual partners are specific to only younger cohorts and emerged coincident with the trends of increasing incarceration rates of the past several decades. These differences did not exist (or are not detected) among older generations, suggesting cohort effects. 13 III. Our Empirical Strategy The empirical strategy taken in this paper builds on the observation that the overwhelming majority of marriages occur within demographic groups defined by the interaction of race, age, socioeconomic markers such as education, and earnings, and state of residence (Charles and Luoh 2005). Moreover, high inter-marriage rates within these demographic and socioeconomic sub-groups mirror the stratification of sexual relationships along these lines, thus creating sharp and distinct sexual relationship markets (Laumann et. al. 1994). To identify the effect of incarceration rates on AIDS infection rates, we exploit this empirical regularity and the substantial variation in the incarceration trends over this period occurring within these demographic groups. Accordingly, we define sexual relationship markets by the interaction of race, age, and state of residence. We use standard panel data methods to estimate the partial effect of incarceration rates on infection rates using variation in both series occurring within the defined sexual relationship markets after purging the data of race-, age-, and state-specific time trends. The strategy presumes that the remaining variation in the male incarceration trends within sexual relationship markets is akin to differential shocks to the sexual relationship markets driven by disparate male incarceration patterns over the past two decades. By focusing the analysis on AIDS cases (i.e., advanced-stage HIV) rather than earlystage HIV (which is often asymptomatic), we minimize differences in reported rates that are simply an artifact of differential interaction with the health care system, which result in differences in rates of early detection. Since our principal dependent variable of analysis is the rate at which demographic subgroups develop full blown AIDS, the relationship between incarceration and new AIDS infection is inherently dynamic. Several factors will induce a delayed response between the male incarceration rate and AIDS infection rates for both men and women. For both genders, variance in the AIDS incubation distribution – where incubation is 14 defined as the time between HIV infection and the development of a measurably suppressed immune system – will induce a lag between any incarceration-induced infections and newly diagnosed AIDS cases. Estimates of the cumulative distribution function (CDF) of incubation for the pre-1996 period6 reveal sharp increases in the proportion developing full blown AIDS starting three years post seroconversion and a flattening of the CDF at around ten years post infection (Bacchetti 1990, Brookmeyer 1991, U.K. Register of HIV Seroconverters Steering Committee 1998). These estimates suggest that roughly one-quarter of HIV-positive individuals develop AIDS within six years, one-half within nine years, and three-quarters within twelve years. For women who are at risk of infection via heterosexual relationships with former inmates, time served will also induce a lag between men becoming infected while incarcerated and the ultimate infection of female partners. Moreover, conditional on having sex with an infected person, the probability of acquiring HIV depends on a number of factors, including whether the sex is unprotected and the specific act. To account for the likely lagged effect of incarceration rates on infection rates, we estimate a dynamic panel data model where we allow incarceration to impact AIDS infection rates over a thirteen-year period. Specifically, our principal estimates come from estimation of the regression equation 13 13 τ =0 τ =0 AIDSRate rsat = ∑ ω mτ IM rsa ,t −τ + ∑ ω fτ IFrsa ,t −τ + δ rsa + λ rt + φ st + π at + ε Rsat , (1) where r indexes racial/ethnic groups, s indexes state of residence, a indexes age groups, and t indexes year of infection. The variable AIDSRatersat measures the number of new AIDS cases diagnosed per 100,000 individuals from race group r, age group a, in state s, during year t; 6 The AIDS incubation period was altered considerably by the introduction of antiretroviral drugs in 1996, with the variance increasing considerably along with the median and mean time to the development of symptoms. For this reason, the empirical tests below will focus on the pre-1996 period. 15 IM rsa ,t − c provides the male incarceration rate (defined as the proportion incarcerated at a point in time for the given year) for the demographic group rsa for the contemporaneous year of infection and for thirteen lagged years; IFrsa ,t −c provides the comparable incarceration rates for women; δ rsa denote a complete set of sexual-relationship market fixed effects defined by the interaction of race, age, and state of residence; λrt denote a complete set of race-specific year effects; φ st denote a complete set of state-specific year effects; π at provides a complete set of age-specific year effects; and ε rsat is the random error term. Finally, the parameters ωmτ and ω fτ provide the coefficients on the contemporaneous and lagged incarceration rates and provide the principal parameters of interest. Before discussing the details of the dynamic structure of the model, a brief discussion of the variation being used to identify the incarceration effects is needed. Equation (1) includes both sexual relationship market fixed effects and allows race-specific, age-specific, and statespecific year effects. The sexual relationship market fixed effects allow us to control for (timeinvariant) market-specific characteristics, such as drug use prevalence or behavioral norms, that are otherwise difficult to quantify. Allowing for race-specific, age-specific, and state-specific individual year effects controls for race- and age-specific trends that might exist in AIDS incidence at the national level, and overall trends that may vary by state. Factors that may create such trends include changes in sexual awareness and AIDS prevention (AIDS-preventative risk behaviors), innovations in medical treatments that delay the onset of advanced stage HIV/AIDS, changes in drug use technologies and prevalence (e.g., crack cocaine epidemic began in 1985), and changes in guidelines for reporting cases. Collectively, the inclusion of the sexual market effects and the various time effects means that we are identifying the effect of incarceration on AIDS infection rates using variation in both series occurring within sexual relationship networks after accounting for race, age, and state 16 level time trends in both variables. That is, the effect of incarceration is estimated off of the differential variation in the incarceration rate in a market over time, relative to overall (racespecific, age-specific, state-specific) trends. We estimate equation (1) using weighted least squares, where we weight by the population size of each group defined by race, state, age, and year. Finally, to ensure that our statistical inferences are robust to serial correlation in the error term, we estimate the standard errors of the model by bootstrapping. We estimate equation (1) separately by gender. Since sexually-transmitted AIDS infection is the hypothesized chief mechanism linking incarceration and AIDS, we also estimate the equation separately for new AIDS infections contracted through heterosexual sex (for women), homosexual sex (for men), in addition to estimating models for overall AIDS infection rates. In each model, we control for the contemporaneous and lagged incarceration rates for both genders. Given the high degree of correlation between current and lagged incarceration rates, we use a third-order polynomial distributed (Almon) lag for both male and female incarceration rates to reduce multicollinearity problems and yet allow a fairly flexible structure on the shape of the lag distribution. Our modeling of the lag structure is guided by the medical and epidemiological evidence regarding the pre-1996 incubation period (which suggests no more than two inflections in the incubation probability distribution function). We tested alternative lag lengths and higher-order polynomials, but none significantly improved the fit of the model. We further constrain the lag coefficients to equal zero for those whose transmission effects correspond with time periods that predate the AIDS epidemic (i.e., before 1980). For example, for AIDS rates in 1985 we constrain all coefficients on lagged incarceration rates in excess of five years to zero; for AIDS rates in 1986 we constrain all coefficients on lagged incarceration rates in excess of six years to zero, and so on. Thus, given that our panel begins in 1982, the matrix of the lag coefficients (W) on the male incarceration rates for the first twelve years of the panel is given by 17 W= 0 0 M ωm13 0 0 M ωm12 0 0 M ωm11 0 0 M ωm10 0 0 0 0 0 0 M M M ωm9 ωm8 ωm7 0 0 M ωm6 0 0 M ωm5 0 0 M ωm4 0 ωm3 M ωm3 ωm2 ωm2 M ωm2 ωm1 ωm1 M ωm1 ωm0 ωm0 M ωm0 where the columns dimension of the matrix pertains to the lag length (with the first column the 13th lag and the final column the contemporaneous effect) and the row dimension to the panel corresponds to year. This constraint essentially means that later lags are being identified by variation occurring later in the panel. This specification of the distributed-lag model parallels that of Pakes and Griliches (1984) and Andrews and Fair (1992) in other applications. The model in Equation (1) measures the effects of incarceration on AIDS transmission using variation within sexual relationship markets after netting out race-, age-, and state-specific time trends in both variables. Thus, any unobserved determinants of the incidence of AIDS that vary across but not within sexual relationship markets, or that drive year-to-year changes for specific racial groups, age groups, or states, are accounted for in this model specification. Nonetheless, there may be omitted variables that vary within the remaining slice of variation that we are using to identify the incarceration lag coefficients, such as changes in high risk behavior. Perhaps the strongest contender for a contaminating omitted variable is crack cocaine usage. There is plenty of speculation that the use of crack cocaine during the late 1980s and early 1990s increased the degree of concurrent sexual relationships, both due to pharmacological effects of the drug as well as users prostituting themselves for money to support their habits (Levenson 2004). Moreover, the surge in the use of crack cocaine has been linked to increase in crime as well as increases in various other adverse social trends (Grogger and Willis 2000, Fryer et. al. 2005). To address this issue, we would need to identify instrumental variables that would cause exogenous variation in incarceration rates across groups defined by the four dimensions of our panel. Unfortunately, we were unable to identify such instruments. 18 Nonetheless, there is substantial cross- and within-state variation in various gauges of sentencing and parole reforms that have differentially affected prison growth rates (Reitz 2005). Following the discussion of our dynamic model estimation results, we employ these sentencing reforms to re-estimate a modified version of Equation (1) using a two-stage-least-squares estimator. We discuss this additional estimation strategy in greater detail along with the presentation of the results. IV. Description of the Panel Data Set and Descriptive Statistics To estimate the model discussed in the previous section, we construct a panel data set covering the period 1982 to 1996 that measures the rate of advanced-stage HIV infection7 for sub-populations of the United States as well as a host of same- and cross-gender incarceration rates. The dimensions of the panel are defined by the interactions between the year of diagnosis, the state of residence at the time of diagnosis, age group, racial/ethnic group, and gender. We calculate AIDS infection rates using data from the 2001 CDC AIDS Public Information Data Set (PIDS) as well as the 1980, 1990, and 2000 five percent Public Use Microdata Samples (PUMS) from the U.S. Census of Population and Housing. We calculate incarceration rates using the census data. In this section, we discuss the construction of these variables and the details of our panel data set. Calculating the AIDS infection rate The AIDS Public Information Data Set provides case-level information on all known AIDS cases measured by the national AIDS surveillance system. Since 1985, all states require health service providers to report diagnosed AIDS cases to state and local health departments. In turn, these departments voluntarily report the details of such cases to the CDC.8 Since the onset of the AIDS epidemic, the definition of a case has changed several times. Prior to the ability to identify the HIV antibody, AIDS cases were defined by the presence of a 7 Advanced-stage HIV is commonly referred to as a full-blown AIDS case. Evaluation studies of the completeness of the reporting of AIDS cases has been estimated to be more than 85 percent complete, with the level of reporting completeness varying by geographic area. For a complete discussion, see Rosenblum et. al. (1992). 