Rand Report on Ca Parolees 2009
Download original document:
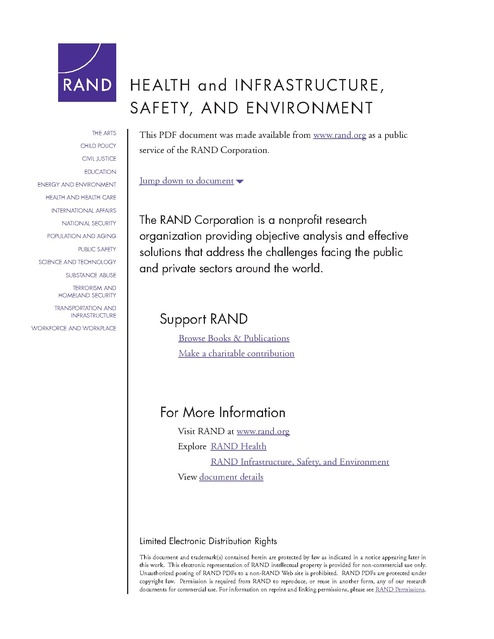
Document text
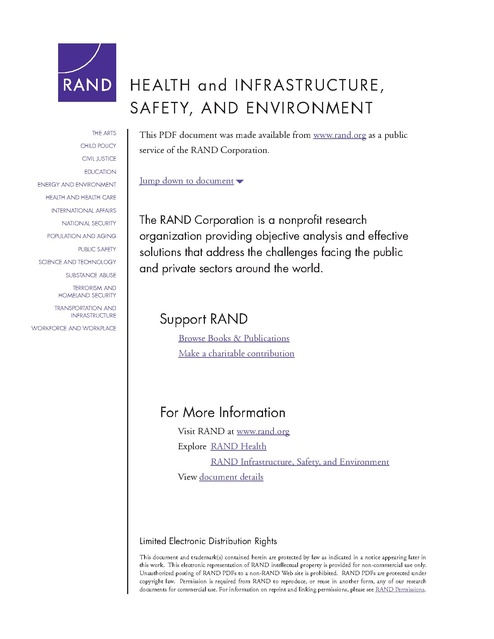
Document text
This text is machine-read, and may contain errors. Check the original document to verify accuracy.
HE A LT H an d I NF RAS TRUCTURE, SA F ET Y, A ND ENVI RONMENT THE ARTS CHILD POLICY CIVIL JUSTICE EDUCATION ENERGY AND ENVIRONMENT This PDF document was made available from www.rand.org as a public service of the RAND Corporation. Jump down to document6 HEALTH AND HEALTH CARE INTERNATIONAL AFFAIRS NATIONAL SECURITY POPULATION AND AGING PUBLIC SAFETY SCIENCE AND TECHNOLOGY SUBSTANCE ABUSE The RAND Corporation is a nonprofit research organization providing objective analysis and effective solutions that address the challenges facing the public and private sectors around the world. TERRORISM AND HOMELAND SECURITY TRANSPORTATION AND INFRASTRUCTURE WORKFORCE AND WORKPLACE Support RAND Browse Books & Publications Make a charitable contribution For More Information Visit RAND at www.rand.org Explore RAND Health RAND Infrastructure, Safety, and Environment View document details Limited Electronic Distribution Rights This document and trademark(s) contained herein are protected by law as indicated in a notice appearing later in this work. This electronic representation of RAND intellectual property is provided for non-commercial use only. Unauthorized posting of RAND PDFs to a non-RAND Web site is prohibited. RAND PDFs are protected under copyright law. Permission is required from RAND to reproduce, or reuse in another form, any of our research documents for commercial use. For information on reprint and linking permissions, please see RAND Permissions. This product is part of the RAND Corporation technical report series. Reports may include research findings on a specific topic that is limited in scope; present discussions of the methodology employed in research; provide literature reviews, survey instruments, modeling exercises, guidelines for practitioners and research professionals, and supporting documentation; or deliver preliminary findings. All RAND reports undergo rigorous peer review to ensure that they meet high standards for research quality and objectivity. TECHNIC A L REP O RT Understanding the Public Health Implications of Prisoner Reentry in California Phase I Report Lois M. Davis • Nancy Nicosia • Adrian Overton • Lisa Miyashiro Kathryn Pitkin Derose • Terry Fain • Susan Turner Paul Steinberg • Eugene Williams III Prepared for The California Endowment H EALT H and I NF R AS T R UCTURE, SAFETY, AND ENV I RONM ENT This work was prepared for The California Endowment and produced within the RAND Health Promotion and Disease Prevention Program (HPDP) and the RAND Infrastructure, Safety, and Environment (ISE) Safety and Justice Program. The RAND Corporation is a nonprofit research organization providing objective analysis and effective solutions that address the challenges facing the public and private sectors around the world. RAND’s publications do not necessarily reflect the opinions of its research clients and sponsors. R® is a registered trademark. © Copyright 2009 RAND Corporation Permission is given to duplicate this document for personal use only, as long as it is unaltered and complete. Copies may not be duplicated for commercial purposes. Unauthorized posting of R AND documents to a non-R AND Web site is prohibited. R AND documents are protected under copyright law. For information on reprint and linking permissions, please visit the RAND permissions page (http://www.rand.org/publications/ permissions.html). Published 2009 by the RAND Corporation 1776 Main Street, P.O. Box 2138, Santa Monica, CA 90407-2138 1200 South Hayes Street, Arlington, VA 22202-5050 4570 Fifth Avenue, Suite 600, Pittsburgh, PA 15213-2665 RAND URL: http://www.rand.org To order RAND documents or to obtain additional information, contact Distribution Services: Telephone: (310) 451-7002; Fax: (310) 451-6915; Email: order@rand.org Preface This report is designed to help readers better understand the public health challenges of prisoner reentry in California. It represents findings from Phase I of our study. Here, we examine the public health issues surrounding prisoner reentry in California, the types of health care needs that ex-offenders bring with them, which communities are disproportionately affected, and the health care system capacity of the communities to which ex-offenders return. As part of this examination, we addressed three research questions: (1) What are the health care needs of prisoners upon their release and return to the community? (2) What is the geographic distribution of state prisoners who return to local communities in California? and (3) What types of health care services are available in these communities, and what is their ability to meet the needs of returning prisoners? In Phase II, we will conduct in-depth case studies in three of the counties (Alameda, Los Angeles, and San Diego) to explore the issues and challenges parolees face in meeting their health care needs and that factors that affect providers’ ability to provide services to this population. These results will be of interest to the California Department of Corrections and Rehabilitation; the California Department of Health Services, Mental Health Care, and Alcohol and Drug Programs; county public health and public safety departments; The California Endowment; and other health care foundations interested in the issue of prisoner reentry. This report also will be of interest to public health officials, health care providers, mental health and alcohol- and drug-treatment service providers, community leaders, and state and local policymakers responsible for providing services to individuals returning to California communities from prison and improving collaboration between key stakeholders at the state and local levels to successfully address the public health challenges of prisoner reentry. This work was prepared for The California Endowment and produced within the RAND Health Promotion and Disease Prevention Program (HPDP) and the RAND Infrastructure, Safety, and Environment (ISE) Safety and Justice Program. The mission of RAND Health is to advance understanding of health and health behaviors and to examine how the organization and financing of care affects costs, quality, and access for Americans and for citizens of other countries. RAND HPDP is a division of RAND Health and addresses issues related to measuring healthy and unhealthy behaviors, examining the distribution of health behaviors across population subgroups, identifying what causes or influences such behaviors, and designing and evaluating interventions to improve health behaviors. Information about RAND Health and its research and publications can be found at http://www.rand.org/health/. The mission of ISE is to improve the development, operation, use, and protection of society’s essential physical assets and natural resources and to enhance the related social assets of iii iv Understanding the Health Implications of Prisoner Reentry in California, Phase I Report safety and security of individuals in transit and in their workplaces and communities. Safety and Justice Program research addresses occupational safety, courts and corrections, and public safety—including violence, policing, substance abuse, and public integrity. Information about the Safety and Justice Program and its research publications can be found at http://www.rand. org/ise/safety. More information about RAND is available at http://www.rand.org. This project was conducted in collaboration with the Regional Congregations and Neighborhood Organizations (RCNO) Training Center, a national faith- and community-based organizing network. Questions or comments about this report are welcome and may be sent to the project leader, Lois M. Davis at Lois_Davis@rand.org. Contents Preface.. . . . . . . . . . . . . . . . . . . . . . . . . . . . . . . . . . . . . . . . . . . . . . . . . . . . . . . . . . . . . . . . . . . . . . . . . . . . . . . . . . . . . . . . . . . . . . . . . . . . . . . . . . . iii Figures.. . . . . . . . . . . . . . . . . . . . . . . . . . . . . . . . . . . . . . . . . . . . . . . . . . . . . . . . . . . . . . . . . . . . . . . . . . . . . . . . . . . . . . . . . . . . . . . . . . . . . . . . . . . ix Tables. . . . . . . . . . . . . . . . . . . . . . . . . . . . . . . . . . . . . . . . . . . . . . . . . . . . . . . . . . . . . . . . . . . . . . . . . . . . . . . . . . . . . . . . . . . . . . . . . . . . . . . . . . . . xiii Summary.. . . . . . . . . . . . . . . . . . . . . . . . . . . . . . . . . . . . . . . . . . . . . . . . . . . . . . . . . . . . . . . . . . . . . . . . . . . . . . . . . . . . . . . . . . . . . . . . . . . . . . . . xv Acknowledgments.. . . . . . . . . . . . . . . . . . . . . . . . . . . . . . . . . . . . . . . . . . . . . . . . . . . . . . . . . . . . . . . . . . . . . . . . . . . . . . . . . . . . . . . . . . xxiii Abbreviations.. . . . . . . . . . . . . . . . . . . . . . . . . . . . . . . . . . . . . . . . . . . . . . . . . . . . . . . . . . . . . . . . . . . . . . . . . . . . . . . . . . . . . . . . . . . . . . . . . xxv Chapter One Introduction.. . . . . . . . . . . . . . . . . . . . . . . . . . . . . . . . . . . . . . . . . . . . . . . . . . . . . . . . . . . . . . . . . . . . . . . . . . . . . . . . . . . . . . . . . . . . . . . . . . . . . 1 Background.. . . . . . . . . . . . . . . . . . . . . . . . . . . . . . . . . . . . . . . . . . . . . . . . . . . . . . . . . . . . . . . . . . . . . . . . . . . . . . . . . . . . . . . . . . . . . . . . . . . . . . . 1 Objective and Scope.. . . . . . . . . . . . . . . . . . . . . . . . . . . . . . . . . . . . . . . . . . . . . . . . . . . . . . . . . . . . . . . . . . . . . . . . . . . . . . . . . . . . . . . . . . . . . 4 Approach.. . . . . . . . . . . . . . . . . . . . . . . . . . . . . . . . . . . . . . . . . . . . . . . . . . . . . . . . . . . . . . . . . . . . . . . . . . . . . . . . . . . . . . . . . . . . . . . . . . . . . . . . . . 5 Limitations.. . . . . . . . . . . . . . . . . . . . . . . . . . . . . . . . . . . . . . . . . . . . . . . . . . . . . . . . . . . . . . . . . . . . . . . . . . . . . . . . . . . . . . . . . . . . . . . . . . . . . . . . 5 Organization of This Report. . . . . . . . . . . . . . . . . . . . . . . . . . . . . . . . . . . . . . . . . . . . . . . . . . . . . . . . . . . . . . . . . . . . . . . . . . . . . . . . . . . . 6 Chapter Two Socioeconomic and Health Characteristics of California State Prisoners.. . . . . . . . . . . . . . . . . . . . . . . . . . . 7 Methods and Data Sources.. . . . . . . . . . . . . . . . . . . . . . . . . . . . . . . . . . . . . . . . . . . . . . . . . . . . . . . . . . . . . . . . . . . . . . . . . . . . . . . . . . . . . 7 Socioeconomic Characteristics of California State Prison Inmates.. . . . . . . . . . . . . . . . . . . . . . . . . . . . . . . . . . . . . . . . 9 Basic Demographics.. . . . . . . . . . . . . . . . . . . . . . . . . . . . . . . . . . . . . . . . . . . . . . . . . . . . . . . . . . . . . . . . . . . . . . . . . . . . . . . . . . . . . . . . . . . 9 Education, Employment, and Military Service. . . . . . . . . . . . . . . . . . . . . . . . . . . . . . . . . . . . . . . . . . . . . . . . . . . . . . . . . . . . 10 Family Ties and Adverse Life Events.. . . . . . . . . . . . . . . . . . . . . . . . . . . . . . . . . . . . . . . . . . . . . . . . . . . . . . . . . . . . . . . . . . . . . . . 13 Criminal History. . . . . . . . . . . . . . . . . . . . . . . . . . . . . . . . . . . . . . . . . . . . . . . . . . . . . . . . . . . . . . . . . . . . . . . . . . . . . . . . . . . . . . . . . . . . . . 15 Physical Health Needs and Utilization.. . . . . . . . . . . . . . . . . . . . . . . . . . . . . . . . . . . . . . . . . . . . . . . . . . . . . . . . . . . . . . . . . . . . . . . 16 Lifetime and Current Physical Health Problems. . . . . . . . . . . . . . . . . . . . . . . . . . . . . . . . . . . . . . . . . . . . . . . . . . . . . . . . . . 17 Infectious Diseases. . . . . . . . . . . . . . . . . . . . . . . . . . . . . . . . . . . . . . . . . . . . . . . . . . . . . . . . . . . . . . . . . . . . . . . . . . . . . . . . . . . . . . . . . . . . 18 Other Physical Health Conditions. . . . . . . . . . . . . . . . . . . . . . . . . . . . . . . . . . . . . . . . . . . . . . . . . . . . . . . . . . . . . . . . . . . . . . . . . 20 Medical Treatment of Physical Health Needs While Incarcerated.. . . . . . . . . . . . . . . . . . . . . . . . . . . . . . . . . . . . . 21 Social and Behavioral Needs and Utilization.. . . . . . . . . . . . . . . . . . . . . . . . . . . . . . . . . . . . . . . . . . . . . . . . . . . . . . . . . . . . . . . 22 Drug Abuse and Dependence.. . . . . . . . . . . . . . . . . . . . . . . . . . . . . . . . . . . . . . . . . . . . . . . . . . . . . . . . . . . . . . . . . . . . . . . . . . . . . . 22 Treatment for Drug Use, Abuse, and Dependence.. . . . . . . . . . . . . . . . . . . . . . . . . . . . . . . . . . . . . . . . . . . . . . . . . . . . . . . 25 Mental Health Conditions. . . . . . . . . . . . . . . . . . . . . . . . . . . . . . . . . . . . . . . . . . . . . . . . . . . . . . . . . . . . . . . . . . . . . . . . . . . . . . . . . . 27 Recent History or Symptoms of Mental Illness.. . . . . . . . . . . . . . . . . . . . . . . . . . . . . . . . . . . . . . . . . . . . . . . . . . . . . . . . . . 27 Lifetime Prevalence of Mental Illness.. . . . . . . . . . . . . . . . . . . . . . . . . . . . . . . . . . . . . . . . . . . . . . . . . . . . . . . . . . . . . . . . . . . . . . 29 Treatment for Mental Illness.. . . . . . . . . . . . . . . . . . . . . . . . . . . . . . . . . . . . . . . . . . . . . . . . . . . . . . . . . . . . . . . . . . . . . . . . . . . . . . . 30 v vi Understanding the Health Implications of Prisoner Reentry in California, Phase I Report Comorbidity/Dual Diagnoses.. . . . . . . . . . . . . . . . . . . . . . . . . . . . . . . . . . . . . . . . . . . . . . . . . . . . . . . . . . . . . . . . . . . . . . . . . . . . . . . 32 Discussion. . . . . . . . . . . . . . . . . . . . . . . . . . . . . . . . . . . . . . . . . . . . . . . . . . . . . . . . . . . . . . . . . . . . . . . . . . . . . . . . . . . . . . . . . . . . . . . . . . . . . . . . 32 Chapter Three Distribution and Concentration of Parolees in California. . . . . . . . . . . . . . . . . . . . . . . . . . . . . . . . . . . . . . . . . . . . . 35 Analysis of Concentration and Distribution of Parolees in California. . . . . . . . . . . . . . . . . . . . . . . . . . . . . . . . . . . . 35 Methods. . . . . . . . . . . . . . . . . . . . . . . . . . . . . . . . . . . . . . . . . . . . . . . . . . . . . . . . . . . . . . . . . . . . . . . . . . . . . . . . . . . . . . . . . . . . . . . . . . . . . . . . 35 Results of Analysis of Concentration and Distribution of Parolees in California. . . . . . . . . . . . . . . . . . . . . 39 Analysis of Demographic Characteristics of California Parolees and the Socioeconomic Characteristics of Areas to Which They Return. . . . . . . . . . . . . . . . . . . . . . . . . . . . . . . . . . . . . . . . . . . . . . . . . . . . . . . . 52 Methods. . . . . . . . . . . . . . . . . . . . . . . . . . . . . . . . . . . . . . . . . . . . . . . . . . . . . . . . . . . . . . . . . . . . . . . . . . . . . . . . . . . . . . . . . . . . . . . . . . . . . . . . 52 Results of Analysis of Demographic Characteristics of California Parolees.. . . . . . . . . . . . . . . . . . . . . . . . . . . 52 Results of Analysis of Socioeconomic Characteristics of Areas to Which Parolees Return.. . . . . . . . . 54 Discussion. . . . . . . . . . . . . . . . . . . . . . . . . . . . . . . . . . . . . . . . . . . . . . . . . . . . . . . . . . . . . . . . . . . . . . . . . . . . . . . . . . . . . . . . . . . . . . . . . . . . . . . . 57 Chapter Four Conceptual Framework and Methods for Defining the Health Care Safety Net for Parolees.. . . . . . . . . . . . . . . . . . . . . . . . . . . . . . . . . . . . . . . . . . . . . . . . . . . . . . . . . . . . . . . . . . . . . . . . . . . . . . . . . . . . . . . . . . . . . . . . . . . . . 59 Conceptual Framework. . . . . . . . . . . . . . . . . . . . . . . . . . . . . . . . . . . . . . . . . . . . . . . . . . . . . . . . . . . . . . . . . . . . . . . . . . . . . . . . . . . . . . . . . 59 Overall Approach to Defining the Safety Net for the Reentry Population and Constructing Accessibility Measures.. . . . . . . . . . . . . . . . . . . . . . . . . . . . . . . . . . . . . . . . . . . . . . . . . . . . . . . . . . . . . . . . . . . . . . . . . . . . . . . . . . . . . 63 Methods for Defining County Safety Nets and Developing Accessibility Measures.. . . . . . . . . . . . . . . . . . 64 Defining the Health Care Safety Net.. . . . . . . . . . . . . . . . . . . . . . . . . . . . . . . . . . . . . . . . . . . . . . . . . . . . . . . . . . . . . . . . . . . . . 64 Developing a Measure of Accessibility for Hospitals and Primary Care Clinics.. . . . . . . . . . . . . . . . . . . . . 66 Defining the Mental Health Safety Net. . . . . . . . . . . . . . . . . . . . . . . . . . . . . . . . . . . . . . . . . . . . . . . . . . . . . . . . . . . . . . . . . . . 68 Defining the Substance Abuse Safety Net.. . . . . . . . . . . . . . . . . . . . . . . . . . . . . . . . . . . . . . . . . . . . . . . . . . . . . . . . . . . . . . . . . 69 Developing a Measure of Accessibility for Mental Health and Substance Abuse Providers.. . . . . . . . . 72 Discussion. . . . . . . . . . . . . . . . . . . . . . . . . . . . . . . . . . . . . . . . . . . . . . . . . . . . . . . . . . . . . . . . . . . . . . . . . . . . . . . . . . . . . . . . . . . . . . . . . . . . . . . . 72 Chapter Five Counties’ Capacity to Meet the Health Care Needs of the Reentry Population.. . . . . . . . . . . . . . . . . . . 75 Alameda County.. . . . . . . . . . . . . . . . . . . . . . . . . . . . . . . . . . . . . . . . . . . . . . . . . . . . . . . . . . . . . . . . . . . . . . . . . . . . . . . . . . . . . . . . . . . . . . . . 76 Kern County. . . . . . . . . . . . . . . . . . . . . . . . . . . . . . . . . . . . . . . . . . . . . . . . . . . . . . . . . . . . . . . . . . . . . . . . . . . . . . . . . . . . . . . . . . . . . . . . . . . . . 81 Los Angeles County. . . . . . . . . . . . . . . . . . . . . . . . . . . . . . . . . . . . . . . . . . . . . . . . . . . . . . . . . . . . . . . . . . . . . . . . . . . . . . . . . . . . . . . . . . . . 87 San Diego County.. . . . . . . . . . . . . . . . . . . . . . . . . . . . . . . . . . . . . . . . . . . . . . . . . . . . . . . . . . . . . . . . . . . . . . . . . . . . . . . . . . . . . . . . . . . . . . 95 Discussion. . . . . . . . . . . . . . . . . . . . . . . . . . . . . . . . . . . . . . . . . . . . . . . . . . . . . . . . . . . . . . . . . . . . . . . . . . . . . . . . . . . . . . . . . . . . . . . . . . . . . . 101 Chapter Six Counties’ Capacity to Meet the Mental Health and Substance Abuse Treatment Needs of the Reentry Population. . . . . . . . . . . . . . . . . . . . . . . . . . . . . . . . . . . . . . . . . . . . . . . . . . . . . . . . . . . . . . . . . . . . . . . . . . . . . Introduction. . . . . . . . . . . . . . . . . . . . . . . . . . . . . . . . . . . . . . . . . . . . . . . . . . . . . . . . . . . . . . . . . . . . . . . . . . . . . . . . . . . . . . . . . . . . . . . . . . . . Analytic Findings for Mental Health Care Safety Net.. . . . . . . . . . . . . . . . . . . . . . . . . . . . . . . . . . . . . . . . . . . . . . . . . . . Alameda County.. . . . . . . . . . . . . . . . . . . . . . . . . . . . . . . . . . . . . . . . . . . . . . . . . . . . . . . . . . . . . . . . . . . . . . . . . . . . . . . . . . . . . . . . . . . . . . Kern County. . . . . . . . . . . . . . . . . . . . . . . . . . . . . . . . . . . . . . . . . . . . . . . . . . . . . . . . . . . . . . . . . . . . . . . . . . . . . . . . . . . . . . . . . . . . . . . . . Los Angeles County.. . . . . . . . . . . . . . . . . . . . . . . . . . . . . . . . . . . . . . . . . . . . . . . . . . . . . . . . . . . . . . . . . . . . . . . . . . . . . . . . . . . . . . . . San Diego County.. . . . . . . . . . . . . . . . . . . . . . . . . . . . . . . . . . . . . . . . . . . . . . . . . . . . . . . . . . . . . . . . . . . . . . . . . . . . . . . . . . . . . . . . . . 107 107 108 108 111 114 118 Contents vii Analytic Findings for the Substance Abuse Safety Net. . . . . . . . . . . . . . . . . . . . . . . . . . . . . . . . . . . . . . . . . . . . . . . . . . . Alameda County.. . . . . . . . . . . . . . . . . . . . . . . . . . . . . . . . . . . . . . . . . . . . . . . . . . . . . . . . . . . . . . . . . . . . . . . . . . . . . . . . . . . . . . . . . . . . Kern County. . . . . . . . . . . . . . . . . . . . . . . . . . . . . . . . . . . . . . . . . . . . . . . . . . . . . . . . . . . . . . . . . . . . . . . . . . . . . . . . . . . . . . . . . . . . . . . . . Los Angeles County.. . . . . . . . . . . . . . . . . . . . . . . . . . . . . . . . . . . . . . . . . . . . . . . . . . . . . . . . . . . . . . . . . . . . . . . . . . . . . . . . . . . . . . . . San Diego County.. . . . . . . . . . . . . . . . . . . . . . . . . . . . . . . . . . . . . . . . . . . . . . . . . . . . . . . . . . . . . . . . . . . . . . . . . . . . . . . . . . . . . . . . . . Discussion. . . . . . . . . . . . . . . . . . . . . . . . . . . . . . . . . . . . . . . . . . . . . . . . . . . . . . . . . . . . . . . . . . . . . . . . . . . . . . . . . . . . . . . . . . . . . . . . . . . . . . 121 121 124 127 132 136 Chapter Seven Summary and Implications.. . . . . . . . . . . . . . . . . . . . . . . . . . . . . . . . . . . . . . . . . . . . . . . . . . . . . . . . . . . . . . . . . . . . . . . . . . . . . . . . Introduction. . . . . . . . . . . . . . . . . . . . . . . . . . . . . . . . . . . . . . . . . . . . . . . . . . . . . . . . . . . . . . . . . . . . . . . . . . . . . . . . . . . . . . . . . . . . . . . . . . . . Summary of Findings. . . . . . . . . . . . . . . . . . . . . . . . . . . . . . . . . . . . . . . . . . . . . . . . . . . . . . . . . . . . . . . . . . . . . . . . . . . . . . . . . . . . . . . . . Health Care Needs of the Reentry Population.. . . . . . . . . . . . . . . . . . . . . . . . . . . . . . . . . . . . . . . . . . . . . . . . . . . . . . . . . . Certain Counties and Communities Are Disproportionately Affected by Reentry. . . . . . . . . . . . . . . . . Access to Safety-Net Resources Varies Substantially.. . . . . . . . . . . . . . . . . . . . . . . . . . . . . . . . . . . . . . . . . . . . . . . . . . . Implications for Policymakers and Practitioners.. . . . . . . . . . . . . . . . . . . . . . . . . . . . . . . . . . . . . . . . . . . . . . . . . . . . . . . . . . Strengths and Limitations of Our Approach.. . . . . . . . . . . . . . . . . . . . . . . . . . . . . . . . . . . . . . . . . . . . . . . . . . . . . . . . . . . . Next Steps. . . . . . . . . . . . . . . . . . . . . . . . . . . . . . . . . . . . . . . . . . . . . . . . . . . . . . . . . . . . . . . . . . . . . . . . . . . . . . . . . . . . . . . . . . . . . . . . . . . . . . 141 141 142 142 142 143 144 146 147 Appendixes A. Parolee Clusters in Four California Counties, by Count of Parolees.. . . . . . . . . . . . . . . . . . . . . . . . . B. Methods. . . . . . . . . . . . . . . . . . . . . . . . . . . . . . . . . . . . . . . . . . . . . . . . . . . . . . . . . . . . . . . . . . . . . . . . . . . . . . . . . . . . . . . . . . . . . . . . . . . C. Detailed Results Tables Used in Estimating Prevalence of Symptoms of Drug Abuse and Dependence and Mental Health Problems.. . . . . . . . . . . . . . . . . . . . . . . . . . . . . . . . . . . . . . . . . . . . . . . . . . . D. Additional Results for Physical Health and Mental Health Problems. . . . . . . . . . . . . . . . . . . . . . . . E. Sensitivity Analysis of Missing Zip Code Data and Alternate Accessibility Measures for Hospitals and Clinics.. . . . . . . . . . . . . . . . . . . . . . . . . . . . . . . . . . . . . . . . . . . . . . . . . . . . . . . . . . . . . . . . . . . . . . . . . . . . . . 149 155 165 171 175 References.. . . . . . . . . . . . . . . . . . . . . . . . . . . . . . . . . . . . . . . . . . . . . . . . . . . . . . . . . . . . . . . . . . . . . . . . . . . . . . . . . . . . . . . . . . . . . . . . . . . . . 187 Figures S.1. 3.1. 3.2. 3.3. 3.4. 3.5. 3.6. 3.7. 3.8. 3.9. 3.10. 4.1. 5.1. 5.2. 5.3. 5.4. 5.5. 5.6. 5.7. 5.8. 5.9. 5.10. 5.11. 5.12. 5.13. 5.14. 5.15. 5.16. 5.17. 6.1. 6.2. 6.3. Relative Concentrations of Parolees in California, by County.. . . . . . . . . . . . . . . . . . . . . . . . . . . . . xvii Relative Concentrations of Parolees in California, by County.. . . . . . . . . . . . . . . . . . . . . . . . . . . . . . 40 Relative Concentrations of Parolees in Alameda County.. . . . . . . . . . . . . . . . . . . . . . . . . . . . . . . . . . . . 44 Five Clusters in Alameda County Account for Nearly Half of Parolees.. . . . . . . . . . . . . . . . . . . . 45 Relative Concentrations of Parolees in Kern County. . . . . . . . . . . . . . . . . . . . . . . . . . . . . . . . . . . . . . . . . 46 Four Clusters in Kern County Account for More than Half of Parolees. . . . . . . . . . . . . . . . . . . . 47 Relative Concentrations of Parolees in Los Angeles County.. . . . . . . . . . . . . . . . . . . . . . . . . . . . . . . . 48 Twenty-Three Clusters Account for 35 Percent of Parolees in Los Angeles County.. . . . . . . 49 Relative Concentrations of Parolees in San Diego County.. . . . . . . . . . . . . . . . . . . . . . . . . . . . . . . . . . 50 Eight Clusters Account for Nearly Half of Parolees in San Diego County.. . . . . . . . . . . . . . . . . 51 Summary of Statewide Socioeconomic Clusters of Census Tracts. . . . . . . . . . . . . . . . . . . . . . . . . . 54 Conceptual Framework for Evaluating the Health Care Safety Net for the Reentry Population.. . . . . . . . . . . . . . . . . . . . . . . . . . . . . . . . . . . . . . . . . . . . . . . . . . . . . . . . . . . . . . . . . . . . . . . . . . . . . . . . . . . . . . . . . 60 Map of Primary Care Clinics, by Supervisorial District, Alameda County.. . . . . . . . . . . . . . . 77 Map of Primary Care Clinic Accessibility Measure, Alameda County.. . . . . . . . . . . . . . . . . . . . . 78 Map of General Acute Care Hospitals, by Supervisorial District, Alameda County.. . . . . 80 Map of General Acute Care Hospital Accessibility Measure, Alameda County.. . . . . . . . . . . 81 Map of Clinics, by Supervisorial District, Kern County. . . . . . . . . . . . . . . . . . . . . . . . . . . . . . . . . . . . . . 82 Map of Primary Care Clinic Accessibility Measure, Kern County.. . . . . . . . . . . . . . . . . . . . . . . . . . 83 Map of General Acute Care Hospitals, by Supervisorial District, Kern County. . . . . . . . . . . 85 Map of General Acute Care Hospital Accessibility Measure, Kern County. . . . . . . . . . . . . . . 86 Map of Primary Care Clinics, by Supervisorial District, Los Angeles County.. . . . . . . . . . . 88 Map of Primary Care Clinics, by Service Planning Area, Los Angeles County. . . . . . . . . . . . 89 Map of Primary Care Clinic Accessibility Measure, Los Angeles County. . . . . . . . . . . . . . . . . 90 Map of General Acute Care Hospitals, by Service Planning Area, Los Angeles County.. . . . . . . . . . . . . . . . . . . . . . . . . . . . . . . . . . . . . . . . . . . . . . . . . . . . . . . . . . . . . . . . . . . . . . . . . . . . . . 92 Map of General Acute Care Hospital Accessibility Measure, Los Angeles County. . . . . . . . 93 Map of Primary Care Clinics, by Supervisorial District, San Diego County.. . . . . . . . . . . . . . 95 Map of Primary Care Clinic Accessibility Measure, San Diego County. . . . . . . . . . . . . . . . . . . 96 Map of General Acute Care Hospitals, by Supervisorial District, San Diego County.. . . 99 Map of General Acute Care Hospitals Accessibility Measure, San Diego County.. . . . . . 100 Map of Mental Health Providers and Parole Outpatient Clinics, by Supervisorial District, Alameda County.. . . . . . . . . . . . . . . . . . . . . . . . . . . . . . . . . . . . . . . . . . . . . . . . . . . . . . . . . . . . . . . . . . . . . . 109 Map of Mental Health Provider Accessibility Measure, by Supervisorial District, Alameda County. . . . . . . . . . . . . . . . . . . . . . . . . . . . . . . . . . . . . . . . . . . . . . . . . . . . . . . . . . . . . . . . . . . . . . . . . . . . . . . . . 110 Map of Mental Health Providers and Parole Outpatient Clinics, by Supervisorial District, Kern County. . . . . . . . . . . . . . . . . . . . . . . . . . . . . . . . . . . . . . . . . . . . . . . . . . . . . . . . . . . . . . . . . . . . . . . . . . . 112 ix x Understanding the Health Implications of Prisoner Reentry in California, Phase I Report 6.4. Map of Mental Health Provider Accessibility Measure, by Supervisorial District, Kern County. . . . . . . . . . . . . . . . . . . . . . . . . . . . . . . . . . . . . . . . . . . . . . . . . . . . . . . . . . . . . . . . . . . . . . . . . . . . . . . . . . . . . . 113 6.5. Map of MHSA and Other Mental Health Providers and Parole Outpatient Clinics, by Supervisorial District, Los Angeles County. . . . . . . . . . . . . . . . . . . . . . . . . . . . . . . . . . . . . . . . . . . . . . . 115 6.6. Map of MHSA and Other Mental Health Providers and Parole Outpatient Clinics, by Service Planning Area, Los Angeles County. . . . . . . . . . . . . . . . . . . . . . . . . . . . . . . . . . . . . . . . . . . . . . 116 6.7. Map of Mental Health Provider Accessibility Measure, by Service Planning Area, Los Angeles County.. . . . . . . . . . . . . . . . . . . . . . . . . . . . . . . . . . . . . . . . . . . . . . . . . . . . . . . . . . . . . . . . . . . . . . . . . . . . . 117 6.8. Map of MHSA, Other Mental Health Clinics, and Parole Outpatient Clinics, by Supervisorial District, San Diego County.. . . . . . . . . . . . . . . . . . . . . . . . . . . . . . . . . . . . . . . . . . . . . . . . . . . . 119 6.9. Map of MHSA and Other County Mental Health Clinic Accessibility Measure, by Supervisorial District, San Diego County.. . . . . . . . . . . . . . . . . . . . . . . . . . . . . . . . . . . . . . . . . . . . . . . . . . . . 120 6.10. Map of Proposition 36 and Other Alcohol- and Drug-Treatment Providers, by Supervisorial District, Alameda County.. . . . . . . . . . . . . . . . . . . . . . . . . . . . . . . . . . . . . . . . . . . . . . . . . . . . . . 121 6.11. Map of PSN Providers, by Supervisorial District, Alameda County.. . . . . . . . . . . . . . . . . . . . . . 122 6.12. Map of Proposition 36 and Other Alcohol and Drug Treatment Provider Accessibility Measure, by Supervisorial District, Alameda County.. . . . . . . . . . . . . . . . . . . . . . . 123 6.13. Map of Proposition 36 and Other Alcohol- and Drug-Treatment Providers, by Supervisorial District, Kern County. . . . . . . . . . . . . . . . . . . . . . . . . . . . . . . . . . . . . . . . . . . . . . . . . . . . . . . . . . . 124 6.14. Map of PSN Providers, by Supervisorial District, Kern County. . . . . . . . . . . . . . . . . . . . . . . . . . . 125 6.15. Map of Proposition 36 and Other Alcohol- and Drug-Treatment Provider Accessibility Measure, by Supervisorial District, Kern County.. . . . . . . . . . . . . . . . . . . . . . . . . . . . 126 6.16. Map of Proposition 36 and Other Alcohol- and Drug-Treatment Providers, by Supervisorial District, Los Angeles County.. . . . . . . . . . . . . . . . . . . . . . . . . . . . . . . . . . . . . . . . . . . . . . . . . . 128 6.17. Map of Proposition 36 and Other Alcohol- and Drug-Treatment Providers, by Service Planning Area, Los Angeles County.. . . . . . . . . . . . . . . . . . . . . . . . . . . . . . . . . . . . . . . . . . . . . . . . . 129 6.18. Map of PSN Providers, by Service Planning Area, Los Angeles County. . . . . . . . . . . . . . . . . . 130 6.19. Map of Proposition 36 and Other Alcohol- and Drug-Treatment Provider Accessibility Measure, by Service Planning Area, Los Angeles County. . . . . . . . . . . . . . . . . . . 131 6.20. Map of Proposition 36 and Other Alcohol- and Drug-Treatment Providers, by Supervisorial District, San Diego County.. . . . . . . . . . . . . . . . . . . . . . . . . . . . . . . . . . . . . . . . . . . . . . . . . . . . 133 6.21. Map of PSN Providers, by Supervisorial District, San Diego County.. . . . . . . . . . . . . . . . . . . . 134 6.22. Map of Proposition 36 and Other Alcohol- and Drug-Treatment Provider Accessibility Measure, by Supervisorial District, San Diego County. . . . . . . . . . . . . . . . . . . . . . 135 A.1. Parolee Clusters in Alameda County, by Count of Parolees.. . . . . . . . . . . . . . . . . . . . . . . . . . . . . . . . 150 A.2. Parolee Clusters in Kern County, by Count of Parolees. . . . . . . . . . . . . . . . . . . . . . . . . . . . . . . . . . . . . 151 A.3. Parolee Clusters in Los Angeles County, by Count of Parolees.. . . . . . . . . . . . . . . . . . . . . . . . . . . . 152 A.4. Parolee Clusters in San Diego County, by Count of Parolees.. . . . . . . . . . . . . . . . . . . . . . . . . . . . . . 153 E.1. Comparison of Parolee Rates of Return, by County, Using Cases with Zip Code Data Versus Cases Missing Zip Code Data. . . . . . . . . . . . . . . . . . . . . . . . . . . . . . . . . . . . . . . . . . . . . . . . . . . 176 E.2. Map of Primary Care Clinics Using Alternate Accessibility Measure, Alameda County. . . . . . . . . . . . . . . . . . . . . . . . . . . . . . . . . . . . . . . . . . . . . . . . . . . . . . . . . . . . . . . . . . . . . . . . . . . . . . . . . 178 E.3. Map of Hospitals Using Alternate Accessibility Measure, Alameda County.. . . . . . . . . . . . . 179 E.4. Map of Primary Care Clinics Using Alternate Accessibility Measure, Kern County.. . . 180 E.5. Map of Hospitals Using Alternate Accessibility Measure, Kern County. . . . . . . . . . . . . . . . . . 181 E.6. Map of Primary Care Clinics Using Alternate Accessibility Measure, by SPA, Los Angeles County.. . . . . . . . . . . . . . . . . . . . . . . . . . . . . . . . . . . . . . . . . . . . . . . . . . . . . . . . . . . . . . . . . . . . . . . . . . . . . 182 E.7. Map of Hospitals Using Alternate Accessibility Measure, by SPA, Los Angeles County.. . . . . . . . . . . . . . . . . . . . . . . . . . . . . . . . . . . . . . . . . . . . . . . . . . . . . . . . . . . . . . . . . . . . . . . . . . . . . 183 Figures xi E.8. Map of Primary Care Clinics Using Alternate Accessibility Measure, San Diego County.. . . . . . . . . . . . . . . . . . . . . . . . . . . . . . . . . . . . . . . . . . . . . . . . . . . . . . . . . . . . . . . . . . . . . . . . . . . . . . . 184 E.9. Map of Hospitals Using Alternate Accessibility Measure, San Diego County.. . . . . . . . . . . 185 Tables 2.1. 2.2. 2.3. 2.4. 2.5. 2.6. 2.7. 2.8. 2.9. 2.10. 2.11. 2.12. 2.13. 3.1. 3.2. 3.3. 3.4. 4.1. 5.1. 5.2. 5.3. 5.4. 5.5. 5.6. 5.7. 5.8. 5.9. Basic Demographics.. . . . . . . . . . . . . . . . . . . . . . . . . . . . . . . . . . . . . . . . . . . . . . . . . . . . . . . . . . . . . . . . . . . . . . . . . . . . . . . . 9 Education, Employment, and Military Experience.. . . . . . . . . . . . . . . . . . . . . . . . . . . . . . . . . . . . . . . . . . . . 11 Family Ties and Adverse Life Events.. . . . . . . . . . . . . . . . . . . . . . . . . . . . . . . . . . . . . . . . . . . . . . . . . . . . . . . . . . . . 13 Criminal History. . . . . . . . . . . . . . . . . . . . . . . . . . . . . . . . . . . . . . . . . . . . . . . . . . . . . . . . . . . . . . . . . . . . . . . . . . . . . . . . . . . 15 Lifetime and Chronic Physical Health Problems.. . . . . . . . . . . . . . . . . . . . . . . . . . . . . . . . . . . . . . . . . . . . . . 18 Infectious Diseases. . . . . . . . . . . . . . . . . . . . . . . . . . . . . . . . . . . . . . . . . . . . . . . . . . . . . . . . . . . . . . . . . . . . . . . . . . . . . . . . . 19 Other Physical Health Conditions. . . . . . . . . . . . . . . . . . . . . . . . . . . . . . . . . . . . . . . . . . . . . . . . . . . . . . . . . . . . . . 20 Medical Treatment/Utilization. . . . . . . . . . . . . . . . . . . . . . . . . . . . . . . . . . . . . . . . . . . . . . . . . . . . . . . . . . . . . . . . . . 22 Drug Use, Abuse, and Dependence. . . . . . . . . . . . . . . . . . . . . . . . . . . . . . . . . . . . . . . . . . . . . . . . . . . . . . . . . . . . . 24 Drug Treatment. . . . . . . . . . . . . . . . . . . . . . . . . . . . . . . . . . . . . . . . . . . . . . . . . . . . . . . . . . . . . . . . . . . . . . . . . . . . . . . . . . . 26 Recent History of Symptoms of Mental Illness.. . . . . . . . . . . . . . . . . . . . . . . . . . . . . . . . . . . . . . . . . . . . . . . 28 Lifetime Prevalence of Mental Illness.. . . . . . . . . . . . . . . . . . . . . . . . . . . . . . . . . . . . . . . . . . . . . . . . . . . . . . . . . . 30 Mental Health Treatment Among Those with a Mental Health Problem.. . . . . . . . . . . . . . . . . . 31 Statewide Clusters Responsible for Nearly Half of Parolees in California.. . . . . . . . . . . . . . . . . . 41 Demographic Characteristics of California Parolees and Terms Served, Overall and for Four Counties .. . . . . . . . . . . . . . . . . . . . . . . . . . . . . . . . . . . . . . . . . . . . . . . . . . . . . . . . . . . . . . . . . . 53 Socioeconomic Characteristics of the Seven Cluster Areas.. . . . . . . . . . . . . . . . . . . . . . . . . . . . . . . . . . . 55 Distribution of Parolees Across the Seven Cluster Areas, Overall and by Race/Ethnicity.. . . . . . . . . . . . . . . . . . . . . . . . . . . . . . . . . . . . . . . . . . . . . . . . . . . . . . . . . . . . . . . . . . . . . . . . . . . . . . . . . . . . 56 Parole Services Network Allocations, FYs 2005–2006 and 2007–2008.. . . . . . . . . . . . . . . . . . . . 71 Distribution of Parolees Across Clinic Accessibility, by Quartile and Race/Ethnicity, Alameda County. . . . . . . . . . . . . . . . . . . . . . . . . . . . . . . . . . . . . . . . . . . . . . . . . . . . . . . . . . . . . . . . . . . . . . . . . . . . . . . . . . . 78 Distribution of Parolees Across Hospital Accessibility, by Quartile and Race/Ethnicity, Alameda County. . . . . . . . . . . . . . . . . . . . . . . . . . . . . . . . . . . . . . . . . . . . . . . . . . . . . . . . . . . . . . . . 81 Distribution of Parolees Across Clinic Accessibility, by Quartile and Race/Ethnicity, Kern County.. . . . . . . . . . . . . . . . . . . . . . . . . . . . . . . . . . . . . . . . . . . . . . . . . . . . . . . . . . . . . . . . . . . 84 Distribution of Parolees Across Hospital Accessibility, by Quartile and Race/Ethnicity, Kern County.. . . . . . . . . . . . . . . . . . . . . . . . . . . . . . . . . . . . . . . . . . . . . . . . . . . . . . . . . . . . . . . . . . . 86 Distribution of Parolees Across Clinic Accessibility Categories, by Quartile and Race/Ethnicity, Los Angeles County.. . . . . . . . . . . . . . . . . . . . . . . . . . . . . . . . . . . . . . . . . . . . . . . . . . . . . . . . . . . . 91 Distribution of Parolees Across Hospital Accessibility Categories, by Quartile and Race/Ethnicity, Los Angeles County.. . . . . . . . . . . . . . . . . . . . . . . . . . . . . . . . . . . . . . . . . . . . . . . . . . . . . . . . . . . 94 Distribution of Parolees Across Clinic Accessibility Categories, by Quartile and Race/Ethnicity, San Diego County. . . . . . . . . . . . . . . . . . . . . . . . . . . . . . . . . . . . . . . . . . . . . . . . . . . . . . . . . . . . . 97 Distribution of Parolees Across Hospital Accessibility Categories, by Quartile and Race/Ethnicity, San Diego County. . . . . . . . . . . . . . . . . . . . . . . . . . . . . . . . . . . . . . . . . . . . . . . . . . . . . . . . . . . . 100 Summary of Accessibility Results, by County and Type of Facility.. . . . . . . . . . . . . . . . . . . . . . . 102 xiii xiv Understanding the Health Implications of Prisoner Reentry in California, Phase I Report 5.10. Summary of Accessibility Results, by County, Type of Facility, and by Race/Ethnicity.. . . . . . . . . . . . . . . . . . . . . . . . . . . . . . . . . . . . . . . . . . . . . . . . . . . . . . . . . . . . . . . . . . . . . . . . . . . . . . . . . . . 6.1. Distribution of Parolees Across Mental Health Provider Accessibility Quartile Categories, by Race/Ethnicity, Alameda County.. . . . . . . . . . . . . . . . . . . . . . . . . . . . . . . . . . . . . . . . . . . . 6.2. Distribution of Parolees Across Mental Health Provider Accessibility Quartile Categories, by Race/Ethnicity, Kern County. . . . . . . . . . . . . . . . . . . . . . . . . . . . . . . . . . . . . . . . . . . . . . . . . 6.3. Distribution of Parolees Across Mental Health Provider Accessibility Quartile Categories, by Race/Ethnicity, Los Angeles County.. . . . . . . . . . . . . . . . . . . . . . . . . . . . . . . . . . . . . . . . 6.4. Distribution of Parolees Across Mental Health Provider Accessibility Quartile Categories, by Race/Ethnicity, San Diego County.. . . . . . . . . . . . . . . . . . . . . . . . . . . . . . . . . . . . . . . . . . 6.5. Distribution of Parolees Across Proposition 36 and Other Alcohol- and Drug-Treatment Provider Accessibility Quartile Categories, by Race/Ethnicity, Alameda County. . . . . . . . . . . . . . . . . . . . . . . . . . . . . . . . . . . . . . . . . . . . . . . . . . . . . . . . . . . . . . . . . . . . . . . . . . . . . . . . . 6.6. Distribution of Parolees Across Proposition 36 and Other Alcohol- and Drug-Treatment Provider Accessibility Quartile Categories, by Race/Ethnicity, Kern County. . . . . . . . . . . . . . . . . . . . . . . . . . . . . . . . . . . . . . . . . . . . . . . . . . . . . . . . . . . . . . . . . . . . . . . . . . . . . . . . . . . . . . 6.7. Distribution of Parolees Across Proposition 36 and Other Alcohol- and Drug-Treatment Provider Accessibility Quartile Categories, by Race/Ethnicity, Los Angeles County.. . . . . . . . . . . . . . . . . . . . . . . . . . . . . . . . . . . . . . . . . . . . . . . . . . . . . . . . . . . . . . . . . . . . . . . . . . . . . 6.8. Distribution of Parolees Across Alcohol- and Drug-Treatment Provider Accessibility Quartile Categories, by Race/Ethnicity, San Diego County. . . . . . . . . . . . . . . . 6.9. Overall Average Level of Accessibility in the Four Counties.. . . . . . . . . . . . . . . . . . . . . . . . . . . . . . . 6.10. Summary of Accessibility Results for Mental Health and Alcohol- and Drug-Treatment Providers.. . . . . . . . . . . . . . . . . . . . . . . . . . . . . . . . . . . . . . . . . . . . . . . . . . . . . . . . . . . . . . . . . . . . . . 6.11. Summary of Mental Health and Alcohol- and Drug-Treatment Accessibility Results, by County, Type of Facility, and Race/Ethnicity.. . . . . . . . . . . . . . . . . . . . . . . . . . . . . . . . . . 6.12. Los Angeles County Parolee Admissions to Proposition 36 and Other County Alcohol- and Drug-Treatment Services, FY 2007–2008.. . . . . . . . . . . . . . . . . . . . . . . . . . . . . . . . . . . . B.1. Demographic Characteristics of All Parolees, Analytic Sample, and BJS Survey of State Prisoners.. . . . . . . . . . . . . . . . . . . . . . . . . . . . . . . . . . . . . . . . . . . . . . . . . . . . . . . . . . . . . . . . . . . . . . . . . . . . . . . . . . . . C.1. Symptoms of Drug Abuse and Dependence. . . . . . . . . . . . . . . . . . . . . . . . . . . . . . . . . . . . . . . . . . . . . . . . . . C.2. Patterns of Drug Use as Measured by Lifetime Prevalence, Regular Use, and Use in Month Prior to Arrest.. . . . . . . . . . . . . . . . . . . . . . . . . . . . . . . . . . . . . . . . . . . . . . . . . . . . . . . . . . . . . . . . . . . . . . . . C.3. Responses to Individual Questions on Mental Health That Served as Input for DSM-IV Category Assignment.. . . . . . . . . . . . . . . . . . . . . . . . . . . . . . . . . . . . . . . . . . . . . . . . . . . . . . . . . . . . . . . . D.1. Dental Problems and Treatment Since Admission. . . . . . . . . . . . . . . . . . . . . . . . . . . . . . . . . . . . . . . . . . . D.2. Disability Rates (percent).. . . . . . . . . . . . . . . . . . . . . . . . . . . . . . . . . . . . . . . . . . . . . . . . . . . . . . . . . . . . . . . . . . . . . . . D.3. Mental Health Treatment Among All Inmates.. . . . . . . . . . . . . . . . . . . . . . . . . . . . . . . . . . . . . . . . . . . . . . E.1. Distribution of Cases with Missing Zip Codes, State and County Levels. . . . . . . . . . . . . . . . 