8 19 disease indicative of a suppressed immune system, such as pneumocystis carinii pneumonia, Kaposi’s sarcoma, and other opportunistic infections. The definition was changed in 1985, reflecting the discovery of HIV as a causative agent of AIDS. The 1985 change was restricted to those with HIV infections and included additional medical conditions. The number of admissible conditions for an AIDS diagnosis was expanded again in 1987 and 1993. The definition of AIDS was expanded to more generally reflect those with HIV infections and measurably-suppressed immune systems. These redefinitions also expanded the number of medical conditions that lead to an AIDS diagnosis for an HIV positive individual. The three redefinitions of an AIDS case increased the likelihood of an AIDS diagnosis independent of actual incidence. The CDC reports that the 1985 redefinition added 3 to 4 percent to total annual new diagnoses, while the 1987 change augmented cases by nearly 25 percent. Similarly, the expanded definition based on a gauge of a suppressed immune system caused a discrete change in reported cases. Moreover, there is evidence that the redefinitions had larger effects on reporting for racial and ethnic minorities and on AIDS cases that were not contracted through male homosexual activity. To control for the effects of these case reporting redefinitions and any other common temporal changes, we include complete controls for year of diagnosis as well as complete sets of race-, age-, and state-specific year effects. Using the AIDS PIDS database, we first tabulate the total number of newly diagnosed AIDS cases by the state of residence, race, age, gender and year of diagnosis for individuals with advanced-stage HIV. We then use data from the 1980, 1990, and 2000 Census PUMS to estimate the national population corresponding to each state/race/age/gender/year cell. For census years, we directly calculate the population with the sample data by summing the provided sample weights within cells. For inter-census years, we linearly interpolate the population using the population estimates for the respective cell for the two census year bracketing the year in 20 question. With these population estimates, we tabulate an AIDS diagnosis rate expressed per 100,000 individuals. This variable is the principal dependent variable of our analysis. An individual’s race/ethnicity is defined by four mutually-exclusive categories nonHispanic white, non-Hispanic black, non-Hispanic Asian, and Hispanic. We use nine of the ten age groupings used to characterize new diagnoses in the AIDS PIDS data, effectively limiting the analysis to AIDS cases among individuals between 20 and 65 years of age.9 The introduction and widespread use of medical therapies, particularly antiretroviral drugs introduced in 1996, have slowed the HIV progression to AIDS. These medical advances since 1996 may have altered and elongated the lagged structure of the relationship between incarceration and AIDS incidence. In light of this fact, our analysis focuses on the period from 1982 to 1996. One problem with the AIDS PIDS data concerns the ability to identify the state of residence at the time of diagnosis for each demographic sub-group of our analysis. Because of confidentiality restrictions due to small cell sizes within some dimensions of our panel (state, race, age, gender, and mode of transmission), roughly 15 percent of AIDS cases observed over this period lack state identifiers. For the levels of disaggregation of AIDS cases required by our analysis, the PIDS identifies the metropolitan area of residence for those individuals residing in large metropolitan areas. This accounts for 85 percent of documented AIDS cases, and includes AIDS cases from 38 states plus Washington, D.C..10 For the remaining 15 percent of documented AIDS cases, the only geographic identifier is the region of residence (defined as west, south, midwest and northeast). Thus, the infection rates in our panel data set are estimated using only 85 percent of the total number of AIDS cases recorded in the U.S. To make use of all cases, we also estimated the models below using the four-category region of residence to define 9 The age ranges describing each infected individual refer to age at infection and are 20 to 24, 25 to 29, 30 to 34, 35 to 39, 40 to 44, 45 to 49, 50 to 54, 55 to 59, 60 to 64, and 65 plus. We drop the 65 plus category since many of those 65 plus in the census defined as institutionalized are in nursing homes. 10 The twelve states with missing disaggregated AIDS case-level information (due to confidentiality restrictions because of small cell sizes) are: Alaska, Iowa, Idaho, Maine, Mississippi, Montana, North Dakota, New Hampshire, South Dakota, Vermont, West Virginia, Wyoming. There are also missing state identifiers for some AIDS cases in small rural areas, disproportionately in the South. 21 geographic location rather than state of residence. The results are qualitatively and numerically similar to what we present below and are available from the authors upon request. Given that the panel spans fifteen years (1982 to 1996) and covers 38 states plus Washington, D.C., the dimensions of the panel define 21,060 individual demographic groups for each gender.11 Figures 1 and 2 present our estimates of the annual newly-diagnosed AIDS cases (expressed per 100,000) for men and women for 1982 through 2000.12 The figure for men reveals that the incidence rate for black men is between three and nine times the comparable rate for white men (with the larger figures pertaining to the latter periods). The rate of new AIDS cases for black women is between 12 and 24 times the annual rate of new diagnoses for white women. For all racial/ethnic groups, the AIDS infection rates for men are generally many times greater than the comparable rates for women. Figures 3 through 6 decompose newly diagnosed AIDS cases per 100,000 by the reported mechanism of transmission for men and for each of the racial/ethnic groups in the panel. Figures 7 through 10 present the comparable decompositions for women. Among white men with AIDS, the large majority of new cases are attributable to transmission through sexual contact (with the lion’s share of this subset attributable to homosexual activity). Intravenous drug use is a significant contributor to infection rates among black men, especially for cases diagnosed during the early 1990s. At the same time, sexual contact is also an important transmission mechanism for black men, and towards the end of the 1990s, it is the dominant mode of transmission. Comparable patterns are observed for Hispanic men. 11 For cells with a positive population estimate and zero new AIDS cases, we set the AIDS infection rate to zero. After omitting those cells where the population estimates from the census are zero, there are 21,018 observations for men and women. 12 For the descriptive statistics in Figures 1 through 10, we use all AIDS cases recorded in the AIDS PIDS data set, since the analysis is at the national level. The model estimates that follow are based on the 85 percent of cases where we can identify the state of residence. 22 For women, transmission through intravenous drug use is consistently a proportionately greater contributor to AIDS infections than it is for men. Nonetheless, a significant proportion of AIDS cases among women are attributable to infections through sexual contact. For both black and white women, roughly twenty percent of cases during the early 1980s are attributable to sexual transmission. This figure increases to over 40 percent during the 1990s. For Hispanic women, sexual transmission accounts for over half of new AIDS cases for several years during the early and mid-1990s. Moreover, closer investigation reveal that during the decade of the 1990s, the largest component of the growth in the disparity in female AIDS infection rates between whites and minorities resulted from infection occurring through heterosexual sex. Calculating Incarceration Rates from the PUMS Estimating Equation (1) requires data on current and lagged incarceration rates for both men and women. Here we first describe how we estimate incarceration rates with data from the U.S. Census. We then describe the lagged structure of our panel data set and the manner in which we calculated the lagged incarceration rates. To estimate the proportion incarcerated for each sub-group of our panel, we make use of the group-quarters identifier included in the PUMS data. The decennial Census enumerates both the institutionalized as well as the non-institutionalized population. The PUMS data for each census includes a flag for the institutionalized as well as micro-level information on age, education, race and all other information available for other non-institutionalized long-form respondents. The group-quarters variable allows one to identify those individuals residing in non-military institutions, a category that includes inmates of federal and state prisons, local jail inmates, residents of inpatient mental hospitals, and residents of other non-aged institutions. We use this variable as our principal indicator of incarceration.13 Raphael (2005) presents a comparison of incarceration estimates from the census to those tabulated by the Bureau of Justice 13 See Butcher and Piehl (1998) for an analysis of incarceration among immigrant men that also uses the group quarter variable to identify the incarcerated. 23 Statistics using alternative data sources and shows that the institutionalized in the decennial census provide a good proxy for the incarcerated population. For the census years 1980, 1990 and 2000, we measure the contemporary incarceration rate for each demographic group defined by state of residence, age group, racial/ethnic group, and gender as the proportion of the members of the demographic cell that is institutionalized. For non-census years, we linearly interpolate the incarceration rate using the estimated rates for the two years bracketing the year in question. Our model requires that we estimate lagged incarceration rates for each demographic group defined by our panel data set. We assume that the AIDS epidemic begins in 1980 and allow for up to 13 lags of the incarceration rate.14 We calculate the lagged incarceration rates in the following manner. First, we redefine the age groupings of our panel to reflect the effect of a time lag. For example, for black women 30 to 34 in New Jersey who are infected in 1990, the one-year lagged incarceration rate should correspond to New Jersey black women that are 29 to 33 in 1989, the two-year lagged incarceration rate should correspond to New Jersey black women that are 28 to 32 in 1988, and so on. Given that the maximum number of lags in our panel is 13 years, we must adjust the age structure 13 times. Next, for each of these 13 additional age structures interacted with the other dimensions of our panel, we estimate the contemporary incarceration rate for each year from 1980 to 2000 using the PUMS. This essentially creates 13 ancillary panel data sets using 13 alternative age groupings. Finally, we match observations from our original panel to the corresponding observations from each of the 13 ancillary panels that gauge the appropriate time lags. For example, using the ancillary panel where the age structure is lagged one year, the 1995 incarceration rates provide the one-year lag for 1996, the 1994 incarceration rate provides the one-year lag for 1995 and so 14 Recall from our methodological discussion above, for any year where lags one through thirteen occur prior to 1980, we constrain the coefficient on that lag for that year to zero. 24 on. Using the ancillary panel where the age structure is lagged two years, the 1994 observations provide the two-year lag for 1996, the 1993 observation provides the two-year lag for 1995, and so on. Each observation in our final data set is matched to 13 lags of the own-gender incarceration rate, where observations with infection years between 1982 and 1992 will have missing values for lags that date prior to 1980. In addition, each observation is matched by year of infection, state of residence, race/ethnicity, and age to the contemporary and lagged incarceration rates for individuals of the opposite gender. Figure 11 presents our estimated incarceration rates for men by race and ethnicity for the period 1982 to 2000. Figure 12 presents the comparable figure for women. There are notable and large differences in incarceration rates between our four mutually exclusive racial/ethnic groups. Over this time period, the incarceration rate for black men increases from roughly 4 percent in 1982 to nearly 9 percent in 2000. Tabulating these figures separately by age reveals even larger increases for younger black men. For black men 20 to 29 years of age, the fraction incarcerated increases from 5.8 to 12.3 percent. For black men 30 to 39 year of age, the comparable figures are 4.4 and 11.1 percent. The increase in incarceration for white men is markedly smaller. Overall, the incarceration rate increases from 0.8 to 1.3 percent between 1982 and 2000. Again, we observe the largest increases for young white men, although the changes are small compared to those for blacks. For white men 20 to 29, the incarceration rate increases from 1.0 to 1.9 percent. The increases for all other age groups are considerably smaller. Changes in incarceration rates for Hispanics are slightly larger than those for whites, though considerably smaller than the change observed for black men. The incarceration rates for men in the “non-Hispanic other” category parallel the results for white men. 25 Incarceration rates are markedly lower for women relative to men for all racial/ethnic groups. The overall racial differences in incarceration parallel those observed for men (black women have the highest rate, followed by Hispanic women and white women), though the magnitude of these differences are very small. Moreover, the increases in incarceration rates for women are minuscule compared to those for men. For example, the proportion of black women incarcerated on any given day increases from 0.5 percent to 0.9 percent over the time period. The incarceration rates among white women and other women actually decline. V. Empirical Results from the Dynamic Regression Models In this section we present various estimates of the dynamic model of AIDS transmission presented in Equation (1). Our goals are two-fold. First, we aim to estimate the overall dynamic relationship between incarceration rates and AIDS infection rates among men and women, for all AIDS cases as well as the special case of AIDS cases transmitted through sexual contact. We focus on the special case of sexually-transmitted HIV/AIDS infections to ensure that the patterns that we observe are not being driven by intravenous drug use alone. Second, we wish to use these results to provide a statistical accounting of the fraction of the racial differences in AIDS infection rates attributable to differences in incarceration rates. Here, we discuss three sets of results that permit an assessment of these two questions. First, we discuss the results from a set of somewhat restrictive specifications that permit assessing the effect of controlling for incarceration on the overall estimate of the racial/ethnic differentials in infection rates. We then present the estimated lag effects of male incarceration from more liberal specifications that allow for sexual relationship market fixed effects and other effects geared at netting out common time trends. Finally, we use the full specification from this second set of regression results to simulate the effect of incarceration on the time-path of blackwhite differences in infection as well as how infection rates would have differed had incarceration rates remained at their 1980 levels. 26 Controlling for incarceration and the overall race/ethnic differences in infection Tables 1 and 2 present some preliminary estimates of the lagged effects of incarceration on AIDS incidence per 100,000 for men and women using a rather restrictive version of the model in Equation (1). Table 1 models the AIDS infection rate for men for both AIDS transmission from any source, and homosexually-contracted AIDS cases. For each dependent variable, the table presents two specifications: (1) a model including race, year, state, and age effects, and (2) a model with all of these fixed effects plus the contemporaneous and thirteen years of lags of the incarceration rate for men and the comparable incarcerations rates for women. To assess the effects of controlling for incarceration on the racial/ethnic differentials in AIDS infection, the table displays the estimated race effects in each model. The female incarceration coefficients are suppressed to conserve space. Regression (1) indicates that over the course of the panel annual black male infection rates exceed annual white male infection rates by over 87 incidents per 100,000 people. Hispanic male infection rates exceed white male rates by 21 per 100,000, while other male infection rates are roughly 35 per 100,000 lower than white male infection rates. Adding the incarceration rates in regression (2) substantially reduces the black-white difference, eliminates the Hispanic-white differences, and slightly widens the other-white difference in infection rates. Concerning the effects of incarceration, the estimates reveal no measurable effects of contemporaneous incarceration rates and lagged effects that are increasing with the time lag. In particular, the lagged incarceration effects become significant at lag year four, reach a maximum at lag year 10, and remain significant through lag year 13. Regressions (3) and (4) reproduce these models where the dependent variable is restricted to homosexually-contracted AIDS incidence. The race effects presented in regression (3) are considerably smaller than the effects presented in regression (1). This is consistent with the fact (revealed in Figure 3 and 4) that transmission through homosexual contact is a proportionally 27 less important avenue of transmission for black men relative to white men. Nonetheless, the average annual infection rates for black men are considerably higher than those for white men (by roughly 29 per 100,000) while the transmission rates for Hispanic men are slightly lower. For the black-white difference, controlling for incarceration reduces the coefficient on the black dummy from roughly 29 to -14, while for the Hispanic-white difference adding incarceration rates widens the negative differential. The lag coefficients on the male incarceration rates parallel those in regression (2) with two important differences. First, the magnitudes of the lag coefficients are considerably smaller (a pattern which is not surprising given our focus on one source of transmission). Second, the contemporaneous incarceration effect is positive. Given that only a small fraction of those who contract HIV develop AIDS within the same year of being infected, any contemporaneous effects are likely to be driven by something other than transmission while incarcerated. For example, a contemporaneous effect may be indicative of an effect of wide-scale testing of the incarcerated on the number of new diagnoses. Table 2 presents comparable regression results for women. Again, the first specification includes race, age, year, and state effects only, while the second specification includes these fixed effects along with the male and female incarceration rate variables. For AIDS cases transmitted by any source, there are large average racial/ethnic differentials in the annual average infection rate. The black-white difference for women is on the order of 31 cases per 100,000, the Hispanic-white differential is approximately 10 per 100,000, while the other-white differential is approximately -3 per 100,000. These absolute differentials are smaller than those observed for men, resulting from the relatively lower infection rates among women. The inclusion of the incarceration rate variables completely eliminates the positive black-white and Hispanic-white differentials in infection rates, while not affecting the other-white differential. Concerning the lag coefficients on male incarceration rates, there is no measurable effect of the 28 contemporaneous incarceration rate and lagged effects that increase monotonically with the lag length. Regressions (3) and (4) present comparable results where the dependent variable is annual AIDS infections contracted through heterosexual sex. Here, the average differentials relative to whites in infection rates are roughly one-third the differentials observed for the models of all AIDS cases. Nonetheless, these differentials are large and significant, with a difference between black and white women of 11 cases per 100,000 and a Hispanic-white difference of 5 per 100,000. Adding the incarceration variables to the specification again eliminates the black-white differential and the Hispanic-white differentials in these variables. In fact, the black-white differential becomes negative and significant, suggesting that holding incarceration rates constant, black women are infected at a lower rate than white women. The shape of the lag function is similar to that observed for the model using the overall AIDS infection rate, although the coefficients are smaller. Allowing for sexual relationship market fixed effects Tables 3 and 4 present estimates of the lagged effects of male incarceration rate on AIDS infection rates using more flexible specifications than those employed in Tables 1 and 2. Table 3 displays estimation results for men and Table 4 presents results for women. The first three models pertain to AIDS infection through any source, while the second three models pertain specifically to sexually-contracted AIDS infection rates (via homosexual contact for men and heterosexual contact for women). For each group of regressions, we present the results from three alternative specifications: (1) a model including the male and female contemporary and lagged incarceration rates, a complete set of fixed effects for race/state/age groups (which we refer to as the sexual relationship market fixed effects), and year effects, (2) a model with the incarceration rate variables, the relationship market fixed effects, and race-specific year effects, 29 and (3) a model with the incarceration rate variables, the relationship market fixed effects, and race-specific, age-specific, and state-specific year effects. We report only the coefficients on the contemporaneous and lagged male incarceration rates. For the overall AIDS infection rate models for men, the parameter estimates of the lag coefficients look very similar to the parameter estimates from the lag coefficients using the somewhat restrictive model in Table 1. There is little evidence of a positive contemporaneous effect of incarceration on male AIDS infections, or of effects of the first three lags. The lag coefficients become positive and significant at the 4th lag, increase through the 10th year (the 11th in the third specification), and decline thereafter. Adding the race-, age-, and state-specific year effects diminishes the magnitude of the coefficients only slightly. The results are similar for homosexually-contracted AIDS infections. Like the results from the restricted models presented in Table 1, we observe a statistically significant contemporaneous incarceration effect, and small or insignificant effects for the first four lags. The lagged effects become somewhat larger and significant for the 5th lag and increase in magnitude through the 10th year. Here, adding the race-, age-, and state-specific year effects reduce the lag coefficients somewhat, although many remain significant and the temporal pattern of the lag function remains the same. The robustness of these results for homosexually- contracted AIDS is particularly suggestive of a link between male incarceration and AIDS, since potential omitted variables such as changes in drug use within relationship markets are not typically associated with increases in homosexual activity. Table 4 presents the comparable results modeling the AIDS infection rates for women. The first three models pertain to overall AIDS infections, while the second three columns pertain to AIDS infection rates where transmission occurs through sexual contact. Again, the models controlling for sexual relationship market fixed effects and the various year effects yield a lag structure that is nearly identical to those from the restrictive models estimated in Table 2. The 30 results from the full specification in regression (3) reveals no significant contemporaneous male incarceration rate effects and lagged effects that increase monotonically in the lag length. The results for heterosexually-contracted infections are qualitatively similar, although the coefficients are smaller in magnitude reflecting the fact that infection through heterosexual sex accounts for at most half of infection among women in any given year. The lag structures revealed in Tables 3 and 4 suggest that the effects of male incarceration on AIDS incidence do not surface for several years and increase considerably over a ten-year period for men and over at least a thirteen-year period for women. Several factors may be