103 111 113 117 120 124 127 132 136 137 138 138 140 156 166 167 169 171 172 173 176 Summary Introduction The past few decades have witnessed a significant increase in the number of ex-prisoners returning to communities nationwide. These trends are important for a number of reasons, not the least of which is that this population presents multiple needs for services, including employment, housing, and social services. Often overlooked are the physical and social-behavioral health concerns of this population and, consequently, the role that health care plays in influencing the success of reintegration. Examining the demographic and health profiles of the prison population shows that it is disproportionately sicker on average than the U.S. population in general, with substantially higher rates of infectious diseases (such as HIV/AIDS, tuberculosis, and hepatitis B and C), serious mental illness, and substance abuse disorders. What is occurring nationally is mirrored in California. Over the past 20 years, the number of individuals released from California prisons has increased nearly threefold, and most of the state’s prisoners ultimately will return to California communities, bringing with them a variety of health and social needs that must be addressed. The explosion of the California prison population and their health profiles raise key public health challenges. To address them, we need to better understand the health care needs of these former inmates and the capacity of the health care safety net in the communities to which they return. In this report, we addressed three research questions: (1) What are the health care needs of prisoners in California upon their release and return to the community? (2) What is the geographic distribution of state prisoners who return to local communities in California? and (3) What types of health care services are available in these communities, and what is their ability to meet the needs of returning prisoners? To examine the first research question, we conducted a state-level analysis using data for California from a national survey of state prison inmates to examine the health care needs of prisoners. For the second research question, we used geocoded corrections data on parolees released from California state prisons in 2005–2006 and cluster analysis to examine the geographic distribution of parolees and identify concentrations of parolees across and within counties. To address the third research question, we focused on four counties in which nearly one-third of parolees reside: Los Angeles, Alameda, Kern, and San Diego. We drew on facility data for hospitals, clinics, mental health treatment providers, and substance abuse services to characterize the health care, mental health, and substance abuse treatment safety nets that serve the uninsured and the parolee population in these counties. Finally, we also developed measures based on capacity, demand, and distance to assess the accessibility of the safety net in areas with high concentration of parolees. xv xvi Understanding the Health Implications of Prisoner Reentry in California, Phase I Report Key Findings Reentry Population Health Care Needs Are High, and Mental Health Care and Substance Abuse Needs Are Even Higher With respect to health conditions, our analysis of survey data showed that California prison inmates bear a high burden of chronic diseases, such as asthma and hypertension, and infectious diseases, such as hepatitis and tuberculosis—conditions that require regular use of health care for effective management. Among California inmates who reported a current medical issue, the majority reported having seen a medical professional since admission to prison. But the likelihood of receiving health care once they are released from prison seems low, given the high rates of uninsurance and other barriers to accessing care and obtaining health insurance or Medi-Cal. Care for communicable and chronic diseases effectively falls under county jurisdiction; thus, much will depend on particular counties’ abilities to meet these needs. The substance abuse treatment and mental health care needs of prisoners are even more pronounced than their physical health care needs. About two-thirds of California inmates reported having a drug abuse or dependence problem. Yet, among California inmates reporting drug abuse or dependence, only 22 percent reported receiving treatment since admission to prison, which is lower than that for state prisoners nationally. More than half of California inmates reported a recent mental health problem, and about half of them received treatment in prison. While this is the same as for U.S. prison population, it still suggests that care may not be reaching all in need. Given the high prevalence of mental health problems and drug abuse and dependence among the prison population, the need for services in communities may be particularly high. Certain Counties and Communities Are Disproportionately Affected by Reentry Figure S.1 shows the clustering of parolees in California. The distribution of parolees shows that they are concentrated in 11 counties, around the Bay Area and in the southern part of the state. Our analysis of the distribution and concentration of parolees across California and in the four selected counties—Alameda, Kern, Los Angeles, and San Diego—shows that certain counties have higher rates of return and that, within counties, there are distinct clusters of parolees, which has implications in terms of targeting reentry resources to these areas. In Alameda County, five distinct clusters of parolees, concentrated primarily around Oakland and the northern section of the county, accounted for almost 45 percent of the returning parolee population. In Kern County, we found four distinct clusters of parolees, with concentrations around the urban area of Bakersfield and two others in the northern and northeastern sections of the county; these clusters accounted for almost 58 percent of parolees. In San Diego County, eight clusters accounted for nearly half the parolee population, with the largest clusters in the areas of downtown San Diego and Southeast San Diego. Unlike in the other counties, Los Angeles County has 23 distinct clusters of parolees covering a large geographic area, but they account for only 35 percent of the county’s total parolees. This dispersion suggests that providing services to the reentry population requires a targeted approach within the various supervisorial districts and service planning areas (SPAs). Summary Figure S.1 Relative Concentrations of Parolees in California, by County Source: CDCR parolee data, 2005–2006. xvii xviii Understanding the Health Implications of Prisoner Reentry in California, Phase I Report Access to Safety-Net Resources Varies Substantially Our geographic mapping and accessibility measures suggest that parolees’ access to health care resources varies by facility type, by geographic area (across and within counties), and by race/ethnicity. One issue that stands out is that in all three of the large urban counties— Alameda, Los Angeles, and San Diego—most parolees resided in areas with the lowest levels of accessibility to general acute care hospitals, with Alameda County having the largest share of parolees in the lowest accessibility areas. In all the counties, community clinics appear to play an important role in filling gaps in coverage by medically indigent service providers vis-à-vis the reentry population. Still, all four counties show that some important geographical gaps in health care resources remain, given the distribution of parolees. The most prominent gaps appear to be in Los Angeles County, where certain supervisorial districts and SPAs with high concentrations of parolees have sparse hospital and clinic resources (the most striking of which is SPA 6, or South Los Angeles). Accessibility to health care resources also varied by parolees’ race/ethnicity. For example, in terms of accessibility to hospitals in Los Angeles and Alameda counties, more African-American parolees resided in areas with lower levels of accessibility than did Latino or white parolees. However, in Kern and San Diego counties, more Latino parolees resided in areas with lower levels of accessibility to hospitals than did white or African-American parolees. With respect to mental health resources, a larger share of parolees in Kern and San Diego counties fell into the two lowest accessibility categories than in Alameda and Los Angeles counties. In terms of substance abuse treatment, about a third of parolees in Kern and San Diego counties fell into the two lowest accessibility categories, compared to between 42 and 46 percent in Alameda and Los Angeles counties, respectively. Accessibility to mental health and substance abuse resources also varied by race/ethnicity. For example, in Kern County, 15 percent of African-American parolees resided in areas with lower levels of accessibility to alcohol- and drug-treatment resources, compared to only 32 percent of Latino parolees and 30 percent of white parolees. San Diego County was similar, with 22 percent of AfricanAmericans residing in areas with the lowest accessibility, compared to 36 percent of Latinos and 30 percent of whites. In Los Angeles County, 47 percent of African-American and 45 percent of Latino parolees, compared to 37 percent of white parolees, resided in areas with lower accessibility. In Alameda County, the share of parolees residing in areas with the lowest accessibility to substance abuse providers was slightly higher for African-Americans (44 percent) relative to Latinos (38 percent) and whites (39 percent). For mental health care and substance abuse treatment, separate networks were dedicated to providing services to the parolee population and served as the initial safety net for the reentry population. However, both networks have very limited capacity, suggesting that many parolees may not be receiving these services and that the majority of the reentry population must rely on resources available to the uninsured and low-income populations in a county. Implications These findings have implications for policymakers. Our analysis of the distribution and concentration of parolees in California showed that there are distinct clusters across the state and that, within counties, parolees tend to cluster in certain communities and neighborhoods. Such clustering has implications in terms of developing strategies to provide health care ser- Summary xix vices and other resources to parolees and to better link this population to needed services. The fact that parolees in more rural counties tend to be more dispersed suggests that a different strategy for providing health care services to these individuals is needed. In addition, Los Angeles County (the county with the largest proportion of parolees) includes a combination of urban and more sparsely populated areas. Los Angeles County had a large number of distinct clusters of parolees with these clusters covering a broad geographical area. This suggests that strategies for providing services to the parolee population will need to be tailored by supervisorial district and SPA. In California, African-American and Latino parolees, in particular, tend to return to disadvantaged neighborhoods and communities, defined by high poverty rates, high unemployment rates, low educational attainment, and a higher share of households in which English is not the primary language. This suggests that reentry in these communities will be even more challenging in that the parolees’ needs for health care, housing, employment, and other services will be harder to meet. How do policymakers, public health and public safety officials, and communities begin to prioritize their efforts? When it comes to physical health conditions, we found that prisoners bear a high burden of chronic diseases, such as hypertension and asthma, and infectious diseases, such as hepatitis and tuberculosis—conditions that require regular use of health care to manage effectively. The chronic nature of these diseases suggests that access to care will be important, yet, in general, many parolees return to disadvantaged areas where there may be few community health care resources. We also found that California prisoners’ mental health care and substance abuse treatment needs appear even more pronounced than their physical health needs, suggesting that this is an important area on which to focus. Our analysis showed that there are three potential safety nets for the parolee population. Particularly in the area of mental health care and substance abuse treatment services, the safety net is made up of a patchwork of different networks of service providers. For parolees and community organizations trying to serve this population, navigating this patchwork of services is a complex and challenging task. For example, in terms of the substance abuse treatment safety net, there are distinct networks of providers that are contracted by either the state or counties to provide services. However, these networks tend to be small relative to the overall size of the parolee population. Clearly, there is a need to consider how to better integrate these different networks of substance abuse services and to assess where greater investments may be needed. Successful recovery requires developing positive coping skills, such as identifying “triggers,” organizing the day, and avoiding negative situations. Strong social and family support is an immeasurable advantage that many addicted offenders do not enjoy. Instead, they suffer weakened family ties instead and long-standing psychosocial problems that put them at great risk for relapse by virtue of their extreme socioeconomic deficits, exposure to drug-using associates, and other high-risk situations. Relapse increases the recidivism risk for technical violations and new offenses, so it is not surprising that research shows that drug and property offenses account for a large part of the recidivism rate in California. Investing in improving access to substance abuse treatment services in communities where parolees are concentrated may yield long-term benefits when it comes to improving the chances of successful reentry, including positive treatment and criminal justice outcomes. Policymakers may also consider ways to improve access to county alcohol- and drug-treatment services. xx Understanding the Health Implications of Prisoner Reentry in California, Phase I Report Like the substance abuse safety net, the mental health safety net also comprises a patchwork of networks that represent potential access for the reentry population. County mental health and contract services make up a large component of the potential safety net for the reentry population. In addition, the Parole Outpatient Clinics (POCs) represent an important initial source of mental health care for parolees and, at least in one county we studied, serve as gatekeepers to accessing county mental health services. Coupled with prerelease assessment, the POCs have been shown to contribute to lower recidivism among mentally ill offenders. However, we found that there are relatively few POCs in the counties that we studied and that many parolees have to travel far to access these services. Further, anecdotal information raises concerns that some POCs may see staffing cutbacks, given the current economic crisis. It is also important to assess policy and institutional barriers that may prevent access to needed services. One area worth assessing is the role of POCs as gatekeepers to county mental health services. From a health services perspective, this may undermine parolee reentry efforts, given that some POCs are understaffed and have long waiting lists. Moreover, anecdotally, it appears that there is a strong disincentive for parolees to report mental health problems to their parole officer for fear of being labeled as a troublemaker or at high risk of recidivism. We will examine these types of policy and institutional barriers in more depth as part of the second phase of our study. With respect to the health care safety net, we found much variability in the potential access to clinics and hospitals across and within counties. An important finding was the role that community clinics play in filling geographic gaps in the safety net. Clearly, one strategy to improve accessibility in those areas with high concentrations of parolees would be to fund more community clinics. At the same time, in the current economic crisis, we expect safetynet providers to become even more stressed. As California considers options for reducing correctional costs by releasing more parolees or offenders without conditions of parole, it will be important to assess the health care impact of these decisions. We expect that community clinics and hospitals, particularly in areas with high concentrations of parolees and where there are few other community resources, will be especially affected. Beyond the specific research-related implications detailed here, key innovations of this study include our approach to defining the safety net for parolees and developing measures of accessibility to examine potential access to health care resources. The approach of mapping parolee clusters in the context of the safety net and their accessibility to health care resources is an important step not only in helping policymakers and practitioners understand the public health implications of reentry, but also in helping them best allocate and fund resources for this population. Our measures provide an indication of how potential access to safety-net resources varies within each county for the parolee population. We validated our approach to measuring accessibility by comparing how well our measures correlate with measures of medically underserved areas and populations in California. These results provide us with confidence that our measures are fairly robust and can serve as a useful planning tool. Further steps would improve this approach and further enhance our understanding of the public health implications of prisoner reentry: • Use street addresses for more precision in identifying concentrations of parolees; more detailed data would allow policymakers to fine-tune their understanding of which communities are disproportionately affected by reentry and the health care capacity of these communities. Summary xxi • To better understand the health care needs of the parolee population and inform planning, survey inmates who are about to be released and track them over time. • Improve data collection on capacity measures for the hospitals, clinics, substance abuse, mental health, and other relevant facilities that represent the potential safety net for parolees, an approach that will, in turn, improve the accuracy of accessibility measures. • Establish an expert panel to make recommendations on how to refine the definition of the safety net for this population as a way of helping policymakers better understand and facilitate care. Acknowledgments This work was sponsored by The California Endowment. We are particularly grateful for the guidance and feedback provided throughout the project by our project officer, Steve Eldred. We owe a huge debt of gratitude for the insights provided by our technical reviewers— Professor Jeff Mellow of the Department of Criminal Justice at John Jay College of Criminal Justice, City University of New York, and Carole Gresenz, senior economist at RAND. Their thoughtful review and feedback served to strengthen this report. We also wish to thank Christopher Mumola and Lauren Glaze of the Bureau of Justice Statistics for their input on the analyses of the mental health and substance abuse survey data. We also would like to acknowledge Keesha Johnson at the Regional Congregations and Neighborhood Organizations. We received input from a number of reentry stakeholders throughout California—too numerous to mention here—but we gratefully acknowledge their time and valuable insights. At RAND, we greatly benefited from the editing and publications production support provided by Lauren Skrabala and Stacie McKee. We also thank Christopher Dirks for his support in preparing the document. Finally, while we appreciate the improvements provided by all who reviewed and commented on this document, we emphasize that the findings and recommendations, as well as any errors, are those of the authors alone. xxiii Abbreviations 2SFCA two-step floating catchment area ADFLC alcohol- and drug-free living center ADP Alcohol and Drug Program ATP ability to pay BJS Bureau of Justice Statistics CASC community assessment service centers CBO community-based organization CDCR California Department of Corrections and Rehabilitation DSM-IV Diagnostic and Statistical Manual of Mental Disorders, fourth edition ECA Epidemiologic Catchment Area EOP Expanded Outpatient Program FBO faith-based organization FIPS Federal Information Processing Standard FPL federal poverty level FQHC Federally Qualified Health Center FTE full-time equivalent FY fiscal year GED General Education Development GIS geographic information system HPDP RAND Health Promotion and Disease Prevention Program ISE RAND Infrastructure, Safety, and Environment MHSA Mental Health Services Act MISP Medically Indigent Services Program xxv xxvi Understanding the Health Implications of Prisoner Reentry in California, Phase I Report NCCHC National Commission for Correctional Health Care NESARC National Epidemiologic Survey on Alcohol and Related Conditions NNH nearest neighbor hierarchical ODFC outpatient drug-free counseling ORSA Outpatient Reduced-Cost Simplified Application OSHPD California Office of Statewide Health Planning and Development PCC primary care clinic POC Parole Outpatient Clinic PPN Prison Parolee Network PPP public-private partnership PSN Parolee Services Network PTSD post-traumatic stress disorder SACPA Substance Abuse and Crime Prevention Act SAMHSA Substance Abuse and Mental Health Services Administration SASCA Substance Abuse Coordinating Agencies SLE sober-living environment SPA service planning area SSDI Social Security Disability Insurance STD sexually transmitted disease TB tuberculosis UC University of California UCLA University of California, Los Angeles USC University of Southern California Chapter One Introduction Background The explosive growth of the prison population in the United States has been well documented. In his aptly titled report But They All Come Back, Jeremy Travis (2005) made a compelling case for the consequences to communities when parolees return to the general population. He estimated that, given trends in incarceration at the time of his study, nearly half a million people would be released from prison and return to neighborhoods across the country—an enormous shift compared to the fewer than 170,000 prisoners released in 1980 (Travis, 2005). This increase in the number of ex-prisoners returning to communities comes at a time when traditional mechanisms for managing reentry have been significantly weakened (Travis, 2005). Travis notes that, given underfunded parole agencies in many communities, parole has become more of a legal status than a systematic process for reintegrating those returning to the community. Further, parole violations in the past eight years have driven much of the prison growth in the United States, with parole violators representing 34 percent of all admissions, double the percentage in 1980 (Travis, 2005). One disturbing trend is the recognition that prison is a revolving door in that many of those released will be returned to prison within several years. What is occurring nationally is mirrored by trends in California. Over the past 20 years, the number of individuals released from California prisons has increased nearly threefold. Between 1986 and 2006, California’s state prison population grew from 59,000 inmates to 173,000 inmates (California Legislative Analyst’s Office, 2007). The growth in the prison population from 1990 to 2005 alone was three times faster than that of the general adult population in California (Bailey and Hayes, 2006). In addition, 70 percent of those released from California prisons will be reincarcerated within two years of their release, with parole violations being an important reason for recidivism. Further, the racial, ethnic, and demographic composition of California prisons has changed dramatically over the past 40 years. Between 1964 and 1984, African-Americans and Latinos were incarcerated in increasingly higher numbers, while the number of white inmates has increased only somewhat (Petersilia, 2006). In 2007, Latinos constituted the largest group in the California prison system. The prison population is also changing demographically. The share of inmates age 50 or older has more than doubled during this period. In addition, female prisoners represent a growing proportion (currently 11 percent) of the state’s prison population (CDCR, 2008). These trends are important for any number of reasons, least of which is that those returning from prison have multiple needs, including employment, housing, and social services. 1 2 Understanding the Health Implications of Prisoner Reentry in California, Phase I Report Often overlooked among these needs is the role that health care plays in influencing the success of reintegration of those returning from prison: ”With 1,600 individuals walking out of prison gates every day, the health needs and risks of returning prisoners are central to the reintegration process” (Urban Institute, 2002). A retrospective cohort study by Binswanger et al. (2007) highlighted that individuals released from prison have substantial health risks with higher mortality rates than the general population. The most important finding was that former inmates had a high risk of death, particularly during the first two weeks following release, with the leading causes of death being drug overdose, cardiovascular disease, homicide, and suicide. For its landmark 2002 study, The Health Status of Soon-to-Be-Released Inmates: A Report to Congress, the National Commission for Correctional Health Care (NCCHC) commissioned a series of papers to summarize what is currently known about the health status of prisoners and to examine the implications for their treatment needs upon release from prison and for the correctional treatment and community health care systems. The study culled all available data sources to derive prevalence estimates on infectious diseases, chronic diseases, and mental health disorders.1 Despite important methodological challenges, the NCCHC study represents the best and most comprehensive source of information on the health status of inmates. The NCCHC study found that the prison population is disproportionately sicker on average than the U.S. population in general, with substantially higher burdens of infectious diseases (such as HIV/AIDS, tuberculosis, and hepatitis B and C), serious mental illness, and comorbidities, or co-occurring disorders (NCCHC, 2002). The NCCHC did not estimate the prevalence of substance abuse and dependence among state prisoners. With regard to health status, there is no comprehensive source of data on parolees or the reentry population. Instead, a number of individual studies have been undertaken, with a tendency to focus on specific health conditions (e.g., HIV rates) and select small samples of parolees. Data are somewhat better for the inmate population. The most important source of information on the self-reported health care needs of state prisoners is a national survey conducted by the Bureau of Justice Statistics (BJS). Mallik-Kane and Vischer (2008) provide important insights into how health care impacts the process of reentry. They conducted a survey of Ohio and Texas state prisoners during 2004 and 2005 that asked prisoners about their health status, treatment received, and how health has impacted the reentry process. In addition, they conducted interviews with these individuals shortly before release and up to three times following their release. They found that most returning prisoners were without health insurance (68 percent of men and 58 percent of women) eight to 10 months after release. Yet, these individuals also were heavy users of health care services by way of emergency room visits and hospitalizations. “Although returning prisoners received these health services, their rates of treatment for specific health conditions deteriorated, suggesting that they received episodic care for acute problems but that continuous treatment of specific health conditions suffered” (Mallik-Kane and Vischer, 2008, p. 2). In addition, Mallik-Kane and Vischer found that health influenced reentry outcomes. Individuals with physical and mental health problems reported poorer employment outcomes than those without such problems. Mentally ill men and women and substance-abusing women were more likely to be homeless upon release from prison. Individuals with substance abuse 1 Prevalence estimates for substance abuse and dependence were not part of the study. Introduction 3 problems reported more criminal behavior, having been more likely to earn money through illegal activities. Having any type of health condition was associated with engaging in criminal activity or a higher likelihood of being reincarcerated. In their sample, one out of five individuals was reincarcerated in state prison within a year of release. What do such insights mean for California? Most of the state’s prisoners ultimately will return to California communities, bringing with them a variety of health and social needs that must be addressed. Yet, the public is largely unaware of the health needs of parolees, and the challenges they present to their communities are not being addressed in an explicit manner, despite the fact that reentry directly affects almost every California community. Further, correctional health care in California has been declared unacceptable and since June 30, 2005, has been under a court-appointed federal receiver. The lack of quality health care provided to California’s prisoners and lack of communication with prisoners about their health issues upon release allow them to carry their health problems into the communities that receive them. The release of increasing numbers of individuals from prison raises serious public health concerns. In addition to being disproportionately sicker than the general population— particularly with chronic conditions, such as diabetes or hypertension, as well as such highly contagious diseases as tuberculosis, HIV/AIDS, and hepatitis C—the prison population is aging. In essence, it is undergoing a “demographic and epidemiologic transition” like that seen in many developing countries. In such transitions, the burden of disease shifts from infectious to chronic diseases. Because of this transition, the burden of chronic diseases, such as diabetes and hypertension—typically associated with older people but already higher than what one would expect in the prison population—is likely to grow. Further, a disproportionate share of these individuals will likely be released into disadvantaged communities and neighborhoods in California with limited health care and social services and an already overextended safety net. The explosion of the California prison population and the infectious and chronic disease profiles of this population raise some key public health challenges, including the need for improved screening, prevention, and treatment programs for state prison inmates; better tracking systems and databases to allow for effective coordination between the correctional health care and public health systems; and improved discharge and transitional planning for those with special health care needs. Further, the involvement of community-based organizations in linking prison and community health systems to facilitate reintegration into the community is essential (Urban Institute, 2002). Little data exist on the specific health needs of California parolees or the kinds of services they receive when they are released into the community. The nationwide Survey of Inmates in State and Federal Correctional Facilities, conducted by BJS every five to six years, provides the best available data on California prison inmates and can serve as a proxy for parolees’ health care needs (see BJS, 2009). Further, the BJS inmate survey allows researchers and policymakers to compare California inmates’ health needs relative to those of the overall U.S. state prison population. Selected mapping projects in California provide insights for several counties as to the distribution and concentration of parolees (e.g., Heiser and Williams, 2008). However, no single study has comprehensively examined which counties and communities are disproportionately affected by reentry or the link between the health care needs of parolees in California and how well the health care safety net in affected communities is able to meet their needs. Such a study is a much-needed step to enable better policy and planning decisions for this population. 4 Understanding the Health Implications of Prisoner Reentry in California, Phase I Report Objective and Scope This study, funded by The California Endowment, is part of a two-phase effort examining prisoner reentry. This report presents the results of Phase I, which examines public health issues surrounding prisoner reentry in California, including the type of health care needs that exoffenders bring with them, which communities are disproportionately affected, and the health care system capacity of those communities to which ex-offenders return. In Phase II, we will examine in depth the challenges that parolees face in meeting their health care needs and factors that have influenced service providers’ ability to extend care to this population. We addressed the following three research questions in Phase I: 1. What are the health care needs of prisoners upon their release and return to the community? 2. What is the geographic distribution of state prisoners who return to local communities in California? 3. What types of health care services are available in these communities, and what is their ability to meet the needs of returning prisoners? In terms of the study’s scope, we considered a wide range of health care needs, including physical health, mental health, and substance abuse. We decided to focus exclusively on the male parolee portion of the reentry population for two reasons. First, the vast majority of inmates and returnees in California are male; as such, their characteristics are likely to dominate our findings. Second, we recognize that females represent a growing, but unique, share of the reentry population. However, because their socioeconomic, health, and other concerns are likely to differ in important ways from those of their male counterparts, we decided that it was not feasible in this report to comprehensively address the public health issues and needs of this special population. Further, it was not feasible to disaggregate California female parolees by race and ethnicity because the samples become quite small. In addition, we focused only on parolees’ potential access to health care and, specifically, to safety-net resources (i.e., having health care resources within a reasonable distance and the capacity of those resources to meet the broader community’s demand). We could not address realized access to health care (e.g., actual use of health care), because the data do not exist for parolees. We also did not have the data to address access outcomes, such as whether parolees who received services have better treatment and criminal justice outcomes as a result. Finally, while we consider the health concerns of inmates and the concentration of parolees statewide, the majority of the analyses in this report focus on four counties: Alameda, Kern, Los Angeles, and San Diego. These four counties were chosen because they represent a substantial share of the state’s parolee population and because they allow us to compare urban and rural counties, which are likely to present different issues for policymakers. Introduction 5 Approach The approach we used to answer the three research questions is discussed in more detail in the body of this report and in some of the appendixes. Here, we briefly summarize the approaches that we used. To examine the first research question, we conducted a state-level analysis using data for California from a national survey of state prison inmates to examine the range of health care needs reported by prisoners as a proxy for understanding the health care needs of the reentry population. Specifically, we used the BJS 2004 Survey of Inmates in State and Federal Correctional Facilities sample based on all state prison inmates who resided in California. For the second research question, we used geocoded corrections data for parolees released in 2005 and 2006 from California state prisons and cluster analysis to examine the geographic distribution of parolees and to identify concentrations of parolees at the state and county levels. Specifically, we used the California Department of Corrections and Rehabilitation (CDCR) data file of parolees released in calendar years 2005 and 2006 from California state prisons. To address the third research question, we focused on four counties with a significant share of parolees: Los Angeles, Alameda, Kern, and San Diego. We drew on publicly available facility data for hospitals, clinics, mental health providers, and substance abuse services to characterize the health care, mental health, and substance abuse treatment safety nets in these counties for the uninsured population and for the parolee population. We also developed measures to assess the accessibility of the safety net in those areas with a high concentration of parolees. Limitations While we made an effort to advance our understanding of parolee needs and access, there were some limitations to this study. First, we did not have any information about the health care needs of the parolee population in California. As a result, we had to rely on data for a proxy population, in this case, a survey of state prison inmates. While useful, these data only allowed us to assess the needs of the reentry population at the state level, because the survey did not include geographic identifiers for county. Second, the ideal approach to geographic mapping and geographic information system (GIS) techniques is to use the exact residential address of the individual (parolee) and the facilities of interest. We requested this information, but CDCR only provided us with the census tract locations of parolees. As a result, we placed each parolee in the population-weighted centroid of his census tract. This approach may have had some implications for identifying clusters of parolees. Without the residential addresses, we were also unable to assess the accuracy of census tract geocodes and address the substantial number of invalid or missing codes. The lack of data on the residential addresses of the parolees also affects our accessibility measures. For our measure of accessibility, we selected a 10-minute drive time, which is calculated from the facility’s exact address to the population centroid of a parolee’s census tract (in lieu of the residential address). That said, our measure of accessibility is also influenced by other factors. This measure takes into account driving distance, capacity, and potential demand. If one were to increase the acceptable drive time or use a different measure of potential demand 6 Understanding the Health Implications of Prisoner Reentry in California, Phase I Report and capacity, a different set of results might be obtained. In some cases, we were able to assess the sensitivity of our results to these factors. Organization of This Report This report is organized as follows. Chapter Two presents the results from our analysis of the first research question, which provides some context for the chapters that follow. In particular, it examines the socioeconomic characteristics, health care needs, and access to care of California prison inmates. Chapter Three presents the results of our analysis of the second research question, describing the distribution of parolees in California and in the four counties with the largest share of parolees—Los Angeles, Alameda, Kern, and San Diego—which are the focus of our subsequent analyses. Chapter Three also provides a summary of the socioeconomic characteristics of the communities to which parolees return as well as a summary of the sociodemographic characteristics of parolees during the study period (2005–2006). Chapter Four provides the analytical underpinnings for the third research question. In particular, it presents the underlying conceptual framework that motivates the analysis and discusses the specific methodology used to determine health care, mental health, and substance abuse safety nets in the four counties and to develop access measures. Chapters Five and Six present the results of our analysis of the third research question, which examines the four counties’ health care, mental health, and substance abuse safety nets and their ability to meet the health care needs of the reentry population. Chapters Five and Six both construct accessibility measures for the safety-net resources and examine how accessibility varies for the parolee population by type of health care resource and by race and ethnicity. Finally, Chapter Seven summarizes the analytic findings, provides some implications for policymakers, and discusses some steps to improve understanding of these issues. It also briefly discusses what will be done in Phase II of the study. Appendix A presents additional maps showing the clustering of parolees at the county level by count of parolees (rather than rate), and Appendix B discusses in greater detail the methods used in this study. Appendix C presents additional tables describing the mental health and substance abuse characteristics of the inmate population. Appendix D includes additional findings on the health problems of California inmates that were not presented in Chapter Two. Appendix E contains alternate measures of accessibility for hospitals and clinics and provides the results of our analysis of the sensitivity of geocoding to missing zip code data. Chapter Two Socioeconomic and Health Characteristics of California State Prisoners To understand the health needs of parolees, it is important to examine the broader trends with respect to the reentry population as a whole and the changing characteristics of individuals incarcerated in state prisons. Such trends as an aging prison population, longer prison stays, and an increase in the number of released prisoners who are former drug offenders—as well as disparities in the racial and ethnic composition of state prison inmates and parolees—have implications for the health care needs of parolees and the public health challenges such individuals represent to local communities. A recent study by the Urban Institute found that the prevalence of physical, mental health, and substance abuse problems among their sample of 1,100 returning prisoners was substantial (Mallik-Kane and Visher, 2008). Furthermore, the authors found that the reentry population’s physical and social-behavioral conditions influenced their reentry experiences. In this chapter, our goal is to increase our understanding of the socioeconomic characteristics, physical health care needs, and social and behavioral needs of California parolees and how they vary across racial/ethnic groups. Methods and Data Sources To examine parolees’ needs, it was necessary to obtain detailed socioeconomic and health information on a large and representative sample of parolees nationally and in California. It is, however, very difficult to do so. Therefore, as a proxy, we used (as discussed in Chapter One) self-reported data from the 2004 Survey of Inmates in State and Federal Correctional Facilities conducted by the BJS (see BJS, 2009). All estimates in this chapter are based only on responses by males incarcerated in state prisons.1 The BJS inmate survey provides a rich resource for understanding the potential needs of the reentry population, but we should keep in mind that inmates are an imperfect proxy for parolees released in a particular year. Most individuals who are incarcerated in state prisons will eventually be released, but certain types of offenders serve longer sentences and so are more prevalent in the incarcerated population than in a given parolee population (e.g., violent offenders). However, we thought it would be useful to include the full sample of incarcerated individuals in our analysis because most will eventually be released and need care in the community.2 1 Federal prisoners are excluded. 2 Only 1 percent of the sample of California inmates in the BJS survey had been sentenced to life without parole. 7 8 Understanding the Health Implications of Prisoner Reentry in California, Phase I Report We compared the BJS sample to the CDCR parolee population on available demographics and criminal justice involvement to assess how much these populations differ (see Table B.1 in Appendix B). As discussed in Appendix B, the samples were similar in terms of race/ethnicity composition, average age, and gender. In terms of differences, we found that a higher percentage of surveyed inmates reported being incarcerated for a violent offense than was the case for parolees in our sample (48 percent versus 23 percent). Conversely, a third of parolees had property crimes and a third had drug crimes as their controlling offense, a higher share than was reported by the inmates surveyed (25 percent versus 20 percent, respectively). In addition, the average sentence length in the inmate survey sample was three times as high, averaging nine years compared to three years for the parolee sample. Finally, some differences may also result from the fact that the parolee sample was based on the individual’s recorded controlling offense, whereas the inmate data were self-reported. We do not have any information, however, to compare the health needs of these populations, and so we focus on the entire BJS sample. We rely primarily on data for those inmates who lived in California before their arrest but also make comparisons to the U.S. state prison population.3 Specifically, this chapter makes the following comparisons and assesses the statistical significance of differences between (1) U.S. males and California males4 and (2) California males by race/ethnicity. Racial/ethnic groups are defined according to four categories: white non-Hispanic, African-American non-Hispanic, Latino, and other. A drawback of the BJS data relative to the CDCR administrative data is that we cannot examine geographic variation below the state level. The tables in this chapter show the estimates for U.S. males, California males, and each racial/ethnic group. Statistically significant differences between U.S and California males and between white male inmates and the other three other racial/ethnic groups in California are identified in bold. The accompanying text focuses mainly on statistically significant differences. It is important to note that statistical significance is a function not only of the size of observed differences across groups but also the sample size and resulting precision of those estimates. For many of areas of interest, the inmate survey gives us multiple measures of the issues. The approach taken here is to keep the summary measures in the body of the document and then present the elements that contribute to those measures in Appendixes C and D. For example, we include the share of inmates who are substance-dependent/abusing in the main body of the report. But substance dependence is constructed based on responses to elements, such as symptoms of withdrawal, tolerance, and other factors that we include only in appendix tables. Major differences in these components are discussed in the main body, when relevant, with a reference to the appendix tables. 3 State of residence is not available in the public-use dataset, but there is considerable overlap between state of residence and state of arrest. 4 The U.S. statistics include California inmates, who account for approximately 12 percent of the total unweighted sample. Socioeconomic and Health Characteristics of California State Prisoners 9 Socioeconomic Characteristics of California State Prison Inmates This section describes the demographic and socioeconomic characteristics of male inmates in California state prisons. The goal is to describe these inmates and their backgrounds to better understand the potential needs of parolees as they reenter society. To examine disparities within the male inmate population, we tabulate summary statistics for U.S. inmates, for California inmates, and for four major racial/ethnic groups in California. Where relevant, we discuss how inmates compare to the general population in California to give the reader an understanding of where inmates are at a particular disadvantage. Specifically, we focus on those issues that are likely to influence whether inmates will be able to successfully reenter society and are likely to influence their health status and access to care. These factors fall into four broad categories: (1) demographics; (2) education, employment, and military service; (3) family ties and adverse life events; and (4) criminal history. Basic Demographics In this section, we examine age and nativity among the prison population (see Table 2.1). One concern is that older inmates may have more and different health needs than younger inmates. Also, foreign-born inmates may have more difficulty accessing health and other services needed to reintegrate successfully, for example, because of language limitations. As expected, the inmate population is relatively young overall. The average age of inmates in the United States was 35 years, and the average age of California inmates was 36 years. The only statistically significant difference in demographic comparisons was that white inmates were somewhat older than Latino inmates in California (38 years old versus 34 years old, respectively). The distribution of inmates by age likewise shows that Latinos are more likely to be in the younger age groups. Table 2.1 Basic Demographics By Race/Ethnicity (California) Total U.S. Total California White AfricanAmerican Latino Other Mean 35.34 35.86 38.23 36.62 33.57 34.57 Under 25 (%) 17.57 15.38 9.49 14.15 20.38 19.83 25–34 (%) 33.22 31.88 27.59 26.50 38.28 40.97 35–44 (%) 29.90 33.14 38.78 37.81 26.79 19.28 44–54 (%) 14.09 14.36 17.03 15.72 11.38 13.15 5.22 5.24 7.11 5.82 3.16 6.77 8.45 16.23 3.93 1.83 32.72 51.44 Characteristic Age 55 and over (%) Foreign-born (%) Source: BJS 2004 inmate survey. Note: Sample includes male inmates incarcerated in state prisons only. Statistical significance is denoted by bold (California versus United States; race/ethnicity comparisons are African-American, Latino, and other versus white). 10 Understanding the Health Implications of Prisoner Reentry in California, Phase I Report California has a large foreign-born population. More than a quarter of Californians (27.2 percent) are foreign-born compared with 12.4 percent of the U.S. population (U.S. Census Bureau, 2008, Table 40). This difference is reflected in the inmate population. Nationally, only 8 percent of inmates are foreign-born. In California, that share is twice as high (16 percent)— a statistically significant difference relative to the United States. In both cases, however, the foreign-born are underrepresented in the inmate population. Not surprisingly, foreign-born status is significantly higher among Latino inmates in California (33 percent) and among other race/ethnicity (51 percent). Nearly all inmates reported that they were U.S. citizens; there were no significant differences across racial/ethnic groups (not shown). Education, Employment, and Military Service Offenders may face difficult labor markets after their release because of the stigma and erosion of job-market skills, although the causal evidence for income is greater than for employment (e.g., see Western, King, and Weiman, 2001). Work that is available to parolees is often unskilled, with restricted opportunities to advance (Petersilia, 2006). How this relates to the financial support of parolees in California is illustrated by analyses conducted by Frank Williams of a representative sample of 4,047 California parolees released in 1997 whose “street time”5 was tracked for 12 months and who were asked about their employment experience (Petersilia, 2006). During the first year of release from prison, only 22.7 percent of California parolees reported employment as their primary financial support, 32.1 percent reported they were supported by friends and family, 13.2 percent reported support by unlawful means, 11.7 percent reported relying on public assistance, 0.6 percent were supported by social security, and the remainder reported other means of support (Petersilia, 2006, p. 68). In this section, we explore inmates’ education levels, employment experiences, income sources, and military service because these characteristics are likely to have implications for inmates’ labor-market outcomes upon release. While employment itself is clearly an important part of successful reentry, it can also influence access to care through insurance coverage and, consequently, parolees’ health. Lower academic achievement, and particularly the absence of a high school diploma or General Education Development (GED) credential, can have negative implications for labormarket opportunities and, consequently, successful reentry into the community. The educational attainment of prison inmates compares poorly with that of the general population even after we disaggregate comparisons by race/ethnicity. About nine out of 10 native-born Californians have a high school degree, compared with only half of noncitizens and three-quarters of naturalized citizens (California Department of Finance, 2007). Similarly, among adult Californians over age 25, about 93 percent of whites have a high school diploma, compared to only 87 percent of African-Americans and 55 percent of Latinos. In analyzing our sample of surveyed inmates, we found that inmates had substantially lower educational attainment than the general California population (see Table 2.2). Less than two-thirds of inmates (63 percent) reported completing high school or earning a GED. California inmates were less likely to have obtained a diploma or GED (60 percent) than inmates nationally. The lower educational attainment of inmates in California appears to be driven by statistically lower achievement by Latino inmates, among whom only 45 percent 5 Parolees’ street time was tracked for a cumulative 12 months; if parolees had their parole revoked and returned to prison, they were tracked again upon the next parole release until they accumulated a total of 12 months’ street time. Socioeconomic and Health Characteristics of California State Prisoners 11 Table 2.2 Education, Employment, and Military Experience By Race/Ethnicity (California) Total U.S. Total California White AfricanAmerican Latino Other High school diploma or GED (%) 63.43 60.29 75.97 63.16 45.48 64.45 High school diploma (%) 33.33 39.84 50.92 45.38 26.00 49.30 GED (%) 36.15 27.28 33.56 25.79 23.82 24.84 73.50 68.56 69.02 58.53 75.16 74.68 68.80 62.94 63.42 54.69 68.46 65.82 Welfare (%) 4.32 4.88 3.27 6.68 4.94 3.40 SSDI (%) 5.46 6.15 7.88 7.77 3.72 5.15 41.84 40.57 47.37 46.94 30.88 38.89 11.05 9.68 18.39 9.75 2.99 8.48 Characteristic Education Employment Employed in the month prior to arrest (%) Income source in the month prior to arrest Employment (%) Other source (%) Military experience Veteran (%) Source: BJS 2004 inmate survey. Note: Sample includes male inmates incarcerated in state prisons only. Statistical significance is denoted by bold (California versus United States; race/ethnicity comparisons are African-American, Latino, and other versus white). SSDI = Social Security Disability Insurance. reported having obtained a high school degree or GED; however, African-American inmates also reported significantly lower achievement (63 percent) compared to white inmates in California (76 percent). When we disaggregate educational attainment, we find that California inmates were significantly more likely to have completed high school than U.S. inmates overall (39 percent versus 33 percent), implying that the educational difference between these two groups is the result of significantly lower GED attainment. In terms of racial/ethnic disparities, one of the most dramatic differences was that Latino inmates in California completed high school at only half the rate of their white counterparts (26 percent versus 51 percent). Latinos also obtained GEDs at a rate that was 10 percentage points lower than whites (24 percent versus 34 percent). Education is not the only determinant of labor-market success. Previous employment and military experience can also provide the skills necessary for inmates to successfully reenter the workforce. The inmate survey provides us with two imperfect measures of labor-force attachment: (1) employment (or business ownership) in the month prior to arrest and (2) income from employment prior to arrest. While these are interesting insights into inmates’ activities at a point in time, they do not tell us whether inmates have sufficient skills and experience to reenter the workforce. Both measures indicate that, while the majority of inmates have 12 Understanding the Health Implications of Prisoner Reentry in California, Phase I Report some labor-force experience, a substantial share of inmates were not employed prior to their most recent arrest (see Table 2.2). Nationally, less than three-fourths of inmates (74 percent) were employed in the month prior to their arrest.6 California inmates fared even more poorly. California inmates were significantly less likely to have been employed in the month prior to arrest (69 percent). The employment status of California inmates prior to arrest also compares poorly with that of their counterparts in the noninstitutional population in California, among whom nearly 77 percent were employed in 2005.7 Among California inmates, Latino inmates had greater employment participation (75 percent) than white inmates (69 percent) while African-American inmates had the lowest (59 percent). A related question is whether the inmates received income from these employment activities. Not surprisingly, the results for income from employment are similar to those for employment. Nationally, more than twothirds of inmates reported earnings from employment in the month prior to arrest. Following the results for employment, the prevalence of income from employment was significantly lower among California inmates (63 percent). While there were no statistically significant differences across racial/ethnic groups, the pattern is similar to that observed for employment. Latino inmates had the greatest share of inmates who received income from employment at the time of their arrest, followed by white inmates and then African-American inmates. Of course, employment is not the only source of potential income. Inmates were also asked whether, in the month prior to arrest, they received any income from welfare, SSDI, or from other sources. Overall, nearly 5 percent of California inmates were receiving welfare benefits and 6 percent were receiving SSDI (Table 2.2). These results can be viewed in two ways. On the one hand, the findings imply that a substantial number of inmates already relied on public assistance prior to their current incarceration. On the other hand, it also means that they are aware of the resources that may be available to them upon release.8 Income from welfare and SSDI was quite low nationally (4 percent and 5 percent, respectively) and in California (5 percent and 6 percent, respectively). It is unclear to what extent previous criminal involvement limited inmates’ access to these resources. Serving in the military may also provide inmates with experiences that will be useful in the labor force. As veterans, they may also be eligible for health care benefits from the U.S. Department of Veterans Affairs upon release from prison. Overall, the share of U.S. inmates with military experience was 11 percent. California inmates had similar rates of military service (10 percent). In California, African-American inmates were only about half as likely (10 percent) and Latino inmates only one-sixth as likely (3 percent) to have served in the military relative to white inmates (18 percent). 