driving these delayed responses. For men infected while in prison, infection may not occur immediately inducing a delay between incarceration and the transmission of HIV. In addition, the incubation delay following seroconversion will further add to the lag. For women infected through contact with former inmates, the transmission of the disease must await the release of the inmate and the formation of a heterosexual relationship. Thus, for both men and women, the expected patterns of the lagged effects of incarceration would parallel the incubation distribution of the disease, but with additional delays. In other words, the lag structure should peak later than the peak in the incubation distribution due to factors that cause delay between an increase in incarceration rates and a new HIV infection. To assess whether this is the case, Figures 13 and 14 plot the lagged coefficients from the third specifications of Tables 3 and 4 (the lag effects for the overall AIDS rates from the most complete specification) along with two alternative estimates of the probability distribution functions of the incubation period between seroconversion and the onset of AIDS. The first incubation distribution is calculated using the United Kingdom AIDS registry and pertains to HIV infections in the U.K. occurring prior to 1996 (U.K. Register of Seroconverters Steering 31 Committee 1998).15 The second incubation distribution estimate comes from an analysis of the incubation period among homosexual men in San Francisco during the pre-1996 period (Bachetti 1990). Based on both incubation period distribution estimates, the probability of becoming advanced-stage HIV (following seroconversion) increases in each of years one through seven, reaching a peak likelihood in the seventh year, and declining thereafter. By comparison, the lagged effects of male incarceration on overall AIDS infections for men follow a similar shape, though delayed an additional four years (with a peak at the 11th lag). For women, the delay appears to be greater, as the lag coefficients increase through the thirteen-year period suggesting a maximum effect beyond the lag length allowed in our panel regressions. Simulating the effect of racial differences in incarceration and the post 1980 increase To summarize the results thus far, for both men and women we find significant effects of the time path of male incarceration rates on the rate at which men and women become infected with AIDS. The lagged effects of incarceration increase with time, suggesting significant delays between the incarceration of men and the onset of AIDS for both genders. The temporal pattern of these lagged effects parallel the pre-1996 incubation distribution for the disease with an additional time lag: very small effects early on followed by increasingly larger effects that peak later than previously-estimated peaks in the pre-1996 incubation distribution. The results in Tables 1 and 2 indicate that racial differences in incarceration rates largely explain the sizable overall black-white differential in annual AIDS infection rates. However, there are additional questions to explore that require further analysis and probing of the dynamic model estimates presented in Tables 3 and 4. First, to what extent does adjusting for incarceration rates explain the time path of the racial difference in incarceration rates? Second, to what extent is the increase in incarceration rates since 1980 responsible for the subsequently higher infection rates among African-Americans? 15 The figure in the graph smooths the raw estimate of the pdf reported by the U.K. Register of Seroconverters Steering Committee using a third-order polynomial regression of the infection probability on the time since seroconversion. 32 Answering the first question provides a more detailed decomposition of the racial differentials in infection rates accounting for the changes in incarceration rates over time and the lagged effects of incarceration on AIDS infection rates. In effect, the question asks how the black-white AIDS infection rate differential would have changed over time had the incarceration rates for these two groups been equal. Using the most complete specifications for overall infection rates in Tables 3 and 4 (regression 3 in each table), we calculate the counterfactual black-white difference in AIDS infection rate that would have occurred had black male incarceration rates equaled white male incarceration rates. We do so by subtracting the predicted AIDS differential caused by male differences in incarceration rates from the overall black-white difference in AIDS infection rates.16 Figure 15 displays the actual black-white differential in overall AIDS incidence among men along with the predicted black-white differentials after accounting for black-white differences in male incarceration rates. Figure 16 presents the comparable series for women. Figure 15 reveals that racial differentials in incarceration rates explains little of the racial differentials early on in the epidemic but account for a proportionally increasing share as we progress through the time period of the panel. In the latter years of the panel, racial differences 16 To illustrate this decomposition, here we present a simplified version of Equation (1). Suppose that the AIDS infection rates depends on a set of sexual relationship market fixed effects, and race-, age-, and state-specific year effects, and the contemporaneous incarceration for males only (the decomposition can be easily extended to the dynamic model we estimate in Tables 1 through 4). In other words, we would estimate the equation AIDS rast = α ras + γ rt + δ at + θ st + β MI rast + ε rast . Taking expectations of this equation conditional on race=B and t=t0 and allowing the subscript, Bt0, to denote this conditional expectation gives the expression AIDS Bt0 = α Bt0 + γ Bt0 + δ Bt0 + θ Bt0 + βMI Bt0 , where the first fixed effect is the average network effect for blacks, the second effect is the black time effect for the given year, the following fixed effect is the average age-time effect for blacks, and the remaining provides the average state effect for blacks. If we take a similar expectation for whites and subtract this expectation from that for blacks, we get the final expression AIDS Bt0 − AIDSWt0 = (α Bt0 − α Wt0 ) + (γ Bt0 − γ Wt0 ) + (δ Bt0 − δ Wt0 ) + (θ Bt0 − θ Wt0 ) + β ( MI Bt0 − MI Wt0 ). The first term in the decomposition provides the average black-white differential for the whole panel, the second difference provides the additional period specific difference, the third term provide the differential attributable to difference in the age distribution, while the next term provides the portion of the differential attributable to difference in the geographic distribution. The final term provides the portion of the difference in AIDS infection rates attributable to racial difference in incarceration rates. The tabulations in figures 13 through 16 show the overall unadjusted differential (the left hand side of this equation) as well as the overall differential less the final component of the decomposition due to incarceration. 33 in incarceration rates account for between 70 and 100 percent of the black-white differences in AIDS infection rates. For women, Figure 16 reveals that accounting for the effect of racial differences in male incarceration rates yields negative black-white differentials in overall AIDS infection rates. In other words, the model predicts that if black male incarceration rates had been at the lower level experienced by whites, black women would have been infected with AIDS at a rate that fell short of that for white women between 1982 and 1996. The second question that we ask of our model is somewhat more subtle, and is an attempt to estimate the magnitude of a potential externality of the post-1980 increase in incarceration rates. The substance of this question is best illustrated by the following counterfactual scenario. Suppose, for the moment, that the post-1980 increases in incarceration rates were driven by statelevel policy choices regarding sentencing and parole policy (we discuss these reforms in greater detail below). Had individual states chosen not to implement these reforms (i.e., had held incarceration rates at their 1980 levels), our models would still predict racial differentials in infection rates that would have increased over the first thirteen years of the AIDS epidemic. This counterfactual increase in incarceration rates would be driven by two factors. First, there are substantial racial differences in incarceration rates in 1980, likely reflecting racial differences in the propensity to offend as well as racial discrimination in the criminal justice system (Raphael and Sills 2005). Second, the positive and increasing lagged effects of incarceration on AIDS infections should translate this initial incarceration differential into increasing racial infection differentials. Thus, the introduction of an infectious disease in an environment where blacks are disproportionately incarcerated will generate increasing racial differentials in infection, even in the absence of “tough-on-crime” reforms to the criminal justice system. To the extent that the actual differentials exceed those of this counterfactual scenario, one can infer the extent to which post-1980 policy choices exacerbated racial differentials in AIDS infection rates. 34 We use our models to simulate two sets of black-white differentials in AIDS infection rates. First, we predict the path of the racial differences in AIDS infection rates based on the actual incarceration rates observed between 1982 and 1996. Second, we simulate the counterfactual path in these differentials that would have resulted had incarceration remained at its pre-AIDS epidemic levels (for our purposes as of 1980). We interpret the difference between these two series as the unintended consequences of the increase in incarceration rates on racial inequality in AIDS infections. Figure 17 presents these simulations for men using the most complete specification of the dynamic model of overall AIDS infection rates (model 3 in Table 3). Figure 18 presents the comparable simulations for women. The results for men reveal that the lion’s share of the increase in the black-white differential in AIDS infection rates attributable to incarceration would have occurred in the absence of a post-1980 increase in incarceration. However, the differential would have been lower had incarceration rates not increased. Moreover, the gap between the black-white differential predicted using the actual incarceration rates and the differential predicted holding incarceration constant at 1980 levels increases considerably with time, reaching nearly 60 incidents per 100,000 by the end of the panel. The results for women are comparable. The two simulations yield fairly similar incarceration-induced infection rates during the 1980s. In every year between 1982 and 1996, the predicted black-white differential using 1980 male incarceration rates is less than the predicted differential using historical incarceration rates. However, the disparity between these two series widens significantly over the period, reaching a peak of nearly 33 incidents per 100,000 by 1996. VI. Are These Effects Causal? Thus far, we have documented strong partial correlations between the rate at which men and women become infected with full blown AIDS and lagged values of the incarceration rate 35 for males in one’s demographic group defined by age, race, year, and state of residence. These correlations persist when we focus only on variation occurring within sexual relationship markets over time and after removing race-, age-, and state-specific year-to-year changes in both AIDS infection and incarceration rates. These partial correlations are highly significant and the implied lagged effects of incarceration parallel estimates of the pre-1996 AIDS incubation period distribution. Moreover, the effect sizes suggest that much of the racial differential in AIDS infection rates are attributable to historical differences in the rates at which black men are incarcerated. There are three key additional econometric modeling issues this analysis confronts: (1) the (non-)stationarity of AIDS and prison population data; (2) the stability of the relationship between incarceration and AIDS infection rates over the analysis period (structural change); and (3) unobserved determinants of AIDS infection rates that are correlated with incarceration rates (resulting in omitted variable bias). We consider and discuss aspects of each in turn. We use the annual state-level data to test for unit roots in both the AIDS incidence and incarceration rate time series. It is well known that OLS regressions performed on non- stationary data series can yield spurious results unless the trend is removed by direct subtraction or by differencing. The unit root tests, which include state-specific time trends, show that these series appear to be stationary or I(0) processes. For completeness and as a further check of the robustness of the results, however, we also transform the model and re-estimate it in first-difference form. The first-difference form of the model estimates the annual change in the state’s AIDS incidence rate on 13-year distributed lags of annual changes in the state’s incarceration rate—in other words, the model estimates the effects of an increase in incarceration on the acceleration in the growth of AIDS cases distributed over the subsequent 13-year period. Our first-difference specifications include state fixed effects and use prison data from the Bureau of Justice Statistics. The first-difference results show that 36 an increase in incarceration rate accelerates the growth rate of AIDS infection cases, reaching peak acceleration in years 7 and 8 following the incarceration rate increase. This dynamic structure mirrors with a two-year lag the corresponding growth path of the estimated incubation period distribution of HIV infection to AIDS (in this case, the first derivative of the probability density function or the second derivative of the cumulative density function of the incubation period distribution). The close resemblance of the implied effects of incarceration on AIDS from this alternative model specification lends further support of the hypothesized relationship. (These results are available from the authors upon request). Another potential threat to uncovering unbiased estimates of the effects of incarceration dynamics on AIDS infection rates stems from the fact that the strength of any underlying relationship between the probability of acquiring HIV and the proportion who has ever served time in prison may change as the AIDS epidemic progresses. In particular, we expect any relationship between HIV incidence and prison population size to grow stronger over time, as the prevalence of HIV increases in the population. This resulting lack of stability in the strength of the relationship could lead to biased estimates of the dynamic structure linking incarceration rates and AIDS, since the later lagged incarceration coefficients are identified disproportionately from the most recent observation years on AIDS incidence. We examined the sensitivity of the results to modest changes in the choice of the analysis period and lag length. The results from these checks were not fundamentally altered from the qualitative patterns of results reported in the paper. However, we are more limited in the robustness checks we can perform in this regard. Perhaps the most important caveat to the results above concerns whether we have adequately controlled for all important determinants of AIDS infection rates in our models. Clearly, variation in sexual behavior, as well as variation in the type and/or frequency of drug use, will cause variation in infection rates. While between-group differentials in these behaviors should be captured by our sexual relationship market fixed effects, any changes in behavior that 37 occur over time and within these relationship markets may contaminate the coefficient estimates presented in the previous section. A factor that we do not control for that some have argued helped propagate the AIDS epidemic throughout the black community is the introduction of crack cocaine. Emergency room admission statistics suggests that the use of crack cocaine in American cities began in earnest between 1984 and 1987 (Grogger and Willis 2000); the precise time when HIV infections were on the rise in African-American communities. In his ethnography of the AIDS epidemic in the black community, Levenson (2004) intimates that promiscuity and unprotected sex are integrally related to the crack cocaine trade, as users trade sex for crack or sex for money to buy crack and through a psycho pharmacological effect of the drug itself. Moreover, the introduction of crack cocaine has been linked to a number of negative outcomes, including homicide rates, and infant mortality (see Blumstein 1995, Fryer et. al. 2005). To assess whether the results presented thus far reflect a causal effect of incarceration, we need to identify a source of exogenous variation in prison incarceration rates and then use this variation to identify the causal effect (if any) of incarceration on AIDS infection rates. Towards this end, in this section we present results from TSLS estimates of the effect of incarceration on AIDS infection rates using within-state variation in sentencing policy as instruments for the prison population. We use a set of instrumental variables that characterizes the legal regimes governing criminal sentencing in state courts. Reitz (2004, 2005) has assembled a set of variables characterizing the legal structure within which judges in state courts sentence convicted offenders. Reitz characterizes state sentencing systems as being governed by presumptive guidelines, voluntary guidelines, or traditional indeterminate sentencing systems. Presumptive sentencing guidelines provide a set of predetermined sentencing ranges (a minimum and a maximum) that vary with the severity of criminal offense and the criminal history record of the 38 offender. Judges generally sentence within these guidelines, and judicial departures from presumptive sentencing guidelines must be justified in writing. Voluntary guidelines are, by definition, voluntary and are generally perceived to not constrain judicial discretion in sentencing. Traditional indeterminate sentencing was the dominant sentencing regime in nearly all states prior to the 1970s and remains an important sentencing regime in over half of states today. Indeterminate sentencing regimes are often characterized by complete judicial discretion at the front end of a prison sentence and a fair degree of release discretion afforded to parole boards for early release at the back end of the prison term. In addition to the sentencing regimes governing judicial behavior, Reitz has also assembled data on whether the state has abolished the release authority of parole boards. The abolition of parole boards is generally presumed to increase incarceration rates (see Petersilia 2003), as it constrains a release mechanism that may be used in the event of prisoner overcrowding or to release those who are unlikely to pose a further danger to society. However, Reitz (2004) challenges this presumption based on the reasoning that time off for good behavior or rehabilitative activities can and is incorporated into administrative rules governing parole in mandatory parole states that afford little discretion to a centralized parole board. In the estimates below, we use the two indicators for sentencing regimes (a presumptive guidelines dummy and a voluntary guidelines dummy) interacted with whether the state has abolished parole as instruments for the state prison population in TSLS models of various state-level AIDS infection rates. Unfortunately, these instruments do not vary by all of the dimensions of the panel data set that we use to estimate the models above – i.e., by race, age, year, and state of residence. Adult offenders of all age, race, and gender groups are subject to the same sentencing regime for a given state and year. Moreover, we observe within-state variation in these variables for only thirteen states and for a relatively small number of state-year observations, a fact that makes it 39 difficult to identify the full 13-year lag structure that we estimated above. In light of these constraints, we use a simplified model specification exploiting long differences in cumulative AIDS infections to roughly identify a lagged effect of incarceration on AIDS infection rates. Specifically, we estimate the structural second stage equation, 7 (2) ∑ AIDS i=0 st − i = α s + λ t + β Incarcerat ion st − 7 + χ Admissions st − 7 + δ Re leases st − 7 + ϕ X st − 7 + γ ∆ X st − 7 , st + ε st − 7 , where the dependant variable is the sum of new AIDS cases per 100,000 for each state occurring between years t-7 and t, Incarcerationst-7 is the incarceration rate in state s and year t-7 expressed per 100,000 residents, Admissionsst-7 and Releasesst-7 are the number of prisoner admissions and releases per 100,000 in state s in year t-7, Xst-7 is a vector of demographic control variables for the state in year t-7, and ∆Xst-7,st represents the changes in these variables. We instrument the incarceration rate, prisoner admissions, and prisoner releases using dummy variables indicating presumptive guidelines, voluntary guidelines, and interaction terms between a dummy indicating whether the state has abolished its parole release authority with the two guideline dummy variables. We do not include a base “abolish parole” term, since there are no states that abolish parole without implementing either presumptive or voluntary guidelines. Since we include both state and year effects in the TSLS model, we are identifying the effect of incarceration using within-state variation in the sentencing variables after netting out common year effects. Since we measure incarceration at the state-year level, here we employ state-level prison counts produced by the Bureau of Justice Statistics. Moreover, since data on prison admissions and releases are available at this level of aggregation, we add these flow rates to the specification to test for an independent effect of correlates of prison turnover on AIDS infection rates holding the overall incarceration rate constant.17 These data are also available from the Bureau of Justice Statistics. By lagging the incarceration rate seven years and constructing a dependent variable 17 The results below regarding incarceration are not sensitive to the inclusion of these additional flow variables. 40 equal to the seven-year change in the cumulative number of AIDS cases per 100,000, the coefficient on the incarceration rate reflects the cumulative of the lagged effects through lag year seven of this variable (as well as capturing the effects of previous lags through the correlation between lag year seven incarceration and more distant lagged incarceration rates). Concerning the state-level AIDS infection rates, the AIDS PIDS data set provides a separate set of state-level tabulations of the total number of AIDS cases in each state by year. These state-level totals are provided for all AIDS cases, all AIDS cases by gender, and all AIDS cases by race.18 Before proceeding to the results, we discuss the underlying identification assumption made herein. To identify a causal effect of incarceration on AIDS infection rates, the sentencing variables must influence incarceration rates (be correlated with the incarceration variable) yet influence AIDS rates only through the effect of sentencing reform on incarceration. The latter condition implies that the sentencing reforms must be uncorrelated with any of the unobservable determinants of AIDS infection rates that are swept into the error term of the second-stage equation articulated above. To the extent that the introduction of these sentencing guidelines are driven by changes in sexual behavior or behavior related to drug use, or by the crack epidemic, then our instrumental variables fail the test for exogeneity. While it is difficult to identify the precise motivation behind these sentencing reforms, the extant literature on sentencing guidelines suggests a number of possibilities that have little to do with the crack epidemic. For example, many state efforts to reform their sentencing structure are in response to the “truth-in-sentencing” movement beginning during the mid-1970s devoted to ensuring that sentenced felons ultimately serve an amount of time that reflects the original sentence meted out by the trial judge. In fact, many states had to alter their sentencing regimes to comply with a federal mandate that inmates serve 85 percent of their original sentence, or lose federal funds (Petersilia 2003, Tonry 1998). More generally, the introduction of guidelines and 18 Recall, our AIDS infection rates used above are tabulated with the 85 percent of microdata observations where we can observe the metropolitan area of residence and infer a state of residence. In the TSLS models presented in this section, we are using all AIDS cases to construct the long difference. 