6 The question used to define this characteristic was “During the month before your arrest [CONTROLLING ARREST DATE], did you have a job or a business?” (BJS, undated). 7 The employment population ratio is for the male population aged 25 to 44 in California. We make a comparison to the employment population ratio rather than the employment rate because we do not know the share of inmates in the labor force (e.g., actively looking for work or not) (BLS, 2005). 8 There are limitations on the types of benefits for which ex-offenders may be eligible, which may vary by state (see, e.g., GAO, 2005). As a result, even inmates who are familiar with these programs may not be able to rely on them upon release. Socioeconomic and Health Characteristics of California State Prisoners 13 Family Ties and Adverse Life Events The effects of family and other ties to the community are complex and can positively or negatively influence reentry prospects. For example, having close family ties, such as spouse and significant other, has been shown to be an important predictor of successful reentry (Davis and Pacchiana, 2004). For example, two-thirds of California parolees indicated they had found housing in some sort of familial or quasifamilial setting during the first year of release from prison (Petersilia, 2006). Then again, substance abuse among family members and other negative influences can be a risk factor for subsequent involvement with the criminal justice system (Travis and Waul, 2003). Surveyed inmates were asked about spouses, minor children, living arrangements prior to arrest, and the criminal justice involvement of immediate family members. We found evidence of potentially positive and negative influences on inmates (see Table 2.3). We also consider the prevalence of adverse life events, such as homelessness and abuse, which are potentially signals of difficult family ties or other trauma and which may also complicate reentry and require additional health services. Table 2.3 Family Ties and Adverse Life Events (percent) By Race/Ethnicity (California) Total U.S. Total California White AfricanAmerican Latino Other Currently married 16.32 21.01 14.21 22.68 24.75 23.27 Minor child(ren) 51.18 51.69 46.13 49.12 59.30 40.17 Lived in family unit in the month before arrest 81.13 78.72 73.96 78.18 82.20 83.99 Lived with minor child(ren) in the month before arrest 21.68 21.14 17.18 18.76 25.73 23.70 Lived with minor child(ren) in the month before arrest (if any) 42.37 40.90 37.25 38.19 43.39 59.00 47.10 46.47 42.10 55.71 45.42 24.54 Ever homeless 10.12 16.44 20.21 15.44 14.97 10.54 Ever abused 16.79 15.54 23.96 14.85 9.91 12.29 13.80 12.01 18.19 11.22 7.86 11.87 6.24 5.82 9.80 5.49 3.45 1.80 Characteristic Family ties Criminally involved family Adverse life events Physically abused Sexually abused Source: BJS 2004 inmate survey. Note: Sample includes male inmates incarcerated in state prisons only. Statistical significance is denoted by bold (California versus United States; race/ethnicity comparisons are African-American, Latino, and other versus white). 14 Understanding the Health Implications of Prisoner Reentry in California, Phase I Report Inmates, especially Latinos, had significant attachments to their families and household units. Most inmates in the United States (81 percent) and in California (79 percent) lived in a family unit prior to their arrest.9 The rates were significantly higher for Latino male inmates in California relative to white male inmates (82 percent versus 74 percent). Larger differences were observed with respect to marital status. California inmates were significantly more likely to report being married at the time of the survey than inmates nationally (21 percent versus 16 percent, respectively). This appears to be driven partly by large differences across racial/ethnic groups. Latino inmates (25 percent) and, to a lesser extent, AfricanAmerican inmates (23 percent) were significantly more likely to report being married than white inmates (14 percent). In the BJS survey, approximately half of inmates nationally (51 percent) and in California (52 percent) had minor children at the time of the interview. Approximately 21 percent of California inmates (or 41 percent of those who reported minor children at the time of the interview) had been living with their children at the time of their latest arrest. Among inmates in California, Latino inmates were more likely to have a minor child relative to white inmates (59 percent versus 46 percent) and more likely to have been living with the minor child (26 versus 17 percent). The family characteristics discussed thus far are likely to positively influence a parolee’s behavior. But not all family influences are positive. The influence of other family members who are involved in the criminal justice system is ambiguous. On the one hand, it may help the parolee to have someone with a common experience who has perhaps successfully reintegrated into society. On the other hand, if those family members are involved in ongoing criminal activity, this may represent a significant risk factor for the parolee. In our analysis, nearly half of U.S. (47 percent) and California (46 percent) inmates had a sentenced or incarcerated parent, sibling, spouse, child, or other family member. The rate was significantly higher among African-American inmates relative to white inmates (56 versus 42 percent), but significantly lower among inmates of other race/ethnicity (25 percent). Inmates experienced a number of other adverse life events, including homelessness and physical or sexual abuse. In terms of homelessness, CDCR officials estimate that, overall, 10 percent of the state’s parolees were homeless in 1997, and in urban areas, such as San Francisco and Los Angeles, the rate was estimated to be as high as 30 percent to 50 percent (Petersilia, 2006). Further, 6.5 percent of parolees reported being mostly homeless for the entire first year after prison release (Petersilia, 2006). About 7 percent of the U.S. population reported that they had been homeless at some point in their lives (Link et al., 1994). The probability of experiencing homelessness was higher among inmates, particularly in California (Table 2.3). Nationally, 10 percent of inmates reported being homeless at some point, while among California inmates, the corresponding share was significantly higher at 16 percent. There were no statistically significant differences across racial/ethnic groups. Nearly 17 percent of inmates nationally and 16 percent in California also reported having experienced either physical or sexual abuse. In California, a reported history of abuse was significantly less common among Latino (10 percent) and African-American (15 percent) inmates relative to white inmates (24 percent). When the type of abuse was examined separately, only 9 The question used to define this characteristic was “In the month before your arrest on [CONTROLLING ARREST DATE], was anyone living with you?” (see BJS, undated). Socioeconomic and Health Characteristics of California State Prisoners 15 differences between Latino inmates and white inmates for both types of abuse and between other race/ethnicity and white inmates for sexual abuse only were statistically significant. Criminal History The parolee’s own degree of criminal involvement is likely to be one of the greatest influences on his ability to successfully integrate into society. To the extent that churning in and out of the prison system influences access to care or disrupts continuity of care, there may also be health implications. Factors related to criminal involvement can include the nature of the controlling offense for which the parolee was most recently incarcerated as well as the number of previous arrests and incarcerations. Another consideration is the amount of time served in prison, which has increased in recent years. Between 1993 and 1998, the median time served to first release from prison increased from 12 to 18 months (Lynch and Sabol, 2001). Another study found that the share of those serving at least five years increased from 13 percent in 1991 to 21 percent in 1997 (Hughes and Wilson, 2002). Longer stays in prison have implications for successful reentry because inmates may lose contact with family members (or other associates) who might have facilitated their reintegration into the community (Davis and Pacchiana, 2004). In our analysis of state prison inmates, the mean sentence length was significantly higher nationally at 13 years, compared to just over nine years for California inmates (see Table 2.4).10 Although not statistically significant, in California, African-American inmates had somewhat longer sentence lengths (mean of 11 years), compared to white and Latino inmates, who had mean sentence lengths of nine years and eight years, respectively. Table 2.4 Criminal History By Race/Ethnicity (California) Total U.S. Total California White AfricanAmerican Latino Other Violent (%) 54.23 49.51 42.14 51.23 52.34 60.54 Property (%) 21.14 24.52 32.46 23.57 19.12 25.82 Drug (%) 20.07 19.49 17.59 20.67 21.14 9.97 Other (%) 4.57 6.48 7.80 4.53 7.40 3.67 Sentence length (years) (mean)a 13.36 9.34 8.66 11.22 8.32 11.55 Previous arrest (%) 84.84 86.34 89.48 89.17 83.86 67.45 Characteristic Type of offense Source: BJS 2004 inmate survey. Note: Sample includes male inmates incarcerated in state prisons only. Statistical significance is denoted by bold (California versus United States; race/ethnicity comparisons are African-American, Latino, and other versus white). a Excludes those with categorical sentences such as life or life without parole. 10 These calculations exclude inmates with categorical sentences (e.g., life). 16 Understanding the Health Implications of Prisoner Reentry in California, Phase I Report Both sentence length and potential success of reentry are influenced by the nature of the offense that resulted in incarceration. California inmates were slightly less likely to be incarcerated for a violent crime (50 percent) than inmates nationally (54 percent). The prevalence of violent crime among inmates is likely driven partly by the fact that violent offenders spend more time in prison per offense (Durose and Langan, 2007). California inmates were more likely to be incarcerated for a property crime than U.S. inmates (25 percent versus 21 percent). The share of inmates incarcerated for drug crimes was nearly identical (20 percent nationally versus 19 percent in California). Finally, Californians were also more likely to be incarcerated for other offenses (6 percent versus 5 percent). In California, 42 percent of the white inmates had been incarcerated for a violent crime; there were statistically significant differences (relative to whites) only for Latino inmates (52 percent) and those reporting other race/ethnicity (61 percent). Latino inmates were less likely to be incarcerated for a property crime than whites (19 percent versus 32 percent). One out of five California inmates was incarcerated for a drugrelated offense with no statistically significant differences by race/ethnicity. The current incarceration was not the first or only encounter with the law for most of the surveyed inmates. Nationally, inmates had considerable experience with the criminal justice system. The vast majority of inmates in the United States (85 percent) reported having a previous arrest. In comparison, California inmates were slightly more likely to have had a previous arrest (86 percent). We found no significant racial/ethnic differences among California inmates with respect to previous arrests. The one exception was a significantly lower history of arrests for inmates reporting other race/ethnicity in California relative to their white counterparts (66 percent versus 89 percent). Physical Health Needs and Utilization Understanding the prevalence of disease among the California state prison population is important because it provides some insight into the likely health care needs of ex-offenders and the level and type of demand for health services that they will place on the health care safety net and public health system at the local level. Further, from a public health perspective, prevalence measures provide an indication of what types of diseases—especially infectious diseases—may be brought into a community upon reentry. In May 2002, the NCCHC released a report to Congress on the health status of soon-tobe-released inmates (NCCHC, 2002). Specific conditions in each of the disease categories— infectious disease, chronic disease, and mental illness—were examined. National estimates of the prevalence of diseases within each category were made for prisons and jails to quantify the burden of these conditions among inmates and parolees. We used these data to compare our results for California inmates based on the BJS survey. The BJS survey can provide some insight into the physical health needs of this population as well as their receipt of medical care while incarcerated. In terms of physical health, the survey asked inmates about any cardiac conditions, kidney problems, cancer, asthma, diabetes, hypertension, cirrhosis, hepatitis, paralysis, stroke, sexually transmitted diseases (STDs), tuberculosis, and HIV, among other conditions or problems. For most conditions, the BJS survey asked inmates about lifetime prevalence and prevalence during the current incarceration. Inmates who reported a condition were then asked whether they had seen a medical professional specifically for that condition. Socioeconomic and Health Characteristics of California State Prisoners 17 In this section, we first examine the prevalence of lifetime and current physical health conditions, infectious diseases, and physical disabilities among California state inmates and, where possible, discuss how they compare to rates observed among the general population. In Appendix D, we report the survey results for dental problems, disabilities, and mental health treatment for all inmates. Lifetime and Current Physical Health Problems Previous research suggests that inmates are more likely to suffer chronic conditions than the general population. For example, the prevalence of asthma among U.S. prison and jail inmates was estimated to be higher than it is among the general population (8.5 percent versus 7.8 percent; NCCHC, 2002).11 The prevalence of diabetes and hypertension was estimated to be lower than for the U.S. population in general, but the authors point out that the prevalence of these two conditions is relatively high after taking into account the fact that inmates tend to be younger (Hughes and Wilson, 2002). Because these conditions are typically associated with older people, one might expect them to increase in prevalence as the state prison population ages (Hammett, Roberts, and Kennedy, 2001).12 Nationally, the share of inmates reporting chronic health problems (lifetime and current) was substantial (see Table 2.5). By far, the most common conditions were hypertension (21 percent) and asthma (14 percent), though cardiac conditions (9 percent), kidney problems (6 percent), diabetes (5 percent), and cirrhosis (2 percent) were also reported. For the most part, the health of California inmates was similar to that of their counterparts nationally. There were also very few statistically significant differences within California. The only exceptions were a significantly lower incidence of kidney problems among AfricanAmericans (2 percent versus 8 percent among white inmates) and other race/ethnicity (2 percent versus 8 percent) and lower rates of cirrhosis among African-Americans relative to whites (1 percent versus 5 percent). The findings were similar when we looked at the current (versus lifetime) prevalence of these conditions. As with lifetime prevalence, the most common conditions reported were hypertension (14 percent) and asthma (8 percent). Other chronic and acute conditions currently experienced by inmates included diabetes (4 percent), kidney problems (3 percent), and cirrhosis (1 percent). California inmates reported similar health but were less likely to report hypertension (10 percent versus 14 percent nationally). By race/ethnicity, the only statistically significant differences were a lower incidence of cirrhosis among African-Americans (0 percent versus 3 percent among whites) and a higher incidence of hypertension among African-Americans relative to whites (17 percent versus 9 percent). 11 12 The NCCHC (2002) analysis did not produce separate estimates for inmates in prisons versus those in jails. According to BJS data, the average age of state prisoners being released on parole has been increasing (from 31 years of age in 1999 to 34 years of age in 1999). In 1999, 26 percent of state prisoners who were paroled were 40 years of age or older, compared to 14 percent in 1990. 18 Understanding the Health Implications of Prisoner Reentry in California, Phase I Report Table 2.5 Lifetime and Chronic Physical Health Problems (percent) By Race/Ethnicity (California) Total U.S. Total California White AfricanAmerican Latino Other Cardiac 9.12 7.94 9.13 9.32 6.30 5.11 Kidney 5.74 5.21 7.80 2.35 5.82 1.56 Cirrhosis 1.75 2.55 4.70 0.52 1.97 6.59 Asthma 13.88 13.70 14.28 17.94 10.87 6.72 Diabetes 4.49 4.52 3.51 6.70 3.38 6.85 20.73 18.27 17.35 24.64 13.65 22.07 Cardiac 5.98 4.67 5.55 6.08 3.06 3.40 Kidney 2.90 2.23 2.79 1.82 2.18 1.56 Cirrhosis 1.11 1.74 3.44 0.26 0.99 6.59 Asthma 8.38 8.83 10.07 10.73 6.90 5.04 Diabetes 3.85 3.78 3.00 5.70 2.79 5.10 13.60 10.54 8.61 16.55 6.71 16.86 Type of Problem Lifetime health problems Hypertension Current health problems Hypertension Source: BJS 2004 inmate survey. Note: Sample includes male inmates incarcerated in state prisons only. Statistical significance is denoted by bold (California versus United States; race/ethnicity comparisons are African-American, Latino, and other versus white). Infectious Diseases The existing literature suggests that the burden of infectious diseases among prison inmates and parolees is greater than it is in the U.S. population. The documented prevalence of active tuberculosis (TB) infection among prison inmates is estimated to be four times greater than among the total U.S. population. Among persons in the United States with active TB in 1996, an estimated 35 percent had served time in a prison or jail during that year. The prevalence of hepatitis C, the most common chronic blood-borne viral infection in the United States (Shuter, 2002),13 was estimated to be at least nine to 10 times higher among prison inmates than the U.S. population in general (Hammett, Harmon, and Rhodes, 2002). The authors note that this prevalence likely underestimates the true prevalence of hepatitis C among prison inmates. Therefore, it is striking that in 1996 approximately 29–32 percent of the estimated 4.5 million people infected with hepatitis C in the United States had served time in a prison or jail during that year (Hammett, Harmon, and Rhodes, 2002). The prevalence of AIDS among prison inmates is estimated to be five times greater than the prevalence among the U.S. population in general, while HIV infection is estimated to 13 Unlike hepatitis B, those infected with hepatitis C often remain chronically infected, with complications from hepatitis C infection accounting for approximately 1 percent of all deaths in the United States. Socioeconomic and Health Characteristics of California State Prisoners 19 be eight to nine times greater than in the U.S. population in general (Davis and Pacchiana, 2004). Parolees were estimated to represent 17 percent of the total U.S. population with AIDS and approximately 13 percent of the total U.S. population with HIV in 1996 (Hammett, Harmon, and Rhodes, 2002). Further, the burden of AIDS and HIV infection among state prison inmates appears to be concentrated within a few states. Recent BJS data show that New York, Florida, and Texas housed nearly half of all HIV-infected inmates in state prisons at yearend in 2000 (Maruschak, 2002).14 As expected, infectious diseases were common among inmates (Table 2.6). Approximately 11 percent of inmates reported having had a sexually transmitted disease, and 2 percent reported testing positive for HIV. Nearly one in 10 prisoners reported testing positive for TB, and 9 percent had hepatitis. The differences between inmates nationally and in California were mixed. California inmates were more likely to report tuberculosis (13 percent versus 10 percent) and hepatitis (13 percent versus 9 percent) but less likely to report STDs (9 percent versus 11 percent). Unlike other conditions, there were common differences across racial/ethnic groups. AfricanAmericans (9 percent), Latinos (11 percent), and other race/ethnicity (8 percent) were significantly less likely to report hepatitis than white inmates (20 percent). Tuberculosis, however, was more common among minorities, with statistically significant differences for Latinos (17 percent versus 9 percent among whites) and other race/ethnicity (25 percent versus 9 percent among whites). There were no statistically significant differences for STDs or HIV except for a statistically lower prevalence of STDs among other race/ethnicity. In terms of current infectious disease problems, Latino and African-American inmates were less likely than white inmates to report having hepatitis. Table 2.6 Infectious Diseases (percent) By Race/Ethnicity (California) Total U.S. Total California White AfricanAmerican Latino Other 9.13 12.95 19.82 8.66 11.48 8.29 11.14 8.77 7.98 13.49 6.63 1.56 TB 9.62 13.28 8.69 10.84 17.26 25.35 HIV 1.56 1.08 0.35 2.05 0.97 0.00 Hepatitis 4.96 7.37 12.54 3.42 6.91 3.14 STD 0.70 0.29 0.22 0.26 0.39 0.00 Type of Problem Lifetime health problems Hepatitis STD Current health problems Source: BJS 2004 inmate survey. Note: Sample includes male inmates incarcerated in state prisons only. Statistical significance is denoted by bold (California versus U.S.; race/ethnicity comparisons are African-American, Latino, and other versus white). 14 California was fourth, with 1,638 state prison inmates known to be HIV-positive at year-end in 2000. 20 Understanding the Health Implications of Prisoner Reentry in California, Phase I Report We did not assess the degree of comorbidity in terms of infectious diseases. However, it is worth nothing that one form of comorbidity involves the co-occurrence of HIV and hepatitis B or C. Hepatitis B and C can be acquired through exposure to contaminated blood during injection-drug use. Hepatitis C also can be sexually transmitted. As Shuter (2002, p. 189) notes, “High rates of hepatitis B and C in [intravenous drug users] and in the socioeconomically disadvantaged have resulted in a disproportionate burden of disease in prisoners.” He goes on to comment that one of the known risk factors for the transmission of HIV and hepatitis is the use of illicit intravenous drugs involving needle sharing. Various studies have suggested that prison inmates are at increased risk of contracting HIV disease and hepatitis B and C because of their use of illicit intravenous drugs as well as other high-risk behaviors (e.g., unprotected sex) among this population both prior to and during incarceration. Other Physical Health Conditions Prisoners were also asked whether they had experienced cancer, stroke, or paralysis (Table 2.7). Given the age distribution of this population, it is not surprising that these conditions were not very common among inmates. Nationally, 6 percent of inmates had experienced paralysis, 4 percent experienced a stroke, and 2 percent had had cancer. Rates were similar for California inmates and across all racial/ethnic groups. Current prevalence rates were about half of lifetime levels for each condition: paralysis (1 percent), stroke (3 percent), and cancer (1 percent). Again, there were no statistically significant differences for California and across racial/ethnic groups in California. The one exception was a significantly lower rate of strokes among Latinos (1 percent) relative to whites (5 percent). Table 2.7 Other Physical Health Conditions (percent) By Race/Ethnicity (California) Total U.S. Total California White AfricanAmerican Latino Other Cancer 1.80 2.00 3.21 2.10 1.24 0.00 Paralysis 6.12 7.70 9.64 8.17 5.95 6.80 Stroke 4.42 4.77 7.17 4.24 3.29 5.12 Cancer 0.76 1.17 1.90 0.78 1.04 0.00 Paralysis 1.44 2.10 3.12 2.08 1.38 1.68 Stroke 2.53 3.09 5.18 3.13 1.42 3.42 Type of Problem Lifetime health problems Current health problems Source: BJS 2004 inmate survey. Note: Sample includes male inmates incarcerated in state prisons only. Statistical significance is denoted by bold (California versus United States; race/ethnicity comparisons are African-American, Latino, and other versus white). Socioeconomic and Health Characteristics of California State Prisoners 21 Medical Treatment of Physical Health Needs While Incarcerated Access to care is an important issue, and inmates are constitutionally entitled to at least a basic level of care. However, in 2005, the California prison health care system was placed in federal receivership to address gross health care inadequacies and to prevent further unnecessary deaths (see California Prison Health Care Services, undated). Health issues that remain untreated during incarceration may be brought into the community and impose a financial burden on community resources. In addition, poor health can affect in a number of ways an individual’s ability to find employment or housing, reunite with family, or take other necessary steps to successfully reintegrate into the community. Many inmates have not had access to medical care prior to incarceration, which poses a challenge not only for the correctional health care system but also for communities’ health care safety nets upon their release (NCCHC, 2002). The BJS survey asked prisoners about whether they had received any medical care while in custody for their medical problems. Here, we summarize the findings for U.S. inmates overall and then for California inmates. The vast majority of inmates in state prisons reported receiving at least a medical exam during their incarceration (84 percent), although reported receipt of a medical exam was a significant 9 percentage points lower in California (75 percent) (Table 2.8). The lower rates in California are suggestive, but these shares do not control for need. Far fewer inmates reported having surgery (12 percent). Given the likely urgent nature of a condition requiring surgery, it is not surprising that we observe no significant differences based on geography and across demographic groups. Inmates were also asked about seeing a medical professional for each of the physical conditions they reported. Because these questions were asked only of those inmates who responded that they experienced a condition during incarceration, sample sizes are very small and statistical significance more difficult to identify, especially when making comparisons among racial/ ethnic groups. In some cases, we observe that all or none of the individuals in a group reporting a condition received care (i.e., 100 percent or 0 percent), presumably, in part, because of small sample sizes. In such cases, there is no standard error with which to identify statistically significant differences. Overall, the majority of inmates with a current medical condition reported seeing a medical professional for that particular condition during their incarceration nationally and in California, and hardly any statistically significant differences were identified. However, we caution that the results for utilization among inmates reporting a condition are much less reliable because the sample sizes are limited. 22 Understanding the Health Implications of Prisoner Reentry in California, Phase I Report Table 2.8 Medical Treatment/Utilization (percent) By Race/Ethnicity (California) Total U.S. Total California White AfricanAmerican Latino Other Medical exam 83.99 75.20 78.33 75.75 72.43 74.76 Had surgery 12.36 11.70 12.96 13.47 9.38 11.71 Type of Care All Inmates Since admission, saw a physician for a specific conditiona Cardiac 74.32 71.60 79.06 69.01 61.32 100.00 Kidney 70.22 66.46 100.00 71.74 27.24 100.00 Cancer 86.84 68.04 86.26 67.36 42.85 NA Asthma 76.55 72.00 81.98 70.94 59.73 100.00 Diabetes 94.12 89.52 100.00 89.45 85.72 67.17 Hypertension 89.60 87.70 82.24 91.62 82.10 100.00 Cirrhosis 87.08 83.66 86.16 100.00 100.00 49.82 Hepatitis 88.10 86.83 89.85 92.29 79.91 100.00 Paralysis 73.74 78.49 83.11 87.36 57.31 100.00 Stroke 63.41 53.69 50.87 58.02 41.36 100.00 STD 71.32 73.78 100.00 100.00 49.52 NA Source: BJS 2004 inmate survey. Note: Sample includes male inmates incarcerated in state prisons only. Statistical significance is denoted by bold (California versus United States; race/ethnicity comparisons are African-American, Latino, and other versus white). a Among those reporting current condition. Social and Behavioral Needs and Utilization The previous section examined physical health concerns and associated treatment. But inmates are also likely to suffer from social and behavioral conditions that complicate reentry. Selfreported information about the prevalence of drug abuse and dependence, as well as mental health problems, is summarized in this section.15 Drug Abuse and Dependence The use of alcohol and illicit substances plays a potentially important role in successful reentry. In its 1997 survey of federal and state inmates, BJS reported that a quarter of male and 15 The approach to identifying substance abuse and mental health disorders largely follows the BJS methods used in Mumola and Karberg (2007) and James and Glaze (2006), though, obviously, the sample (males only) differs. Socioeconomic and Health Characteristics of California State Prisoners 23 female state prison inmates fit the CAGE16 profile of alcohol dependence (Mumola, 1999, p. 8). Further, male inmates were more likely to have been drinking at the time of their offense (38 percent) or to report past binge drinking (42 percent) than female inmates (29 percent). Overall, in 1997, 83 percent of state prison inmates reported using drugs in the month prior to their offense, and 33 percent at the time of the offense (Mumola, 1999). The 2004 BJS inmate survey provides more updated information on the use of drugs and alcohol by inmates. Based on the responses to questions about behaviors relating to drug use, the majority of inmates qualified as dependent on or abusing a drug in the 12 months prior to incarceration (53 percent) (Table 2.9).17 Although not a focus of this section, we note that dependence and abuse of alcohol among the state inmate population is nearly as prevalent, at 44 percent (James and Glaze, 2006).18 Rates of drug abuse and dependence were greater among California inmates (58 percent versus 53 percent nationally). Rates of drug abuse/dependence were significantly lower among African-Americans (49 percent) and those reporting other race/ ethnicity (37 percent) relative to whites (67 percent). Given the extraordinary rates of dependence and abuse, it is not surprising that the majority of inmates (69 percent) reported that they had used drugs regularly (defined as having ever used a drug at least once a week for at least one month). Marijuana (60 percent) was the most commonly used drug, followed by cocaine/crack (29 percent), stimulants (17 percent), hallucinogens (13 percent), heroin/opiates (13 percent), depressants (10 percent), inhalants (5 percent), and other drugs (1 percent). California inmates were more likely to be regular drug users (73 percent). There were also significant differences in the types of drugs used. California inmates were approximately twice as likely to use stimulants (33 percent versus 17 percent nationally), with much of the stimulant use concentrated in methamphetamine (30 percent versus 14 percent nationally). Although the differences are not as large, heroin/ opiate use (16 percent versus 13 percent) and inhalant use (7 percent versus 5 percent) were also more common among California inmates. But California inmates were less likely to use depressants (7 percent versus 10 percent). The prevalence of regular drug use did vary across race/ethnicity. Latino inmates (68 percent) and inmates reporting other race/ethnicity (55 percent) were significantly less likely to be regular drug users than white inmates (81 percent). The use of specific substances also varied substantially by race/ethnicity. African-Americans were less likely than whites to use heroin/ opiates (6 percent versus 19 percent), depressants (3 percent versus 13 percent), stimulants (7 percent versus 56 percent), methamphetamine (7 percent versus 52 percent), and inhalants (2 percent versus 9 percent). Although their drug use was more similar to that of whites, Latino inmates were still significantly less likely than white inmates to use depressants (6 percent versus 13 percent), stimulants (35 percent versus 56 percent), and methamphetamine (31 percent versus 52 percent). Finally, inmates reporting other race/ethnicity were less likely than whites to report regular use of marijuana (42 percent versus 62 percent), depressants (2 percent 16 “The CAGE questionnaire is a diagnostic instrument for detecting a person’s history of alcohol abuse or dependence. CAGE is an acronym for the four questions used by the instrument [and assesses] attempts to (C)ut back on drinking, (A)nnoyance at others’ criticism of one’s drinking, feelings of (G)uilt about drinking, and needing a drink first thing in the morning as an (E)ye opener to steady the nerves” (Mumola, 1999, p. 5). 17 Responses to individual questions regarding elements of dependence and abuse for alcohol and for drugs are available in Appendix C. 18 James and Glaze (2006) examine the same sample of inmates but include the small share of inmates who are female. 24 Understanding the Health Implications of Prisoner Reentry in California, Phase I Report versus 13 percent), stimulants (28 percent versus 56 percent), and methamphetamine (27 percent versus 52 percent). Table 2.9 Drug Use, Abuse, and Dependence (percent) By Race/Ethnicity (California) Total U.S. Total California White AfricanAmerican Latino Other 53.16 57.83 67.20 49.41 59.51 36.57 Drug abuse 51.99 56.45 65.89 47.26 58.53 36.57 Drug dependence 35.30 43.51 54.85 34.65 44.22 20.57 69.00 72.64 80.78 72.80 68.25 55.24 Marijuana/hashish 59.80 57.01 61.77 60.94 52.02 42.10 Cocaine/crack 28.99 27.53 29.13 30.16 26.14 11.73 Heroin/opiates 12.60 15.65 18.76 6.12 21.03 10.85 9.66 7.01 13.20 2.91 5.96 1.71 17.26 32.74 55.96 7.37 34.61 28.24 14.25 30.08 51.73 6.84 31.43 26.67 13.45 15.60 17.94 14.53 14.43 17.16 Inhalants 4.55 6.66 9.44 2.35 8.21 3.35 Other Drugs 1.05 0.38 1.03 0.27 0.00 0.00 Lifetime 83.24 84.96 90.00 85.48 82.46 69.56 Regular use 69.00 72.64 80.78 72.80 68.25 55.24 Month prior to arrest 55.77 58.31 64.32 55.31 59.08 31.56 Type of Problem Drug abuse/dependencea Drug abuse/dependence Regular use (by type of drug)b Any drug Depressants Stimulants Methamphetamine Hallucinogens Timing of use (any drug) Source: BJS 2004 inmate survey. Note: Sample includes male inmates incarcerated in state prisons only. Statistical significance denoted by bold (California versus United States; race/ethnicity comparisons are African-American, Latino, and other versus white). Dependence is any behavior that meets at least three of the seven criteria during the same 12-month period: (1) tolerance, (2) withdrawal, (3) difficulty controlling use, (4) negative consequences, (5) significant time or emotional energy spent, (6) putting off or neglecting activities, and (7) desire to cut down (AddictionsandRecovery.org, 2009). Abuse is determined according to at least one of the following four criteria: (1) continued use despite social or interpersonal problems; (2) repeated use resulting in failure to fulfill obligations at work, school, or home; (3) repeated use resulting in physically hazardous situations; and (4) use resulting in legal problems (AddictionsandRecovery.org, 2009). a Drug abuse and dependence measures are constructed based on a series of questions regarding symptoms experienced in 12 months prior to incarceration. b Regular use is defined as having ever used at least once a week for at least one month. The results are based on questions regarding frequency of use. Socioeconomic and Health Characteristics of California State Prisoners 25 In this section, we discussed the prevalence of regular drug use among the inmate population. But drug use is dynamic. Individuals may experiment with an illicit substance but never become regular users. Likewise, regular users or even dependent users may not continue to use. The survey provides additional detail to examine the nature of the inmates’ drug use— specifically, whether the inmates had ever tried a substance, whether they were regular users, whether they had used in the month prior to arrest, and whether they had used at the time of the offense. These data indicate that a large majority of inmates had experimented with illicit drugs. Lifetime use exceeded regular use by about 10 to 15 percentage points across our analysis groups. However, not all regular users were using at the time of their arrest. Additional details on how drug use varies over time by specific substance are available in Appendix C. Treatment for Drug Use, Abuse, and Dependence Inmates who are using, dependent on, or abusing drugs may be treated in state prison prior to their release. Treatment prior to release can prepare the inmate for reentry and reduce the burden on the receiving community. For those inmates who were identified as abusing or dependent on a drug and for inmates who used in the month prior to incarceration, we examined whether they received any treatment during their current incarceration. While these are the best measures available to us, they are somewhat imperfect, because not everyone who met the criteria for abuse or dependence necessarily needed care during this incarceration. Some dependent inmates may have been treated prior to their current incarceration, while some of the inmates who used in the previous month may have been infrequent users and did not warrant treatment. Even if we were able to condition on the proper population of inmates who needed care during their incarceration, the data cannot tell us whether the inmates did not receive care because of a lack of availability or a refusal on their part to seek or participate in treatment. Approximately 39 percent of drug-abusing and drug-dependent inmates received some form of treatment during their current stay in prison (Table 2.10). The most common forms of treatment were the less resource-intensive self-help (28 percent) and educational programs (18 percent). More intensive treatments, such as residential programs (9 percent) and professional counseling (6 percent), were less common. Detox programs (1 percent) and drug maintenance (0 percent) were extremely rare in prison. Again, we caution that we cannot distinguish whether the fact that few inmates received care was because of a lack of availability or lack of motivation, although it is likely that both played some role. One of the most interesting findings was that California inmates were only about half as likely to receive any treatment (22 percent) relative to their counterparts nationally. This result held for each type of treatment considered. Interestingly, all California inmates experienced low utilization of treatment: There were no significant differences across racial and ethnic groups. A second approach, which conditions on inmates using in the month prior to admission to prison, yielded generally similar findings.19 Although both sets of measures about treatment received in prison are imperfect (as described earlier), the findings raise concerns about whether more could be done to prepare these inmates for reentry, particularly in California. 19 The only significant difference was lower utilization of self-help among Latino inmates. 26 Understanding the Health Implications of Prisoner Reentry in California, Phase I Report Table 2.10 Drug Treatment (percent) By Race/Ethnicity (California) Type of Treatment Total U.S. Total California White AfricanAmerican Latino Other Treatment (conditional on drug abuse/dependence) Any drug treatment or program since admission to prison 39.22 21.64 24.87 23.39 17.59 23.18 14.18 6.55 7.31 8.44 4.84 4.60 Residential facility or unit 9.09 4.47 4.99 4.22 4.17 4.60 Counseling by a professional 6.32 2.40 2.70 4.19 0.84 4.60 Detoxification unit 0.73 0.53 0.78 1.07 0.00 0.00 Maintenance drug 0.24 0.13 0.40 0.00 0.00 0.00 33.97 18.77 21.77 18.64 15.91 23.18 Self-help group/ peer counseling 27.68 16.31 19.45 16.55 12.93 23.18 Education program 17.77 6.87 7.78 7.89 5.59 4.60 Drug treatment Other programs Treatment (conditional on drug use in month before arrest) Any drug treatment or program since admission to prison 37.91 21.80 25.97 23.09 17.72 16.12 13.44 7.14 8.07 8.94 5.18 5.33 Residential facility or unit 8.76 5.08 5.25 5.18 4.85 5.33 Counseling by a professional 5.72 2.38 3.22 3.75 0.51 5.33 Detoxification unit 0.85 0.52 0.81 0.96 0.00 0.00 Maintenance drug 0.23 0.13 0.41 0.00 0.00 0.00 32.70 18.45 22.34 18.38 15.38 16.12 Self-help group/ peer counseling 26.51 15.73 20.73 16.04 11.29 16.12 Education program 16.88 6.97 6.96 7.50 6.69 5.33 Drug treatment Other programs Source: BJS 2004 inmate survey. Note: Sample includes male inmates incarcerated in state prisons only. Statistical significance is denoted by bold (California versus United States; race/ethnicity comparisons are African-American, Latino, and other versus white). Socioeconomic and Health Characteristics of California State Prisoners 27 Mental Health Conditions The NCCHC report to Congress (2002) provided national estimates for the prevalence of six psychiatric disorders among prison inmates. According to the NCCHC, among state prison inmates, the prevalence of schizophrenia and other psychotic disorders and bipolar disorder is more common among inmates than it is in the overall U.S. population. The prevalence of schizophrenia or other psychotic disorders is between three and five times greater in the inmate population than it is in the total U.S. population. The prevalence of major depression is similar to the general U.S. population; among state prison inmates, major depression is estimated to be between 13.1 and 18.6 percent, compared to 18.1 percent for the total U.S. population. The prevalence of bipolar disorder is between 2.1 and 4.3 percent, nearly three times greater than it is in the U.S. population as a whole. Dysthymia, a less severe form of depression, is also more prevalent among the state prison population than it is among the U.S. population as a whole. The prevalence estimates cited here are not directly comparable to the self-reports of history or symptoms of mental illness in the 2004 BJS survey of prison inmates. However, this summary underscores that mental illness in general is more prevalent in the prison population than in the general population and poses a significant health burden and that it is important to address the mental health treatment needs of this population. Next, we summarize the survey findings with respect to self-reported history of mental illness and treatment. Recent History or Symptoms of Mental Illness Following the previous analysis, we consider diagnoses, symptoms, and history of treatment in creating our measures of prevalence of mental illness (see James and Glaze, 2006). A small majority (55 percent) of inmates reported a recent history or symptoms of mental illness (Table 2.11). There were no differences between inmates nationally and in California. But within California, white inmates presented the greatest prevalence of a history or symptoms of mental illness (62 percent). The lowest incidence of mental health problems was reported by Latino inmates (45 percent), mostly due to a lower prevalence of recent history of mental problems relative to whites (5 percent versus 11 percent). It is unclear, however, to what extent the differences are due to a lower incidence of symptoms, poor access to care, or a lower likelihood to seek care (and therefore a lower likelihood of being diagnosed if a mental health problem exists). Previous studies have found that Latinos tend to underutilize mental health services, are less likely to receive evidence-based mental health care, and rely more often on primary care rather than specialty care for mental health issues (Cabassa, Zayas, and Hansen, 2006). The reasons for this are not completely clear, although three major hypotheses have been proposed: (1) lower frequency and severity of mental illness, (2) use of alternative treatment sources (e.g., folk healers), and (3) access barriers (e.g., geographic inaccessibility, language, class-bound and culture-bound values of most therapists) (Terviño and Rendón, 1994). Focusing only on recent symptoms, the picture is very similar. Nearly half (49 percent) of inmates presented recent symptoms of major depression (23 percent), mania (43 percent), or psychosis (15 percent). Conditional on showing symptoms, most inmates reported multiple symptoms. Those with major depression symptoms typically reported more than six symptoms of depression, those with mania symptoms reported an average of nearly three symptoms, and those exhibiting symptoms of psychosis generally reported 1.3 symptoms. The results for California inmates were comparable, but we do find differences across race/ethnicity in California. Consistent with our previous findings, Latino inmates were less likely to exhibit recent 28 Understanding the Health Implications of Prisoner Reentry in California, Phase I Report Table 2.11 Recent History of Symptoms of Mental Illness By Race/Ethnicity (California) Recent History of Mental Illness Total U.S. Total California White AfricanAmerican Latino Other 55.24 54.84 61.55 60.90 45.17 53.61 16.21 17.76 21.30 22.76 11.68 13.72 6.44 6.76 10.55 5.05 4.69 10.33 32.40 30.22 29.70 32.91 28.68 29.55 48.69 48.01 50.83 55.76 40.48 43.27 22.49 20.30 22.54 23.41 15.83 23.91 Number of symptoms, if any (mean) 6.09 6.19 6.14 6.26 6.19 6.15 Mania disorder (%) 42.57 42.45 46.27 48.82 35.56 34.57 Number of symptoms, if any (mean) 2.89 2.84 2.82 2.91 2.78 2.89 14.96 16.12 13.20 23.62 13.43 10.01 1.28 1.35 1.32 1.37 1.34 1.51 22.62 24.30 31.77 27.68 16.08 23.66 Told had a disorder by mental health professional (%) 8.47 11.51 14.18 15.24 6.99 8.47 Had overnight hospital stay (%) 5.15 4.95 6.26 6.79 2.54 5.09 Used prescribed medications (%) 16.42 18.37 25.47 20.65 11.56 15.26 Had professional mental health therapy (%) 13.89 13.48 16.63 16.91 8.67 11.84 Recent history or symptoms Recent history or symptoms (%) Recent history and symptoms (%) Recent history only (%) Recent symptoms only (%) Recent symptoms Recent symptoms (%) Major depressive disorder (%) Psychotic disorder (%) Number of symptoms, if any (mean) Recent history Recent history (%) Source: BJS 2004 inmate survey. Note: Sample includes male inmates incarcerated in state prisons only. Statistical significance is denoted by bold (California versus United States; race/ethnicity comparisons are African-American, Latino, and other versus white). Socioeconomic and Health Characteristics of California State Prisoners 29 symptoms (40 percent) than whites (51 percent). The difference is mainly the result of a lower prevalence of mania symptoms (36 percent versus 46 percent). African-Americans, however, were more likely to exhibit symptoms of a psychotic disorder (24 percent versus 13 percent). Further details on the nature of these symptoms are available in Appendix C. We characterized inmates as having a recent history of mental health problems if they had been told that they had a disorder by a professional, had an overnight stay in the hospital, used prescription medications for a mental health issue, or had therapy with a mental health professional. About one in four inmates (23 percent) had a recent history of mental health problems. The most common reason was the use of prescription medications (16 percent), followed by mental health therapy (14 percent), a diagnosis by a mental health professional (8 percent), or an overnight stay (5 percent). There were no significant differences for California inmates and few differences across race/ethnicity. Only Latinos reported statistically significantly lower rates. Only 16 percent of Latino inmates reported a recent history of mental illness, compared to 32 percent of whites. Latinos were half as likely to report a recent diagnosis (7 percent versus 14 percent), use of prescription medications (12 percent versus 25 percent), and utilization of mental health therapy (9 percent versus 17 percent).20 Details on the exact nature of the issues presented by inmates are available in Appendix C. Lifetime Prevalence of Mental Illness Although only 9 percent of inmates reported a recent diagnosis of major depression, mania, or psychosis, nearly a fourth of inmates (24 percent) reported being diagnosed with a mental health disorder during their lifetime (Table 2.12). In this case, inmates were asked whether they had ever been diagnosed with depression (18 percent), mania (9 percent), post-traumatic stress disorder (PTSD) (5 percent), schizophrenia (4 percent), anxiety (6 percent), and personality (6 percent) or other disorder (2 percent). Those inmates who had received a diagnosis, on average, had been diagnosed with multiple disorders (2.06 disorders). California inmates had similar rates of lifetime mental health disorders as their national counterparts. Within California, Latino inmates had a lower incidence of mental health diagnoses (16 percent) relative to whites (36 percent). The lower incidence is the result of significantly lower reporting of all disorders. Inmates reporting other race/ethnicity also had a lower incidence of lifetime diagnoses (20 percent versus 36 percent for whites), but the disaggregated results were significant only for mania (5 percent versus 15 percent). Details on the exact nature of the symptoms presented by inmates are available in Appendix C. 20 Latino inmates were also only half as likely to report a hospital stay (3 percent versus 6 percent), but the difference was not statistically significant. 