41 abolishing of parole release authority can be viewed as attempts to increase the transparency in sentencing and to concentrate the power of the amount of time served into the hands of state sentencing commissions and judges (Reitz 2004). Moreover, these reforms have also been characterized as state efforts to rationalize the state department of corrections and prevent excessive growth in the prison population (Sorensen and Stemen 2002). To the extent that the variation we observe reflects inter-state policy experimentation in response to a common motivation (increase transparency, response to a federal mandate), then the variation in incarceration rates predicted by our instruments should be exogenous. To be sure, future research on this question that employs alternative identification strategies is needed.19 Table 5 presents the first-stage regression results. Since there are three endogenous explanatory variables, there are three first-stage models. Concerning the first-stage results for the incarceration rates, each of the four sentencing reform variables is statistically significant with an F-statistic of the joint significance of these variables equal to 10.0. Presumptive guidelines exert a significant negative effect on incarceration rates, a pattern consistent with previous research (Reitz 2004, Sorensen and Stemen 2002). However, when combined with the abolition of parole release authority, the incarceration rate is predicted to be slightly higher than 19 In addition to employing the sentencing variables as instruments, we also experimented with the prisonerovercrowding law suit variables used by Levitt (1996) in his study of the effect of incarceration rates on crime. Levitt uses state-level law suits and court decisions pertaining to prisoner overcrowding to identify exogenous variation in the prison population and, in turn, identify the crime-prison elasticity. In an alternative model specification, we estimated the second-stage model above where we regressed the long difference in cumulative AIDS cases on the seven-year lag in prison releases (since the Levitt instruments primarily impact the release of prisoners to relieve, under court mandate, overcrowding in prison). In both OLS and TSLS models, we find positive and significant effects of lagged prisoner releases on the long difference in cumulative AIDS cases. Moreover, the magnitude of the effects is comparable in OLS and TSLS. However, it is difficult to interpret these results relative to the primary questions that we seek to answer in this study. We are primarily interested in the effect of the overall incarceration rate on the AIDS infection rate, operating through differential transmission rates in prison, general equilibrium effects on sexual relation networks, and through any other channel. The Levitt instruments permit us to assess the causal effect of releasing inmates a little earlier than they would otherwise be released on overall AIDS infection rates. A positive impact of early release on AIDS infection rates is consistent with both prison increasing the overall AIDS infection rate (by higher transmission behind bars which eventually raises transmission rates when inmates reenter the community) and prison decreasing the overall AIDS infection rate (no higher transmission rate behind bars but prison incapacitates high risk offenders, temporarily preventing them from spreading the disease among members of the non-institutionalized community). Nonetheless, the positive effect of releases on AIDS infection rates using this alternative IV strategy does document the transmission of HIV/AIDS from ex-prisoners to the general community. These additional results are available upon request. 42 that for indeterminate states. Voluntary guidelines independently exert a positive significant effect on incarceration rates. One explanation consistent with these findings is that in voluntary guideline states where the guidelines are essentially non-binding on judicial behavior, judges may face an incentive to exceed the guidelines to establish a record for being tough. However, the abolition of parole release authority appears to moderate prison growth in these states. This may be consistent with judges exercising greater restraint when the back-end release valve operating through a parole board is constrained. The first-stage effects of the sentencing variables on prison admissions and releases are comparable. This is not too surprising considering that the flow into, and out of, prison are strongly correlated with the overall incarceration rate. Table 6 presents OLS and TSLS estimates of Equation (2) for four separate AIDS dependent variables: the change in the cumulative AIDS infection rate among all state residents, all men, all women, and African-Americans. For all models, we generally find positive and significant effects of overall prison population size (per 100,000 residents) in both the OLS and TSLS models and no measurable effects of the admission and release rates. For the overall AIDS infection rate, the predicted marginal effect of an increase in prison population size (per 100,000 residents) is 0.7 compared to the TSLS estimate of 1.4. Both are statistically significant at the one percent level of confidence. The estimated effects of prison population size for the overall AIDS models among males are somewhat larger, with an OLS estimate of 1.1 and a TSLS estimate of 2.22. The comparable estimates for women are 0.3 and 0.6, while the comparable effects for the AIDS infection rates for African-Americans are 0.7 and 2.3. In all models, the positive effects of prison population size are statistically significant at the one percent level of confidence with the exception of the TSLS estimate for black infection rates (the coefficient has a p-value of 0.052). 43 VII. Conclusion The findings of this study are several. We demonstrate a strong positive correlation between increases in incarceration rates occurring among men within narrowly defined demographic groups and corresponding increases in the incidence of new AIDS infection among both men and women. This relationship survives detailed controls for sexual relationship market fixed effects, overall national time trends, and time trends that are specific to age, racial, and state groups. The estimated dynamic relationship between male incarceration and AIDS infections resembles estimates of the probability distribution of the incubation period between seroconversion and the onset of symptomatic AIDS. For men, the lagged effects of incarceration peak two years after the estimated peak in the incubation distribution. For women, the added delay is larger. This is a sensible pattern considering the greater transaction delays that are likely to influence the effect of male incarceration rates on female AIDS infections relative to male infections. Moreover, given the sizable racial differentials in incarceration rates at the beginning of the AIDS epidemic and the increases in these differentials thereafter, our model estimates suggest that the lion’s share of the racial differentials in AIDS infections rates for both men and women are attributable to racial differences in incarceration trends. While our models predict that much of the post-1982 increases in the racial differential in AIDS infection rates would have occurred had incarceration rates remained at the their 1980 levels, increasing relative incarceration rates contributed significantly to the relatively high AIDS infection rates of African-Americans, especially during the 1990s. In a separate analysis, we re-estimate the relationship between male incarceration and AIDS infection rates using within-state variation in sentencing regimes as instruments for statelevel incarceration rates. We find a strong first-stage relationship between our instruments and incarceration rates, and TSLS estimates of the prison-AIDS effects that are positive, statistically 44 significant, and comparable in magnitude to the OLS estimates. While we are unable to reproduce exactly the dynamic specification providing us with our principal model estimate (due to constraints on the dimensions of variation in our instruments), these TSLS results provide evidence that buttress our principal analysis. Exploring the causality of this relationship employing alternative instrumental variables strategies provides a fruitful avenue for future inquiry. While we have focused explicitly on the transmission of HIV/AIDS, the theoretical story being told here as well as the empirical analysis can easily be extended to other communicable diseases that are thought to be transmitted among prisoners. For example, we have cited existing evidence of higher than usual inter-personal transmission of the Hepatitis-B and Hepatitis-C viruses as well as tuberculosis among inmates. In the recent past, there have been media reports of the spread of a streptococcal skin infection from prisoners released from the California state prison system to members of the non-institutionalized public. Given the large numbers of individuals cycling through corrections systems in the United States, the more general issue of how prison is impacting the transmission of communicable diseases broadly defined is clearly an issue in need of further research as well as attention from policy makers. What do these results imply for national and state-level policy debates regarding the optimal level of incarceration? Existing research clearly documents the benefits of prison in terms of crime reduction that extend beyond society’s desire to punish those who transgress the law. However, imprisonment is costly, and some of the costs come in the form of unintended consequences. To assess whether we are at, below, or beyond the optimal level of incarceration, one would need to put a monetary value on the benefits to society in terms of the crime reduction of incarcerating the last offender and compare these benefits to the costs. Donohue (2005) estimates that we are currently incarcerating people at a rate beyond the point where the benefits 45 exceed the costs. Based on an annual per-inmate cost of $46,000 per year, Donohue argues that the optimal incarceration level is roughly 300,000 persons less than the current level. The findings of our study suggest that there are additional costs to society of imprisonment that extend beyond the per-inmate per-year costs of incarceration. These additional costs include the additional medical expenditure for post-release treatment of offenders and the treatment of others who are infected as a result of incarceration, as well as the loss of health and happiness among those affected. While it is difficult to place a monetary value on these factors, they certainly add to overall costs of incarceration, and their incorporation into cost-benefit accounting would certainly lower the optimal incarceration point even further than that estimated by Donohue. Our results suggest that there are large and important unintended health consequences for former offenders and for non-incarcerated members of the communities that disproportionately send young men into the state and federal prison systems. A comprehensive assessment of criminal justice policy in the United States should clearly be taking these considerations into account. 46 References Adimora, Adaora A. and Victor J. Schoenback (2005), “Social Context, Sexual Networks, and Racial Disparities in Rates of Sexually Transmitted Infections,” The Journal of Infectious Diseases, 191(S1): 115-122. Andrews, D.K. and Fair, Ray C. (1992), “Estimation of Distributed Lags and Leads with End Point Constraints,” Journal of Econometrics, 53: 123-139. Ahituv, Avner, V. Joseph Hotz, and Tomas Philipson (1996), “The Responsiveness of the Demand for Condoms to the Local Prevalence of AIDS,” Journal of Human Resources, 31(4): 869-897. Bacchetti, Peter (1990), “Estimating the Incubation Period of AIDS by Comparing Population Infection and Diagnosis Patterns,” Journal of the American Statistical Association, 85(4121) 1002-1018. Baumeister, Roy F. and Kathleen Vohs (2005), “Sexual Economics: Sex as a Female Resource for Social Exchange in Heterosexual Relationships,” unpublished manuscript. Becker, Gary S. (1981), A Treatise on the Family. Cambridge: Harvard University Press. Blumstein, Alfred (1995), “Youth Violence, Guns, and the Illicit Drug Industry,” Journal of Criminal Law and Criminology, 86(1): 10-36. Brewer, TF; Vlahov G.; Taylor, E; Hall, D; Munoz, A; and BF Polk (1988), “Transmission of HIV-1 Within a Statewide Prison System,” AIDS, 2(5): 363-367. Brookmeyer, Ron (1991), “Reconstruction and Future Trends of the AIDS Epidemic in the United States, Science, 253(5015): 37-42. Butcher, Kirsten F. and Anne Morrison Piehl (1998), “Recent Immigrants: Unexpected Implications for Crime and Incarceration,” Industrial and Labor Relations Review, 51(4): 654679. Castro, KG; Conley, LJ; Shansky, R; Scardino, V; Green, T; Narkunas, J; Coe, J; Horsburgh, LR; and T. Hammett (1994), “Report of a Study of HIV Infection in Selected Illinois Correctional Facilities,” unpublished manuscript. Charles, Kerwin K. and Ming Ching Luoh (2005), “Male Incarceration, the Marriage Market and Female Outcomes,” unpublished manuscript. Donohue, John J. (2005), “Fighting Crime: An Economist’s View,” The Milken Institute Review, First Quarters: 46-58. Freudenberg, Nicholas (2001), “Jails, Prisons, and the Health of Urban Populations: A Review of the Impact of the Correctional System on Community Health,” Journal of Urban Health 78(2): 214-235. 