30 Understanding the Health Implications of Prisoner Reentry in California, Phase I Report Table 2.12 Lifetime Prevalence of Mental Illness By Race/Ethnicity (California) Total U.S. Total California White AfricanAmerican Latino Other Ever diagnosed with mental health disorder (%) 24.19 26.08 36.21 29.88 16.08 20.34 Depression (%) 17.73 18.81 24.29 22.42 12.29 15.20 Mania (%) 8.66 9.94 15.45 10.57 5.79 5.15 PTSD (%) 5.08 6.01 9.31 7.86 2.37 3.45 Schizophrenia (%) 4.46 6.30 7.83 9.77 2.83 3.44 Anxiety (%) 6.34 8.28 11.23 9.88 5.18 5.03 Personality (%) 5.74 6.98 10.92 8.33 2.77 8.44 Other disorder (%) 1.90 2.22 3.77 2.64 0.98 0.00 Number of diagnoses, if any (mean) 2.06 2.24 2.28 2.39 2.00 1.99 Diagnosis Source: BJS 2004 inmate survey. Note: Sample includes male inmates incarcerated in state prisons only. Statistical significance is denoted by bold (California versus United States; race/ethnicity comparisons are African-American, Latino, and other versus white). Treatment for Mental Illness As with substance abuse, it is important to identify the share of inmates who have been treated for their mental health problems. Having received treatment may indicate that the mental health issue has been addressed, but one might also consider that more severe or chronic problems may require continued monitoring and care after release.21 Unfortunately, we cannot precisely identify those inmates who needed treatment during their incarceration. Therefore, we present the findings in two ways. First, we show the share of inmates with a recent history of mental health problems who received care. For comparison, we look at the share of all inmates who received care in Appendix C. The timing of the treatment received is also shown in three ways: (1) ever received care, (2) in the year prior to admission or since admission, and (3) since admission. Among those with a recent history of mental illness (see Table 2.13), nearly half (48 percent) received some form of care during their lifetime. The most common mode was prescription medication (37 percent), professional therapy (34 percent), and inpatient stays (19 percent), while 6 percent received some other form of care. If we confine our examination to care received recently (defined as the year prior to admission to prison, at admission, or during incarceration), the rates are lower (38 percent). The rates of treatment are lower still when we look at treatment received during the current incarceration. Less than one-third (32 percent) received some care. There is also evidence that the mode of treatment changes. Inpatient stays, 21 For example, one might consider that those who required an overnight stay for their illness might represent more severe cases than those requiring only medication. However, we do not know whether everyone who needed an overnight stay received one. Socioeconomic and Health Characteristics of California State Prisoners 31 in particular decline dramatically when we focus on treatment received recently or during incarceration. Contrary to our findings for drug abuse, there is no evidence that California inmates are less likely to receive care in general or any particular form of care. However, Latino inmates were significantly less likely to receive care during their lifetime (43 percent versus 61 percent Table 2.13 Mental Health Treatment Among Those with a Mental Health Problem (percent) By Race/Ethnicity (California) Total U.S. Total California White AfricanAmerican Latino Other Ever received mental health treatment 47.76 51.41 60.65 51.14 43.21 41.69 Had overnight hospital stay 19.06 17.26 20.99 18.71 12.00 16.02 Used prescribed medications 37.39 41.03 51.37 39.54 32.80 32.10 Had professional mental health therapy 33.56 33.63 40.53 34.83 25.76 28.86 5.86 7.10 8.00 9.24 4.07 6.38 38.46 41.32 48.03 43.54 32.77 35.30 9.42 9.14 10.20 11.34 5.72 9.66 Used prescribed medications 30.02 33.93 41.49 34.38 26.12 28.95 Had professional mental health therapy 25.40 24.90 27.09 28.20 19.54 22.46 3.74 4.41 4.17 6.57 2.21 6.38 32.40 35.27 38.35 39.07 29.44 25.64 5.52 5.59 5.86 6.82 4.39 3.19 Used prescribed medications 25.25 29.40 33.49 31.24 24.57 19.29 Had professional mental health therapy 21.35 20.31 22.11 22.82 16.89 12.81 3.12 3.71 2.90 6.12 2.21 3.19 Treatment Used other mental health treatment Received treatment during year before arrest or since admission Had overnight hospital stay Used other mental health treatment Received treatment after admission Had overnight hospital stay Used other mental health treatment Source: BJS 2004 inmate survey. Note: Sample includes male inmates incarcerated in state prisons only. Statistical significance is denoted by bold (California versus United States; race/ethnicity comparisons are African-American, Latino, and other versus white). 32 Understanding the Health Implications of Prisoner Reentry in California, Phase I Report for whites), mostly because of a lower use of prescription drugs and professional therapy. This overall finding, however, does not hold for care received during incarceration. The results are qualitatively similar for care received by all inmates regardless of mental health status (not shown here, see Table D.4, Appendix D). The disparity for Latinos is stronger, but is likely driven in part by a lower prevalence of reported mental illness. Comorbidity/Dual Diagnoses The co-occurrence of substance-use disorders and other mental disorders is common and has been studied extensively. For example, the Epidemiologic Catchment Area (ECA) study found that, among those with an alcohol disorder, 37 percent had a comorbid mental disorder (Regier et al., 1990). In more recent years, the National Epidemiologic Survey on Alcohol and Related Conditions (NESARC) survey estimated that 22 percent of individuals with a mood disorder and 19 percent of individuals with an anxiety disorder had a co-occurring drug-use disorder (Brady, Verduin, and Tolliver, 2007). The prevalence estimates presented by NCCHC for mental illness did not take into account co-occurring mental illnesses or co-occurring substance abuse and dependence disorders, yet we know that they are common. For example, BJS estimates in 1997 that approximately 60 percent of mentally ill inmates in state prisons were under the influence of alcohol or drugs at the time of their current offense (Mumola, 1999). Kokkevi and Stefanis (1995) reported that anxiety disorders were found in 32 percent and affective disorders in 25 percent of opiate-dependent male prisoners. In their analysis of the BJS data, James and Glaze (2006) found that there is significant overlap between the state inmate populations that suffer substance abuse/dependence and mental health problems. According to that report, more than two-fifths of inmates (42 percent) demonstrated both mental health issues and substance abuse/dependence (including alcohol). This comorbidity has implications for how to deliver care most effectively to these individuals. Discussion Our analyses of parolees’ socioeconomic characteristics and of the BJS inmate survey tell us a number of things about the health care needs of men returning from prison in California. First, male prisoners are relatively young and have low educational attainment. Although the BJS survey does not have information about literacy, it is well known that 50 to 75 percent of inmates are functionally illiterate, a rate three times that of the general population (Newman, 1993). These low levels educational attainment and literacy are likely to negatively affect their employment opportunities post-release and, concomitantly, their health and health care access. Half of California inmates had minor children, and approximately 21 percent of California inmates (or 41 percent of those who reported minor children at the time of the interview) had been living with their children at the time of their latest arrest. The imprisonment of parents disrupts parent-child relationships, alters the networks of familial support, and places new burdens on governmental services, such as schools, foster care, adoption agencies, and youthserving organizations (Travis, McBride, and Solomon, 2005). Socioeconomic and Health Characteristics of California State Prisoners 33 When it comes to physical health conditions, prisoners bear a high burden of chronic diseases, such as hypertension and asthma, and infectious diseases, such as hepatitis and tuberculosis—conditions that require regular use of health care to manage effectively. Unfortunately, the likelihood of these men receiving adequate health care once released from prison is probably low, given their high rates of uninsurance and other barriers to care. A final area concerns mental health care and drug treatment. Prisoners’ needs in these areas are even more pronounced than their physical health needs, with more than half of California inmates reporting recent mental health problems and more than half reporting drug abuse or dependence. Yet, among California inmates reporting abuse or dependence, the share receiving treatment since admission to prison is lower than among all U.S. prisoners. California prisons appear to be doing a little better when it comes to mental health, with about half of prisoners receiving treatment in prison (among those reporting mental health problems), the same as the U.S. prison population. Our measures are admittedly imperfect (as discussed earlier), but if prisons fail to reach a substantial share of those in need, then the care provided still falls short. Given the high prevalence of mental health problems and substance abuse in the prison population, finding services to meet these needs once inmates are released is likely to be critical. This summary provides a rich picture of the health care needs of California inmates and serves as a useful proxy for understanding the reentry population’s needs. These figures are based on self-reported data and so they should be taken as rough estimates. From previous research on the general population, we know that there likely is some underreporting in areas such as mental health, substance abuse, or infectious diseases, and that such underreporting will vary by race or ethnicity. Because we do not expect that inmates are less likely to underreport, we should consider that the prevalence rates presented here may be lower-bound estimates. Chapter Three Distribution and Concentration of Parolees in California In Chapter Two, we discussed the sociodemographic and health characteristics of California state prisoners as a way to help us gain an understanding of parolees’ socioeconomic characteristics, health care needs, and access to care. We now turn to analyzing the distribution and concentration of parolees in California, as well as the overall characteristics of parolees and the communities to which they are disproportionately returning. In particular, we discuss the results of our analysis identifying the counties in California with the greatest concentration of parolees based on the census tract of residence. We then provide an in-depth examination for four counties in the state: Los Angeles, Alameda, Kern, and San Diego. We focus specifically on these counties because they represent a significant share of the parolees, and we wanted to achieve a balance between northern, central, and southern California, as well as include both urban and rural counties in our comparisons. Then, we discuss the results of our analysis of the demographics of California parolees (e.g., race/ethnicity) and their term served (e.g., type of offense, number of prior offenses), providing descriptive information about the socioeconomic characteristics of areas where parolees cluster by race and ethnicity, poverty, unemployment, educational attainment, and degree of linguistic isolation of households. Analysis of Concentration and Distribution of Parolees in California In this section, we present the results of our analysis of the concentration and distribution of parolees in California, starting with a discussion of the methods underlying that analysis. Methods In this section, we describe the methods we used in mapping the location of parolees—along with some of the data concerns we encountered in the process and how we assessed their impacts—and in conducting the cluster analysis to identify “clusters,” or concentrations, of parolees. Mapping the Location of Parolees. We obtained data from the CDCR on California parolees released in 2005–2006.1 Although our original request was for the exact street address of each parolee, the CDCR’s Office of Research elected—for confidentiality reasons—to pro1 Focusing on parolees captured the majority of individuals released from prison. We did not include in our sample those individuals who were unconditionally released from prison. In a typical year, approximately 97 percent of individuals released from California’s prisons are parolees; only 3 percent are released unconditionally, having served their entire sentence. 35 36 Understanding the Health Implications of Prisoner Reentry in California, Phase I Report vide only information on parolees’ census tract location, zip code, and Thomas Brothers Guide map cell and to omit street address from the file. For our analysis, we focused on the census tract location for the geographic mapping of the distribution of parolees using the populationweighted centroid of each tract as the residential location.2 The census tract data were better suited for our purposes than the other variables provided by the CDCR because census tracts are potentially small in urban areas, allowing us to capture any variation that exists and map the results to other data that we would be using to characterize the safety net and underlying demand for services in each of the counties. However, without the actual residence address of returning CDCR inmates, we could not validate their census tract geocoding or compute other aggregations of the data for cluster analysis or individual accessibility. To create the parolee analytic file, we first combined data from the two files provided by the CDCR. The first file (TERMGRUP) is essentially a log of all the times an individual enters or leaves prison for any reason. Using this file, we determined that 248,833 paroles occurred in 2005–2006. Some individuals were paroled more than once during this period, so we selected only their first 2005–2006 parole, giving us 176,618 parolees. The second file (TERMCASE) includes only records for new court commitments and returns to prison with a new sentence. Revocations of parole with no additional sentence, for example, would be included in the first file but not in the second file. TERMCASE includes information about the initial sentence, prior record, and demographic characteristics. By appending this information to that in the TERMGRUP file, we were able to analyze the demographic and other characteristics of the 2005–2006 parolees. We then utilized data from a log of addresses recorded by parole officers on all the places that parolees resided in 2005 and 2006 after being released from prison. From this file, we chose the earliest record in 2005–2006 that included a valid census tract number and appended it to the file created by merging the TERMGRUP and TERMCASE files. Of the total 176,618 cases in our file, 22 percent (n = 39,313) of cases did not have a valid Federal Information Processing Standard (FIPS) code. The FIPS county code is a five-digit code that uniquely identifies counties in the United States, with the first two digits representing the FIPS state code and the last three digits representing the county code within a state. In addition, we used the six-digit FIPS census tract code and the concatenated FIPS codes to identify parolee locations by state, county, and census tract. In this way, we were able to find census tract numbers for 137,305 parolees. To describe the characteristics of parolees (part of the analysis described in the second half of this chapter), we used the entire sample of 176,618 parolees released in 2005 and 2006; however, all our maps are necessarily based on the subset of parolees (n = 137,305) for whom we had valid census tract data. We note that there were a couple of issues that could have an impact on the analyses we conducted on mapping parolees’ locations and identifying clusters. As such, we conducted some analyses to determine what impact such issues might have. We discuss these next in more detail. As noted, out of necessity, all the maps in this chapter exclude the 22 percent of 2005– 2006 parolees for whom we did not have valid census tract data. We compared the distribution and characteristics of the parolees who were included in the final analysis file with those who were excluded. Our analysis did not reveal any systematic differences across the two groups in 2 We chose not to use the Thomas Brothers map cell variable because 40 percent of the sample was missing this information. Distribution and Concentration of Parolees in California 37 terms of distribution by county or by race/ethnicity, which provides some evidence that the missing data would not affect the distribution patterns identified in our analysis. A potential source of error in calculating the geographic analysis results from the method used by the CDCR to assign census tract numbers. In creating the 11-digit FIPS code, the CDCR used the zip code as a control. For those cases that were missing a zip code, the CDCR took the first random location of the street address that came up in its search and assigned a FIPS code to it. We know that this introduced some error into the assignment of tract location, particularly in cases in which the street name was a commonly used one (e.g., Main Street). Specifically, we found cases in which the cities were not consistent with the FIPS code. When we analyzed the FIPS codes, we found, for example, that the San Francisco FIPS code included many cities in Southern California. Without the parolees’ street addresses, we were unable to assess whether the FIPS code was incorrectly assigned or whether the city name was incorrectly entered. We conducted an analysis, the details of which are described in Appendix E, and found extensive overlap between the clusters identified using cases with and without zip codes, regardless of cluster size used. Thus, we are confident that by including the cases with missing zip codes in our analyses, the overall pattern of clustering is not substantively biased by any potential errors in assignment of census tract information to those cases without zip codes. Finally, our analysis of the concentration of parolees by county and within counties is based on the first valid geocoded census tract information for an individual, which accurately assesses where parolees first go upon release from prison.3 However, some researchers have observed high rates of residential mobility and homelessness among parolees, with some individuals spending time at multiple locations while providing parole officers the address of stable relatives or friends (Cahill and LaVigne, 2009). Although assessing mobility among the California parolees was beyond the scope of this project, we were interested in understanding the impact of such residential mobility on our analyses. To assess the likelihood that parolees were still residing in the census tract we used for mapping purposes, we computed the percentage of cases in which the parolee had multiple missing or different location information that was obtained after the initial census tract location we used for our maps. Approximately 48 percent of parolees were possibly residing in a different census tract than the one used in our maps and analyses. So, there is about a 50-50 chance that our maps show the actual residence locations of parolees for the entire period of our analysis. While this may introduce some error into our mapping, an analysis of parolees in San Diego County found that, although 52 percent of parolees moved, their first move was, on average, over four miles and subsequent moves were a lesser distance, suggesting that they tend to stay in the same general neighborhoods or communities to which they first return upon release from prison. In addition, Cahill and LaVigne (2009) found that parolees originally return to areas that are far more disadvantaged than the average neighborhood in San Diego County, and when they do move, they tend to go to similar areas or areas that are worse off. This suggests that the pattern that we saw of parolees going to disadvantaged neighborhoods upon release and the implications for the safety net in these localities remains robust. 3 This may be the best time to get parolees to access health care and other needed services. Further, we know that this initial period is important for providing services to this group, as evidenced by a retrospective cohort study of prisoners that found that parolees are at increased risk of mortality upon release from prison, especially during the first several weeks (Binswanger et al., 2007). The leading causes of death were drug overdose, cardiovascular disease, homicide, and suicide. 38 Understanding the Health Implications of Prisoner Reentry in California, Phase I Report Cluster Analysis. In addition to mapping the location of parolees in the state by county and within counties by census tract, we also wanted to identify clusters, or concentrations, of parolees. To do so, we used the 2005–2006 parolee data to assign parolees to the populationweighted centroids of their census tracts. To determine the population-weighted centroid location of each tract, we used the geometric center point of each census block unit (equivalent to street blocks) nested within a census tract. On average, census tracts in California have eight blocks per tract. We then used the 2000 population and geometric center point coordinates of each census block to compute the weighted mean center location for the tract. Our intent in using the population-weighted census tract centroid (instead of the geometric center point) was to account for fact that the population is not uniformly distributed within a census tract. Since we did not have data on exact addresses of residence for each parolee, we wanted to assign the parolees residing in various census tracts to those areas that were at least near the highestpopulated areas of the different census tracts. Using the weighted mean census tract centroids, we then geocoded or matched the CDCR parolee data to them. This allowed us to represent parolee residence locations in the population-weighted mean census tract centroids as points in our maps for cluster and accessibility analyses.4 We then used a nearest neighbor hierarchical (NNH) cluster routine to group parolees into clusters based on spatial proximity. Specifically, we used the cluster analysis tools in the freely available and widely used CrimeStat® III software to create the NNH clusters. In conducting the NNH analysis, we set the search radius at 50 percent and minimum cluster size at 30 (except for Kern County, where the minimum cluster size was set at 20) with one standard deviation.5 That is, we represented clusters on maps using one-standard deviation ellipses of the population-weighted census tract center points. The decision to use the 50-percent search radius and minimum size criteria of at least 20 parolees was based on a sensitivity analysis of cluster results for a limited set of possible criteria. The NNH cluster method identifies clusters regardless of which criteria are chosen. Thus, our aim in using the NNH method was to clearly delineate subcounty areas that included the largest proportion of the parolee data. For instance, by increasing the search radius and/or increasing the minimum number criteria, the size of the clusters, in some instances, was larger than a single county when analysis was conducted over the entire state. Decreasing the size of the search radius and/or the minimum number created subcounty clusters but accounted for less than 50 percent of all parolees in the data file obtained from the CDCR for the two-year period. Thus, our decision represents a compromise based on our subjective requirements for how to delineate cluster areas to draw attention to the mapping of spatial patterns or “hotspots” in the data. The 50-percent search radius used by the CrimeStat package is based on a mean random distance, given the number of “events” or cases occurring divided by the total area. The 50-percent probability standard is used to set the threshold distance that defines the “nearest neighbor”: If the cases are distributed at random, then for all distances between pairs of points, fewer than 50 percent of the pairs will be shorter than the threshold distance. By increasing the percent search radius criteria, we were, in effect, increasing the distance and likelihood that pairs of points would have 4 Again, this was necessary because the CDCR did not provide us with the actual street addresses of the individuals in our sample. 5 For Alameda, Los Angeles, and San Diego counties, the minimum cluster size was set at 30. Because Kern County is less densely populated, we set the minimum cluster size at 20. Distribution and Concentration of Parolees in California 39 distances below the threshold distance for forming a cluster—and vice versa for decreasing the percent search radius. To name each of the clusters that we identified with the NNH routine, we used the nearest zip code for a given cluster. We then overlaid this information with the raw count of parolees by tract to get a better idea of the locations with the highest density of parolees in each zip code area to resolve ambiguous assignments or where more than one cluster was closest to the same zip code area. This step was used to assign names to the clusters across California. To name the clusters at the county level, we conducted an NNH analysis for each county and used a two-step process to name the clusters: (1) if the cluster was mainly in one zip code area in a county, the cluster was given that zip code name; and (2) for clusters covering multiple zip code areas in a county, we used the parolee density layer in the census tracts to name the cluster (i.e., if a cluster stretched across multiple zip code names and had a relatively equal density of parolees, we used both zip code names, e.g., the Arvin/Lamont cluster in Kern County). We used a somewhat different approach in Los Angeles County for the Los Angeles zip code area only. We had a number of clusters in the Los Angeles zip code area, and so we elected to use the urban neighborhood area name to get a finer naming scheme than the zip code could provide.6 Specifically, a large segment of the City of Los Angeles falls within a single zip code area; however, there are several officially recognized urban neighborhoods within that zip code area, which would allow better precision in describing the location of the clusters in this zip code area (e.g., South Los Angeles, Southeast Los Angeles). Results of Analysis of Concentration and Distribution of Parolees in California Using the methods just described, we conducted an analysis of the concentration and distribution of parolees in California, first in the state as a whole and then in the four selected counties. Results of State Analysis. Figure 3.1 shows the clusters of parolees in California. The clusters are shown as yellow ellipses; the minimum cluster size means that the minimum number of parolees in each cluster is 30. The black dots represent the count of parolees. The blue shading shows the concentration of parolees statewide. Parolee rates are the number of parolees per 1,000 of the total parolee population. Examining the distribution of parolees shows that they are concentrated in 11 counties around the Bay Area and in the southern part of the state. By far, the highest rate of returnees is in southern counties, especially Los Angeles, Orange, San Bernardino, Riverside, and San Diego counties. Looking within counties on the map, we find that most of the clusters fall into urban areas, for example, near San Francisco–Oakland, Los Angeles, and San Diego. Given that the amount of concentration varies across urban and rural counties, a different strategy will be needed to provide services to parolees located in more rural counties. Based on the clusters identified in Figure 3.1, we selected those that contributed most to the total number of parolees. Table 3.1 shows the clusters that are responsible for nearly half of the parolees in the state. The clusters are named based on the nearest city, as described in the methodology. The exception is Los Angeles County, where we used neighborhood names to designate cluster areas within the City of Los Angeles. Importantly, in the counties to which 6 This enabled us to distinguish, for example, between the South Los Angeles and Southeast Los Angeles clusters. 40 Understanding the Health Implications of Prisoner Reentry in California, Phase I Report the majority of parolees return, the NNH cluster analysis shows that parolees are concentrated in certain areas within a county. That is, small areas in these counties have disproportionately higher concentrations of parolees. Figure 3.1 Relative Concentrations of Parolees in California, by County Source: CDCR parolee data, 2005–2006. Distribution and Concentration of Parolees in California Table 3.1 Statewide Clusters Responsible for Nearly Half of Parolees in California Number Proportion by Total State Parolees Cumulative Percentage South Los Angeles 7,908 4.5 4.5 Long Beach 5,131 2.9 7.4 San Bernardino 3,772 2.1 9.5 San Diego/National City 3,611 2.0 11.6 Fresno 3,528 2.0 13.6 Bakersfield 3,364 1.9 15.5 Sacramento 3,140 1.8 17.2 San Jose 2,986 1.7 18.9 Metro/North Los Angeles 2,599 1.5 20.4 Riverside 2,514 1.4 21.8 Stockton 2,317 1.3 23.1 Van Nuys 2,272 1.3 24.4 Oakland 2,269 1.3 25.7 San Francisco 1,556 0.9 26.6 Ontario 1,436 0.8 27.4 Lancaster/Palmdale 1,311 0.7 28.1 El Monte 1,195 0.7 28.8 Victorville 1,195 0.7 29.5 Pomona/Covina 1,161 0.7 30.2 Modesto 1,158 0.7 30.8 Redding 1,143 0.6 31.5 Yuba City 949 0.5 32.0 Oceanside/Vista 913 0.5 32.5 Venice 904 0.5 33.0 Visalia 846 0.5 33.5 Oxnard 829 0.5 34.0 Redlands 757 0.4 34.4 Eureka 745 0.4 34.8 Hemet 717 0.4 35.2 Vallejo 710 0.4 35.6 Torrance/Wilmington 671 0.4 36.0 Fairfield 640 0.4 36.4 Cluster 41 42 Understanding the Health Implications of Prisoner Reentry in California, Phase I Report Table 3.1—Continued Number Proportion by Total State Parolees Cumulative Percentage La Puente 627 0.4 36.7 Salinas 609 0.3 37.1 Mission Valley/Old Town 603 0.3 37.4 Fremont/Menlo Park 589 0.3 37.8 Merced 575 0.3 38.1 Madera 552 0.3 38.4 Hanford 551 0.3 38.7 El Cajon 522 0.3 39.0 Indio 504 0.3 39.3 Concord 471 0.3 39.5 Hayward 466 0.3 39.8 South Sacramento 453 0.3 40.1 Porterville 431 0.2 40.3 Woodland 425 0.2 40.6 Sanger/Selma 411 0.2 40.8 Oroville 398 0.2 41.0 Chico 385 0.2 41.2 Santa Rosa 383 0.2 41.4 Santa Maria 364 0.2 41.7 Pasadena 320 0.2 41.8 Escondido 308 0.2 42.0 Yucca Valley 274 0.2 42.2 Roseville 248 0.1 42.3 Richmond 245 0.1 42.4 Desert Hot Springs 241 0.1 42.6 Murrieta 240 0.1 42.7 Gilroy 206 0.1 42.8 Delano 168 0.1 42.9 Turlock 121 0.1 43.0 Cluster Distribution and Concentration of Parolees in California 43 Results of Analysis of Four Selected Counties. Given that our analysis of the distribution of parolees across California showed uneven distribution within counties, we now focus on understanding clustering within the four counties that are the focus of this study. We again used NNH cluster analysis, this time at the county level, to refine our picture of where parolees are concentrated in Alameda, Kern, Los Angeles, and San Diego counties. As discussed in the methodology, we felt that it was informative to map the concentration of parolees at the county level in two ways. First, we shaded the maps based on the concentration of parolees using the rate of return for each census tract. Using parolee rates of return allowed us to identify which areas or neighborhoods in a county are more severely burdened by returning prisoners. This background was overlaid with the secondary clusters identified using the NNH methodology. A useful alternative would be to use raw counts of parolees as our background shading (rather than the rate of return). Such a measure might be useful for service providers and planners in understanding the actual number of parolees in a given area. The maps showing the actual counts of parolees can be found in Appendix A. Borders are shown in each of the maps in this report. These borders identify the supervisorial districts within each county. To maximize the use of our maps by policymakers, health providers, and community organizations, we included supervisorial district boundaries in the subcounty maps. For Los Angeles only, we also show the eight service planning area (SPA) borders in alternate maps. The SPA designation is used for health-planning purposes, with each SPA having a health office responsible for planning public health and clinical services according to the health needs of local communities (County of Los Angeles Department of Public Health, undated). Showing the SPA boundaries serves to provide additional context from a health-planning perspective in understanding where the health care impact of the reentry population may be greatest and to help focus efforts in this area. 44 Understanding the Health Implications of Prisoner Reentry in California, Phase I Report Alameda County. Figure 3.2 shows the cluster of parolees in Alameda County overlaid on the census tract parolee rates of return per 1,000 parolees. In Alameda County, we identified five distinct clusters of parolees concentrated primarily around Oakland and the northern section of the county (see Figure 3.3). These five clusters, located near south Oakland, Emeryville, Hayward, north Oakland, and San Leandro, account for almost 45 percent of the parolee population returning to Alameda County, suggesting that these areas are likely to be more severely affected by the health care needs of the reentry population. Figure 3.2 Relative Concentrations of Parolees in Alameda County Source: CDCR parolee data, 2005–2006. Distribution and Concentration of Parolees in California 45 Figure 3.3 Five Clusters in Alameda County Account for Nearly Half of Parolees 18 45 16 40 Cumulative percentage Percentage 12 35 30 10 25 8 20 6 15 4 10 2 5 0 South Oakland Emeryville Hayward Cluster RAND TR687-3.3 North Oakland San Leandro 0 Cumulative percentage 14 46 Understanding the Health Implications of Prisoner Reentry in California, Phase I Report Kern County. Figure 3.4 shows the clusters of parolees in Kern County overlaid on the census tract parolee rates of return per 1,000 parolees. Within Kern County, there are four distinct clusters of parolees (Figure 3.5)—two of the clusters show concentrations primarily around the urban area of Bakersfield, and two others are located in the northern and northeastern sections of the county (District 1). As Figure 3.5 shows, these four clusters account for almost 58 percent of the parolee population returning to Kern County, with Bakersfield alone accounting for nearly 50 percent of the returnees. Thus, the Bakersfield area disproportionately bears the burden with respect to meeting the health care needs of the reentry population in Kern County. Figure 3.4 Relative Concentrations of Parolees in Kern County Source: CDCR parolee data, 2005–2006. Distribution and Concentration of Parolees in California 47 Figure 3.5 Four Clusters in Kern County Account for More than Half of Parolees 60 60 58 50 Percentage 40 Cumulative percentage 54 52 30 50 20 48 10 0 46 Bakersfield Arvin/Lamont Delano Cluster RAND TR687-3.5 Ridgecrest 44 Cumulative percentage 56 48 Understanding the Health Implications of Prisoner Reentry in California, Phase I Report Los Angeles County. Figure 3.6 shows the clusters of parolees in Los Angeles County overlaid on the census tract parolee rates of return per 1,000 parolees. We see that there are many more clusters in Los Angeles County than in other counties. We identified 23 distinct clusters of parolees covering a large geographic area (Figure 3.7), suggesting that providing services to the reentry population requires a targeted approach in supervisorial districts. Two clusters are located in the northern part of the county around Lancaster and Palmdale and in the southern section of District 5 around Pasadena. The San Fernando Valley has three distinct clusters of parolees. The southern half of the county is where the majority of parolee clusters are concentrated, primarily around South and Southeast Los Angeles, Inglewood, Compton, and Long Beach (Districts 2 and 4) and in the eastern section of the county around Covina, West Covina, and Pomona (District 1). Figure 3.7 shows that 23 clusters in Los Angeles County are responsible for about 35 percent of parolees. Importantly, South Los Angeles, Southeast Los Angeles, and Long Beach are the largest clusters. It is interesting to note that Los Angeles County shows less concentration than the other three counties in the sense that the 23 clusters shown in Figure 3.7 account for only about a third of the county’s parolees, whereas just a handful of clusters in the other counties generally comprise nearly one-half of the counties’ parolees. Figure 3.6 Relative Concentrations of Parolees in Los Angeles County Source: CDCR parolee data, 2005–2006. S So ou ut th he Lo as s t L An os ge A le Lo ng s ng ele Be s W es Va ac tH n h ol Nu ly ys w N or Lan ood So th C cas ut o ter h mp Co t m on p Po ton m o W na hi La tt ke ier w G oo ar d In de gl n ew a La oo Pu d N en or th C te H ov ol in ly a w o N W or od es w Ly t C al nw k oo Pa ovi d/ sa na So de ut na h Pa Ga lm te Sa da n le H Pe aw d th ro or ne Percentage 7 40 6 35 5 RAND TR687-3.7 30 4 Cumulative percentage 2 Cluster 25 3 20 15 10 1 5 0 0 Cumulative percentage Distribution and Concentration of Parolees in California 49 Figure 3.7 Twenty-Three Clusters Account for 35 Percent of Parolees in Los Angeles County 50 Understanding the Health Implications of Prisoner Reentry in California, Phase I Report San Diego County. Figure 3.8 shows the clusters of parolees in San Diego County overlaid on the census tract parolee rates of return per 1,000 parolees. In San Diego County, there are eight distinct clusters of parolees (Figure 3.9). Three clusters are located in the northern part of the county around Oceanside, Vista, and Escondido (District 5 and the northernmost section of District 3). The remaining clusters of parolees are concentrated around downtown and southeast San Diego and eastward toward Spring Valley and El Cajon (Districts 4 and 2). In addition, there is a cluster of parolees around Chula Vista (District 1). Figure 3.9 shows that the eight clusters account for nearly half of the parolee population returning to San Diego County, with the largest clusters in areas of downtown San Diego and Southeast San Diego. Thus, communities within which these eight clusters are located will be disproportionately affected by the health care needs of the reentry population in San Diego County. Figure 3.8 Relative Concentrations of Parolees in San Diego County Source: CDCR parolee data, 2005–2006. Distribution and Concentration of Parolees in California 51 Figure 3.9 Eight Clusters Account for Nearly Half of Parolees in San Diego County 50 16 45 14 35 Percentage 10 30 8 25 20 6 15 4 10 2 0 5 Southeast Downtown San Diego San Diego RAND TR687-3.9 Lemon Oceanside Vista Grove/ Spring Valley Cluster Chula Vista El Cajon Escondido 0 Cumulative percentage 40 Cumulative percentage 12 52 Understanding the Health Implications of Prisoner Reentry in California, Phase I Report Analysis of Demographic Characteristics of California Parolees and the Socioeconomic Characteristics of Areas to Which They Return Methods In Chapter Two, we examined some of the characteristics of the inmate population nationally and in California. We did so because there are no available datasets that would have allowed us to characterize the physical and social-behavioral conditions facing parolees. However, the CDCR data allow us to summarize a limited set of characteristics of individuals returning to communities in California. Here, we use those data to determine the characteristics of returning parolees both for the state and for the four selected counties. In addition, we were interested in understanding whether parolees tend to locate in areas that reflect to some degree the characteristics of the parolees themselves (i.e., in terms of race/ ethnicity) and whether they tend to locate in areas that are disadvantaged, as measured by high rates of unemployment and poverty. To do so, we relied on census data to determine the demographic and socioeconomic characteristics of census tracts within California by race/ethnicity of the population, educational attainment, poverty rate, and degree of linguistic isolation of households. We aggregated the census tracts into seven clusters with similar characteristics. Specifically, to summarize census tract characteristics across the entire state, we used a statistical cluster analysis approach to group the tracts that were more similar to other tracts in the same cluster than they were to other tracts outside the cluster. We used a nonhierarchical method known as k-medoids to cluster the tracts. The k-medoids method is an alternative to the more commonly used k-means and has the added benefit of being less influenced by extreme values or outliers within a cluster. The tracts were grouped into a predetermined number of clusters that are usually arbitrarily chosen. We chose seven socioeconomic clusters to keep the number manageable and because we felt that seven was a reasonable number of clusters that could be easily mapped and still convey distinct characteristics for each cluster. We standardized all variables using the z-score. Results of Analysis of Demographic Characteristics of California Parolees Table 3.2 summarizes the demographic characteristics of parolees returning in calendar years 2005 and 2006—overall and then for each of the four counties highlighted in this report. To determine the characteristics of parolees, we used the entire sample of 176,618 parolees released in 2005 and 2006; however, for the analysis of the socioeconomic characteristics of the areas to which those parolees return, we used the subset of cases (137,305 parolees) that had valid census tract data. In calendar years 2005 and 2006, approximately 176,618 parolees were released. One out of five parolees returned to Los Angeles County. The characteristics of parolees did not differ significantly from what we saw in the inmate survey discussed in Chapter Two. Overall, nearly nine out of every 10 parolees were male, and the median age was 34 years. The four counties in our study accounted for nearly one-third of the parolees released in 2005–2006. Los Angeles County received one out of every five parolees in California, while San Diego County received 6 percent and Kern and Alameda counties each received 3 percent. The racial/ethnic composition of the parolee population varied by county. Overall, 38 percent of parolees were Hispanic or Latino. However, Los Angeles and Kern counties had a larger share of Hispanic or Latino parolees compared to Alameda and San Diego Distribution and Concentration of Parolees in California 53 Table 3.2 Demographic Characteristics of California Parolees and Terms Served, Overall and for Four Counties Variable California (n = 176,618; 100%) County Los Angeles Alameda (n = 35,710; 20%) (n = 4,689; 3%) San Diego (n = 9,782; 6%) Kern (n = 5,936; 3%) Demographic characteristics Gender: % male 89.04 88.10 90.47 88.06 87.50 34 34 34 35.5 33 % Hispanic 37.64 41.78 16.55 29.31 37.85 % black 25.69 36.08 61.93 27.09 18.62 % white 32.36 18.68 16.53 37.53 41.75 % other 4.31 3.46 4.99 6.07 1.78 % violent offenders 23.13 21.32 22.36 20.90 18.26 % property offenders 33.28 35.03 36.41 35.07 27.12 % drug-related offenders 32.23 31.54 32.56 34.90 42.81 Mean no. of prior offenses 1.60 1.76 1.57 1.88 1.35 Mean no. of serious priors 0.16 0.17 0.18 0.20 0.12 Mean no. of violent priors 0.14 0.21 0.20 0.13 0.07 Mean no. of offenses 1.46 1.44 1.37 1.45 1.63 Median age Ethnicity/race Characteristics of term served Source: CDCR parolee data, 2005–2006. Note: The numbers represent the total number of parolees released in calendar years 2005 and 2006. counties. This is consistent with the overall demographics of these counties.7 Although blacks or African-Americans account for only a quarter of the parolee population statewide, they represented three-fifths of parolees in Alameda County and 36 percent of parolees in Los Angeles County. With respect to the characteristics of the prison term served, nearly a quarter of parolees in this two-year period were violent offenders, a third were property offenders, and another third were incarcerated for drug-related offenses. There are few differences in the proportion of these offender categories across the four counties, except in Kern County, which appears to 7 Overall, Latinos/Hispanics represent about 36 percent of the total California population. Kern and Los Angeles counties’ share of the Latino/Hispanic population in 2007 was 46 percent and 47 percent, respectively; Alameda and San Diego counties’ share was 21 percent and 30 percent, respectively. African-Americans represent about 6.7 percent of the total California population. Alameda and Los Angeles counties had a larger share of the African-American population in 2007 (13.7 percent and 9.5 percent, respectively); San Diego and Kern counties’ share was 5.5 percent and 6.4 percent, respectively (U.S. Census Bureau, 2009a). 54 Understanding the Health Implications of Prisoner Reentry in California, Phase I Report have a higher proportion of drug-related offenders among its total 5,936 returning parolees. The average number of prior offenses was 1.6, with those returning to Los Angeles and San Diego counties having a somewhat higher mean number of offenses. Results of Analysis of Socioeconomic Characteristics of Areas to Which Parolees Return In terms of where the parolees return, we grouped the census tracts into seven categories or clusters that summarize the key socioeconomic features. Figure 3.10 shows the seven socioeconomic clusters that we use to characterize California. We described how the socioeconomic clusters were derived on page 52. Figure 3.10 Summary of Statewide Socioeconomic Clusters of Census Tracts Distribution and Concentration of Parolees in California 55 Table 3.3 summarizes the socioeconomic characteristics of the seven cluster areas shown in Figure 3.10. For example, Cluster 5 (shown in red in Figure 3.10) includes areas of greatest disadvantage: 33 percent of the households are linguistically isolated, 8 50 percent of adults age 25 years or older do not have a high school diploma, the median unemployment rate is 7.1 percent, and the median household poverty rate is 24.6 percent. With the exception of linguistically isolated households, Cluster 5 has the highest level of disadvantage in each of the socioecomonic characteristics. Table 3.4 summarizes the distribution of parolees overall and by race/ethnicity across the seven socioeconomic clusters. The three most common socioeconomic clusters to which parolees returned were Clusters 4, 5, and 6. Overall, 43 percent of parolees returned to Cluster 5 areas (shown in red in Figure 3.10). We see important differences by race/ethnicity. About half of African-American and Latino parolees returned to disadvantaged areas, such as Cluster 5 areas; only a little more than a quarter of white parolees returned to Cluster 5 areas. Cluster 5 can be characterized as areas of disadvantage as measured by high poverty rates, high unemployment rates, and low educational attainment (Table 3.3). That is, 25 percent of households in Cluster 5 areas are below the federal poverty line (FPL), the average unemployment rate is 7.1 percent, and 50 percent of adults 25 years or older do not have a high school diploma.9 In terms of Cluster 5’s ethnic composition, 64 percent of the population is Hispanic or Latino and 13 percent is African-American (Table 3.3). Table 3.3 Socioeconomic Characteristics of the Seven Cluster Areas Race/Ethnicity (%) Socioeconomic Characteristics (%) White Black Hispanic Asian Linguistically Isolated Householdsa 1 39.3 5.6 17.5 33.3 11.3 14.6 3.3 7.5 2 32.0 4.8 54.4 5.6 15.2 32.5 4.8 11.0 3 63.5 2.7 25.4 3.9 32.6 22.2 4.6 12.2 4 72.4 3.6 12.6 6.7 4.8 9.5 3.0 5.9 5 15.9 13.3 63.7 4.6 33.4 50.0 7.1 24.6 6 25.0 8.5 38.3 24.1 35.0 33.8 4.9 18.0 7 77.1 1.7 13.0 4.2 17.7 11.9 3.2 7.9 Cluster No HS Diplomab Unemployment Household Ratec Poverty Rated a The U.S. Census Bureau defines linguistically isolated households as households in which no one 14 years or older speaks only English or speaks a non-English language and speaks English “very well.” b No high school diploma is measured as the percentage of adults 25 years or older without a high school diploma. c Unemployment rate is calculated for those 16 years or older who are in the labor force. d Household poverty rate is the percentage of households below the FPL. 8 Linguistically isolated households are households in which no one 14 years or older speaks only English or speaks a nonEnglish language and speaks English “very well.” 9 The unemployment rate includes adults age 16 years or older who are in the labor force. 