47 Fryer, Roland G; Heaton, Paul S.; Levitt, Steven D. and Kevin M. Murphy (2005), “Measuring the Impact of Crack Cocaine,” National Bureau of Economic Research Working Paper #11318 Grogger, Jeffrey and Michael Willis (2000), “The Emergence of Crack Cocaine and the Rise in Urban Crime Rates,” Review of Economics and Statistics, 82(4): 519-529. Hammett, Theodore M.; Harmon, Mary Patricia and William Rhodes (2002), “The Burden of Infectious Disease Among Inmates of and Releasees from US Correctional Facilities, 1997,” American Journal of Public Health, 92(11): 1789-1794. Hammett, Theodore M. (2004), “Transmission of HIV and other Infectious Diseases in Correctional Facilities,” unpublished manuscript. Horsburgh, LR; Jarvis, JQ; McCarthur, T; Ignacio, T and P. Stock (1990), “Seroconversion to HIV in Prison Inmates,” American Journal of Public Health, 80(2): 209-210. Kilmarx, PH; Zaidi, AA; and Thomas, JC (1997), “Ecological Analysis of Sociodemographic Factors and the Variation in Syphilis Rates Among Counties in the United States, 1984 to 1993,” American Journal of Public Health, 87: 1937-1943. Krebs, Christopher P. (2002), “High-Risk HIV Transmission Behavior in Prison and the Prison Sub-Culture,” The Prison Journal, 82(1): 19-49. Krebs, Christopher P. and Melanie Simmons (2002), “Intraprison HIV Transmission: An Assessment of Whether it Occurs, How it Occurs, and Who is at Risk,” AIDS Education and Prevention, 14(Supplement B): 53-64. Kremer, Michael (1996), “Integrating Behavioral Choice into Epidemiological Models of AIDS,” Quarterly Journal of Economics, 11(2): 549-573. Laumann, EO; Gagnon, JH; Michael, RT; and S. Michaels (1994), The Social Organization of Sexuality, Chicago: University of Chicago Press. Levenson, Jacob (2004), The Secret Epidemic: The Story of AIDS and Black America, New York: Anchor Books. Levitt, Steven D. (1996), “The Effect of Prison Population Size on Crime Rates: Evidence from Prison Overcrowding Litigation,” Quarterly Journal of Economics, 111(2): 319-351. Macalino, Grace R.; Vlahov, David; Sanford-Colby, Stephanie; Patel, Sarju; Sabin, Keith; Salas, Kristopher and Josiah D. Rich (2004), “Prevalence and Incidence of HIV, Hepatitis B Virus, and Hepatitis C Virus Infections Among Males in Rhode Island Prisons,” American Journal of Public Health, 94(7): 1218-1223. Morris, Martina and Mirjam Kretzchmar (1995), “Concurrent Partnerships and Transmission Dynamics in Networks,” Social Networks, 17: 299-318. Morris, Martina and Mirjam Kretzchmar (1996), “Measures of Concurrency in Networks and the Spread of Infectious Disease,” Mathematical Biosciences 133: 165-195. 48 Mutter, RC; Grimes, RM and D. Labarthe (1994), “Evidence of Intraprison Spread of HIV Infection,” Archives of Internal Medicine, 154(7): 793-795. Pakes, Ariel and Zvi Griliches (1984), “Estimating Distributed Lags in Short Panels with an Application to the Specification of Depreciation Patterns and Capital Stock Constructs,” Review of Economic Studies, 51: 243-262. Petersilia, Joan (2003), When Prisoners Come Home: Parole and Prisoner Reentry, Oxford: Oxford University Press. Philipson, T., and R. Posner. 1993. Private Choices and Public Health: An Economic Interpretation of the AIDS Epidemic. Cambridge: Harvard University Press. Raphael (2005), “The Socioeconomic Status of Black Males: The Increasing Importance of Incarceration,” forthcoming in Auerbach, Alan J; Card, David and John M. Quigley (eds.) Poverty, the Distribution of Income, and Public Policy, New York: Russell Sage Foundation. Raphael and Sills (2005), “Urban Crime in the United States,” forthcoming in Richard Arnott and Dan McMillan (eds.) Companion to the Handbook of Urban Economics. Raphael, Steven and Michael A. Stoll (2005), “The Effect of Prison Releases on Regional Crime Rates,” in William G. Gale and Janet Rothenberg-Pack (eds.) The Brookings-Wharton Papers on Urban Economic Affairs, Volume 5, Washington, D.C.: The Brookings Institution. Reitz (2004), “Questioning the Conventional Wisdom of Parole Release Authority,” in Michael Tonry (editor), The Future of Imprisonment, New York: Oxford University Press, pp. 199-236. Reitz, Kevin R. (2005), “The New Sentencing Conundrum: Policy and Constitutional Law at Cross-Purposes,” Columbia Law Review, 105: 1082-1123. Rosenblum, L; Buehler, JW; Morgan, MW; Costa, S; Hidalgo, J; Holmes, R; Lieb, L; Shields, A and BM Whytle (1992), “The Completeness of AIDS Case Reporting, 1988: A Multisite Collaborative Surveillance Project,” American Journal of Public Health, 82: 1495-1499. Sampson, RJ (1995), “Unemployment and Imbalanced Sex Ratios: Race-Specific Consequences for Family Structure and Crime,” in Tucker, MB and C. Mitchell-Kern (eds.), The Decline in Marriage Among African-Americans: Causes, Consequences, and Policy Implications, New York: Russell Sage Foundation: pp. 229-260. Sorensen, Jon and Don Stemen (2002), “The Effect of State Sentencing Policies on Incarceration Rates,” Crime & Delinquency, 48(3): 456-475. Swartz, James E; Lurigio, Arthur J. and Dana Aron Weiner (2004), “Correlates of HIV Risk Behavior Among Prison Inmates: Implications for Tailored AIDS Prevention Programming,” The Prison Journal, 84(4): 486-504. Tewksbury, R. (1989), “Measures of Sexual Behavior in an Ohio Prison,” Sociology and Social Research, 74(1): 34-39. 49 Thomas, James C. and Mary E. Gaffield (2003), “Social Structure, Race, and Gonorrhea Rates in the Southeastern United States,” 13(3): 362-368. Tonry, Michael (1998), Sentencing Matters, Oxford: Oxford University Press. U.K. Register of HIV Seroconverters Steering Committee (1998), “The AIDS Incubation Period in the U.K. Estimates from a National Register of HIV Seroconverters,” AIDS, 12(6): 659-667/ Wooden, W. and J. Parker (1982), Men Behind Bars: Sexual Exploitation in Prison, New York: Plenum. World Health Organization (2001), HIV in Prison: A Reader with Particular Relevance to the Newly Independent States, World Health Organization. 50 Figure 1 Annual Newly-Diagnosed AIDS Cases Per 100,000 Men Age 20t to 64 by Race/Ethnicity, 1982 to 2001 300 AIDS Cases per 100,000 250 200 Non-Hispanic White Non-Hispanic Black 150 Hispanic Non-Hispanic Other 100 50 0 1982 1983 1984 1985 1986 1987 1988 1989 1990 1991 1992 1993 1994 1995 1996 1997 1998 1999 2000 2001 Figure 2 Annual Newly-Diagnosed AIDS Case Per 100,000 Women Age 20 to 64 by Race/Ethnicity, 1982 to 2001 100 90 80 AIDS Cases per 100,000 70 60 Non-Hispanic White Non-Hispanic Black 50 Hispanic Non-Hispanic Other 40 30 20 10 0 1982 1983 1984 1985 1986 1987 1988 1989 1990 1991 1992 1993 1994 1995 1996 1997 1998 1999 2000 2001 51 Figure 3 Distribution of Newly-Diagnosed AIDS Cases Per 100,000 White Men Age 20 to 64 by Transmission Mechanism, 1982 to 2001 70 60 AIDS Cases per 100,000 50 Cause Unreported 40 Medical Procedure Intravenious Drug Use Sexual Contact 30 20 10 0 1982 1983 1984 1985 1986 1987 1988 1989 1990 1991 1992 1993 1994 1995 1996 1997 1998 1999 2000 2001 Figure 4 Distribution of Newly-Diagnosed AIDS Cases Per 100,000 Black Men Age 20 to 64 by Transmission Mechanism, 1982 to 2001 300 AIDS Cases per 100,000 250 200 Cause Unreported Medical Procedure 150 Intravenious Drug Use Sexual Contact 100 50 0 1982 1983 1984 1985 1986 1987 1988 1989 1990 1991 1992 1993 1994 1995 1996 1997 1998 1999 2000 2001 52 Figure 5 Distribution of Newly Diagnosed AIDS Cases Per 100,000 Hispanic Men Age 20 to 64 by Transmission Mechanism, 1982 to 2001 180 160 AIDS Cases per 100,000 140 120 Cause Unreported 100 Medical Procedure Intravenious Drug Use Sexual Contact 80 60 40 20 0 1982 1983 1984 1985 1986 1987 1988 1989 1990 1991 1992 1993 1994 1995 1996 1997 1998 1999 2000 2001 Figure 6 Distribution of Newly Diagnosed AIDS Cases Per 100,000 Non-Hispanic Other Men Age 20 to 64 by Transmission Mechanism, 1982 to 2001 30 AIDS Cases per 100,000 25 20 Cause Unreported Medical Procedure 15 Intravenious Drug Use Sexual Contact 10 5 0 1982 1983 1984 1985 1986 1987 1988 1989 1990 1991 1992 1993 1994 1995 1996 1997 1998 1999 2000 2001 53 Figure 7 Distribution of Newly-Diagnosed AIDS Cases Per 100,000 White Women Age 20 to 64 by Transmission Mechanism, 1982 to 2001 6 AIDS Cases per 100,000 5 4 Cause Unreported Medical Procedure 3 Intravenious Drug Use Sexual Contact 2 1 0 1982 1983 1984 1985 1986 1987 1988 1989 1990 1991 1992 1993 1994 1995 1996 1997 1998 1999 2000 2001 Figure 8 Distribution of Newly-Diagnosed AIDS Cases Per 100,000 Black Women Age 20 to 64 by Transmission Mechanism, 1982 to 2001 100 90 80 AIDS Cases per 100,000 70 60 Cause Unreported Medical Procedure 50 Intravenious Drug Use Sexual Contact 40 30 20 10 0 1982 1983 1984 1985 1986 1987 1988 1989 1990 1991 1992 1993 1994 1995 1996 1997 1998 1999 2000 2001 54 Figure 9 Distribution of Newly-Diagnosed AIDS Cases Per 100,000 Hispanic Women Age 20 to 64 by Transmission Mechanism, 1982 to 2001 45 40 AIDS Cases per 100,000 35 30 Cause Unreported 25 Medical Procedure Intravenious Drug Use Sexual Contact 20 15 10 5 0 1982 1983 1984 1985 1986 1987 1988 1989 1990 1991 1992 1993 1994 1995 1996 1997 1998 1999 2000 2001 Figure 10 Distribution of Newly-Diagnosed AIDS Cases Per 100,000 Non-Hispanic Other Women Age 20 to 64 by Transmission Mechanism, 1982 to 2001 3.5 3 AIDS Cases per 100,000 2.5 Cause Unreported 2 Medical Procedure Intravenious Drug Use Sexual Contact 1.5 1 0.5 0 1982 1983 1984 1985 1986 1987 1988 1989 1990 1991 1992 1993 1994 1995 1996 1997 1998 1999 2000 2001 55 Figure 11 Overall Male Incarceration Rates by Race/Ethnicity, 1982 to 2001 0.100 0.090 0.080 Proportion Incarcerated 0.070 0.060 Non-Hispanic White Non-Hispanic Black 0.050 Non-Hispanic Other Hispanic 0.040 0.030 0.020 0.010 0.000 1982 1983 1984 1985 1986 1987 1988 1989 1990 1991 1992 1993 1994 1995 1996 1997 1998 1999 2000 2001 Figure 12 Overall Female Incarceration Rates by Race/Ethnicity, 1982 to 2001 0.090 0.080 0.070 Proportion Incarcerated 0.060 Non-Hispanic White 0.050 Non-Hispanic Black Non-Hispanic Other Hispanic 0.040 0.030 0.020 0.010 0.000 1982 1983 1984 1985 1986 1987 1988 1989 1990 1991 1992 1993 1994 1995 1996 1997 1998 1999 2000 2001 56 Figure 13 Impact of Male Incarceration on AIDS among Males 0 2 4 6 8 % Become Advanced-stage AIDS (Based on Incubation Dist Estimates) Annual Male AIDS cases per 100,000 Dist Lag Incarc Efx on AIDS 0 2 4 6 Based on 13-yr Distributed Lag Model w/Sex Mkt Fixed Effects(1982-1996) 1 2 3 4 5 6 7 t... 8 9 10 11 12 13 # of Years after Male Incarceration-induced shock to Sex Market Dist Lag Incarc Efx on AIDS predicted Incubation_Dist_pdf_UK Incubation Dist-pdf (SF) Figure 14 Impact of Male Incarceration on AIDS among Females 0 2 4 6 8 % Become Advanced-stage AIDS (Based on Incubation Dist Estimates) Annual Female AIDS cases per 100,000 Dist Lag Incarc Efx on AIDS .5 1 1.5 2 2.5 Based on 13-yr Distributed Lag Model w/Sex Mkt Fixed Effects(1982-1996) 1 2 3 4 5 6 7 t... 8 9 10 11 12 13 # of Years after Male Incarceration-induced shock to Sex Market Dist Lag Incarc Efx on AIDS predicted Incubation_Dist_pdf_UK 57 Incubation Dist-pdf (SF) Figure 15 Actual Black-White Differences in Overall AIDS Infection Rates for Men and the Black-White Difference After Accounting for Male Incarceration Rates 200 Black-White Difference in AIDS Incidence per 100,000 180 160 140 120 Actual Differential 100 Differential after accounting for differences in male incarceration rates 80 60 40 20 0 1982 1983 1984 1985 1986 1987 1988 1989 1990 1991 1992 1993 1994 1995 1996 Figure 16 Actual Black-White Difference in Overall AIDS Infection Rates for Women and the Black-White Difference After Accounting for Male Incarceration Rates 80 Black-White Difference in AIDS Incidence per 100,000 70 60 50 40 30 Actual differential 20 Differential after accounting for differences in male incarceration rates 10 0 1982 1983 1984 1985 1986 1987 1988 1989 1990 1991 1992 1993 1994 1995 1996 -10 -20 -30 58 Figure 17 Simulated Black-White Differentials in Overall AIDS Infection Rates for Men, Allowing Incarceration to Pursue its Historical Path and Constraining Incarceration Rates to 1980 Levels 180 Black-White Difference in AIDS Incidence per 100,000 160 140 120 100 Predicted difference, actual incaceration rate Predicted differential, 1980 incarceration rate 80 60 40 20 0 1982 1983 1984 1985 1986 1987 1988 1989 1990 1991 1992 1993 1994 1995 1996 Figure 18 Simulated Black-White Differential in Overall AIDS Infection Rates for Women, Allowing Male Incarceration to Pursue its Historical Path and Constraining Incarceration Rates to 1980 Levels 100 Black-White Differences in AIDS Incidence per 100,000 90 80 70 60 Predicted difference, actual incaceration rate 50 Predicted differential, 1980 incarceration rate 40 30 20 10 0 1982 1983 1984 1985 1986 1987 1988 1989 1990 1991 1992 1993 1994 1995 1996 59 Table 1: Regression Models Examining the Role of Male Incarceration Rates and Overall