56 Understanding the Health Implications of Prisoner Reentry in California, Phase I Report Table 3.4 Distribution of Parolees Across the Seven Cluster Areas, Overall and by Race/Ethnicity African-American Cluster Total Parolees Latino White % of Total Parolees n % n % n % 1 8,667 6.3 2,327 6.4 2,801 5.7 2,618 5.7 2 11,359 8.3 1,744 4.8 6,100 12.4 3,184 6.9 3 12,961 9.4 2,222 6.1 3,462 7.0 6,763 14.7 4 20,411 14.9 3,876 10.7 4,909 10.0 10,727 23.3 5 58,665 42.7 19,572 54.2 24,779 50.3 12,721 27.6 6 14,268 10.4 4,941 13.7 4,677 9.5 3,486 7.6 7 10,974 8.0 1,454 4.0 2,510 5.1 6,547 14.2 137,305 — 36,136 — 49,238 — 46,046 Total — Note: The category for other race/ethnicity is not shown. Table 3.4 also shows that, overall, 10 percent of parolees returned to Cluster 6–type areas (shown in maroon in the map in Figure 3.10). Parolee returns varied somewhat by ethnicity. Fourteen percent of African-American parolees, 10 percent of Latino parolees, and 8 percent of white parolees returned to Cluster 6 areas. Cluster 6 can be characterized as areas of disadvantage as measured by high poverty rates, households that are linguistically isolated, and low educational attainment (Table 3.3). That is, 18 percent of households are below the FPL, 35 percent of households are considered linguistically isolated, and 34 percent of adults 25 years or older do not have a high school diploma. In terms of its ethnic composition, Cluster 6 has no single race/ethnic group that dominates: 38 percent of the population is Hispanic or Latino, 24 percent is Asian, and 25 percent is white (Table 3.3). Finally, Table 3.4 shows that, overall, 15 percent of parolees returned to Cluster 4–type areas (shown in blue in the map in Figure 3.10). More white paroles (23 percent) than African-American (11 percent) and Latino parolees (10 percent) returned to Cluster 4 areas. Cluster 4 areas fare better on all of the socioeconomic indicators shown in Table 3.3. In addition, the population of Cluster 4 areas tends to be predominantly white (72 percent) (Table 3.3). Figure 3.10 also allows us to examine the clusters within the four counties we selected for this study. We see that Alameda County is characterized by three cluster types: Cluster 4 predominates in the eastern half of the county; Cluster 5, in the areas near San Lorenzo, Oakland, Alameda, and Berkeley; and Cluster 3, in the areas around Hayward and Union City. Kern County is a mixture of socioeconomic clusters, with Cluster 5 being predominant around the city of Bakersfield; Cluster 3, in the eastern third of the county; and Cluster 7, in the middle of the county. Los Angeles County is characterized by Cluster 5 areas in South Los Angeles, Long Beach, parts of the San Fernando Valley, and Palmdale/Lancaster. Cluster 3 predominates in parts of the county that include downtown Los Angeles, Pasadena, Monrovia, Covina, and Pomona. Distribution and Concentration of Parolees in California 57 The western third of San Diego County is characterized by Cluster 4, with concentrations of Cluster 5 areas around San Diego, National City, and Imperial Beach. The eastern third of the San Diego County is characterized by Cluster 3 areas. Discussion A number of trends complicate the successful reentry of parolees into communities. Our analysis of the distribution and concentration of parolees across California and within the four counties showed that certain counties have higher rates of return. Furthermore, there are distinct clusters of parolees across California and within counties. This has implications in terms of targeting reentry resources to these areas. Parolees located in more rural counties tend to be more dispersed, as measured by our cluster analysis, suggesting that a different strategy will be needed to provide services to these individuals. In terms of the demographic composition of the reentry population, parolees are predominantly male, with a median age of 34 years. The racial and ethnic composition of the reentry population is important for understanding their needs. For example, if a given number of parolees are foreign-born, language issues may complicate outreach, and addressing language barriers should be a critical component of reentry programs. The racial/ethnic composition of those released from California prisons varies across the four counties of interest. In Alameda County, two out of three parolees are African-American, whereas in Los Angeles and Kern counties, one out of four parolees are Latino/Hispanic. In San Diego County, the composition of parolees is more evenly distributed across the various racial/ethnic groups. With respect to reason for incarceration, a third of parolees in California were in prison for drugrelated offenses and another third for property-related offenses. One might expect some overlap between these two groups, with drugs being a common denominator. Finally, in California, African-American and Latino parolees, in particular, tend to return to disadvantaged areas, as measured by high poverty rates, high unemployment rates, low educational attainment, and more households that are linguistically isolated. This suggests that, for these individuals, reentry will be even more challenging because of poor employment opportunities and other factors. Further, serving time in prison tends to have a large and persistent negative impact on earnings, especially for older offenders and those in white-collar occupations (Western, Kling, and Weiman, 2001). Then again, one might argue that returning to communities from which they came may have a positive effect on parolees by increasing the chances that they will have family or other support to assist with the reentry process. Having close family ties has been shown to be an important predictor of successful reentry, yet correctional policies often undermine the maintenance of ties between prisoners and families, with a majority of state prisoners being held in facilities more than 100 miles from their homes (Mumola, 2000). Research has shown that inmates who are released into the community and who have close ties to family and friends tend to have lower recidivism rates than those without close contacts (Travis and Waul, 2003). Further, we know that the length of time that released offenders have served in prison has increased. Longer prison stays also have implications for the reentry process; in particular, there is evidence that, over time, inmates begin to lose contact with family members—contact that is important for facilitating reintegration into the community (McMurray, 1993; Davis and Pacchiana, 2004). As noted in Chapter Two, however, the effect of family can also be 58 Understanding the Health Implications of Prisoner Reentry in California, Phase I Report mixed in that family members who are dealing with their own crises, such as poverty, physical or mental problems, or drug addiction, may not be in a position to help with reentry; substance abuse and other negative influences can also be a risk factor for an individual’s subsequent involvement with the criminal justice system (Travis and Waul, 2003). While the needs of parolees may be evolving, it is also the case that their needs are not evenly distributed. In the United States, returning prisoners increasingly are concentrated in core counties—that is, counties that include the central city of a metropolitan area. Our maps of California confirm that a very limited number of counties and communities within counties account for a disproportionate share of parolees. Within central cities, parolees are traditionally concentrated in relatively few communities and in the poorest neighborhoods. This concentration suggests that returning prisoners will find themselves competing with those leaving welfare for similar low-skill jobs. It also raises the question about the capacity of these communities—especially the capacity of their health care, mental health, and substance abuse safety nets—to absorb and reintegrate ex-prisoners. Further, this trend comes at a time when the nation’s and California’s economic downturn has seriously strained many communities’ health care resources and public health systems. Chapter Four Conceptual Framework and Methods for Defining the Health Care Safety Net for Parolees In Chapter Three, we analyzed the distribution and concentration of parolees in California as well as the overall characteristics of parolees and the communities to which they are disproportionately returning. In particular, we discussed the results of our analysis, identifying the locations with the greatest concentration of parolees in the state by county based on the census tract of residence. We then provided an in-depth examination of four counties: Los Angeles, Alameda, Kern, and San Diego. In Chapters Five and Six, we focus on analyzing how well the health care safety nets of these four counties are able to meet the health care needs of the reentry population in terms of accessibility, looking first at health care treatment needs (Chapter Five) and then at mental health and substance abuse treatment needs (Chapter Six). However, to examine the four counties’ health care safety nets and their ability to meet the health care needs of the reentry population, we first must define what we consider to constitute the safety net for the parolee population. This chapter starts by presenting our conceptual framework, which is the basis for identifying the different components of the health care, mental health, and substance abuse treatment safety nets for parolees. We then summarize the methods used to define each of the safety nets for the four counties that were the focus of this study and to analyze parolees’ access to safety-net facilities. These methods underlie the analyses presented in Chapters Five and Six. Conceptual Framework To conceptualize the health care safety net for the reentry population, we drew on two prior frameworks, both of which extend the much-used Andersen behavioral model of access to care. The Andersen model (Andersen, 1968; Andersen and Newman, 1973) emphasizes the importance of predisposing factors (demographics, social structure, health beliefs), enabling factors (personal, family, and community resources), and illness level or need factors. The first extension, by Davidson et al. (2004), builds on the Andersen framework to examine the effects of safety-net and other community-level factors on access and access outcomes, particularly among low-income populations. The second extension, by Gelberg, Andersen, and Leake (2000), includes factors within the predisposing, enabling, and need categories that are particularly important to consider when studying access by vulnerable populations, such as the homeless. We modified these frameworks to include predisposing and enabling factors and community characteristics that are important to the reentry population and access outcomes at the individual level (see Figure 4.1). 59 60 Understanding the Health Implications of Prisoner Reentry in California, Phase I Report Figure 4.1 Conceptual Framework for Evaluating the Health Care Safety Net for the Reentry Population Individual and Community Characteristics Individual Characteristics • • • • • • Predisposing Needs Demographics Social factors Health beliefs Incarceration history Prison health care experience Length of time in community Health Care Needs • • • • • Chronic conditions: hypertension, diabetes Infectious diseases: HIV, TB, Hep B, Hep C Mental illness Substance abuse Prevention needs Enabling Needs • Personal/family resources: income, health insurance, social support • Competing nonhealth needs: housing, employment, physician supply, managed care penetration/competition, hospital bed supply Community Characteristics Health Care Access and Outcomes for Reentry Population Potential Access • Usual source of care • Accessibility/capacity of safety-net providers Realized Access • • • • Primary care visits Hospital/emergency dept. visits Preventive screening Infectious disease treatment and monitoring • Psych and drug treatment visits • Preventable hospitalizations • Unnecessary delays in receiving care • County and public hospitals, clinics, public access programs • Community resources (CBOs and FBOs) for medically indigent and reentry population • Specific parolee networks of care (e.g., PSN) • Other characteristics (insured and uninsured) NOTE: CBO = community-based organization. FBO = faith-based organization. PSN = Parolee Services Network. RAND TR687-4.1 Figure 4.1 provides an overview of the conceptual framework used in this study. The framework shows three needs under individual characteristics: predisposing needs, health care needs, and enabling needs. Under “predisposing needs,” we include those that commonly affect individuals’ access to and use of health services, such as demographics (age, gender, raceethnicity), social factors (education, employment), and health beliefs (regarding disease and health services), as well as factors that are particularly relevant to the reentry population, such as incarceration history, prison health care experiences, and length of time in the community. Under “health care needs,” we include those health conditions that disproportionately affect the reentry population (infectious diseases, such as HIV, TB, hepatitis B and C; mental illness; substance abuse; chronic health conditions, such as hypertension and diabetes; and preventive screening). In Chapter Two, we discussed how California prisoners bear a high burden of chronic diseases, such as hypertension and asthma, and infectious diseases, such as hepatitis and TB. Prisoners’ mental health and substance abuse treatment needs were even more pronounced than their physical health needs, with more than half of California inmates reporting recent mental health problems and more than three-quarters reporting substance abuse or dependence. Although inmates provide an imperfect proxy for parolees’ health needs, the findings in Chapter Two provide us with reasonable insight into the needs of the reentry population. Conceptual Framework and Methods for Defining the Health Care Safety Net for Parolees 61 Under “enabling needs,” we include personal/family resources, such as income, health insurance, and social support. In Chapter Two, we discussed the socioeconomic characteristics of prisoners that will potentially influence their access to health care, both prior to incarceration and upon release from prison, including low educational attainment, high unemployment, extensive incarceration history, and high rates of homelessness, as well as other adverse life events. We also reported on prison health experiences, including the use of prison health services. In addition, we discussed variability in such enabling factors, such as the degree of family ties and social support, with three-quarters of prisoners having lived in a family before their arrest, one out of five prisoners being currently married, and half having minor children. The relative strength of these predisposing and enabling factors varies by race and ethnicity. In Figure 4.1, “community characteristics” draws on the Davidson et al. (2004) framework and elaborates on the safety-net characteristics and other community-level factors that affect the availability and accessibility of health services for the reentry population. We define the safety net for the reentry population as health resources that aim to provide services to the uninsured or medically indigent adults. Parolees must share these limited resources with other vulnerable populations, such as the uninsured, Medicaid recipients, the homeless, and undocumented immigrants. The safety-net population is an important community characteristic to measure because of competition for scarce resources. Pauly and Pagán (2007) found negative spillover effects from the uninsured to the insured. They found that a higher financial burden of uncompensated and charity care at the local level appears to affect insured individuals in terms of access and the overall quality of care provided within a community. California’s safety net includes county facilities (hospitals, clinics, and public health centers), free and community clinics, some private hospitals, physicians who see large numbers of uninsured patients, and some health plans that have designated innovative coverage plans for the uninsured (Tuttle and Wulsin, 2008). In addition, there are community resources that are specific to the reentry population (e.g., health care, employment or housing programs, such as Second Chance in San Diego, that assist parolees in meeting other needs and help them link to health care services and other case management services). We consider hospitals and clinics in our mapping efforts in Chapters Five and Six but excluded private physician practices for two reasons. First, while some may provide charity care to the indigent, parolees tend to be a difficult population to treat in these settings (for example, because of their threatening appearance, homelessness, and high risk). In our view, county and community resources (rather than private providers) most likely will be the front line in providing services to this population. Second, from a practical standpoint, we could not identify a comprehensive source of information on the charity care provided by private physician practices. In California, county health systems that serve the indigent adult population vary considerably in terms of funding, access, and eligibility. For example, provider counties, such as Los Angeles and Alameda, have public hospitals, whereas payer counties contract with private providers. Small counties pool their resources to form a uniform Medi-Cal–like system, and hybrid counties combine county clinics and private hospitals. State and federal governments are the primary source of funding for county care to the uninsured, with county funding varying considerably (Tuttle and Wulsin, 2008). County health care resources can include government and public hospitals, county primary care clinics, public health centers, and county mental health and substance abuse treatment providers. In addition, counties may contract with private nonprofit and for-profit clinics and hospitals to provide services to the safety-net population. For example, county/city and 62 Understanding the Health Implications of Prisoner Reentry in California, Phase I Report public hospitals in such counties as Los Angeles and Alameda are an important component of the health care safety net, and their emergency departments often become the default source of care for the medically indigent and uninsured. In other counties, such as San Diego, teaching and private hospitals are contracted to provide services to the medically indigent. In addition to county resources, other community resources, such as free and community clinics, play an important role in the safety net. These providers vary in their relationships to county indigent systems, with some clinics being reimbursed for providing services to the indigent population while other clinics are not. They also vary widely in terms of services provided, degree of specialization, sophistication, and complexity of their clinic operations, as well as in the mix of patients they serve (e.g., concentration on the uninsured or Medi-Cal or a combination of the two) (Tuttle and Wulsin, 2008). In some communities, nonprofit clinics and private hospitals work collaboratively in delivering care to the uninsured. Likewise, some private and public hospitals cooperate in caring for the uninsured. Hospitals sometimes help fund community clinics for the uninsured, while others have helped start and fund local coverage initiatives for the uninsured (Tuttle and Wulsin, 2008). Finally, in the area of mental health and substance abuse treatment, there are specific and small networks targeted to the parolee population. For example, in certain counties, the PSN provides substance abuse services funded by the state that target a relatively small number of parolees. Counties vary considerably in how they allow medically indigent adults to access services; this is compounded by variability in access and eligibility rules for parolees. For example, in Los Angeles County, parolees must be referred by Parole Outpatient Clinics (POCs) to access county mental health services. However, this is not necessarily the case in other counties. In addition, parolees are eligible for substance abuse services in some counties through several different mechanisms, including a small parolee-specific network of care, Proposition 36 drug treatment programs, and general county substance abuse services. Together, individual and community characteristics ultimately affect the health care access of the reentry population (shown in Figure 4.1), including (1) potential access (usual source of care, accessibility/capacity of safety-net providers) and (2) realized access, or health services utilization (doctor and clinic visits and preventive screening, plus areas of utilization that are particularly relevant for the reentry population, such as emergency department visits and mental health and drug treatment services), and access outcomes, such as preventable hospitalizations or unnecessary delays in receiving care. Figure 4.1 and the previous discussion summarize the broader conceptual framework to understand how the reentry population fits into the larger picture of California’s safety net. In this study, we were interested in defining the potential safety net for parolees in terms of health care, mental health care, and substance abuse treatment and in describing how it varies across the four counties. In addition, we focused specifically on parolees’ potential access to safety-net services, taking into account the capacity of safety-net providers and the potential demands placed on these facilities from other sources. This report addresses potential access, but it does not consider realized access per se in terms of whether parolees take advantage of this access or whether they have better outcomes as a result. In the second phase of this study, we will examine in depth why parolees might not take advantage of these resources (i.e., barriers to access), the challenges faced by parolees and providers, and options for removing the identified barriers. Conceptual Framework and Methods for Defining the Health Care Safety Net for Parolees 63 Overall Approach to Defining the Safety Net for the Reentry Population and Constructing Accessibility Measures Given the health and social-behavioral concerns with which parolees may present, we wanted to understand whether parolees reside in areas where they have access to the necessary care. Using the maps in Chapter Three that showed parolee concentrations in the four counties, we took both a geographic and statistical approach. For the four counties, we mapped nonprofit and government/public general acute short-stay hospitals1 and community and free clinics to examine the health care safety net. To assess resources for social-behavioral needs, we also mapped mental health and alcohol and drug facilities to examine the mental health and substance abuse safety nets. For each county, we compiled comprehensive lists of health care, mental health, and substance abuse treatment safety nets for the uninsured and low-income populations through publicly available lists and consultations with local health leaders. We used the geographic locations of the facilities for mapping purposes. Distances were calculated based on the exact location of the facility and the approximate location of the parolee (i.e., population-weighted centroid of the residential census tract). While simply enumerating and mapping the facilities within a certain distance is informative, there are some limitations to such a measure. The first issue is whether a particular facility is truly available to parolees. Not all facilities serve the poor, and other facilities focus on specific populations, such as children or women. To address these concerns, we further limited our mapping to only facilities that we believed would be “available” to the parolee population. For example, hospitals serving primarily children and women are not likely to provide significant health care resources to the predominantly adult male parolee population. Likewise, many for-profit or investor-owned hospitals would not likely provide a significant share of uncompensated care to this population. In addition, there are defined networks that specifically provide mental health and substance abuse services to parolees. Given these differences, we developed a methodology specific to each facility type and, where relevant, each county. We provide a brief description later in this chapter, but specific details on the methodologies used to include or exclude particular facilities of each type (e.g., substance abuse) are provided in Appendix B. A second concern in simply enumerating the number of facilities is that not all facilities should be considered equally in a measure of accessibility. For example, smaller hospitals may have fewer resources available to serve their communities than larger hospitals. Similarly, hospitals in more disadvantaged areas may have more severely stressed resources because of demand from other vulnerable populations. The approach we used was to develop a measure of accessibility based on methodologies that measure potential accessibility (see, e.g., Luo and Wang, 2003). To construct the accessibility measures, we needed a measure of the size of the facility (capacity) and the demands placed on it (utilization). We considered different measures of size that would capture differences in capacity and utilization-adjust (i.e., weight) the facility based on availability of resources. We ultimately decided to use total full-time equivalents (FTEs) as our measure of capacity for both hospitals and clinics.2 Unfortunately, we did not have available a measure of capacity for the mental health and substance abuse providers; thus, we simply 1 2 We included nonprofit and government/public hospitals in our analysis. We excluded investor-owned hospitals. We also considered beds for hospitals, but there is no equivalent on the clinic side. Moreover, the bed option fails to account for resources in the emergency department. 64 Understanding the Health Implications of Prisoner Reentry in California, Phase I Report used each facility as a single unit of supply (which is equivalent to weighting them equally). In calculating the accessibility measures, we also took into account the potential demands (utilization) for these resources from the population in that community. In this case, we used the total population living in households with income below the FPL as our measure of potential demand for safety-net facilities that would likely serve the parolee population. Finally, to calculate the accessibility measure, we had to choose a cutoff in terms of proximity—that is, how close the facility needed to be for it to be “counted” in the accessibility measure for a given census tract. We chose a drive time of 10 minutes as the cutoff for all the accessibility maps. We used a shorter drive time because of concerns that this population may be less mobile than the general population and likely more reliant on public transportation. The additional benefit of the accessibility measures is that they also allow us to summarize whether certain groups of parolees, for example, Latinos and African-Americans, are more or less likely to have access to scarce health care resources. A more detailed summary of the construction of the accessibility measures is provided next and in Appendix B. Methods for Defining County Safety Nets and Developing Accessibility Measures Previously, we described our overall approach to defining the safety net for the reentry population and in constructing measures of accessibility. Here, we provide specific details about how we defined the various safety nets for each of the four counties and the methods used to develop a set of accessibility measures for hospitals and clinics and for mental health and substance abuse treatment facilities. For a more detailed discussion of the methodology for each type of facility, see Appendix B. Defining the Health Care Safety Net To define the health care safety net available to the parolee population, we used the following strategy. We first identified for each county the hospitals and clinics that were part of the Medically Indigent Services Program (MISP). “Under California law, counties are the ‘providers of last resort’ for health services to low-income uninsured people with no other source of care” (California HealthCare Foundation, 2006a). The larger and more urban counties each have their own distinct MISP and discretion in setting funding levels, eligibility requirements, enrollment procedures, and covered health services. Note that indigent mental health services, not typically provided by MISPs, are available through other county programs, and no MISP county covers substance abuse treatment (California HealthCare Foundation, 2006b). We relied on the California HealthCare Foundation’s list of MISP providers but augmented it with county lists whenever available. For example, Alameda County listed more locations for MISP providers than were on the California HealthCare Foundation list, so we added these additional locations.3 Almost all of the MISP providers are also Federally Qualified Health Centers (FQHCs). 3 For each county, we included all the MISP providers listed in the California HealthCare Foundation’s report (2006b) and by the county itself, with one exception. For San Diego County, Loma Linda University Community Medical Center was listed as an MISP hospital provider. However, this facility is located outside the county and not near the county’s Conceptual Framework and Methods for Defining the Health Care Safety Net for Parolees 65 With respect to non-MISP clinics, we used California Office of Statewide Health Planning and Development (OSHPD) data for 2005 and 2006 to identify other primary care clinics that provided outpatient services in each county. This list included community clinics as well as free clinics and mobile clinics. Their inclusion in the broader category of clinics provided insight into their role in filling in gaps in the safety net. We similarly used the OSHPD hospital data to identify the other general acute care hospitals in each county (i.e., the non-MISP hospitals). We included a flag for public hospitals and for other general acute care hospitals. We excluded from our analysis specialty hospitals, investor-owned hospitals, and those that serve only children or women.4 In other words, we included in our list general acute care hospitals that were either nonprofit or government/public hospitals.5 By this categorization, we also captured teaching hospitals. Mapping the other general acute care hospitals in a county provides an overview of what geographic areas these hospitals cover; some of the hospitals may also provide uncompensated care. For Los Angeles County, we categorized the hospitals and clinics somewhat differently than for the other three counties because Los Angeles County is the only county that has three components to its MISP (California HealthCare Foundation, 2006b): (1) MISP ability-topay (ATP) hospitals and clinics, 6 (2) MISP Outpatient Reduced-Cost Simplified Application (ORSA) hospitals and clinics,7 and (3) MISP public-private partnership (PPP) clinics.8 In Los Angeles County, the lists of county providers (hospitals and clinics) for the ATP and ORSA programs are the same. The ATP and ORSA clinics include personal health centers, comprehensive health centers, and one county public health center.9 The PPP program list of primary care clinics differs from that of the ATP/ORSA programs, representing instead noncounty clinics that are contracted to provide services (i.e., MISP PPP clinics). For the list of Los Angeles County’s other primary care clinics (PCCs), we used a list of clinics participating in the county’s PPP program and augmented it with data from a borders. Therefore, for mapping purposes and measures of accessibility, we omitted this facility from our list of San Diego MISP hospitals. 4 The exclusion of hospitals that serve only women and children follows from the fact that the vast majority of parolees are male and this population was the focus of our study. 5 We used two OSHPD data sources to fill in the type of hospital. We used the OSHPD licensure data file (CNTRL_ CTGY variable) to identify control category (nonprofit, investor, government). For hospitals that were missing data for this variable, we used the OSHPD financial data (TYPE_CNTRL variable) to fill in this information. If there was a discrepancy between the two variables in terms of how a hospital was categorized, we assumed that the licensure variable CNTRL_ CTGY was the correct categorization. Any hospitals missing data on both variables were omitted from the analysis file. Any investor hospitals also were omitted. 6 The ATP program provides low-cost/no-cost inpatient and outpatient services in county facilities to medically indigent persons who are not fully covered by Medi-Cal, Medicare, private insurance, or other medical benefits. Income eligibility is determined on a sliding scale based on Medi-Cal maintenance, and assets are considered for liability compensation. 7 The ORSA program similarly provides low-cost/no-cost inpatient and outpatient services in county facilities to medically indigent persons who are not fully covered by Medi-Cal, Medicare, private insurance, or other medical benefits. However, income eligibility is based on 133.33 percent of the FPL, and eligible applicants must also apply for Medi-Cal. 8 The PPP program is a collaboration between county health services and private community providers; it provides outpatient services and is considered part of the safety-net system in Los Angeles County. Income eligibility is based on 133.33 percent of the FPL, with no cost for those using PPP clinics who are on General Relief or who are not qualified for Medi-Cal or other assistance programs. 9 The one county public health center is Glendale Health Center, which provides medical services in addition to public health services. 66 Understanding the Health Implications of Prisoner Reentry in California, Phase I Report list of California clinics that provide general medical services to adults for free or on a slidingscale basis.10 We also used information compiled by Kristina Cordasco and Ninez Ponce, who updated the list of California health care safety-net organizations, in the California HealthCare Foundation’s 2005 report, California’s Safety-Net Clinics: A Primer (Saviano and Powers, 2005).11 Developing a Measure of Accessibility for Hospitals and Primary Care Clinics To understand the interaction between prisoner reentry and the health care safety net beyond simple mapping, we generated quantitative measures of accessibility. The term accessibility refers to the relative ease by which locations of activities, such as work, shopping, and health care, can be reached from a given location (BTS, 1997). Because we do not have access to data that could reveal actual access and utilization of health care services by parolees in California, we have settled for a measure of accessibility from a single point in an area—in this case, census tracts. Our measures provide useful summaries of the availability of safety net resources and allow us to examine how that availability varies for the parolee population. These measures take into account the supply of what we considered to be the relevant facilities comprised by the health care safety net and the potential demands placed on it by those who may not have the ability to pay for services. We adopted Allard’s (2004) notion of potential demand as being the total population living in households with incomes below the FPL. In addition, we used total FTEs as our measure of capacity for these facilities (when available). In addition, the measures take into consideration how existing facilities that compose the health care safety net are geographically distributed. To improve our estimate of the approximate location of the majority of the resident population, we used a population-weighted geographic center point, or centroid, as opposed to the geometric center point of census tracts. The method we used for calculating the accessibility of health care safety-net facilities to census tracts in California is a GIS-based method called “the two-step floating catchment area” method. The measure is a gravity-type measure in that it incorporates distance, supply, and demand in calculating a measure of accessibility. However, it computes a more easily interpretable measure of accessibility than traditional gravity models. The method requires computing the distance matrix from all census tracts in an area and then using it to select all population-weighted centroids within the threshold travel time (10 minutes drive time) of each health facility location. In the first step, the ratio of supply to demand was calculated for each facility. Facilities with missing FTE data were dropped from the analysis. Unfortunately, in some cases, the amount of missing capacity data was significant, so alternate measures were constructed using each facility as a single unit of supply to evaluate sensitivity to missing data (see the next section, titled “Sensitivity Analysis”). In this alternate measure, the ratio is interpreted as facilities per potential demand and represents the number of facilities per 10,000 10 This list was provided to us by Allison Diamant, M.D., M.P.H., who has collaborated with the Los Angeles County Health Department on studies and health care access issues. 11 Kristina Cordasco, M.D., of the Robert Wood Johnson Clinical Scholars Program and the Veterans Health Administration and Ninez Ponce, Ph.D., of the National Institutes of Health/National Cancer Institute updated the California HealthCare Foundation’s list of safety-net organizations with governmental listings of all California FQHCs, FQHC lookalikes, community health clinics, free clinics, rural health clinics, and county clinics. In addition, they administered a brief telephone survey to confirm the information provided. Conceptual Framework and Methods for Defining the Health Care Safety Net for Parolees 67 population in a county. In the next step (for both measures), we used the same distance matrix to determine for each population centroid the number of facilities within the threshold travel time. The sum of the supply-to-demand ratios of the selected facilities is the computed accessibility for the census tract. For the accessibility measures that use FTE data, each accessibility bin or category represents the number of FTEs per 1,000 population within a 10-minute drive time of the population-weighted centroid of a census tract. For the alternate measures that use the simple count of facilities and that were created for our sensitivity analysis, the accessibility bins or categories represent the number of facilities per 10,000 population within a 10-minute drive time of the population-weighted centroid of a census tract. The resulting accessibility for a census tract can be viewed as the estimated average accessibility measure of all persons in the tract, and it incorporates all demands made on a facility from the surrounding area of that facility—not just the tract centroid-based buffer area of each tract. Tracts whose buffer areas (within the travel time threshold) overlap with others in the catchment of the facility are factored in as well. For example, a facility with a relatively high capacity may be located in tract A with high potential demand. However, it is also within the travel-time threshold of other nearby tracts with high potential demand; thus, the net result is that the accessibility of tract A may be lower because of the competing demands on the facility from other census tracts that are within 10 minutes of it. In the resulting maps, we classify the accessibility scores for each census tract into four bins, or quartiles, and color the areas four shades of green. Thus, 25 percent of all census tracts in a county with the lowest accessibility scores are shaded the lightest green on the maps and the 25 percent of census tracts with the highest accessibility scores are shaded the darkest green on the maps. The quartile bins, or map classifications, were constructed separately for each county, not over all four counties. Thus, each classification scheme is based on the distribution of accessibility values for all census tracts in a single county, not on the full distribution in the state or across multiple counties.12 However, one can still make comparisons across counties in terms of differences in relative levels of accessibility. In mapping the health care facilities, we considered only what is available within a county’s border. However, we recognize that some concentrations of parolees near a county’s border may also be near facilities in neighboring counties. Therefore, in calculating the accessibility measure for hospitals, we included hospitals in neighboring counties. However, in calculating the accessibility measure for clinics, we could not to do so because of the challenges in collecting reliable data on these facilities. Therefore, our maps for hospitals and clinics, and our accessibility measure for clinics only, likely underestimate accessibility in some areas along the borders of our study counties. Sensitivity Analysis. Our measures of accessibility for hospitals and clinics use FTE as a proxy for a facility’s capacity. The first sensitivity analysis examined the maps when the number of beds was used as a measure of capacity for hospitals, instead of FTEs. These results were qualitatively similar. A more serious concern, however, was that some hospitals and clinics were missing capacity data. In these instances, we had to drop these facilities from our calculation of the accessibility measures. To assess the effect of missing capacity data, we conducted a sensitivity analysis, examining whether the accessibility patterns within a county changed 12 It is important to keep in mind that the sizes of the census tracts differ by county, with less populated counties tending to have larger census tracts. Thus, it may appear, for example, that there are large underserved areas within a county as an artifact of differences in the size of census tracts. 68 Understanding the Health Implications of Prisoner Reentry in California, Phase I Report when using a simple count of facilities rather than capacity in calculating an alternate measure of capacity. This is equivalent to an unweighted accessibility measure versus that weighted by capacity. Chapter Five, presents the results of the sensitivity analysis for each facility type. Defining the Mental Health Safety Net Our first step was to define the facilities that constitute the mental health safety net for the uninsured and low-income population. The Mental Health Services Act (MHSA), passed in November 2004 and known as Proposition 63, provides funding to counties to expand their programs and develop integrated mental health services for children, adults, and older adults. The counties have taken different approaches in terms of how they use these funds, including focusing on the direct provision of services; on special groups, such as children and families; or on providing prevention, education, or other services in addition to direct mental health care. We used the MHSA list of hospitals and clinics for each county as a starting point for identifying the mental health safety net for low-income and under- and uninsured populations. Many of the MHSA hospitals and clinics are county providers or community providers contracted by the county. We were able to obtain a list of MHSA providers only for Los Angeles and San Diego counties. Kern County has not yet identified its MHSA providers; therefore, we relied on Kern County’s list of Medi-Cal mental health services contract providers. We were unable to locate a list for Alameda County’s MHSA providers and so were unable to differentiate between MHSA and other mental health providers for this one county. To identify the MHSA and Medi-Cal contract providers and other mental health clinics, we used the list of mental health facilities from the Substance Abuse and Mental Health Services Administration (SAMSHA) locator database. We augmented the list of providers using available information from each county (see Appendix B). Our approach to defining the mental health safety net was different from the approach we used for clinics and hospitals. In addition to mapping the safety net for the uninsured and medically indigent population, we also defined a separate mental health safety net that is specific (rather than available) to parolees by mapping the location of the POCs. The process by which a prisoner or parolee accesses POC services is summarized next and is based on a set of informal telephone interviews with 13 POC providers.13 When inmates first enter prison, they are required to undergo a series of mental health screenings. The first screening is a general screening to determine whether inmates have even the slightest indication of a mental health problem. If it is determined that inmates may be at risk, they are then given a second screening that involves interviews with social workers, psychologists, and psychiatrists to determine whether they have a serious mental illness. If so, the inmates would begin participating in a prisoner mental health program. As the inmates approach parole, they are screened again to determine whether they still present a mental health problem and to determine the severity of that problem. If inmates are deemed to have a problem, they are then referred to a POC to seek condition-appropriate services. In addition to being referred prior to release, parolees may also be referred after release by their parole agent, which is called an “agent referral.”14 Once referred, a parolee can receive mental health services provided by a POC provider. POC providers include social workers, psychiatrists, and psychologists, and a parolee would see 13 14 Personal telephone communication with 13 CDCR POC doctors and social workers, January 14, 2009. Parolees may initiate the agent referral process by discussing with their agent that they are experiencing mental health problems and feel that they should seek services. Conceptual Framework and Methods for Defining the Health Care Safety Net for Parolees 69 one or more of the providers, depending on the severity of the mental health condition. Further, parolees would participate in both individual and group therapy sessions, depending on the classification of their condition. For example, a parolee with a severe mental health problem would be enrolled in the Expanded Outpatient Program (EOP), which entails both individual and group sessions on a weekly basis, and the parolee would see all three types of providers for services. A parolee with a less severe condition may participate, for example, in only the group sessions and see only a social worker for services on a more infrequent basis. Although POCs provide a basis of care, parolees are sometimes referred to other community programs for additional services. For example, parolees with severe mental health conditions are often referred to other county or community mental health programs because they may need treatment more often than once per week or they may need more extensive treatment than a POC can provide (e.g., if a parolee is dually diagnosed or has a development disability). Although our focus is on defining the mental health safety net available during the study period, it is likely that the current budget shortfalls in the state of California may have implications for the ability of parole agents to make referrals and for POCs to provide mental health services to parolees. So, in some instances, the mental health safety net for parolees may have declined since 2005–2006.15 Defining the Substance Abuse Safety Net The substance abuse treatment safety net generally includes outpatient services, residential alcohol- and drug-treatment services, detoxification services, and specialized programs targeting specific populations (e.g., the dually diagnosed) or treatment needs (e.g., methamphetamine use and addiction). In addition to county resources, other Alcohol and Drug Program (ADP) resources are available, including counseling, self-help, services available through faith-based organizations, and such organizations as Alcoholics Anonymous, which we do not address. For the uninsured and low-income population, we defined the substance abuse treatment safety net as follows. First, we relied on each county’s summary of its ADP and treatment providers. We also identified for each county the Proposition 36 treatment providers (described next). Where possible, we focused on residential, nonresidential, or outpatient providers; detoxification services; sober-living centers; and specialized programs, such as for methadone treatment. We excluded programs that were aimed primarily at education, prevention, or drug courts. We also excluded services specifically for juveniles, transition-aged youth, women, and the elderly. We defined the substance abuse safety net that is specific to the parolee population as follows. PSN has been in existence for about 15 years and is funded by the CDCR. PSN provides community alcohol and drug treatment and recovery services to parolees in 17 counties statewide (including the four counties we examine in depth). The PSN program provides up to 180 days of treatment and recovery services. It is funded by the CDCR with the goal of reducing recidivism and improving parole outcomes as evidenced by reduced alcohol and drug-related revocations (California Department of Alcohol and Drug Programs, 2009). Parolees are placed in PSN alcohol- and other drug-treatment and recovery programs, either from community parole systems or immediately upon release from prison custody. PSN’s focus is on counseling and recovery services; it does not include specialized programs, such as methadone treatment. 