Racial/Ethnic Differences in AIDS Infection Rates Among Men AIDSrsat (any source) Variables Black (ref cat. White) Hispanic Asian (1) (2) 87.3739*** (2.8102) 20.9899*** (2.8613) -35.0814*** (1.5153) 10.9101*** (3.2807) 0.4095 (2.6441) -35.4066*** (1.3630) Homosexually-contracted AIDSrsat (3) (4) 28.8359*** (1.1620) -3.4704** (1.6813) -31.3973*** (1.2082) -14.7595*** (1.3481) -13.9691*** (1.5089) -30.3543*** (1.1359) Male Incarceration Ratersat -0.8050 (0.7792) 2.1212*** (0.2124) Male Incarceration Ratersat-1 -0.8211*** (0.2546) 0.7073*** (0.0937) Male Incarceration Ratersat-2 -0.3940 (0.3885) -0.0088 (0.1070) Male Incarceration Ratersat-3 0.3732 (0.4850) -0.1660 (0.1229) Male Incarceration Ratersat-4 1.3770*** (0.4303) 0.0969 (0.1102) Male Incarceration Ratersat-5 2.5143*** (0.3045) 0.6407*** (0.0906) Male Incarceration Ratersat-6 3.6818*** (0.2855) 1.3266*** (0.1016) Male Incarceration Ratersat-7 4.7762*** (0.4393) 2.0156*** (0.1410) Male Incarceration Ratersat-8 5.6942*** (0.5940) 2.5686*** (0.1762) Male Incarceration Ratersat-9 6.3326*** (0.6453) 2.8468*** (0.1842) Male Incarceration Ratersat-10 6.5881*** (0.5419) 2.7112*** (0.1526) Male Incarceration Ratersat-11 6.3573*** (0.3933) 2.0228*** (0.1091) Male Incarceration Ratersat-12 5.5372*** (0.8447) 0.6426*** (0.2222) Male Incarceration Ratersat-13 4.0243** -1.5683*** (1.8953) (0.4960) Year controls? yes Yes yes yes State controls? yes Yes yes yes Age group controls? yes Yes yes yes Observations 21,060 21,018 21,060 21,018 Bootstrapped standard errors in parentheses; * significant at 10%; ** significant at 5%; *** significant at 1% Columns 2 and 4 estimate constrained 13-yr distributed lag models, using a third-order polynomial to represent the lag weights. These models include the same series of lagged female incarceration rates as shown for men-coefficient estimates on these variables are suppressed in the Table. All regressions are weighted by cell frequency. 60 Table 2: Regression Models Examining the Role of Male Incarceration Rates and Overall Racial/Ethnic Differences in AIDS Infection Rates Among Women Variables Black (ref cat. White) Hispanic Asian AIDSrsat (any source) (1) (2) 31.0285*** (1.5282) 10.1768*** (1.0101) -2.5483*** (0.3482) -5.1316*** (1.1888) 0.4197 (0.7569) -2.4078*** (0.4415) Heterosexually-contracted AIDSrsat (3) (4) 10.9711*** (0.5100) 4.6943*** (0.3480) -0.8652*** (0.1101) -4.0420*** (0.5189) 0.8141** (0.4014) -0.6524*** (0.1497) Male Incarceration Ratersat -0.0907 (0.3371) 0.2204** (0.1094) Male Incarceration Ratersat-1 0.2442*** (0.0883) 0.2734*** (0.0372) Male Incarceration Ratersat-2 0.5367*** (0.1334) 0.3136*** (0.0463) Male Incarceration Ratersat-3 0.7912*** (0.1867) 0.3446*** (0.0618) Male Incarceration Ratersat-4 1.0123*** (0.1762) 0.3696*** (0.0600) Male Incarceration Ratersat-5 1.2047*** (0.1297) 0.3922*** (0.0484) Male Incarceration Ratersat-6 1.3731*** (0.1036) 0.4159*** (0.0426) Male Incarceration Ratersat-7 1.5219*** (0.1460) 0.4440*** (0.0536) Male Incarceration Ratersat-8 1.6558*** (0.2021) 0.4800*** (0.0700) Male Incarceration Ratersat-9 1.7795*** (0.2267) 0.5274*** (0.0785) Male Incarceration Ratersat-10 1.8975*** (0.2069) 0.5896*** (0.0734) Male Incarceration Ratersat-11 2.0145*** (0.2071) 0.6702*** (0.0660) Male Incarceration Ratersat-12 2.1351*** (0.3995) 0.7724*** (0.1078) Male Incarceration Ratersat-13 2.2638*** 0.8999*** (0.7970) (0.2174) Year controls? yes Yes yes yes State controls? yes Yes yes yes Age group controls? yes Yes yes yes Observations 21,051 21,018 21,051 21,018 Bootstrapped standard errors in parentheses; * significant at 10%; ** significant at 5%; *** significant at 1% Columns 2 and 4 estimate constrained 13-yr distributed lag models, using a third-order polynomial to represent the lag weights. These models include the same series of lagged female incarceration rates as shown for men-coefficient estimates on these variables are suppressed in the Table. All regressions are weighted by cell frequency. 61 Table 3: Regression Models Estimating the Effect of Male Incarceration Rates on AIDS Infection Rates Among Men, Complete Specification AIDSrsat (any source) Variables (1) (2) Homosexually-contracted AIDSrsat (3) (4) (5) (6) Male Incarceration Ratersat -2.2868*** -3.6826*** 0.7772 1.2361*** 1.6494*** (0.6770) (0.7475) (0.6464) (0.3920) (0.4357) 1.7982*** (0.3220) Male Incarceration Ratersat-1 -1.9605*** -2.8746*** 0.0689 (0.3329) (0.3606) (0.3124) 0.3691* (0.2102) 0.8659*** (0.1556) Male Incarceration Ratersat-2 -1.2239*** -1.8544*** -0.1575 -0.4241*** (0.2020) (0.2412) (0.2114) (0.1170) -0.2148 (0.1406) 0.3816*** (0.1053) -0.2466 (0.1618) 0.2551** (0.1203) 0.1294 (0.1678) 0.3961*** (0.1235) 0.0793 (0.1928) Male Incarceration Ratersat-3 -0.1876 (0.2223) Male Incarceration Ratersat-4 1.0382*** (0.2352) Male Incarceration Ratersat-5 2.3430*** 1.7876*** 1.2128*** 0.6218*** 0.7690*** (0.2096) (0.2501) (0.2136) (0.1214) (0.1457) 0.7143*** (0.1064) Male Incarceration Ratersat-6 3.6165*** 2.9645*** 2.0720*** 1.3673*** 1.5278*** (0.1695) (0.1973) (0.1701) (0.0981) (0.1150) 1.1194*** (0.0847) Male Incarceration Ratersat-7 4.7483*** 4.0075*** 2.9922*** 2.0839*** 2.2617*** (0.1578) (0.1855) (0.1663) (0.0914) (0.1081) 1.5211*** (0.0829) Male Incarceration Ratersat-8 5.6279*** 4.8474*** 3.8891*** 2.6349*** 2.8264*** (0.1870) (0.2266) (0.2060) (0.1083) (0.1321) 1.8292*** (0.1026) Male Incarceration Ratersat-9 6.1450*** 5.4149*** 4.6785*** 2.8839*** 3.0776*** (0.2166) (0.2652) (0.2393) (0.1254) (0.1546) 1.9532*** (0.1192) Male Incarceration Ratersat-10 6.1892*** 5.6407*** 5.2763*** 2.6944*** 2.8710*** (0.2098) (0.2569) (0.2301) (0.1215) (0.1497) 1.8029*** (0.1147) Male Incarceration Ratersat-11 5.6502*** 5.4557*** 5.5983*** 1.9298*** 2.0625*** (0.1654) (0.2006) (0.1848) (0.0958) (0.1169) 1.2880*** (0.0920) Male Incarceration Ratersat-12 4.4174*** 4.7905*** 5.5602*** 0.4537*** 0.5077*** (0.2241) (0.2702) (0.2640) (0.1298) (0.1575) 0.3182** (0.1315) Male Incarceration Ratersat-13 2.3805*** 3.5760*** 5.0778*** -1.8704*** -1.9376*** (0.5145) (0.6252) (0.5896) (0.2979) (0.3644) yes yes yes yes yes -1.1968*** (0.2937) yes Year controls? -0.6911** 0.0138 -0.4104*** (0.2776) (0.2414) (0.1287) 0.5459* (0.2879) 0.4986** (0.2478) -0.0163 (0.1362) Sex Market Fixed Effect: Race*State*AgeGroup yes yes yes yes yes yes Race-specific Year Effect: Year*Race no yes yes no yes yes Age group-specific Year Effect: Year*AgeGroup State-specific Year Effect: Year*State Observations no no yes no no yes no no yes no no yes 21,018 21,018 21,018 21,018 21,018 21,018 Standard errors in parentheses; * significant at 10%; ** significant at 5%; *** significant at 1% Columns 1-6 estimate constrained 13-yr distributed lag models, using a third-order polynomial to represent the lag weights. These models include the same series of lagged female incarceration rates as shown for men-coefficient estimates on these variables are suppressed in the Table. All regressions are weighted by cell frequency. 62 Table 4: Regression Models Estimating the Effect of Male Incarceration Rates on AIDS Infection Rates Among Women, Complete Specification AIDSrsat (any source) Variables (1) (2) Heterosexually-contracted AIDSrsat (3) (4) (5) (6) 0.0703 (0.2600) 0.0162 (0.1023) -0.9686*** (0.1124) -0.0182 (0.1090) Male Incarceration Ratersat -0.9744*** -2.4443*** (0.2436) (0.2710) Male Incarceration Ratersat-1 -0.2524** (0.1202) -0.8958*** 0.5001*** (0.1307) (0.1256) 0.2173*** (0.0504) -0.2536*** (0.0542) 0.2664*** (0.0527) Male Incarceration Ratersat-2 0.3178*** (0.0720) 0.2354*** (0.0865) 0.8317*** (0.0842) 0.3522*** (0.0302) 0.2494*** (0.0359) 0.4659*** (0.0353) Male Incarceration Ratersat-3 0.7553*** (0.0787) 1.0077*** (0.0995) 1.0802*** (0.0961) 0.4329*** (0.0331) 0.5705*** (0.0413) 0.5926*** (0.0403) Male Incarceration Ratersat-4 1.0795*** (0.0836) 1.4795*** (0.1035) 1.2611*** (0.0990) 0.4717*** (0.0351) 0.7399*** (0.0429) 0.6585*** (0.0415) Male Incarceration Ratersat-5 1.3096*** (0.0748) 1.7092*** (0.0901) 1.3894*** (0.0856) 0.4807*** (0.0314) 0.7876*** (0.0374) 0.6755*** (0.0359) Male Incarceration Ratersat-6 1.4650*** (0.0606) 1.7553*** (0.0711) 1.4804*** (0.0681) 0.4722*** (0.0254) 0.7437*** (0.0295) 0.6558*** (0.0286) Male Incarceration Ratersat-7 1.5650*** (0.0560) 1.6762*** (0.0665) 1.5494*** (0.0662) 0.4582*** (0.0235) 0.6383*** (0.0276) 0.6114*** (0.0277) Male Incarceration Ratersat-8 1.6289*** (0.0659) 1.5303*** (0.0810) 1.6116*** (0.0817) 0.4511*** (0.0277) 0.5016*** (0.0336) 0.5543*** (0.0342) Male Incarceration Ratersat-9 1.6760*** (0.0763) 1.3760*** (0.0949) 1.6824*** (0.0949) 0.4629*** (0.0320) 0.3636*** (0.0393) 0.4966*** (0.0398) Male Incarceration Ratersat-10 1.7255*** (0.0741) 1.2718*** (0.0920) 1.7768*** (0.0915) 0.5058*** (0.0311) 0.2543*** (0.0382) 0.4504*** (0.0384) Male Incarceration Ratersat-11 1.7968*** (0.0585) 1.2761*** (0.0720) 1.9103*** (0.0736) 0.5920*** (0.0246) 0.2040*** (0.0298) 0.4276*** (0.0308) Male Incarceration Ratersat-12 1.9092*** (0.0790) 1.4474*** (0.0967) 2.0979*** (0.1046) 0.7337*** (0.0332) 0.2427*** (0.0401) 0.4404*** (0.0439) Male Incarceration Ratersat-13 2.0819*** (0.1813) yes 1.8439*** (0.2237) yes 2.3551*** (0.2336) yes 0.9431*** (0.0761) yes 0.4004*** (0.0928) yes 0.5007*** (0.0979) yes Year controls? Sex Market Fixed Effect: yes yes yes yes yes Race*State*AgeGroup Race-specific Year Effect: no yes yes no yes Year*Race Age group-specific Year Effect: no no yes no no Year*AgeGroup State-specific Year Effect: no no yes no no Year*State Observations 21,018 21,018 21,018 21,018 21,018 Standard errors in parentheses; * significant at 10%; ** significant at 5%; *** significant at 1% yes yes yes yes 21,018 Columns 1-6 estimate constrained 13-yr distributed lag models, using a third-order polynomial to represent the lag weights. These models include the same series of lagged female incarceration rates as shown for men--coefficient estimates on these variables are suppressed in the Table. All regressions are weighted by cell frequency. 63 Table 5: First-Stage Results from Regressions of Prison Incarceration Rates, Prison Admission Rates, and Prison Release Rates on State Sentencing Reform Variables and Other Controls Prison Incarceration Prisoner Admission Prisoner Release Rate Rate Rate Presumptive Guidelines -73.358*** -46.471*** -49.083*** (19.303) (14.912) (15.474) Presumptive Guidelines 87.624*** 56.408*** 64.814*** *Abolished Parole (23.937) (18.493) (19.190) Voluntary Guidelines 98.032*** 16.023 21.130 (20.643) (15.949) (16.550) Voluntary Guidelines -99.722*** -56.032*** -50.712*** *Abolished Parole (26.821) (20.736) (21.518) a 10.00 4.56 4.37 F-Statistic (P-value) (<0.0001) (0.0012) (0.0017) N 896 896 896 *. Significant at 10%; ** significant at 5%; *** significant at 1%. Standard errors are in parentheses. Each regression includes a complete set of state and time fixed effects, controls for the percent black, the percent of the population between 18 to 24, 25 to 44, 45 to 64 and 65 and over, the proportion residing in metropolitan areas, and the proportion in poverty. The regression also include controls for the seven year changes in these variables between the year corresponding to the incarceration rate and the final year of the long difference in the cumulative AIDS infection rates. The sample is restricted to the incarceration rates in the years 1977 to 1994. This corresponds to long differences in the AIDS infection rates with an end year ranging from 1984 through 2001. a. This provides the F-statistics from a test of the joint significance of the four sentencing variables in each regression. 64 Table 6: OLS and Two-Stage Least Squares Estimates of the Effect of Incarceration Rates and New AIDS Infections Explanatory Variables Prison Incarceration Prison Admission Prison Release Rate Dependent Rate Rate Variable and Estimation Method Total AIDS Infection Rate OLS 0.689*** 0.087 -0.180* (0.034) (0.106) (0.095) TSLS 1.418*** -1.481 0.017 (0.389) (2.048) (2.343) Male AIDS Infection Rate OLS 1.106*** 0.065 -0.387** (0.057) (0.173) (0.155) TSLS 2.222*** -2.759 0.334 (0.617) (3.246) (3.715) Female AIDS Infection Rate OLS 0.275*** 0.105** 0.029 (0.015) (0.046) (0.042) TSLS 0.607*** -0.268 -0.217 (0.177) (0.931) (1.066) Black AIDS Infection Rate OLS 0.676*** 0.447** -0.205 (0.065) (0.199) (0.178) TSLS 2.314* -10.095 6.060 (1.193) (6.278) (7.185) *. Significant at 10%; ** significant at 5%; *** significant at 1%. Standard errors are in parentheses. The figures reported in the table are the coefficients on the prison incarceration rate, prisoner admission rate, and prisoner release rate from either OLS or TSLS regressions of each of the listed AIDS infection rates on these explanatory variables. In the TSLS models, the four sentencing reform instruments listed in Table 5 are used as instruments for each of the endogenous variables. All of the models include the complete set of control variables used in the first-stage models presented in Table 5. 65