15 Personal telephone communication with 13 CDCR POC doctors and social workers, January 14, 2009. 70 Understanding the Health Implications of Prisoner Reentry in California, Phase I Report For example, in Los Angeles County, the PSN program16 is intended to provide parolees with a variety of alcohol- and drug-free living centers (ADFLCs), outpatient drug-free counseling (ODFC), and residential alcohol and drug treatment and recovery services. Los Angeles County contracts through the Department of Public Health’s Alcohol and Drug Program Administration with community-based agencies to provide ADFLCs, ODFC, and residential treatment services for female and male parolees. As of May 2008, the county had six programs that included six months of community-based ADFLC, ODFC, and residential treatment and recovery services to “civil commitments” (inmates in a specific correctional facility) released on parole from any CDCR institution into the county and to other parolees (Sugita, 2008). There are three major PSN networks in California: the Bay Area, Los Angeles, and San Diego. The following PSN county programs were implemented in fiscal year (FY) 1991–1992, and in November 2004, the treatment capacity was as follows: • Los Angeles County’s treatment capacity was approximately 36 residential beds, 72 nonresidential slots, and 12 sober-living environment (SLE) beds. • San Diego County’s treatment capacity was approximately 50 residential beds, 13 nonresidential slots, 14 SLE beds, and one detox bed (available 183 days per year). • Bay Area counties’ (Alameda, Contra Costa, Marin, San Francisco, San Mateo, Santa Clara, Solano, Napa, and Sonoma) treatment capacity was approximately 30 pretreatment orientation slots, 165 residential beds, 308 nonresidential slots, 15 SLE beds, and five detox beds. In addition to the three major PSN networks, the following PSN county programs were implemented in FY 1999–2000: Kern, Orange, Riverside, Sacramento, and San Bernardino counties. In November 2004, the total treatment capacity in these counties through the PSN program was approximately 52 residential beds, 124 nonresidential slots, 11 SLE beds, and one detox bed. Local assistance funding for PSN has remained about the same over the years. For instance, in FY 2008–2009, it was $10,706,567; in FY 2005–2006, it was $10,700,092 (California Department of Alcohol and Drug Programs, undated). California’s ADP allocates funds to the 17 participating counties through each county’s Negotiated Net Amount Contract. Table 4.1 summarizes the allocations in FYs 2005–2006 and 2007–2008 for the four counties that are the focus of this study. Table 4.1 also provides the unduplicated number of parolees served by the PSN program in FY 2005–2006; the numbers for FY 2007–2008 were unavailable (California Department of Alcohol and Drug Programs, undated). The PSN program is relatively small. In FY 2006–2007, the number of participants in Los Angeles County was 560, accounting for 643 admissions, with 56.5 percent of admissions being for outpatient counseling and 42.9 percent for residential services.17 During FY 2006– 2007, methamphetamine (37.0 percent) and cocaine/crack (28.4 percent) were the most frequently reported primary drug problems among PSN participants in Los Angeles County.18 16 In Los Angeles County, the PSN program is known as the Prison Parolee Network (PPN). 17 In addition, 0.5 percent of admissions were for day care, and 0.2 percent were for residential detoxification in FY 2006– 2007 (Los Angeles County Participant Reporting System data). 18 Alcohol (11.8 percent) and heroin (14.3 percent) were the primary drug programs for PSN program participants in Los Angeles County. Conceptual Framework and Methods for Defining the Health Care Safety Net for Parolees 71 Table 4.1 Parole Services Network Allocations, FYs 2005–2006 and 2007–2008 FY 2005–2006 FY 2007–2008 Allocation ($) Number Served (unduplicated) Allocation ($) Number Served (unduplicated) 1,265,116 291 1,265,116 Not available 325,907 148 325,907 Not available Los Angeles 1,578,440 375 1,584,915 Not available San Diego 1,500,000 296 1,500,000 Not available County Alameda Kern Sources: California Department of Alcohol and Drug Programs (2005–2006, 2009). The small numbers of parolees shown in Table 4.1 who participated in the PSN program and the stable level in funding for this program suggest that, although it is important, the PSN program does not address the treatment needs of a large number of parolees. In addition to the PSN program, parolees are eligible to participate in the Substance Abuse and Crime Prevention Act (SACPA, also known as Proposition 36), which was passed in 2001. The act increased state funding for drug treatment and allowed eligible nonviolent drug offenders to be diverted to drug treatment instead of receiving a traditional sentence. If individuals commit a violation while on parole that meets the criterion for Proposition 36, they are technically eligible to participate in this program. That is, in lieu of incarceration, state parolees who are convicted of nonviolent drug offenses or commit drug-related parole violations are eligible for drug treatment under this program. Under Proposition 36, depending on the drug violation, a parolee may be supervised by parole or dually supervised by parole and probation (Gardiner, 2008). Proposition 36 allows for dismissal of charges upon successful completion of treatment. Parolee participation in Proposition 36 is greater than for the PSN program. In FY 2005–2006, 1,684 parolees participated in Proposition 36 programs in Los Angeles County alone. Finally, there is another source of substance abuse treatment that is available to parolees. The Substance Abuse Coordinating Agencies (SASCA) coordinate networks of communitybased treatment providers to provide services for those released from prison. In 2006, 3.9 percent of those released from California prisons participated in the SASCA (see Walden House, undated). The CDCR contracts directly with providers throughout California. For those participating in prison treatment programs, case managers help with the transition to communitybased treatment.19 For example, in Los Angeles County, Walden House provides up to 180 days of treatment in the community plus 180 days follow-up. Currently, Walden House operates three residential programs in Los Angeles County for male parolees with the potential of serving up to 177 clients. We do not explicitly map the SASCA providers in our study counties because a number of the Proposition 36 and other alcohol- and drug-treatment providers participate in multiple programs, including the SASCA program. Thus, although we do not 19 Eligibility for SASCA includes individuals participating in and successfully completing one of California’s state prison substance abuse programs, state prison inmates who are eligible for drug-treatment furlough, and other parolees deemed eligible for SASCA services as determined by the CDCR. 72 Understanding the Health Implications of Prisoner Reentry in California, Phase I Report explicitly identify these service providers in our analyses, we believe that many of them will already be captured in our mapping of treatment facilities in Chapter Six. Developing a Measure of Accessibility for Mental Health and Substance Abuse Providers To calculate an accessibility measure for each type of provider,20 we computed the distance matrix from all census tracts within an area and then used it to select all population-weighted centroids within the threshold travel time (10 minutes drive time) of each health facility location. The ratio of supply to demand was calculated for each facility. In the case of mental health and substance abuse providers, we did not have a capacity measure. Therefore, we simply counted the facility as a single unit of supply. Thus, the ratio is interpreted as facilities per potential demand and represents the number of facilities per 10,000 population in a county. The next step used the distance matrix to determine for each population centroid the number of facilities within the threshold travel time of the tract centroid. The sum of the supply-todemand ratios of the selected facilities was the computed using accessibility in the census tract. For the alternate measures for mental health and substance abuse facilities, the accessibility bins or categories represent the number of facilities per 10,000 population within a 10-minute drive time of the population-weighted centroid of a census tract. The resulting accessibility for a census tract should be viewed as the estimated average accessibility measure for all persons within the tract, and it incorporates all demands made on a facility from the area surrounding that facility, not just the tract centroid–based buffer area of each tract. Tracts whose buffer areas (within the travel-time threshold) overlap with others within the catchment of the facility are factored in as well. In the resulting maps, we summarize the results by quartiles with the lowest quartile (shaded light green in the maps) representing the lowest level of accessibility and the highest quartile (shaded dark green) representing the highest level of accessibility. The quartiles for the accessibility measures are based on 25-percent intervals of the population demand versus supply in a specific county. The quartiles are equal-sized bins, and each bin has the same census tracts. The quartile cutoffs are based on distribution at the county level and are internal to a particular county, so they are not strictly comparable across counties. However, the accessibility values (low, medium-low, medium-high, and high, as indicated by different shades of green in the accessibility maps in Chapter Six) are comparable across counties. In mapping the mental health and substance abuse facilities, we show only what is available within a county’s border. However, we recognize that some concentrations of parolees near a county’s border may also be near facilities in neighboring counties. Therefore, our analysis of mental health and substance abuse safety-net facilities likely underestimate accessibility for some areas along the borders of our study counties. Discussion We tried to obtain data to characterize each of the three safety nets—health care, mental health care, and substance abuse treatment—for the period of interest to this study: 2005–2006. However, retrospective data were not always available, and so we sometimes had to incorpo20 Specifically, we wrote Microsoft Visual Basic® for Applications scripts to implement the 2FCSA method using ESRI’s ArcObjects™ application programming interface libraries. Conceptual Framework and Methods for Defining the Health Care Safety Net for Parolees 73 rate later data. For the hospital and clinic data, we used OSHPD data from 2005–2006. We augmented this information with a list of MISP providers (clinics and hospitals) for each study county. These are the current lists for the MISP facilities at the point at which we requested the information (i.e., 2008), so there may have been some changes since 2005. However, we felt that it was important to include these providers in our assessment even though we could not get retrospective data. For the mental health care safety net, we used data on county mental health clinics and MHSA providers (for the counties for which this information was available). The MHSA program came into existence in November 2004, with counties varying in terms of how early they put these resources into place. So, we likely are representing the mental health safety net at the time of our study period as well as more recent changes. For the POCs, we believe that our estimates are relatively stable over time because these clinics are colocated with parole offices in California. For the substance abuse treatment safety net, we used data for county alcohol and drug treatment services and for Proposition 36 services. Proposition 36 was passed in 2001 and so covers the study time period. This program also has evolved over time. We use the current data on Proposition 36 providers in the study counties, the picture of which likely differs somewhat from that in 2005–2006. Overall, however, we believe that it presents a reasonable picture of the distribution of providers during our study period because the funding was similar between these two years. Finally, the PSN program has been in existence for a number of years, with the funding remaining level over time. We believe that our estimates for the PSN program likely reflect the network in 2005–2006 as well, given the similarity in funding levels shown in Table 4.1. For our geographic analyses at the subcounty level in Chapters Five and Six, we focus on the availability and accessibility of safety-net health care resources for the reentry population. These resources include safety net hospitals, PCCs, and mental health and substance abuse treatment providers. Because most of these facilities serve more than just the reentry population, our accessibility analyses presented in Chapters Five and Six take into account the potential demand for such services by considering the percentage of the population living in poverty. Further, because capacity likely varies across these safety-net providers, we incorporate data on facility capacity (when available) in our measure of accessibility. Chapter Five Counties’ Capacity to Meet the Health Care Needs of the Reentry Population In Chapter Two, we showed that California prison inmates bear a high burden of chronic diseases, such as hypertension and asthma, and infectious diseases, such as hepatitis and TB. These conditions require regular access to health care to manage them effectively. Yet, among those who reported a current medical issue, a substantial share of inmates did not receive care while incarcerated, which suggests that there may be a number of unmet health needs that parolees will bring with them when they return to communities. In addition, we are dealing with a population that, as noted earlier, tends to be sicker on average than the general population (NCCHC, 2002). Given these health care needs, what levels of accessibility to health care resources do parolees have in the four counties? This chapter provides an in-depth examination of the four counties’ health care safety nets and their ability to meet the health care needs of the reentry population. More specifically, in this chapter, we present our mapping and accessibility results and a discussion of their implications. Because this chapter focuses on resources to treat the physical health care needs of parolees, it maps such resources as general acute care hospitals and primary care clinics (PCCs) that serve uninsured and low-income populations. Those resources are plotted onto the maps previously presented in Chapter Three to show parolee concentrations and clusters in the four counties selected for in-depth study. We consider the substance abuse and mental health resources to address social and behavioral conditions in Chapter Six. To maximize the maps’ utility for policymakers, we include supervisorial district boundaries. The supervisorial districts represent the political districts within a county. For Los Angeles County only, we also show the eight SPAs. The SPA designation is used for health planning purposes, with each SPA having a health office responsible for planning public health and clinical services in a given area. Using both supervisorial districts and SPA boundaries provides additional context from a policy- and health-planning perspective to help policymakers understand where the health care impact of the reentry population may be greatest. We have organized this chapter by presenting the mapping and accessibility results for each of the four counties followed by an overarching summary of the findings across counties. Those interested in the results for a particular county can proceed directly to the section of this chapter that presents the findings for that county. Those interested in the overall summary of the findings can proceed to the discussion section at the end of this chapter. For each of the four counties, we first examined the geographic distribution of facilities comprised by the health care safety net (specifically, PCCs and general acute care hospitals) and compared them to the distribution of parolee concentrations in each county. We also generated measures of the accessibility of these facilities to the parolee population by using facilities’ capacity and a proxy for the underlying population demand for their services. (For more 75 76 Understanding the Health Implications of Prisoner Reentry in California, Phase I Report detail on the underlying methodology used to create the accessibility measures, see Chapter Four.) To assess the effect of missing capacity data, we also conducted a sensitivity analysis to examine whether accessibility patterns in a county changed when using a simple count of facilities rather than capacity in calculating these measures. The accessibility measure allocates census tracts into quartiles, with the four quartiles representing differing levels of accessibility to health care facilities. We then tabulated the results in terms of how parolees are distributed across these quartiles of census tracts. Also, for mapping purposes, duplicate listings (that is, cases that represented actual duplicates or in which more than one type of service or program was being provided in a given location)1 are shown as a single geographic point on the maps. However, our accessibility measures take into account instances in which it appears that more than one type of service or program is being provided at a single location. The reason for doing this was to ensure that the measure of accessibility reflected as accurately as possible the availability of all relevant facilities in a given county. Next, we summarize our findings for each county. Alameda County For Alameda County’s health care safety net, we focused on both PCCs and general acute care hospitals. We included MISP clinics, which are contracted to provide care to the medically indigent population, as well as other types of PCCs. In Alameda County, both the MISP clinics and the other PCCs follow the corridor of parolee clusters along the western urban areas of the county (Figure 5.1). But only the MISP clinics serve the inland areas in District 1 and are relatively near the concentration of parolees in this area. Around Hayward, there are two concentrations of parolees who do not appear to be served by any of the clinics listed, shown as shaded areas that look like butterfly wings on either side of Hayward. Figure 5.2 shows our measure of accessibility by census tract for the MISP clinics and for all primary care clinics in Alameda County. The areas shaded in dark green represent those areas with the highest level of accessibility in Alameda County; areas in light green fall into the lowest accessibility category. Comparing the accessibility map in Figure 5.2 to the concentration of parolees shown in Figure 5.1, we see that parolee concentrations located in the northern part of Alameda County are in areas with higher levels of accessibility to PCCs (shaded in darker green on the map) compared to parolee concentrations around San Leandro and Hayward. In Alameda County, 10 of the 21 MISP clinics and nine of the 21 other PCCs were missing capacity data (total FTEs); thus, they were excluded from our accessibility measure. The distribution of missing clinics follows the general pattern of clinics in the county. Given the amount of missing data, we conducted a sensitivity analysis to see whether the overall pattern of accessibility categories would change if we calculated accessibility simply using the counts of facilities (rather than their FTEs). For the MISP clinics, we found few differences in the pattern of the accessibility categories, with the exception of the eastern half of District 1, 1 For example, a mental health provider has an outpatient counseling program and a residential treatment program in the same location, or two different mental health providers occupy the same physical location. Counties’ Capacity to Meet the Health Care Needs of the Reentry Population 77 Figure 5.1 Map of Primary Care Clinics, by Supervisorial District, Alameda County where the accessibility category was shaded darkest green (compared to the next shade lighter) when using simple counts of MISP clinics. For the map of all clinics combined, the areas identified as having the lowest levels of accessibility remained the same. However, we did find that the areas around Fremont and Union City had somewhat higher accessibility, as did the area around Livermore. For the alternate accessibility measure, see Appendix E. Table 5.1 compares accessibility of parolees in Alameda County to MISP and all PCCs by race/ethnicity. The quartiles of accessibility categories shown in each panel are defined based on the distribution of census tracts. The table then shows the share of parolees (overall and by race/ethnicity) who fall into each of these census tract quartiles. The way to interpret the quartiles is as follows: For the MISP clinics, Alameda’s census tracts in the mid-low quartile have, at most, 0.4 FTEs within a 10-minute drive per 1,000 individuals under the FPL.2 In the highest quartile for the MISP clinics, these census tracts have at least 1.5 times as many FTEs (0.6). For all clinics, Alameda’s census tracts in the lowest quartile have at most 0.22 FTEs within a 10-minute drive time per 1,000 individuals under the FPL. In the highest quartile, census tracts have at least six times as many FTEs (1.4). 2 Based on the population-weighted centroid of each census tract. 78 Understanding the Health Implications of Prisoner Reentry in California, Phase I Report Figure 5.2 Map of Primary Care Clinic Accessibility Measure, Alameda County Table 5.1 Distribution of Parolees Across Clinic Accessibility, by Quartile and Race/Ethnicity, Alameda County (percent) Total (n = 4,689) African-American (n = 2,904) Latino (n = 776) White (n = 775) Lowest (0.0–0.22) 20.0 13.1 26.9 37.0 Mid-low (0.23–0.85) 14.7 11.1 21.0 22.1 Mid-high (0.86–1.39) 26.7 31.0 22.7 15.4 Highest (1.40–2.00) 38.6 44.8 29.4 25.5 Lowest (0.0) 21.8 12.6 33.5 41.5 Mid-low (0.1–0.4) 24.4 29.8 17.3 15.5 Mid-high (0.50) 15.8 13.9 20.7 17.0 Highest (0.60–2.00) 37.9 43.7 28.5 25.9 Level of Accessibility (quartile) All clinics MISP clinics Note: The “other” race/ethnicity group (n = 234) is not shown. For the lowest quartile, 0.0 indicates that 25 percent of census tracts in Alameda County do not have any FTEs within a 10-minute drive time. Counties’ Capacity to Meet the Health Care Needs of the Reentry Population 79 Overall, about half of parolees reside in areas with higher levels of accessibility to MISP clinics (as measured by the percentage of parolees who fall into the two highest accessibility categories in Table 5.1). If we include all PCCs, a greater share (about two-thirds) of parolees reside in areas with the two highest levels of accessibility, suggesting that, in general, accessibility to PCCs is higher for a significant proportion of parolees. But we see important differences in accessibility by race/ethnicity. Fifty-eight percent of African-Americans reside in areas with the two highest levels of MISP clinic accessibility, and when we add in the other PCCs, this proportion increases to 76 percent. In comparison, only 52 percent of Latino parolees reside in these areas. Similarly, for white parolees, only 41 percent reside in areas with the two highest levels of MISP clinic accessibility, and this percentage stays the same even when we add in the other PCCs. At least in terms of accessibility to MISP clinics and PCCs, AfricanAmerican parolees in Alameda County appear to fare better than Latino or white parolees. Also, other PCCs appear to be important in filling in geographic gaps in coverage for the parolee population. The second type of facility that we considered were nonprofit and government/public general acute care hospitals. In Alameda County, there are two MISP hospitals (Alameda County Medical Center–Highland General Hospital in Oakland and Alameda County Medical Center–Fairmont Hospital in San Leandro) and two public hospitals (Washington Hospital in Fremont, and Alameda Hospital in Alameda). Three of these four MISP and public hospitals have emergency departments. The distribution of hospitals follows a pattern that is similar to what we saw for the clinics—that is, they are distributed along the corridor of the concentrations of parolees along the western urban areas (Figure 5.3). The northern part of Alameda County appears to have a greater number of these hospitals, and as for the map of clinics, there are two areas around Hayward with concentrations of parolees in the shape of butterfly wings that appear to be poorly served. The other nonprofit general acute care hospitals3 similarly follow this corridor but, importantly, also help fill geographic gaps in coverage vis-à-vis the concentration of parolees, particularly in Districts 1 and 2 and in the northernmost part of the county in District 5. Figure 5.4 shows our measure of accessibility by census tract for nonprofit and government/public general acute care hospitals in Alameda County. In this county, five of the 15 hospitals were missing capacity data (as measured by total FTEs) and so are not included in the accessibility measure. The hospitals with missing data included three hospitals (including one MISP hospital) near San Leandro, one hospital near Berkeley (District 5), and one hospital near Fremont and one near Dublin, both in District 1. Therefore, the accessibility measure for these areas is less reliable. Comparing the accessibility map to the concentration of parolees shown in Figure 5.4, we see that the area with the highest concentration of parolees in District 3 falls into the lowest accessibility category (shaded in light green on the map). The areas around the cities of Alameda and Oakland fall into the two lowest accessibility categories after taking into account the underlying demand for hospital services in these areas. We examined the sensitivity of our results to the missing data by recalculating our accessibility measures using the counts of facilities rather than FTEs. For hospitals, we found a similar pattern in the accessibility categories described earlier, with a few exceptions. The area 3 Eight of the eleven other hospitals also have emergency departments. 80 Understanding the Health Implications of Prisoner Reentry in California, Phase I Report Figure 5.3 Map of General Acute Care Hospitals, by Supervisorial District, Alameda County around Dublin increased in accessibility from the lowest category to the highest category. The area west of Berkeley in District 5 increased a level to the highest accessibility category, and the area southeast of Berkeley decreased by one level. For the alternate accessibility measure, see Appendix E. Table 5.2 summarizes the accessibility of parolees to hospitals in Alameda County. For hospitals, Alameda’s census tracts in the lowest quartile have, at most, 40.8 FTEs within a 10-minute drive per 1,000 individuals under the FPL.4 In the highest quartile, census tracts have at least 2.4 times as many FTEs (97.7). Overall, about two-thirds of parolees reside in areas with the two lowest levels of accessibility (Table 5.2), but the distributions of parolees vary substantially across racial/ethnic groups. Nearly three-quarters (72.5 percent) of African-American parolees reside in areas with the two lowest levels of accessibility to hospitals, compared to nearly half of Latino (47 percent) and white (48 percent) parolees. In summary, African-American parolees in Alameda County tend to live in areas with higher levels of accessibility to safety-net clinics than do Latino and white parolees; however, the opposite is true with respect to safety-net hospitals. 4 Based on the population-weighted centroid of each census tract. Counties’ Capacity to Meet the Health Care Needs of the Reentry Population 81 Figure 5.4 Map of General Acute Care Hospital Accessibility Measure, Alameda County Table 5.2 Distribution of Parolees Across Hospital Accessibility, by Quartile and Race/Ethnicity, Alameda County (percent) Total (n = 4,689) African-American (n = 2,904) Latino (n = 776) White (n = 775) Lowest (0.0–40.8) 28.2 33.3 20.6 19.6 Mid-low (40.9–90.0) 35.2 39.2 26.8 28.4 Mid-high (90.1–97.6) 23.5 20.2 30.8 26.6 Highest (97.7–243.1) 13.1 7.3 21.8 25.4 Level of Accessibility (quartile) Note: The “other” race/ethnicity group (n = 234) is not shown. Kern County In Kern County, the clustering of parolees in the two supervisorial districts in the center of the county (Districts 3 and 5 around the city of Bakersfield) correlates with the densest availability of PCC resources (Figure 5.5). District 1 in the northeast part of the county also has a concentration of parolees that correlates well with the availability of PCCs in this area. However, 82 Understanding the Health Implications of Prisoner Reentry in California, Phase I Report Figure 5.5 Map of Clinics, by Supervisorial District, Kern County given District 1’s large geographic size, driving distance may influence actual accessibility in these less densely populated areas (see Figure 5.6). The southern part of Kern County (near Rosamond) and the county’s border with Los Angeles County (near Lancaster) also have a high concentration of parolees; however, there appear to be no clinic resources in this part of the county (Figure 5.5). As we discuss later in this chapter, we know that there are two MISP clinics near Lancaster. The concentration of parolees near Rosamond is also near Kern County’s southern border; however, we do not show facilities in neighboring counties. We acknowledge that our maps will likely underestimate the accessibility to clinics for some areas along the county’s borders as a result. This example illustrates how taking into account resources in surrounding counties (in this case, the Lancaster area of Los Angeles County) would provide a more complete picture of degree of accessibility for parolees in Kern County. However, in calculating the accessibility of hospitals, discussed later, we were able to take into account facilities in neighboring counties. Figure 5.6 shows our measure of accessibility for clinics in Kern County and takes into account driving distance. In rural counties with a lower population density, driving time is expected to be a more important factor in determining the level of accessibility. Fourteen of the 15 MISP clinics and two of the 19 other PCCs were missing capacity data; thus, we were unable to include them in our measure of accessibility. Because most of the MISP clinics in Counties’ Capacity to Meet the Health Care Needs of the Reentry Population 83 Figure 5.6 Map of Primary Care Clinic Accessibility Measure, Kern County Kern County were missing capacity data, we were unable to calculate an accessibility measure separately for them. Thus, the accessibility measure for this county primarily represents accessibility to other PCCs (excluding all but one MISP clinic). Concentrations of parolees around the cities of Bakersfield and Arvin in the center of the county fall into the two highest categories of accessibility. The concentrations of parolees located in District 1 also fall into the two highest accessibility categories. Not surprisingly, concentrations of parolees in District 2 fall into the lowest accessibility category (shaded light green on the map), although as noted earlier, this area’s accessibility is likely underestimated because it does not take into account the clinic resources in neighboring Los Angeles County (Lancaster). Missing data for MISP clinics was a concern; however, most of the primary care clinics had FTE data. Therefore, the accessibility map focuses on the other PCCs in the county. We conducted a sensitivity analysis using a simple count of clinics (with no capacity measure) to measure accessibility and found very minor differences in the accessibility pattern throughout the county. Accessibility increased by a level around the areas of Oildale and Ridgecrest when we used the simple count of clinics. Other than these differences, the overall pattern remained the same. For the alternate accessibility measure, see Appendix E. Table 5.3 summarizes the accessibility of parolees to clinics in Kern County. For hospitals, census tracts in the mid-low quartile have, at most, 0.40 FTEs within a 10-minute drive 84 Understanding the Health Implications of Prisoner Reentry in California, Phase I Report Table 5.3 Distribution of Parolees Across Clinic Accessibility, by Quartile and Race/Ethnicity, Kern County (percent) Total (n = 5,936) African-American (n = 1,105) Latino (n = 2,247) White (n = 2,478) Lowest (0.00) 17.6 11.9 15.9 21.7 Mid-low (0.01–0.40) 12.0 11.7 8.6 15.1 Mid-high (0.41–0.76) 37.4 32.7 40.9 36.3 Highest (0.77–3.37) 33.0 43.7 34.5 26.9 Level of Accessibility (quartile) Note: The “other” race/ethnicity group (n = 106) is not shown. For the lowest quartile, 0.0 indicates that 25 percent of census tracts in Kern County do not have any FTEs within a 10-minute drive time. per 1,000 individuals under the FPL.5 In the highest quartile, census tracts have almost twice as many FTEs (0.77). Overall, 70 percent of parolees reside in areas with the two highest levels of accessibility to clinics (Table 5.3). The only difference in accessibility by race/ethnicity is that fewer white parolees (63 percent) reside in areas with the two highest categories of accessibility, compared to approximately 75 percent of African-American and Latino parolees. In terms of nonprofit and government/public general acute care hospitals, we see a similar pattern as for clinics. There is only one MISP hospital in Kern County (Kern County Medical Center in Bakersfield) and two other public hospitals (Tehachapi Hospital and Kern Valley Healthcare District, located in Districts 1 and 2) (Figure 5.7). All three hospitals have emergency departments. Interestingly, the MISP and public hospitals appear to be fairly well dispersed. When we include the seven other nonprofit general acute care hospitals in the county, 6 we see that they are (not surprisingly) concentrated almost exclusively in the center of the county, where the population density is greatest. Although the concentration of parolees in the lower part of District 2 around the town of Rosamond is some distance from a county hospital, the parolees still are relatively near a facility (Figure 5.7). Moreover, this concentration of parolees is near the border between Kern and Los Angeles counties and is, thus, closer to hospitals in the towns of Lancaster and Palmdale in Los Angeles County than to the hospital in Tehachapi in Kern County. Figure 5.8 shows our measure of accessibility for nonprofit and government/public general acute care hospitals in Kern County by census tract. In this county, two hospitals near Bakersfield (out of 10 hospitals total) were missing capacity data (as measured by total FTEs) and so are not included in our accessibility measure. Comparing the accessibility map in Figure 5.8 to the concentration of parolees shown in Figure 5.7, we see that the concentrations of parolees around the city of Bakersfield in the center of the map, in the northeast part of District 1 around the city of Ridgecrest, and around Tehachapi (District 2) reside in areas with the highest level of accessibility to hospitals (shaded in dark green on the map). The concentration of parolees around the towns of Delano, Wasco, and Shafter (western edge of District 1) have somewhat lower levels of accessibility. Finally, the concentration of parolees in District 2 falls into the lowest accessibility category (with the 5 Based on the population-weighted centroid of each census tract. 6 Six of the seven nonprofit hospitals in this category also have emergency departments. Counties’ Capacity to Meet the Health Care Needs of the Reentry Population 85 Figure 5.7 Map of General Acute Care Hospitals, by Supervisorial District, Kern County exception of the area immediately around the city of Tehachapi, which is the next level up in terms of accessibility). Kern County is a rural county, so it is not surprising to see that hospital accessibility is highest near its major city and towns. A sensitivity analysis using a simple count of hospitals to measure accessibility showed very little difference in the overall pattern of accessibility described here, except for the area around Bakersfield. Using simple counts of hospitals resulted in the census tracts to the north of Bakersfield and the area immediately east of the city to decrease by one accessibility level because we included the two hospitals missing capacity data in the accessibility measure. For the alternate accessibility measure, see Appendix E. Table 5.4 summarizes the accessibility of parolees to hospitals in Kern County. For hospitals, census tracts in the mid-low quartile have, at most, 39.2 FTEs within a 10-minute drive per 1,000 individuals under the FPL.7 In the highest quartile, census tracts have at least twice as many FTEs (89.8). In 2006, this agricultural county had a large Latino population (45 percent), and AfricanAmericans accounted for only 6.3 percent of the population (U.S. Census Bureau, 2009b). Table 5.4 compares accessibility of parolees in Kern County by ethnicity. Overall, 37 percent of parolees fall in to the two lowest accessibility categories (light green and the next 7 Based on the population-weighted centroid of each census tract. 86 Understanding the Health Implications of Prisoner Reentry in California, Phase I Report shade lighter). There are some differences between Latino and white parolees versus AfricanAmerican parolees in terms of accessibility to hospitals. Nearly 40 percent of Latino parolees and 37 percent of white parolees fall into the two lowest accessibility categories, compared to only 31 percent of African-American parolees. In summary, it does not appear that there is a trade-off between access to clinics and hospitals. African-American parolees in Kern County appear to have better access to both clinics and hospitals relative to their white counterparts. Figure 5.8 Map of General Acute Care Hospital Accessibility Measure, Kern County Table 5.4 Distribution of Parolees Across Hospital Accessibility, by Quartile and Race/Ethnicity, Kern County (percent) Total (n = 5,936) African-American (n = 1,105) Latino (n = 2,247) White (n = 2,478) Lowest (0.00) 24.1 18.5 24.1 26.4 Mid-low (0.01–39.2) 13.0 12.3 15.7 10.7 Mid-high (39.3–89.7) 24.9 21.0 25.7 26.3 Highest (89.8–103.3) 38.0 48.2 34.5 36.6 Level of Accessibility (quartile) Note: The “other” race/ethnicity group (n = 106) is not shown. For the lowest quartile, 0.0 indicates that 25 percent of census tracts in Kern County do not have any FTEs within a 10-minute drive time. Counties’ Capacity to Meet the Health Care Needs of the Reentry Population 87 Los Angeles County For the health care safety net in Los Angeles County, we again focused on both PCCs and general acute care hospitals. But in this case, we included MISP clinics, which are contracted to provide care to the medically indigent population; county primary care and comprehensive health services/multiservice ambulatory care clinics; and PPP community clinics that are contracted by the county to provide services to the medically indigent population—we refer to these clinics as MISP (PPPs). We included the MISP (PPP) clinics in our analysis because we hypothesized that they may help fill a number of potential geographic gaps in the safety net. Another difference is that we show the clinic locations with the geographical boundaries of both supervisorial districts (Figure 5.9) and SPAs (Figure 5.10). In terms of PCCs, the northern half of Los Angeles County is less densely covered compared to the southern half of the county (Figures 5.9 and 5.10). In general, MISP facilities appear to be colocated near the highest concentration of parolees; however, these clinics are relatively few in number. This pattern could simply reflect the fact that these areas have the highest population density in the county. PCCs and MISP (PPP) clinics play an important role in filling in geographical gaps in services not covered by the MISP clinics. Yet, some gaps in coverage remain, particularly in District 2 and SPA 6. Figure 5.11 shows our measure of accessibility for MISP (PPP) clinics and other PCCs in Los Angeles County. A significant number of MISP clinics were missing capacity information, so we were unable to include them in our accessibility measure.8 However, 59 of the 80 MISP (PPP) clinics and 51 out of 71 other PCCs had capacity data, so we calculated the accessibility measure for this larger group of clinics. Comparing the accessibility map for MISP (PPP) clinics (Figure 5.11) to the map showing the concentrations of parolees in Los Angeles County (Figure 5.10), we see that accessibility of PCCs is highest in the areas around Venice and Marina Del Rey (SPA 5), the downtown Los Angeles and Glendale areas (SPA 4), San Fernando Valley (SPA 2), and near El Monte and Pomona (SPA 3). However, the largest concentrations of parolees in the areas of Compton and South Central Los Angeles (SPA 6), Long Beach and Carson (SPA 8), and SPA 7 reside in areas with somewhat lower levels of accessibility to PCCs (i.e., as shown by the two lighter shades of green in Figure 5.11). This is also the case for the concentrations of parolees in the Lancaster/Palmdale area (SPA 1). We examined the sensitivity of our results to the missing data for PCCs by recalculating our accessibility measures using the counts of facilities rather than FTEs. We found two significant differences in the accessibility pattern for Los Angeles County. The area around Lancaster/Palmdale (SPA 1) dropped to the lowest level of accessibility, and accessibility in the area around Gardena and Compton (SPA 6) dropped by a level when we did not take clinics’ capacity into account. However, for the remainder of the county, the pattern of accessibility remained the same. For the alternate accessibility measure, see Appendix E. Table 5.5 summarizes the accessibility of parolees to MISP clinics contracted to serve the medically indigent and then to all clinics in Los Angeles County. For the MISP clinics, census tracts in the lowest quartile have, at most, 0.03 FTEs within a 10-minute drive per 1,000 individuals under the FPL.9 8 Of the 21 MISP clinics, we had capacity data for only one. 9 Based on the population-weighted centroid of each census tract. 88 Understanding the Health Implications of Prisoner Reentry in California, Phase I Report Figure 5.9 Map of Primary Care Clinics, by Supervisorial District, Los Angeles County Counties’ Capacity to Meet the Health Care Needs of the Reentry Population Figure 5.10 Map of Primary Care Clinics, by Service Planning Area, Los Angeles County 89 90 Understanding the Health Implications of Prisoner Reentry in California, Phase I Report Figure 5.11 Map of Primary Care Clinic Accessibility Measure, Los Angeles County In the highest quartile, census tracts have at least nine times as many FTEs (0.27). We see a similar result when we look at all PCCs. For all clinics, census tracts in the lowest quartile have, at most, 0.04 FTEs within a 10-minute drive per 1,000 individuals under the FPL.10 In the highest quartile, census tracts have at least nine times as many FTEs (0.37). Table 5.5 compares the accessibility of the MISP clinics and to all PCCs in Los Angeles County by race/ethnicity. Overall, about half of parolees reside in areas with the two highest levels of accessibility to MISP clinics. If we include the other PCCs in the county, the share of parolees in the two most accessible quartiles remains roughly the same. In general, AfricanAmerican and Latino parolees tend to reside in areas with somewhat higher levels of accessibility to clinics than do white parolees in Los Angeles County. About half of African-American and Latino parolees reside in areas with the two highest levels of accessibility to MISP clinics, compared to 45 percent of white parolees. When we add in the other PCCs, the pattern remains roughly the same. 10 Based on the population-weighted centroid of each census tract. Counties’ Capacity to Meet the Health Care Needs of the Reentry Population 91 Table 5.5 Distribution of Parolees Across Clinic Accessibility Categories, by Quartile and Race/Ethnicity, Los Angeles County (percent) Total (n = 35,710) African-American (n = 12,885) Latino (n = 14,921) White (n = 6,671) Lowest (0.00–0.04) 22.7 19.6 20.8 32.3 Mid-low (0.05–0.21) 25.4 24.7 25.9 25.1 Mid-high (0.22–0.36) 23.8 20.8 27.3 22.1 Highest (0.37–16.88) 28.1 34.9 26.0 20.5 Lowest (0.00–0.03) 20.3 14.5 20.3 31.1 Mid-low (0.04–0.17) 30.4 35.7 28.5 24.4 Mid-high (0.18–0.26) 27.8 33.5 26.7 20.5 Highest (0.27–0.91) 21.5 16.3 24.5 24.0 Level of Accessibility (quartile) All clinics MISP clinics Note: The “other” race/ethnicity group (n = 1,233) is not shown. We examined the sensitivity of our results to the missing capacity data by recalculating our accessibility measures using the counts of clinics rather than FTEs. We found three areas where the pattern of accessibility differed significantly when we used simple counts of clinics in our measure. Using simple counts of clinics, accessibility around Lancaster and Palmdale (District 5) increased to the highest level, since we were now taking into account those clinics that were missing capacity data. Accessibility in areas around Hawthorne, Gardena, and Compton (District 2, SPA 8) also increased to the highest level. Finally, accessibility in the area around Long Beach increased to the highest level. For the remainder of the county, the pattern of accessibility remained the same. In this instance, missing capacity data had an important effect on the pattern of clinic accessibility for key parts of the county and especially for areas with high concentrations of parolees. For the alternate accessibility measure, see Appendix E. In terms of nonprofit and government/public general acute care hospitals, there are very few MISP or county hospitals in Los Angeles County. And with the closure of Martin Luther King/Charles R. Drew Medical Center’s inpatient capacity, SPA 6 (District 2, not shown) no longer has an MISP/county hospital. (Only the SPA boundaries are shown in Figure 5.12.) Still, we include King-Drew here because of its presence in 2005. However, the facility’s subsequent closure means that availability in SPA 6 has deteriorated substantially, so we identify King-Drew’s location separately in the maps. The other three MISP/county hospitals are Harbor/University of California, Los Angeles (UCLA) Medical Center in Torrance, Los Angeles County/University of Southern California (USC) Medical Center in Los Angeles, and Olive View/UCLA Medical Center in Sylmar.11 All six government/public hospitals had emergency departments during the period covered by our analysis. Looking at the distribution of nonprofit and government/public general acute care hospitals, we see that hospital resources, 11 The other MISP hospital in Los Angeles County is Rancho Los Amigos National Rehabilitation Center in Downey. For this study, we did not include rehabilitation hospitals. 92 Understanding the Health Implications of Prisoner Reentry in California, Phase I Report Figure 5.12 Map of General Acute Care Hospitals, by Service Planning Area, Los Angeles County Counties’ Capacity to Meet the Health Care Needs of the Reentry Population 93 in general, are not as common in the less urbanized areas of Los Angeles County (e.g., SPA 1 [District 5, not shown]) (Figure 5.12). There is some overlap between these underserved areas and our parolee concentrations. King-Drew is an example of an area where services existed but have since become less available. SPA 1 (District 5, not shown) also presents some concerns. Figure 5.12 also illustrates how other nonprofit hospitals (43 total) play a role in filling in geographic gaps in coverage, with 40 of these other hospitals also having emergency departments. Figure 5.13 shows our measure of accessibility for nonprofit and government/public general acute care hospitals in Los Angeles County by census tract and by SPA. In Los Angeles, three hospitals near Carson (SPA 8 [District 2, not shown]), Glendale (SPA 4 [District 1, not shown]), and Covina (SPA 3 [District 1, not shown]) were missing capacity data (as measured by total FTEs) and so are not included in our accessibility measure. None of these is an MISP hospital. The hospital accessibility measures also show cause for concern in that there are areas with significant concentrations of parolees with relatively poor availability. Comparing the accessibility map in Figure 5.13 to the concentration of parolees shown in Figure 5.12, we see that the concentrations of parolees in SPA 6 and parts of SPA 7 fall into the lowest levels of Figure 5.13 Map of General Acute Care Hospital Accessibility Measure, Los Angeles County 94 Understanding the Health Implications of Prisoner Reentry in California, Phase I Report accessibility (shown in light green on the map). Thus, taking into account capacity and underlying demand for hospital resources, the hospital accessibility of parolees in these areas looks even worse than when we simply look at the geographic distribution of hospitals. We examined the sensitivity of our results to the missing data for hospitals by recalculating our accessibility measures using the counts of facilities rather than FTEs. The pattern of the accessibility changed in those areas where hospitals were missing capacity data. Specifically, the level of accessibility increased to the highest level around Carson (District 2, SPA 8) and around Covina (District 1, SPA 3) when we included the area hospitals that were missing capacity data. In addition, accessibility in the area around the city of Los Angeles and some of the census tracts north of Long Beach increased to the highest level. Similar to our findings for clinics, our sensitivity analysis for Los Angeles County shows that our measure of accessibility is sensitive to the degree of missing capacity data for hospitals. For the alternate accessibility measure, see Appendix E. Table 5.6 summarizes the accessibility of parolees to hospitals in Los Angeles County. Census tracts in the lowest quartile have, at most, 15.9 FTEs within a 10-minute drive per 1,000 individuals under the FPL.12 In the highest quartile, census tracts have at least four times as many FTEs (67.8). Overall, half of parolees reside in areas with the two lowest levels of accessibility (Table 5.6). We see important differences between African-American parolees versus Latino and white parolees in terms of their accessibility to hospitals. Nearly 60 percent of African-American parolees reside in areas with the two lowest levels of accessibility, compared to 51 percent of Latino parolees and 47 percent of white parolees. In summary, the results for Los Angeles County show that African-American and Latino parolees tend to reside in areas with somewhat higher levels of accessibility to safety-net clinics than do white parolees. However, there is a different story with respect to safety-net hospitals. A larger share of African-American parolees have relatively lower levels of access to safety-net hospitals compared to Latino and white parolees. Table 5.6 Distribution of Parolees Across Hospital Accessibility Categories, by Quartile and Race/Ethnicity, Los Angeles County (percent) Total (n = 35,710) African-American (n = 12,885) Latino (n = 14,921) White (n = 6,671) Lowest (0.0–15.9) 24.2 28.8 21.0 23.6 Mid-low (16.0–38.6) 28.8 30.7 29.9 23.4 Mid-high (38.7–67.7) 29.6 25.0 32.6 30.4 Highest (67.8–236.7) 17.4 15.5 16.4 22.7 Level of Accessibility (quartile) Note: The “other” race/ethnicity group (n = 1,233) is not shown. 12 Based on the population-weighted centroid of each census tract. Counties’ Capacity to Meet the Health Care Needs of the Reentry Population 95 San Diego County Unlike the other counties considered in this report, San Diego County does not operate public hospitals or public clinics. Therefore, private, nonprofit community clinics and health centers make up the safety net for primary care services for San Diego’s low-income and uninsured (Council of Community Clinics, undated). In San Diego County, the MISP clinics are concentrated in the urban center of the county, which has some of the highest concentrations of parolees, and also in the northern part of the county near Oceanside and Escondido (Figure 5.14). The concentration of parolees in District 2 near the Mexican border is served by several MISP clinics. Adding in the other PCCs in San Diego County, it appears that the mapping of clinics to concentrations of parolees is quite good for both the MISP clinics and other PCCs. Unlike in the other counties, there do not appear to be large geographic gaps in coverage vis-à-vis areas with concentrations of parolees. Figure 5.15 shows our measures of accessibility for both MISP and other PCCs in San Diego County. Four of the 24 MISP clinics and four of the 31 other PCCs were missing capacity data; thus, they could not be included in our primary measure of accessibility. Comparing Figure 5.14 Map of Primary Care Clinics, by Supervisorial District, San Diego County 96 Understanding the Health Implications of Prisoner Reentry in California, Phase I Report Figure 5.15 Map of Primary Care Clinic Accessibility Measure, San Diego County the accessibility map (Figure 5.15) to the concentrations of parolees in San Diego County (see Figure 5.14), we see that the level of accessibility to clinics is relatively high in the areas around Oceanside, Vista, and Escondido (District 5) and San Diego, National City, Imperial Beach, and Alpine (Districts 1, 2, and 4). Also, the area north of Lakeside and the eastern half of District 2 have high levels of accessibility. Parolee concentrations around Lakeside, El Cajon, and Chula have somewhat lower levels of accessibility (as evidenced by lighter shades of green), as do parolees residing in District 3. We examined the sensitivity of our results to the missing capacity data for clinics by recalculating our accessibility measures using the counts of facilities rather than FTEs. In this instance, our sensitivity analysis showed that our measure of accessibility is sensitive to the degree of missing capacity data for these types of facilities. We see an increase in the level of accessibility around Oceanside (District 5); many of the areas in District 3; the areas around El Cajon, La Mesa, and downtown San Diego; and Imperial Beach. However, accessibility decreased by one level for the area around Alpine. For the alternate accessibility measure, see Appendix E. Table 5.7 summarizes the accessibility of parolees to MISP clinics, contracted to serve the medically indigent, and then to all clinics in San Diego County. For the MISP clinics, census tracts in the mid-low quartile have, at most, 0.33 FTEs within a 10-minute drive per 1,000 Counties’ Capacity to Meet the Health Care Needs of the Reentry Population 97 individuals under the FPL.13 In the highest quartile, census tracts have at least 2.5 times as many FTEs (0.88). We see a similar result when we look at all PCCs. For all clinics, census tracts in the mid-low quartile have, at most, 0.71 FTEs within a 10-minute drive per 1,000 individuals under the FPL.14 In the highest quartile, census tracts have nearly twice as many FTEs (1.38). Table 5.7 compares the accessibility of parolees in San Diego County to MISP and all other PCCs by race/ethnicity. Overall, about 63 percent of parolees reside in areas with reasonable access to MISP clinics as measured by the percentage of parolees residing in areas with the two highest levels of accessibility. If we include the other PCCs in the county, the share of parolees residing in these areas increases another 10 percentage points (to 73 percent), suggesting that the community PCCs play an important role in filling geographic gaps in access to primary care. Accessibility, however, varies across race/ethnicity. About 75 percent of African-American and 64 percent of Latino parolees reside in areas with the two highest levels of accessibility to the MISP clinics, as compared to 53 percent of white parolees. The percentage of Latino parolees in these two categories increases from 64 percent to 80 percent when the other PCCs are included, suggesting that these clinics play an important role in increasing accessibility for this group of parolees. We also see a 5-percentage-point increase for African-American parolees. In addition, inclusion of the other PCCs increases by 10 percentage points the share of white parolees who fall into the two highest accessibility categories. Table 5.7 Distribution of Parolees Across Clinic Accessibility Categories, by Quartile and Race/Ethnicity, San Diego County (percent) Level of Accessibility (quartile) Total (n = 9,782) African-American (n = 2,650) Latino (n = 2,867) White (n = 3,671) MISP clinics Lowest (0.00) 15.8 8.8 13.6 22.7 Mid-low (0.01–0.33) 21.7 16.6 22.0 24.8 Mid-high (0.34–0.87) 19.8 18.9 23.6 17.1 Highest (0.88–7.11) 42.7 55.6 40.8 35.4 Lowest (0.00–0.26) 9.0 5.9 5.5 13.1 Mid-low (0.27–0.71) 17.6 14.6 14.1 22.5 Mid-high (0.72–1.37) 29.2 32.6 31.4 24.5 Highest (1.38–7.11) 44.2 46.9 48.9 39.9 All clinics Note: The “other” race/ethnicity group (n = 594) is not shown. For the lowest quartile for the MISP clinics, 0.0 indicates that 25 percent of census tracts in San Diego County do not have any FTEs within a 10-minute drive time. 13 Based on the population-weighted centroid of each census tract. 14 Based on the population-weighted centroid of each census tract. 98 Understanding the Health Implications of Prisoner Reentry in California, Phase I Report Figure 5.16 shows the distribution of general acute care hospitals in San Diego County. The University of California (UC) San Diego Medical Center provides the largest share of publicly funded services in the county. Unlike the other three counties, San Diego County has a number of MISP hospitals—12 total, including UC San Diego Medical Center—and so appears to have better coverage than the other counties.15 All these hospitals have emergency departments. The MISP hospitals are concentrated in the more densely populated areas of the county, with reasonable coverage in the southern and northern half of the county vis-à-vis concentrations of parolees. District 2 only has general acute care hospitals on the western edge of its boundary. When we add in the remainder of the other nonprofit general acute care hospitals16 (n = 5) in the county (i.e., non-MISP) the pattern remains the same. Figure 5.17 shows our measure of accessibility for general acute care hospitals in San Diego County by census tract. Two of the 11 MISP hospitals and two other general acute care hospitals were missing capacity data and so were not included in our measure of accessibility. Comparing the accessibility map to the concentration of parolees shown in Figure 5.16, we see that parolees concentrated in the downtown area, parts of Southeast San Diego, and near Mission Bay have relatively higher levels of accessibility. Concentrations of parolees in the Chula Vista, Lemon Grove, Spring Valley, Vista, and Escondido areas fall into the middle categories of accessibility. The concentration of parolees in District 2 falls into the lowest accessibility category. We examined the sensitivity of our results to the missing data for hospitals by recalculating our accessibility measures using the counts of facilities rather than FTEs. Accessibility increased by one level for the areas around Oceanside and Vista in the north and around Chula Vista in the southern part of the county when we included in our measure those hospitals that were missing capacity data. In addition, fewer census tracts around the city of San Diego had the highest level of accessibility. Also, accessibility increased by one level for the areas around La Mesa and Lemon Grove. Our sensitivity analysis for hospitals shows that our measure of accessibility is somewhat sensitive to the degree of missing capacity data for hospitals, but not as sensitive as we saw for clinics. For the alternate accessibility measure, see Appendix E. Table 5.8 summarizes the accessibility of parolees to hospitals in San Diego County. Census tracts in the mid-low quartile have, at most, 69.7 FTEs within a 10-minute drive per 1,000 individuals under the FPL.17 In the highest quartile, census tracts have nearly twice as many FTEs (133.6). Overall, more than half of parolees reside in areas with the two lowest levels of accessibility (Table 5.8). Latino parolees have somewhat lower accessibility to general acute care hospitals than do African-American and white parolees. Fifty-nine percent of Latino parolees reside in areas with the two lowest levels of accessibility, compared to 51 percent of African-American parolees and 53 percent of white parolees. 15 The MISP hospitals in San Diego County are UC San Diego Medical Center, Alvarado Hospital Medical Center, Fallbrook Hospital District, Grossmont Hospital, Palomar Medical Center, Paradise Valley Hospital, Pomerado Hospital, Scripps Memorial Hospitals (La Jolla and Encinitas), Scripps Mercy Hospitals (San Diego and Chula Vista), Sharp Coronado Hospital, Sharp Memorial Hospital, and Loma Linda University Community Medical Center. Because Loma Linda University Community Medical Center is located outside of San Diego County, it is not shown in Figure 5.16. 16 Three of the five other nonprofit hospitals in the county have emergency departments. 17 Based on the population-weighted centroid of each census tract. Counties’ Capacity to Meet the Health Care Needs of the Reentry Population Figure 5.16 Map of General Acute Care Hospitals, by Supervisorial District, San Diego County 99 100 Understanding the Health Implications of Prisoner Reentry in California, Phase I Report Figure 5.17 Map of General Acute Care Hospitals Accessibility Measure, San Diego County Table 5.8 Distribution of Parolees Across Hospital Accessibility Categories, by Quartile and Race/Ethnicity, San Diego County (percent) Total (n = 9,782) African-American (n = 2,650) Latino (n = 2,867) White (n = 3,671) Lowest (0.00) 19.6 10.7 21.0 24.5 Mid-low (0.1–69.7) 34.8 40.0 37.9 28.5 Mid-high (69.8–133.5) 21.7 20.0 24.2 20.9 Highest 133.6–465.3) 23.9 29.3 16.9 26.1 Level of Accessibility (quartile) Note: The “other” race/ethnicity group (n = 594) is not shown. In summary, the results for San Diego County show that MISP clinics play an important role in increasing accessibility to clinic services for African-American and Latino parolees. We found only minor differences in accessibility to safety-net hospitals among the three groups of parolees. Counties’ Capacity to Meet the Health Care Needs of the Reentry Population 101 Discussion Our approach to measuring accessibility was to focus on how parolees are distributed within a county vis-à-vis the county’s safety net. We examined parolee locations overall and by race/ ethnicity. An important contribution of our methodology for measuring accessibility is that it moves beyond simple mapping of facilities relative to the location of the parolee population. A common criticism of simply mapping facility locations is that such an approach fails to take into account the capacity of facilities and the underlying population demand for these services. For example, if two clinics are located near a specific population of parolees, simply mapping the location of each clinic would not account for the relative sizes of the clinics. It would miss the fact that the clinics differ in their capacity to serve parolees and other vulnerable populations (e.g., one clinic may have 15 physicians and the other only two). Our accessibility measure takes capacity into account by incorporating a measure of capacity (FTEs) in the calculation. A second criticism acknowledges demand. The size of the population served by the facilities also matters. Because most safety-net facilities do not serve only the parolee population, it is also important to take into account the underlying population’s demand for a facility’s services. For example, two clinics may have the same capacity, but one clinic may be located in an area where the underlying population’s demand for those services is relatively low, whereas the other clinic may be located in an area where the demand for services from other vulnerable populations is high. Because we do not know the actual demand in a neighborhood, we used the share of the population that is below the FPL as our measure of underlying potential demand on the safety net. Importantly, our sensitivity analysis suggested that our method for measuring accessibility was robust in two of the counties (Alameda and Kern) but more sensitive to missing capacity data in the other two counties (Los Angeles and San Diego). For the alternate maps of accessibility that simply used counts of facilities in these four counties, we refer the reader to Appendix E. In some cases, we see differences due to missing data. The underlying causes for these differences are unclear but may be due to the relative size of the facilities that are missing or to the pattern of missing information being not randomly distributed. In other cases, we see differences between when we weighted the results using capacity data versus when we used unweighted counts of facilities. One would not expect the two measures to give the same answer. For example, assume that there are two areas with the same population under the FPL, and it is similarly distributed. The first area has two hospitals, located side by side, with 250 FTEs each. Another area also has 500 FTEs, but they are all located in one hospital. These two areas would score equivalently in their level of accessibility under our primary measure of accessibility because each has 500 FTEs per 50,000 population. But they would score differently under our sensitivity analysis. In that case, the first area would look better because we are counting each facility equally as a single unit of supply. So, the first area would have two facilities per 250,000 population and the other only half as many. For this reason, we consider our primary measure based on FTEs to be more accurate. The concern, however, is that data on capacity needs to improve so that we no longer encounter so much missing data (which can skew findings). We also considered a sensitivity analysis that used beds, instead of FTEs, as our measure of hospital capacity. The results were similar. However, this method did not address the issue of missing data because we found that the pattern of missing data on beds was similar to that for missing data on FTEs. In other words, if a facility was missing data on FTEs, they also were missing data on beds. 102 Understanding the Health Implications of Prisoner Reentry in California, Phase I Report Our geographic mapping and accessibility measures suggest that parolees’ accessibility varies across counties and by race/ethnicity within counties. Parolees’ accessibility also varies by facility type (i.e., hospital or clinic). Table 5.9 summarizes the results from our accessibility measures by county and type of health care setting. In terms of general acute care hospitals, a larger share of parolees in Alameda County resided in areas with lower levels of accessibility than those in the other counties. Interestingly, in all three of the large urban counties (Alameda, Los Angeles, and San Diego), more than half of parolees resided in areas with the two lowest levels of accessibility for general acute care hospitals. Overall, parolees in Kern County (63 percent) were more likely to live in areas that fall into the two lowest levels of hospital accessibility. In terms of general acute care hospitals, a larger share of parolees in Alameda County resided in areas with lower levels of accessibility than in the other counties. Interestingly, within all three of the large urban counties (Alameda, Los Angeles, and San Diego), more than half of parolees resided in areas with the two lowest levels of accessibility for general acute care hospitals. In comparison, only 37 percent of parolees in Kern County lived in areas that had the lowest levels of hospital accessibility.Accessibility to health care resources also varied by parolees’ race/ethnicity (Table 5.10). With respect to accessibility to general acute care hospitals, in Los Angeles and Alameda counties, more African-American parolees were in areas with lower accessibility compared to Latino and white parolees. However, in Kern and San Diego counties, more Latino parolees resided in areas with lower accessibility to hospitals compared to white and African-American parolees. With respect to accessibility to PCCs by race/ethnicity, the story is more complex, with a key theme being that the county matters (see Table 5.10). In three counties, only one out of five African-American parolees resided in areas with the two lowest levels of accessibility. The exception was Los Angeles County, where 44 percent of African-Americans resided in areas Table 5.9 Summary of Accessibility Results, by County and Type of Facility County Percentage of Parolees Who Fell into the Two Lowest Accessibility Categories Hospitals Alameda 63 Kern 37 Los Angeles 53 San Diego 54 Clinics Alameda 38 Kern 30 Los Angelesa 48 San Diego 27 a In Los Angeles County, the comparison represents accessibility to public/private partnership clinics and other PCCs. It differs from the other three counties, whose measure of accessibility includes both MISP clinics and other PCCs. Counties’ Capacity to Meet the Health Care Needs of the Reentry Population 103 Table 5.10 Summary of Accessibility Results, by County, Type of Facility, and by Race/Ethnicity Percentage of Parolees by Race/Ethnicity Who Fell into Two Lowest Accessibility Categories County African-American Latino White Alameda 73 47 48 Kern 31 40 37 Los Angeles 60 51 47 San Diego 51 59 53 Alameda 24 48 59 Kern 24 25 37 Los Angelesa 44 47 57 San Diego 21 20 36 Hospitals Clinics a In Los Angeles County, the comparison represents accessibility to public/private partnership clinics and other PCCs. It differs from the other three counties, whose measure of accessibility includes both MISP clinics and other PCCs. with the two lowest levels of accessibility. For Latino parolees in Alameda and Los Angeles counties, the share residing in areas with the two lowest levels of accessibility to clinics was nearly 50 percent. This is significantly higher than the roughly 20 percent of Latino parolees in Kern and San Diego counties. Finally, about 60 percent of white parolees in Alameda and Los Angeles counties were in areas with the two lowest levels of accessibility to clinics, compared to 37 percent in Kern and 36 percent in San Diego. As discussed earlier, we elected to include in our maps the supervisorial districts and, in the case of Los Angeles County, the SPAs to better place these results both in a policy- and health-planning context. The supervisorial districts represent the political districts in a county. The eight SPAs in Los Angeles County are for health-planning purposes, with each SPA having an assigned Area Health Office that is responsible for planning public health and clinical services according to the health needs of local communities (County of Los Angeles Department of Public Health, undated). These results highlight which districts in the four counties and which SPAs in Los Angeles County are disproportionately affected by prisoner reentry and relative accessibility of parolees to health care resources. All the counties show some geographic gaps in health care resources vis-à-vis the distribution of parolees. However, the most prominent gaps appear to be in Los Angeles County. For this county, the maps and measures of accessibility illustrate how areas with the highest concentration of parolees (e.g., SPA 6 and District 2, which include South Los Angeles) lack health care resources in general. SPAs 3 (San Gabriel area) and 7 (East Los Angeles area) also lack safety-net hospitals. Other general acute care hospitals play an important role in filling gaps in services in most areas of the county, but not in SPA 6 (South Los Angeles) or SPA 1 (Antelope Valley). The situation in SPA 6 may actually have worsened since the period shown in our maps. In 2005, Martin Luther King Jr./Charles R. Drew Medical Center was a fully functioning hospital with both inpatient and outpatient services. It served as a 104 Understanding the Health Implications of Prisoner Reentry in California, Phase I Report major hospital for the uninsured and poor in Los Angeles County. However, in August 2007, the hospital was shut down and, currently, it provides only outpatient services. Other surrounding hospitals have had to fill the gap in inpatient services. The outpatient clinic at King/ Drew has seen a substantial drop in visits because of the negative attention that the medical center has received with community clinics having to pick up the slack (Steinhauer, 2008). In all the counties, community clinics appear to play an important role in filling geographic gaps in coverage relative to the MISP clinics vis-à-vis the reentry population. To illustrate, in Los Angeles County, there are relatively few MISP clinics scattered throughout the county; as a result, PPP community clinics, or MISP (PPPs), extend safety-net services to many areas without MISP clinics. Yet, the areas with some of the highest concentrations of parolees (e.g., SPA 6, South Los Angeles; SPA 7, East Los Angeles; and SPA 8, especially north Long Beach) do not have a commensurate number of facilities nearby. These findings have planning implications for these SPAs and districts in terms of how best to provide services to this population. Our finding that gaps in health care accessibility are more prominent in Los Angeles County and, in particular, in South Los Angeles or SPA 6, is consistent with other research. The South Los Angeles Equity Scorecard provides a comprehensive picture of disparities between South Los Angeles and the rest of the county in terms of availability of health care resources (e.g., health care facilities, access to primary care, and health care coverage) and physical resources (e.g., affordable housing, quality schools, and public safety). Park, Watson, and Galloway-Gilliam (2008) found that the supply of general acute care beds and licensed acute psychiatric beds per 1,000 population was substantially lower in South Los Angeles (0.68) than in Los Angeles County overall, which had with 1.23 beds per 1,000 population. And that the supply of inpatient bed capacity in South Los Angeles decreased further—to 0.43 beds per 1,000 population—following the closure of Martin Luther King/Harbor Hospital and Daniel Freeman Memorial Hospital in 2007. An important contribution of our study is to highlight how such differences in health care resources (in SPA 6 and other areas of Los Angeles County) relate to the parolee population. We used a measure of accessibility that was based on a drive time of 10 minutes to a facility. An alternate measure would rely on the federal health professional shortage area designation of excessive distance: 30 minutes of travel time to primary care (HRSA, undated). However, we used a shorter drive time for several reasons. First, we are concerned that this population may be less mobile than the general population and likely more reliant on public transportation. Second, our goal was to identify variation in terms of how well certain areas are served, rather than to designate shortage areas. Finally, our measure of accessibility takes into account more than simple drive time; it also includes measures of facilities’ capacity (when available) and the underlying population demand for services as measured by the percentage of the population below the FPL. That said, our measure of accessibility is influenced by the 10-minute drive time threshold, our measure of potential demand for safety-net services (i.e., percent of population below the federal poverty level), and the spatial units that we had to use (i.e., census tracts versus street addresses). If one were to increase the drive time, change the spatial units, or use a different proxy to measure potential demand, a different set of results might be obtained. We validated our findings by examining whether areas or populations defined as having relatively lower accessibility according to our metric were similar to those identified as being medically underserved according to other metrics. Our accessibility scale is relative within a Counties’ Capacity to Meet the Health Care Needs of the Reentry Population 105 given county, whereas the areas identified as being underserved are not (those identifications are simply based on an absolute definition). Nevertheless, if there are underserved areas in a county, they should overlap with our lower (rather than higher) accessibility areas. To determine whether an area or population is medically underserved, an index can be computed using four variables: (1) the ratio of primary care physicians per 1,000 population, (2) the infant mortality rate, (3) the percentage of the population with incomes below the poverty level, and (4) the percentage of the population age 65 and over (Rural Policy Research Institute, 2006). We compared the locations of medically underserved areas and populations in California (OSHPD, 2007) with our measures of accessibility. For example, according to the California Office of Statewide Planning and Development, the northwestern section of Alameda County has a medically underserved population (OSHPD, 2007). Using our measure of accessibility, we also found that accessibility to hospitals was low in this area of the county. However, no area of Alameda County was designated as medically underserved (OSHPD, 2007). Approximately two-thirds of Kern County has been designated as a medically underserved area, with the exception of the city of Bakersfield and the southeast corner of the county. In addition, two areas are designated as having medically underserved populations: (1) near the city of Delano and (2) the northeast corner of the county near Ridgecrest. Our analysis indicated that accessibility to clinics in these two areas and to hospitals in the Ridgecrest area was reasonably good. However, with the exception of Bakersfield, we found that many areas of Kern County had low levels of accessibility to hospitals and clinics. The northeastern part of Los Angeles County (east of Lancaster and Palmdale) is designated as a medically underserved area. This is consistent with our finding of low levels of accessibility to clinics and hospitals in this part of the county. In addition, most of SPA 6, the area around San Pedro and Long Beach in SPA 8, the eastern half of SPA 4, the northern section of SPA 7, and part of SPA 2 in the San Fernando Valley are designated as having medically underserved populations. This is consistent with our finding that these areas, particularly SPAs 6 and 7, tend to have some of the lowest levels of accessibility. These gaps have potentially important implications for reentry, because large concentrations of parolees reside in these areas. The eastern half of San Diego County is designated as a medically underserved area, with the areas around the city of San Diego and to the south of San Diego considered to have medically underserved populations. This is consistent with our measure of accessibility for clinics but less so with respect to our measure of accessibility for hospitals. In addition, our measure of accessibility is intended to provide an assessment of the relative differences in accessibility to health care facilities within a county. This relative measure also reflects differences in land use and transportation development within that county. Our intent was not to make judgments about what is or should be an acceptable level of accessibility for a county. It may be that the lowest level of accessibility is an acceptable level for a given area or population, or that the highest level of accessibility is not enough. As noted earlier, there are standard metrics used by health planners to identify medically underserved areas and populations or areas with a shortage of health professionals. Our measure complements these metrics by enabling health planners and policymakers to focus specifically on relative accessibility among the parolee population—a population not typically considered in these other measures. Finally, as noted earlier, one needs to interpret the mapping of concentrations of parolees with caution. Census tracts located in more rural areas tend to be larger in size, so concentrations of parolees may appear to be larger in rural areas than in urban areas, where the census tracts tend to be smaller. Chapter Six Counties’ Capacity to Meet the Mental Health and Substance Abuse Treatment Needs of the Reentry Population Introduction In Chapter Two, we showed that California inmates’ mental health and substance abuse treatment needs appear to be even more pronounced than their physical health needs, with more than half of California inmates reporting recent mental health problems and about two-thirds reporting substance abuse or dependence (James and Glaze, 2006). Comorbidity, with respect to mental health issues and substance abuse/dependence, is also an important concern for this population. Given these needs, this chapter examines parolees’ accessibility to mental health and substance abuse treatment resources within the four counties that were the focus of our study. This chapter provides an in-depth examination of the four counties’ mental health and substance abuse safety nets and their availability to meet the treatment needs of the reentry population. More specifically, in this chapter, we present our mapping and accessibility results for the mental health and substance abuse treatment safety nets in each of the four counties and discuss their implications. In this chapter, we map resources such as county mental health services and county alcohol and drug treatment services that serve the uninsured, medically indigent, and low-income populations. In addition, because there are also several components of the safety net that are specific to the parolee population, we also map the Parole Outpatient Clinics (POCs, colocated with parole offices) that provide mental health services to parolees and the PSN that provides funding to counties for alcohol and drug treatment services for a subset of the parolee population. These resources are plotted onto the maps that we used in Chapter Three to show parolee concentrations and clusters in the four counties selected for indepth study. As we did in Chapter Five when we assessed accessibility results for physical health care needs, we maximize the utility of our maps for policymakers by including supervisorial district boundaries. The supervisorial districts represent the political districts within a county. For Los Angeles County only, we also show the eight SPAs. The SPA designation is used for health planning purposes, with each SPA having a health office responsible for planning public health and clinical services in a given area. Using both supervisorial districts and SPA boundaries provides additional context from a policy- and health-planning perspective, helping policymakers understand where the impact of mental health and substance abuse treatment needs of the reentry population may be greatest. We have organized this chapter by presenting the mapping and accessibility results for the mental health care and substance abuse treatment safety nets for each county, followed by an overarching summary of the findings across counties. Those interested in the results for a 107 108 Understanding the Health Implications of Prisoner Reentry in California, Phase I Report particular county can proceed directly to the section of this chapter that presents the findings for that county. Those interested in the overall summary of the findings can proceed to the discussion section at the end of this chapter. As we did in Chapter Five for the health care safety net, for each of the four counties, we first examined the geographic distribution of facilities that make up the mental health care safety net—specifically, county mental health clinics, MHSA or Medi-Cal mental health providers, and POCs—and compared them to the distribution of parolee concentrations across census tracts within each county. Similarly, for the substance abuse treatment safety net, we first examined the geographic distribution of facilities comprised by this safety net (Proposition 36 and other county alcohol and drug treatment providers, and the PSN) and compared them to the distribution of parolee concentrations in each county. For both safety nets, we also generated measures of the accessibility of facilities to the parolee population using a simple count of facilities and a proxy for the underlying population demand for their services. (For more detail on the underlying methodology used to create the mental health care and substance abuse accessibility measures, see Chapter Four.) Unlike in the case of the health care safety net, we used a simple count of facilities rather than capacity to calculate the accessibility measures; thus, we did not conduct a sensitivity analysis for the mental health care and substance abuse treatment safety nets. The reason was that we did not have capacity data for either the mental health or substance abuse facilities. The accessibility measures for each type of safety net allocate census tracts into quartiles, with the four quartiles representing differing levels of accessibility to mental health or alcohol and drug treatment facilities. We then tabulated the share of parolees living in the census tracts by quartile overall and by race/ethnicity. Also, for mapping purposes, duplicate listings (for example, where several different types of substance abuse treatment programs are provided in a single location)1 are shown as a single geographic point on the maps. However, our accessibility measures take into account those instances in which it appears that more than one type of service or program is being provided at a single location. The reason for doing this was to ensure that the measure of accessibility reflected as accurately as possible the availability of all relevant facilities in a given county. In the following section, for each of the four counties, we summarize our findings, first for the mental health safety net and then for the substance abuse treatment safety net. Analytic Findings for Mental Health Care Safety Net Alameda County For Alameda County’s mental health care safety net, we focused on both the mental health clinics listed in the SAMSHA database and the county mental health providers that represent the safety net for the medically indigent and uninsured population. As noted in the methodology in Chapter Four, we were not able to identify MHSA providers for this county. 1 Some treatment providers may have multiple locations within a county, in which case we map each location. In other instances, two treatment providers, for example, may be housed in the same physical location, in which case we would map the single location but include both providers in our accessibility measures. Finally, in other instances, a treatment provider may have two different types of programs (one residential and the other outpatient) that are housed in the same physical location—again, we would map the single location but include both types of programs in our accessibility measures. Counties’ Capacity to Meet the Mental Health and Substance Abuse Treatment Needs 109 In Alameda County, mental health providers, like the health care safety-net providers, follow the corridor of concentrations of parolees along the western urban areas of the county (Figure 6.1). Some mental health clinics also appear to serve the inland areas in District 1 and are relatively near the concentrations of parolees in this area. As was true for the health care safety net, around Hayward and Union City (District 2), there are several concentrations of parolees who do not appear to be served by any of the mental health providers listed; these are the concentrations that look like butterfly wings on either side of Hayward and the area around Union City. As mentioned in Chapter Four, POCs provide basic mental health services to parolees in a county. There are only three clinic locations in Alameda County, in Hayward, Oakland, and Berkeley (Figure 6.1). The clinics appear to be located relatively near the major concentration of parolees. We calculated an accessibility measure only for the mental health providers in Alameda County. For this and the other three counties, we did not include POCs in our accessibility measure because there are so few of them. Figure 6.2 shows that the pattern of accessibility to mental health resources differs from what we saw for health care resources in the county. As noted earlier, the accessibility measure for a given census tract should be viewed as an average Figure 6.1 Map of Mental Health Providers and Parole Outpatient Clinics, by Supervisorial District, Alameda County 110 Understanding the Health Implications of Prisoner Reentry in California, Phase I Report Figure 6.2 Map of Mental Health Provider Accessibility Measure, by Supervisorial District, Alameda County accessibility measure of all persons within the tract, and it incorporates demands on the facility by the underlying population of the surrounding area (including nonparolees). The parolee concentrations in District 1 around Newark and Fremont and near Livermore are in areas with high levels of accessibility (as reflected by the dark green shading in Figure 6.2). Parolee concentrations in the northern part of the county (District 5) and around Hayward and San Lorenzo (Districts 2 and 3) also appear to be in areas with higher levels of accessibility. The parolee concentrations around Oakland and Alameda (Districts 3 and 5), however, are in areas of the county with the two lowest levels of accessibility. Table 6.1 summarizes the accessibility of parolees in Alameda County to mental health services. The lowest, or 25th, quartile (light green on the map in Figure 6.2) includes areas with the lowest level of accessibility; the highest, or 75th, quartile (dark green on the map) includes areas that have the highest level of accessibility to mental health providers. Based on the location of the population-weighted centroid, census tracts in the lowest quartile have, at most, 3.0 facilities within a 10-minute drive per 10,000 individuals under the FPL. That compares to at least 5.2 facilities in the highest quartile (Table 6.1). There also is considerably more variability in accessibility across census tracts in the highest quartile, as evidenced by the broader range for this quartile. Table 6.1 also compares the accessibility of parolees in Alameda County to mental health services by race/ethnicity. Overall, nearly half (47 percent) of parolees lived in areas with greater access to mental health providers, as measured by the percentage of parolees who fall into the Counties’ Capacity to Meet the Mental Health and Substance Abuse Treatment Needs 111 Table 6.1 Distribution of Parolees Across Mental Health Provider Accessibility Quartile Categories, by Race/Ethnicity, Alameda County (percent) Total (n = 4,689) African-American (n = 2,904) Latino (n = 776) White (n = 775) Lowest (0.0–3.0) 18.3 17.8 20.9 14.2 Mid-low (3.1–4.3) 34.8 39.3 30.9 23.7 Mid-high (4.4–5.1) 24.4 23.1 20.9 32.3 Highest (5.2–15.5) 22.5 19.7 27.3 29.8 Level of Accessibility (quartile) Note: The “other” race/ethnicity group (n = 234) is not shown. two highest accessibility categories (medium and dark green areas in Figure 6.3 and the last two rows of Table 6.1). Accessibility to mental health providers varies by race/ethnicity. White parolees appear to have substantially higher levels of accessibility, with about 62 percent living in areas with the two highest accessibility levels. This is substantially higher than the corresponding share of African-American (43 percent) and Latino parolees (48 percent). In summary, the mental health care safety-net results for Alameda County show that African-American and Latino parolees tend to live in areas with lower levels of accessibility to mental health clinics than do white parolees. In terms of the different areas of the county, the parolee concentrations around Oakland and Alameda are in areas with the two lowest levels of accessibility. Although there are few POCs in Alameda County, they tend to be located near some of the larger concentrations of parolees, in Oakland, Hayward, and Berkeley. Kern County For the mental health care safety net in Kern County, we focused on the Medi-Cal contract providers (both county and other Medi-Cal providers) and county mental health providers that represent the safety net for the low-income and uninsured population. In Kern County, the distribution of these mental health providers appears to represent reasonable coverage vis-à-vis the concentrations of parolees shown in Figure 6.3. For example, the map inset in Figure 6.3 shows that, for most of the concentrations of parolees (shaded in darker blue) around the city of Bakersfield, a mental health clinic is located relatively nearby. Looking at the rest of the county, the non-county contract Medi-Cal providers (shown as red circles on the map) are important resources for the concentrations of parolees outside Bakersfield. The only part of the county that does not have a mental health clinic near a concentration of parolees is the Rosamond area, although there are three Medi-Cal contract mental health providers in District 2. With respect to POCs, there are only two clinic locations in Bakersfield (Figure 6.3). Thus, the other concentrations of parolees in Kern County would have to travel to Bakersfield to access these parolee-specific mental health services. We calculated an accessibility measure only for the mental health providers in Kern County; we did not do so for the POCs, since there are only a few locations. Figure 6.4 shows that accessibility to mental health services is highest for the parolee concentrations surrounding Bakersfield, in the western section of District 1 (Wasco and Shafer), the eastern section of District 1 (Ridgecrest), and around Tehachapi in District 2. Other parolee concentrations have lower levels of accessibility to mental health services. 112 Understanding the Health Implications of Prisoner Reentry in California, Phase I Report Figure 6.3 Map of Mental Health Providers and Parole Outpatient Clinics, by Supervisorial District, Kern County Table 6.2 summarizes the accessibility of parolees in Kern County to mental health services. Based on the location of the population-weighted centroid, census tracts in the lowest quartile have, at most, 0.3 facilities within a 10-minute drive per 10,000 individuals under the FPL (Table 6.2). That compares poorly to the highest quartile, where census tracts have at least nearly 13 times as many facilities (3.8). As in Alameda County, there is considerably more variability in accessibility across census tracts in the highest quartile in Kern County. Comparing accessibility to mental health services by race/ethnicity, Table 6.2 shows that, overall, nearly three-quarters of parolees in Kern County reside in areas with the two highest levels of accessibility (shown by the two darkest shades of green in the map in Figure 6.4). Accessibility measures are also high for all three racial/ethnic groups. However, a larger share of African-American parolees (80 percent) fall into the two higher accessibility categories, compared to Latino (70 percent) and white (74 percent) parolees. In summary, overall, the majority of parolees in Kern County reside in areas with the two highest levels of accessibility to mental health clinics, and we did not see major differences in accessibility by race/ethnicity. The two POCs in this county are located around Bakersfield; thus, parolees from other parts of the county would have to travel some distance to access these services. Counties’ Capacity to Meet the Mental Health and Substance Abuse Treatment Needs Figure 6.4 Map of Mental Health Provider Accessibility Measure, by Supervisorial District, Kern County Table 6.2 Distribution of Parolees Across Mental Health Provider Accessibility Quartile Categories, by Race/ Ethnicity, Kern County (percent) Level of Accessibility (quartile) Total (n = 5,936) African-American (n = 1,105) Latino (n = 2,247) White (n = 2,478) Lowest (0.0–0.3) 10.9 7.4 10.0 13.4 Mid-low (0.4–2.2) 15.6 12.7 19.7 12.9 Mid-high (2.3–3.7) 30.2 28.5 24.6 36.2 Highest (3.8–151.5) 43.2 51.4 45.7 37.4 Note: The “other” race/ethnicity group (n = 106) is not shown. 113 114 Understanding the Health Implications of Prisoner Reentry in California, Phase I Report Los Angeles County For the mental health care safety net in Los Angeles County, we focused on MHSA providers and other mental health providers listed in the SAMSHA database, augmented with additional information, as noted in Chapter Four. For Los Angeles County, we show the mental health services safety net overall and then concentrate on the southern half of the county, where the majority of the population is located. Overall, the distribution of MHSA providers (shown as red circles on the map in Figure 6.5) generally seems to be near the majority of concentrations of parolees shown in Figures 6.5 and 6.6. An exception is the Pomona area. In northern Los Angeles County, the Lancaster/Palmdale area is served by only one MHSA provider. When the other community mental health providers (shown as yellow circles in Figures 6.5 and 6.6) are considered, we see that they appear to help fill gaps in coverage in Los Angeles County, except in District 2/SPA 6 (South Los Angeles) and District 3/SPA 2 (San Fernando Valley), where there are relatively large concentrations of parolees but fewer community mental health providers. In these localities, MHSA providers remain the primary source of mental health services. With respect to POCs, there are 11 clinic locations in Los Angeles County (Figures 6.5 and 6.6). Each SPA has at least one POC, with the exception of SPAs 4 (Metro Los Angeles/ Hollywood) and 5 (West Los Angeles). These clinics tend to be located near the largest concentration of parolees; however, there are large geographic gaps in coverage, such as in SPA 8 (South Bay/Long Beach), SPA 2 (San Fernando Valley and Santa Clarita area), and SPA 1 (Lancaster/Palmdale area). The limited number of these clinics suggests that POCs represent a sparser mental health safety net for the parolee population. As was the case for Kern County, we calculated an accessibility measure only for MHSA and other mental health providers in Los Angeles County. Figure 6.7 shows that accessibility to mental health providers is highest for the parolee concentrations in three areas: (1) Santa Monica and Venice, and downtown Los Angeles (SPA 5 [District 2, not shown]); (2) near Long Beach and Norwalk (SPAs 7 and 8 [District 4, not shown]); and (3) the dark green swath shown in Figure 6.7 that runs from Pasadena, El Monte, and Covina to Pomona (SPA 3 [District 2, not shown]). The areas with the highest concentrations of parolees near Gardena, Hawthorne, Compton, and Lynwood (SPA 6 and SPA 7 [Districts 2 and 4, not shown]) have some of the lowest levels of accessibility. Also, parolee concentrations in the San Fernando Valley (SPA 2 [District 3, not shown]) are located in areas with lower levels of accessibility. Table 6.3 summarizes the accessibility of parolees in Los Angeles County to mental health services. Based on the location of the population-weighted centroid, Los Angeles County census tracts in the lowest quartile have, at most, 0.4 facilities within a 10-minute drive per 10,000 individuals under the FPL. In the highest quartile, census tracts have at least four times as many facilities (1.6). Again, as in the other two counties, there is considerably more variability in accessibility across census tracts in the highest quartile. Overall, nearly half of parolees fall into the two highest accessibility categories (Table 6.3). However, a smaller share of African-American parolees (43 percent) fall into the two higher accessibility categories than Latino (51 percent) and white (53 percent) parolees. Counties’ Capacity to Meet the Mental Health and Substance Abuse Treatment Needs Figure 6.5 Map of MHSA and Other Mental Health Providers and Parole Outpatient Clinics, by Supervisorial District, Los Angeles County 115 116 Understanding the Health Implications of Prisoner Reentry in California, Phase I Report Figure 6.6 Map of MHSA and Other Mental Health Providers and Parole Outpatient Clinics, by Service Planning Area, Los Angeles County Counties’ Capacity to Meet the Mental Health and Substance Abuse Treatment Needs 117 Figure 6.7 Map of Mental Health Provider Accessibility Measure, by Service Planning Area, Los Angeles County Table 6.3 Distribution of Parolees Across Mental Health Provider Accessibility Quartile Categories, by Race/Ethnicity, Los Angeles County (percent) Total (n = 35,710) African-American (n = 12,885) Latino (n = 14,921) White (n = 6,671) Lowest (0.0–0.4) 18.5 11.4 20.3 27.9 Mid-low (0.5–1.0) 32.8 45.1 29.0 19.2 Mid-high (1.1–1.5) 27.7 28.8 27.9 24.1 Highest (1.6–31.6) 21.0 14.6 22.9 28.8 Level of Accessibility (quartile) Note: The “other” race/ethnicity group (n = 1,233) is not shown. In summary, the results for Los Angeles County show that, overall, about half of parolees live in areas with the two highest levels of accessibility to mental health clinics, with some modest differences in accessibility by race/ethnicity. MHSA providers are an important potential source of mental health services for the parolee population. However, as we discuss at the end of this chapter, Los Angeles County limits access to these resources by using the POCs as 118 Understanding the Health Implications of Prisoner Reentry in California, Phase I Report “gatekeepers” for referral to county mental health safety-net resources. Six of the eight SPAs have a POC, which tends to be located near some of the largest concentrations of parolees; still, many parolees would have to travel some distance to access these services. San Diego County For the mental health care safety net in San Diego County, we focused on MHSA clinics and other county mental health clinics that represent the safety net for the uninsured population. In addition, we examined the distribution of POCs for the parolee safety net. For San Diego County, we found that MHSA clinics and other county mental health clinics are located primarily in the more urban parts of the county (Figure 6.8). Areas without coverage that also have concentrations of parolees are more inland, such as National City (southern area of District 4) and Lakeside, Alpine, Lemon Grove, and the southern half of District 2. Other mental health clinics appear to play an important role in helping to fill gaps in coverage, particularly in the areas of La Mesa, El Cajon, and the southern half of District 2; Chula Vista and Imperial Beach in District 1; and the city of San Diego (District 4). With respect to POCs, there are five locations in San Diego County (Figure 6.8). These POC locations are spread across the county, with some gaps in coverage around the downtown San Diego and Southeast San Diego areas and most of District 2. The limited number of these clinics suggests that the POCs represent a geographically sparser mental health safety net for the parolee population. As for the other counties, we calculated an accessibility measure only for MHSA and other county mental health clinics in San Diego County. In general, many of the parolee concentrations in San Diego County seem to be in areas with relatively good accessibility to mental health services, as measured by the two darker shades of green in Figure 6.9. Parolee concentrations near downtown San Diego and Southeast San Diego, as well as those near Chula Vista and National City, are in areas that fall into the two highest levels of accessibility. Parolee concentrations near Escondido and Oceanside also fall into the two highest accessibility categories. Unlike what we saw for other health care resources (see Chapter Five), parolee concentrations near the international border in District 2 are in areas with the highest level of accessibility to mental health providers. The parolee concentrations near El Cajon and Lakeside (District 5) tend to be in areas with somewhat lower levels of accessibility. Table 6.4 summarizes the accessibility of parolees in San Diego County to mental health services. Based on the location of the population-weighted centroid, census tracts in the lowest quartile have, at most, 0.7 facilities within a 10-minute drive per 10,000 individuals under the FPL. By comparison, the census tracts in the highest quartile have at least 3.6 times as many facilities (2.5). For San Diego County, there is more variability in accessibility across census tracts in the highest quartile. Overall, about 62 percent of parolees fall into the two highest accessibility categories (Table 6.4). We do not see substantive differences in mental health accessibility by race/ethnicity. In summary, the results for San Diego County show that more than three-fifths of parolees reside in areas with the two highest levels of accessibility to mental health clinics with no differences by race/ethnicity. Most of the mental health resources are located near the major urban areas, which means that parolees residing more inland and in the southern section of the county tend to have lower levels of accessibility. Also, the other community mental health providers play an important role in filling geographic gaps in coverage. The POCs are in five Counties’ Capacity to Meet the Mental Health and Substance Abuse Treatment Needs 119 locations spread across the county; however, the concentration of parolees around downtown San Diego and Southeast San Diego and in the southern half of District 2 are not near any of these clinics. Figure 6.8 Map of MHSA, Other Mental Health Clinics, and Parole Outpatient Clinics, by Supervisorial District, San Diego County 120 Understanding the Health Implications of Prisoner Reentry in California, Phase I Report Figure 6.9 Map of MHSA and Other County Mental Health Clinic Accessibility Measure, by Supervisorial District, San Diego County Table 6.4 Distribution of Parolees Across Mental Health Provider Accessibility Quartile Categories, by Race/Ethnicity, San Diego County (percent) Total (n = 9,782) African-American (n = 2,650) Latino (n = 2,867) White (n = 3,671) Lowest (0.0–0.7) 13.3 12.8 11.9 14.1 Mid-low (0.8–1.6) 24.4 25.0 24.0 24.3 Mid-high (1.7–2.4) 31.6 29.5 39.2 28.2 Highest (2.5–7.9) 30.8 32.6 25.0 33.5 Level of Accessibility (quartile) Note: The “other” race/ethnicity group (n = 594) is not shown. Counties’ Capacity to Meet the Mental Health and Substance Abuse Treatment Needs 121 Analytic Findings for the Substance Abuse Safety Net For each of the four counties, we examined the distribution of facilities that make up the substance abuse safety net2 and compare it to the distribution of parolee concentrations in the counties. In addition, we discuss our measure of accessibility with respect to the overall substance abuse treatment safety net vis-à-vis the parolee population. We did not calculate a measure of accessibility for the PSN facilities because there are so few of them in a given county. Alameda County For the substance abuse safety net in Alameda County, we focused on the Proposition 36 contract providers and other county alcohol- and drug-treatment providers. In Alameda County, the distribution of Proposition 36 providers and other alcohol- and drug-treatment providers follows the corridor of concentrations of parolees along the western urban areas of the county (Figure 6.10). Proposition 36 providers make up a larger proportion of the treatment capacity, as measured by the number of providers in this county. Figure 6.10 Map of Proposition 36 and Other Alcohol- and Drug-Treatment Providers, by Supervisorial District, Alameda County 2 We use the phrase substance abuse safety net to refer to alcohol- and drug-treatment services available to the under- and uninsured population in a county as well as, potentially, to parolees. 122 Understanding the Health Implications of Prisoner Reentry in California, Phase I Report With respect to the PSN, there are 17 treatment providers, two of which provide substance abuse services specifically for women. The majority of the PSN providers are located in Oakland, three are in Newark, and there is one each in Fremont and Hayward (Figure 6.11).3 Given the geographic distribution of these providers, it appears that the concentrations of parolees around San Leandro and the two parolee concentrations that look like butterfly wings on either side of Hayward have lower levels of accessibility to the PSN providers. We calculated an accessibility measure for the Proposition 36 and other alcohol and drug treatment providers in Alameda County but not for the PSNs, because access to them is determined primarily by the prison treatment program and by the parole agent. Overall, accessibility to substance abuse providers appears to be relatively good in many parts of Alameda County. Figure 6.12 shows that parolee concentrations in the northern part of Alameda County (District 5), Alameda and Oakland, San Lorenzo, and the Newark and Fremont areas have the two highest levels of accessibility to substance abuse providers. Parolee concentrations around Livermore and Dublin also have high levels of accessibility. Some exceptions are parts of District 3 around San Leandro and much of District 4 (excluding the area near Oakland), where there are parolee concentrations with lower levels of accessibility to substance abuse providers. Figure 6.11 Map of PSN Providers, by Supervisorial District, Alameda County 3 The map shows only 16 locations because two of the PSN providers are located at the same address. Counties’ Capacity to Meet the Mental Health and Substance Abuse Treatment Needs 123 Figure 6.12 Map of Proposition 36 and Other Alcohol and Drug Treatment Provider Accessibility Measure, by Supervisorial District, Alameda County Table 6.5 summarizes the accessibility of parolees in Alameda County to alcohol- and drug-treatment services. Based on the location of the population-weighted centroid, census tracts in the lowest quartile have, at most, 2.7 facilities within a 10-minute drive per 10,000 individuals under the FPL. By comparison, the census tracts in the highest quartile have at least 1.6 times as many facilities (4.4). In Alameda County, there is considerably more variability in accessibility across census tracts in the highest quartile. Comparing accessibility to substance abuse providers by race/ethnicity, we see that, overall, nearly two-thirds of parolees fall into the two highest accessibility categories (Table 6.5). We see very minor differences in substance abuse accessibility by race/ethnicity. About 62 percent of Latino and white parolees fall into the two highest accessibility categories, as compared to 56 percent of African-American parolees. In summary, the results for Alameda County show that Proposition 36 and other alcoholand drug-treatment providers are predominantly in the urban areas in the western part of the county, so the concentrations of parolees in these areas tend to have higher levels of accessibility than parolees residing in other parts of the county. Overall, nearly two-thirds of parolees reside in areas with the two highest levels of accessibility, with very minor differences in terms of race/ethnicity. The majority of the PSN providers are located in the areas around Oakland and Newark, with several near Hayward and Fremont. Other areas of the county are not covered by a PSN provider. 124 Understanding the Health Implications of Prisoner Reentry in California, Phase I Report Table 6.5 Distribution of Parolees Across Proposition 36 and Other Alcohol- and Drug-Treatment Provider Accessibility Quartile Categories, by Race/Ethnicity, Alameda County (percent) Level of Accessibility (quartile) Total (n = 4,689) African-American (n = 2,904) Latino (n = 776) White (n = 775) Lowest (0.0–2.7) 13.2 12.0 14.8 16.0 Mid-low (2.8–3.7) 28.3 32.0 23.3 23.0 Mid-high (3.8–4.3) 37.7 38.8 37.0 34.5 Highest (4.4.–27.9) 20.8 17.2 24.9 26.6 Note: The “other” race/ethnicity group (n = 234) is not shown. Kern County For the substance abuse safety net in Kern County, we focused on Proposition 36 contract providers and the other alcohol- and drug-treatment providers in the county. In Kern County, the majority of Proposition 36 and other treatment providers are concentrated around the city of Bakersfield, as shown in Figure 6.13. In the other parts of the county, treatment resources are sparse, although most concentrations of parolees appear to be Figure 6.13 Map of Proposition 36 and Other Alcohol- and Drug-Treatment Providers, by Supervisorial District, Kern County Counties’ Capacity to Meet the Mental Health and Substance Abuse Treatment Needs 125 somewhat near a provider. In addition, in Districts 2 and 4, the other treatment providers are important in filling gaps not covered by Proposition 36 providers. With respect to the PSN, there are five PSN providers in Kern County, with three located in Bakersfield and two in District 1 (Figure 6.14).4 However, Districts 2 and 3 do not have a PSN clinic, suggesting that parolees in these districts must rely on Proposition 36 and other alcohol- and drug-treatment providers in the county. We calculated an accessibility measure only for the Proposition 36 and other alcohol- and drug-treatment providers in Kern County; we did not do so for the PSN providers because they are much sparser. Figure 6.15 shows that accessibility to substance abuse providers is highest (as indicated by the dark green shading on the map) for parolee concentrations around Bakersfield and in several locations in District 2. The parolee concentrations near Delano and Wasco and Ridgecrest (District 1) and Tehachapi (District 2) are in areas with the next highest level of accessibility (as indicated by the medium shade of green). Parolee concentrations in the middle section of District 1 and near the southern county border of District 2 near Rosamond are in areas with the lowest level of accessibility (shaded light green). Figure 6.14 Map of PSN Providers, by Supervisorial District, Kern County 4 Unlike for the other three counties, we do not have treatment capacity information for the PSN providers in Kern County. 126 Understanding the Health Implications of Prisoner Reentry in California, Phase I Report Figure 6.15 Map of Proposition 36 and Other Alcohol- and Drug-Treatment Provider Accessibility Measure, by Supervisorial District, Kern County Table 6.6 summarizes the accessibility of parolees in Kern County to alcohol- and drugtreatment services. Based on the location of the population-weighted centroid, census tracts in the lowest quartile have, at most, 0.9 facilities within a 10-minute drive per 10,000 individuals under the FPL. By comparison, the census tracts in the highest quartile have at least six times as many facilities (5.7). Comparing accessibility to substance abuse providers by race/ethnicity, we see that, overall, 72 percent of parolees fall into the two highest accessibility categories (Table 6.6). We see some differences in accessibility by race/ethnicity. The share of African-American parolees (85 percent) who fall into the two highest levels of accessibility is about 15 percentage points higher than that of Latino (68 percent) and white (70 percent) parolees. In Kern County, there is more variability in accessibility across census tracts in the highest quartile. In summary, the results for Kern County show that Proposition 36 and other alcoholand drug-treatment providers, as well as PSN providers, are located predominantly around the city of Bakersfield. In addition, Proposition 36 and other alcohol- and drug-treatment providers provide coverage to other parts of the county, particularly in smaller towns. AfricanAmerican parolees tend to have higher levels of accessibility to substance abuse treatment providers than do Latino or white parolees. Counties’ Capacity to Meet the Mental Health and Substance Abuse Treatment Needs 127 Table 6.6 Distribution of Parolees Across Proposition 36 and Other Alcohol- and Drug-Treatment Provider Accessibility Quartile Categories, by Race/Ethnicity, Kern County (percent) Level of Accessibility (quartile) Total (n = 5,936) African-American (n = 1,105) Latino (n = 2,247) White (n = 2,478) Lowest (0.0–0.9) 13.4 7.4 11.5 17.7 Mid-low (1.0–3.2) 14.9 7.6 20.7 12.3 Mid-high (3.3–5.6) 20.9 13.1 15.8 29.4 Highest (5.7–9.8) 50.8 71.9 51.9 40.6 Note: The “other” race/ethnicity group (n = 106) is not shown. Los Angeles County For the substance abuse safety net in Los Angeles County, we focus on both Proposition 36 contract providers and other alcohol- and drug-treatment providers. In addition, we indicate on our maps which facilities represent the community assessment service centers (CASCs)5 and which are responsible for assessing treatment needs and triaging individuals to Proposition 36 services. For Los Angeles County, we show the substance abuse safety net overall and then concentrate on the southern half of the county, where the majority of the population is located. Overall, the distribution of Proposition 36 and other treatment providers seems to be generally near the majority of the concentrations of parolees shown in Figures 6.16 and 6.17. However, we see sparser coverage in SPA 8/District 4 (South Bay/Carson) and SPA 7/District 4 (North Long Beach/Huntington Park). Although the CASCs focus primarily on the Proposition 36 population, these assessment centers appear to be located relatively near concentrations of parolees throughout the county.6 With respect to the PSN, there are eight PSN providers7 in Los Angeles County (Figure 6.18). These providers represented a treatment capacity in FY 2004 of approximately 36 residential beds, 72 nonresidential slots, and 12 SLE beds. The PSN providers are sparsely located and, for the most part, not close to the areas with the highest concentration of parolees. The eastern section of the county around Pomona and the northern half (District 5) do not have PSN providers. The comparison is starkest for the SPAs. SPA 6, which has one of the highest concentrations of parolees, does not have any PSN providers, nor do SPAs 1 and 3. The limited number and capacity of these facilities suggest that many parolees instead must rely primarily on Proposition 36 and other substance abuse treatment resources in the county. 5 The CASCs provide screening, comprehensive assessment, and referral services for individuals seeking assistance for their alcohol and other drug problems. 6 There are a total of 19 CASCs in Los Angeles County, although only 18 are shown in Figures 6.16 and 6.17. This is because one of the CASCs is also a PSN provider. Therefore, we mapped this one facility as a PSN provider (see Figure 6.18). 7 The map shows only seven PSN provider locations in Los Angeles County because two are very close together. 128 Understanding the Health Implications of Prisoner Reentry in California, Phase I Report Figure 6.16 Map of Proposition 36 and Other Alcohol- and Drug-Treatment Providers, by Supervisorial District, Los Angeles County Counties’ Capacity to Meet the Mental Health and Substance Abuse Treatment Needs 129 Figure 6.17 Map of Proposition 36 and Other Alcohol- and Drug-Treatment Providers, by Service Planning Area, Los Angeles County 130 Understanding the Health Implications of Prisoner Reentry in California, Phase I Report Figure 6.18 Map of PSN Providers, by Service Planning Area, Los Angeles County Counties’ Capacity to Meet the Mental Health and Substance Abuse Treatment Needs 131 As for the other counties, we calculated an accessibility measure only for Proposition 36 providers and the other alcohol- and drug-treatment providers in Los Angeles County. We did not calculate a measure of accessibility for the PSN providers because they are relatively few in number. The accessibility pattern that we see for Proposition 36 and other alcohol- and drug-treatment providers is similar to what we saw for mental health providers in Los Angeles County. Figure 6.19 shows that many areas of the county have relatively high levels of accessibility to these providers (except for the parolee concentrations in the San Fernando Valley), compared to the low levels of accessibility to mental health providers. However, consistent with other findings, after taking into account the underlying population demand for these services, the parolee concentrations in SPA 6 (South Los Angeles; District 2, not shown) have some of the lowest levels of accessibility to alcohol- and drug-treatment providers. Table 6.7 summarizes the accessibility of parolees in Los Angeles County to alcohol- and drug-treatment services. Based on the location of the population-weighted centroid, census tracts in the lowest quartile have, at most, 0.9 facilities within a 10-minute drive per 10,000 individuals under the FPL. By comparison, the census tracts in the highest quartile have at least 2.7 times as many facilities (2.4). For Los Angeles County, there is considerably more variability in accessibility across census tracts in the highest quartile. Figure 6.19 Map of Proposition 36 and Other Alcohol- and Drug-Treatment Provider Accessibility Measure, by Service Planning Area, Los Angeles County 132 Understanding the Health Implications of Prisoner Reentry in California, Phase I Report Table 6.7 Distribution of Parolees Across Proposition 36 and Other Alcohol- and Drug-Treatment Provider Accessibility Quartile Categories, by Race/Ethnicity, Los Angeles County (percent) Total (n = 35,710) African-American (n = 12,885) Latino (n = 14,921) White (n = 6,671) Lowest (0.0–0.9) 16.3 15.1 16.5 18.0 Mid-low (1.0–1.4) 27.7 32.0 28.4 18.5 Mid-high (1.5–2.3) 29.2 31.9 28.1 26.7 Highest (2.4–147.1) 26.7 20.9 26.9 36.9 Level of Accessibility (quartile) Note: The “other” race/ethnicity group (n = 1,233) is not shown. Comparing accessibility to alcohol- and drug-treatment providers by race/ethnicity, we see that, overall, 56 percent of parolees fall into the two highest accessibility categories (Table 6.7). We see some differences in substance abuse accessibility by race/ethnicity. The share of African-American (53 percent) and Latino (55 percent) parolees who reside in areas with the two highest levels of accessibility is about 10 percentage points lower than that of white parolees (64 percent). In summary, the results for Los Angeles County show that, overall, the distribution of Proposition 36 and other treatment providers seems to be generally near the major concentrations of parolees. And, correspondingly, nearly two-thirds of the concentrations of parolees are in areas with the two higher accessibility levels. However, after accounting for the underlying population demand for these services, and consistent with other findings, we see that the parolee concentrations in District 2/SPA 6 (South Los Angeles) are in areas with lower levels of accessibility to alcohol- and drug-treatment providers. Somewhat fewer African-American and Latino parolees reside in areas with higher levels of accessibility than do white parolees. San Diego County For the substance abuse safety net in San Diego County, we focused on Proposition 36 contract providers and other alcohol- and drug-treatment providers that represent the safety net for the uninsured population. In addition, we examined the distribution of the PSN clinics specific for the parolee population. For San Diego County, we see that, combined, the Proposition 36 providers and other treatment providers are geographically located in a number of areas of the county with parolee concentrations. Even District 2 has a number of treatment providers, as shown in Figure 6.20. With respect to the PSN, there are only two PSN locations in San Diego County (Figure 6.21). These locations represented a treatment capacity in FY 2004 of approximately 50 residential beds, 13 nonresidential slots, 14 SLE beds, and one detox bed. The limited number of these facilities suggests that most parolees must rely primarily, instead, on Proposition 36 and other treatment resources in this county. Counties’ Capacity to Meet the Mental Health and Substance Abuse Treatment Needs 133 Figure 6.20 Map of Proposition 36 and Other Alcohol- and Drug-Treatment Providers, by Supervisorial District, San Diego County 134 Understanding the Health Implications of Prisoner Reentry in California, Phase I Report Figure 6.21 Map of PSN Providers, by Supervisorial District, San Diego County Counties’ Capacity to Meet the Mental Health and Substance Abuse Treatment Needs 135 As with the other counties, we calculated an accessibility measure only for Proposition 36 providers and the other alcohol- and drug-treatment providers in San Diego County. We did not calculate a measure of accessibility for the PSN providers because they are few in number. In comparison to accessibility to mental health providers, we see a similar pattern for substance abuse providers, with one exception. Whereas many of the parolee concentrations in San Diego County appear to have relatively good accessibility to alcohol- and drug-treatment services (as measured by the two darker shades of green in Figure 6.22), the parolee concentrations near Imperial Beach and National City are in areas with the lowest levels of accessibility. Table 6.8 summarizes the accessibility of parolees in San Diego County to alcohol- and drug-treatment services. Based on the location of the population-weighted centroid, census tracts in the lowest quartile have, at most, one facility within a 10-minute drive per 10,000 individuals under the FPL. By comparison, the census tracts in the highest quartile have at least five times as many facilities (5.2). For San Diego County, there is considerably more variability in accessibility across census tracts in the highest quartile. Figure 6.22 Map of Proposition 36 and Other Alcohol- and Drug-Treatment Provider Accessibility Measure, by Supervisorial District, San Diego County 136 Understanding the Health Implications of Prisoner Reentry in California, Phase I Report Table 6.8 Distribution of Parolees Across Alcohol- and Drug-Treatment Provider Accessibility Quartile Categories, by Race/Ethnicity, San Diego County (percent) Level of Accessibility (quartile) Total (n = 9,782) African-American (n = 2,650) Latino (n = 2,867) White (n = 3,671) Lowest (0.0–1.1) 12.0 6.0 16.1 12.9 Mid-low (1.2–2.6) 17.7 15.8 19.5 17.2 Mid-high (2.7–5.1) 25.0 26.8 21.1 26.1 Highest (5.2–15.8 ) 45.3 51.4 43.3 43.7 Note: The “other” race/ethnicity group (n = 594) is not shown. Comparing accessibility to substance abuse providers by race/ethnicity, we see that, overall, 68 percent of parolees fall into the two highest accessibility categories (Table 6.8). We see some differences in substance abuse accessibility by race/ethnicity. A larger share of AfricanAmerican parolees (78 percent) fall into the two highest levels of accessibility, compared to 65 percent of Latino parolees and 70 percent of white parolees. Discussion As we did for the health care safety net, we used a measure of accessibility for the mental health and substance abuse safety nets that was based on a drive time of 10 minutes to a facility. An alternate measure would be the federal health professional shortage area designation of excessive distance: more than 40 minutes of travel time to mental health care (HRSA, undated). We elected to use a shorter drive time for several reasons. First, we were concerned that, because the parolee population is more reliant on public transportation than the general population, proximity to facilities may be important. Second, our goal was to identify variation in how well certain areas are served rather than to designate shortage areas. Finally, our measure of accessibility for the mental health and substance abuse safety nets takes into account more than simple drive time; it also includes the underlying population demand for services, as measured by the percentage of the population below the FPL. We chose to focus on these four counties out of the total 58 counties in California because three of the counties comprise the largest share of parolees and the fourth, Kern County, is a rural county, and we expected that rural counties may face a different set of challenges in providing services to this population. Given this, how do the four counties rank relative to one another in terms of overall accessibility? Table 6.9 shows the median and mean accessibility measures across the census tracts within each county. Interestingly, the ranking based on mean accessibility is identical for substance abuse and mental health, and the rankings remain the same if based on the median census tract rather than the mean across census tracts. Los Angeles County has the lowest mean and median accessibility, followed by San Diego County, then Kern County, and, finally, Alameda County. But interestingly, the range for substance abuse is smaller than for mental health. The mean accessibility for Alameda County is four times that of Los Angeles County for mental health but only 2.5 times higher for substance abuse. Counties’ Capacity to Meet the Mental Health and Substance Abuse Treatment Needs 137 Table 6.9 Overall Average Level of Accessibility in the Four Counties County Alameda Kern Los Angeles San Diego Census Tracts (mean/median) Number of Facilities Within 10-Minute Drive, per 10,000 Individuals Under FPL Mental Health Substance Abuse Mean 4.47 4.31 Median 4.20 3.66 Mean 3.43 3.39 Median 2.20 3.16 Mean 1.12 1.86 Median 0.99 1.43 Mean 1.64 3.07 Median 1.58 2.60 We mapped the locations of facilities with our measure of accessibility representing “potential” versus “realized” access of mental health and alcohol- and drug-treatment services. Our geographic mapping and accessibility measures suggest that the accessibility of parolees to mental health and substance abuse providers varies by county. Table 6.10 summarizes the results of our accessibility measures for the individual counties. In terms of accessibility to mental health providers, a larger share of parolees in Kern and San Diego counties reside in areas with the two lowest levels of accessibility; this is less the case for parolees in Alameda and Los Angeles counties. In terms of accessibility to alcohol- and drug-treatment providers, the opposite is true. Only about a third of parolees in Kern and San Diego counties reside in areas with the two lowest levels of accessibility to alcohol- and drug-treatment providers, compared to between 42 and 44 percent of parolees in Alameda and Los Angeles counties (Table 6.10). Accessibility to mental health and substance abuse resources also varied by race/ ethnicity (Table 6.11). With respect to accessibility to mental health resources, in Los Angeles and Alameda counties, a larger share of African-American and Latino parolees fell into the two lowest accessibility categories than did white parolees. However, in Kern County, the share of African-American parolees who resided in areas with the lowest levels of accessibility was smaller than for the other two groups. We found no difference in accessibility to mental health resources by race/ethnicity in San Diego County. Accessibility to substance abuse providers also varied by race/ethnicity. In Kern County, only 15 percent of African-American parolees resided in areas with the lowest accessibility, as compared to only 32 percent of Latino parolees and 30 percent of white parolees. San Diego was similar, with 22 percent of African-Americans residing in areas with the lowest accessibility, compared to 36 percent of Latinos and 30 percent of whites. In Los Angeles County, 47 percent of African-American and 45 percent of Latino parolees, compared to 37 percent of white parolees lived in the lowest accessibility census tracts. In Alameda County, the share of parolees residing in areas with the lowest accessibility to substance abuse providers was slightly higher for African-Americans (44 percent) relative to Latinos (38 percent) and whites (39 percent). 138 Understanding the Health Implications of Prisoner Reentry in California, Phase I Report Table 6.10 Summary of Accessibility Results for Mental Health and Alcohol- and Drug-Treatment Providers Percent of Parolees Who Fall into Two Lowest Accessibility Categories County Mental health providers Alameda 53.1 Kern 26.5 Los Angeles 51.3 San Diego 37.7 Alcohol- and drug-treatment providers Alameda 41.5 Kern 28.3 Los Angeles 44.0 San Diego 29.7 Table 6.11 Summary of Mental Health and Alcohol- and Drug-Treatment Accessibility Results, by County, Type of Facility, and Race/Ethnicity Percent of Parolees by Race/Ethnicity Who Fell into Two Lowest Accessibility Categories County African-American Latino White Mental health providers Alameda 57.1 51.8 37.9 Kern 20.1 29.7 26.3 Los Angeles 56.5 49.3 47.1 San Diego 37.8 35.9 38.4 Alcohol- and drug-treatment providers Alameda 44.0 38.1 39.0 Kern 15.0 32.2 30.0 Los Angeles 47.1 44.9 36.5 San Diego 21.8 35.6 30.1 Defining the mental health and substance abuse safety nets was challenging because each county varies in terms of how its mental health and alcohol- and drug-treatment systems are organized. We used as a proxy measure for the mental health care safety net for the uninsured population: the MHSA providers in a county or Medi-Cal contract providers, as in the case of Kern County. We also included from the SAMSHA record locator files other community organizations that may provide mental health services. Similarly, for the substance abuse safety net, we relied on the lists of Proposition 36 contract providers and other county alcohol- and Counties’ Capacity to Meet the Mental Health and Substance Abuse Treatment Needs 139 drug-treatment resources. For both mental health and substance abuse, we used the safety net for the uninsured population as an upper-bound estimate of the potential safety net available to the parolee population. The POCs serve as the first component of the mental health safety net for parolees, and the PSN serves as the first component of their alcohol- and drug-treatment safety net. Thus, there are specific networks of mental health and substance abuse providers for parolees, unlike what we saw for primary health care and hospital care (for which parolees likely go to the same facilities as other uninsured and low-income populations). POCs provide basic mental health services to parolees, and parolees who may need more intensive care can be referred to county mental health clinics or free clinics. In the case of PSN providers, this network appears to focus on parolees who are identified by the prison treatment program or parole agents as needing more intensive substance abuse services. Access to PSN providers is determined primarily by the prison treatment program and the parole agent. In general, both the POCs and the PSN represent much sparser safety nets for the parolee population than what is generally true for the under- and uninsured populations in these counties. Examining the treatment capacity of the PSN in the four counties suggests that some parolees may not reside in areas where PSN services are readily available (as summarized in Chapter Four).8 Although an important program, it is clear that the PSN program in the four counties addresses the alcohol- and drug-treatment needs of only a fraction of parolees. Further, the focus of the PSN is on counseling and recovery services; it does not include specialized programs, such as narcotic replacement therapy. Yet, a third of the prison population in the BJS survey reported methamphetamine use. This has implications for the parolee population that are not evenly distributed by race/ethnicity. Methamphetamine was particularly a problem for white prisoners (half reported methamphetamine use) and Latino prisoners (a third reported methamphetamine use), as compared to only 7 percent of African-American prisoners. Instead, Proposition 36 and county alcohol- and drug-treatment programs appear to represent important potential resources for the parolee population. Table 6.12 illustrates for Los Angeles County the total number of parolee admissions to Proposition 36 programs and other county alcohol- and drug-treatment programs in FY 2007–2008. Under Proposition 36, parolee admissions were highest for outpatient counseling and day-care habilitative services.9 With respect to other county alcohol- and drug-treatment programs, however, parolee admissions were highest for residential services as well as for outpatient counseling. Finally, in some ways, one could argue that proximity to residential treatment programs (whether for mental health or substance abuse) may be less important than proximity to outpatient mental health and substance abuse treatment services (and, for that matter, health care services). With respect to substance abuse treatment, for example, it may in fact be desirable to remove parolees from the environment and influences that may hamper their recovery process. We did not differentiate between outpatient and residential treatment programs, with some providers providing both types of services. Nevertheless, our analysis shows that, with respect to mental health and substance abuse treatment services, the parolee population must rely primarily on county and community resources. 8 We did not examine the availability of self-help counseling services, such as Alcoholics Anonymous, Narcotics Anonymous, or other such programs. 9 Day-care habilitative services are nonresidential programs that provide counseling and rehabilitative services to individuals who are eligible for Medi-Cal. 140 Understanding the Health Implications of Prisoner Reentry in California, Phase I Report Table 6.12 Los Angeles County Parolee Admissions to Proposition 36 and Other County Alcohol- and Drug-Treatment Services, FY 2007–2008 Type of Service Parolee Participant Admissions in FY 2007–2008 Proposition 36 programs Residential services 281 Residential detoxification Outpatient counseling 43 1,046 Day-care habilitative services Total 25 1,395 Other county alcohol- and drug-treatment programsa Residential services 1,002 Residential detoxification 218 Outpatient counseling 888 Day-care habilitative services Total 25 2,133 Source: County of Los Angeles Department of Public Health, Alcohol and Drug Program Administration, Los Angeles County Participant Reporting System data on parole admissions in FY 2007–2008, provided to the authors on February 23, 2009. a This estimate excludes specialized programs, including drug courts, female offender programs, Proposition 36, and the PSN program. Finally, a potential limitation of our measures of accessibility for the mental health and substance abuse safety nets is that we were unable to take into account the capacity of these facilities as we did for the hospitals and PCCs in Chapter Five. Therefore, for mental health care and substance abuse treatment, we cannot assess the sensitivity of our accessibility measure to alternative specifications. Chapter Seven Summary and Implications Introduction We began this report noting the apt title of Jeremy Travis’s 2005 report on prisoner reentry, But They All Come Back. As we noted, Travis was writing about the nation as a whole, but the title is certainly true for California. We would modify this phrase to say that they all come back and many bring with them a complex set of physical health, mental health, and alcohol- and drug-treatment needs that the state, counties, and local communities must address. The recent attention that prisoner reentry has received at the state and federal levels and the critical need to improve the care provided in California’s correctional health care system provide an important opportunity for California to address the public health implications of reentry and to develop and refine strategies at the state and local levels to meet this challenge. Recognizing the scope of the problem is an important first step in understanding how prisoner reentry disproportionately affects counties, communities, and neighborhoods, as well as in understanding the implications for the health care safety nets on which parolees will likely rely. Phase I of our study is aimed at understanding what could be learned from examining the distribution of parolees and the types of communities in which they reside, in light of their characteristics and health care needs. It also serves as an initial overview of California’s healthrelated safety nets vis-à-vis the needs of the parolee population. This report represents one of the first systematic attempts to define the health care safety net for the reentry population and to assess California communities’ ability to meet the health care needs of parolees, especially in those communities with high concentrations of parolees. An innovation of this study was the development and implementation of accessibility measures that take into account the underlying demand for safety-net health care services, the capacity of those facilities to meet this demand, and the distance that a parolee would have to travel to access services. Most mapping of health care resources for parolees or other specialized populations has tended to look solely at the geographic proximity of services, but proximity alone does not take into account the capacity of those facilities to meet parolees’ needs and the underlying demand by other vulnerable populations for those same services. Our intent was to provide California communities and counties with a rich picture of how prisoner reentry looks in their localities from a public health perspective. Our hope is that health care and public health providers, community organizations, corrections and other public safety officials, and policymakers—as well as other stakeholders concerned with reentry—can use this information to inform their own understanding of these issues and their planning processes. 141 142 Understanding the Health Implications of Prisoner Reentry in California, Phase I Report In this chapter, we discuss some of the findings from Phase I of the project and discuss their implications for California as its communities explore ways to address the public health implications of prisoner reentry. We conclude with a brief discussion of the next steps in Phase II. Summary of Findings Health Care Needs of the Reentry Population Our analysis of survey data indicated that California prison inmates bear a high burden of chronic diseases, such as hypertension and asthma, and infectious diseases, such as hepatitis and tuberculosis—conditions that require regular use of health care for effective management. Yet, among California inmates who reported a current medical issue, a substantial share do not report having seen a physician since their admission to prison. The share is even greater among Latino prisoners. This suggests that prisoners returning to California communities bring with them a number of unmet health needs. But the likelihood of these individuals receiving adequate health care once they are released from prison seems low, given the high rates of uninsurance and other barriers to accessing care and obtaining health insurance or Medi-Cal. Care for communicable and chronic diseases effectively falls under county jurisdiction; therefore, much will depend on a given county’s ability to meet these needs. The substance abuse treatment and mental health care needs of prisoners are even more pronounced than their physical health care needs. About two-thirds of California inmates reported having a drug abuse or dependence problem. Yet, among California inmates reporting drug abuse or dependence, only 22 percent reported receiving treatment since admission to prison, which is lower than the rate for state prisoners nationally. More than half of California inmates reported a recent mental health problem. Some of these mental health issues may be due to particular life experiences. For example, 17 percent of California inmates reported having been homeless at some point, and 16 percent reported a history of sexual or physical abuse. California prisons fare a little better when it comes to mental health care with about half of prisoners receiving treatment in prison among those reporting mental health problems. While this is the same for the U.S. prison population, this figure still suggests that care may not be reaching all those in need. Given the high prevalence of mental health problems and drug abuse and dependence among the prison population, the need for services will likely be particularly high upon their release into the community. Inmates face additional barriers (beyond health care) in reintegrating into the community. For example, inmates may face greater difficulty than the typical Californian in finding employment after their release. Analysis of the BJS survey indicates that California’s prison inmates have substantially lower levels of educational attainment than the general California population and relatively low levels of employment prior to incarceration. These factors may complicate their labor-market prospects, given that these inmates are also locating in areas where the unemployment rate is significantly higher than average. Certain Counties and Communities Are Disproportionately Affected by Reentry Our analysis of the distribution and concentration of parolees across California and within the four counties demonstrated that certain counties are disproportionately affected. And within Summary and Conclusions 143 counties, there are distinct clusters of parolees, which has implications in terms of targeting reentry resources to these areas. Our within-county analysis focused on four counties in California. These four counties—Alameda, Kern, Los Angeles, and San Diego—accounted for nearly one-third of the parolee population in California. We found that, in Alameda County, five distinct clusters of parolees concentrated primarily around Oakland and the northern section of the county accounted for almost 45 percent of the parolee population. In Kern County, there are four distinct clusters of parolees concentrated around the urban area of Bakersfield, along with two others in the northern and northeastern sections of the county. These clusters accounted for almost 58 percent of parolees in this county. Unlike in other counties, we see that there are many more clusters in Los Angeles County. We identified 23 distinct clusters of parolees covering a large geographic area, but they accounted for only 35 percent of the total 35,710 parolees who returned to this county. This dispersion suggests that providing services to the reentry population requires a targeted approach in the various supervisorial districts and service planning areas (SPAs). In San Diego County, eight clusters account for nearly half of the parolee population, with the largest located in the areas of downtown San Diego and Southeast San Diego. Access to Safety-Net Resources Varies Substantially Our geographic mapping and accessibility measures suggest that parolees’ access to health care resources varies by facility type, by geographic area (across counties and within the county), and by race/ethnicity. One issue that stands out is that, in all three of the large urban counties (Alameda, Los Angeles, and San Diego), the majority of parolees resided in areas with the lowest levels of accessibility to general acute care hospitals. The largest share of parolees residing in the lowest accessibility areas was in Alameda County. In all of the counties, community clinics appeared to play an important role in filling gaps in coverage by medically indigent service providers (MISPs) vis-à-vis the reentry population. Still, our examination of all four counties shows that some important geographical gaps in health care resources remain in terms of the distribution of parolees. The most prominent gaps appear to be in Los Angeles County, where certain supervisorial districts and SPAs with high concentrations of parolees had sparse hospital and clinic resources. The most striking example is SPA 6, which covers the area called South Los Angeles. Accessibility to health care resources also varied by parolees’ race/ethnicity. For example, in terms of accessibility to hospitals, in Los Angeles and Alameda counties, more African-American parolees resided in areas with lower accessibility than did Latino or white parolees. However, in Kern and San Diego counties, more Latino parolees resided in areas with lower accessibility to hospitals than did white or African-American parolees. With respect to mental health resources, a larger share of parolees in Kern and San Diego counties fell into the two lowest accessibility categories than was the case in Alameda and Los Angeles counties. In terms of substance abuse treatment accessibility, about a third of parolees in Kern and San Diego counties fell into the two lowest accessibility categories, compared to between 42 and 46 percent in Alameda and Los Angeles counties, respectively. Accessibility to mental health and substance abuse resources also varied by race/ethnicity. For example, in Kern County, 15 percent of African-American parolees resided in areas with lower levels of accessibility to alcohol- and drug-treatment resources, compared to only 32 percent of Latino parolees and 30 percent of white parolees. San Diego County was similar, with 22 percent of 144 Understanding the Health Implications of Prisoner Reentry in California, Phase I Report African-Americans residing in areas with the lowest accessibility, compared to 36 percent of Latinos and 30 percent of whites. In Los Angeles County, 47 percent of African-American and 45 percent of Latino parolees, compared to 37 percent of white parolees, resided in areas with lower accessibility. In Alameda County, the share of parolees residing in areas with the lowest accessibility to substance abuse providers was slightly higher for African-Americans (44 percent) relative to Latinos (38 percent) and whites (39 percent). For mental health care and substance abuse treatment, there were also separate networks dedicated to providing services to the parolee population, and they served as this population’s initial safety net. However, both networks have very limited capacity,, which suggests that many parolees may not be receiving these services and so instead must rely on resources available to the uninsured and low-income populations in a county. Implications for Policymakers and Practitioners Our analysis of the distribution and concentration of parolees in California showed that there are distinct clusters across the state and that, within counties, parolees tend to cluster in certain communities and neighborhoods. Such clustering has implications in terms of developing strategies to provide health care services and other resources to parolees and to better link this population to needed services. The fact that parolees in more rural counties tend to be more dispersed suggests that a different strategy for providing health care services to these individuals is needed. In addition, Los Angeles County (the county with the largest proportion of parolees) is a combination of both urban and more sparsely populated areas. Los Angeles County had a large number of distinct clusters of parolees, with these clusters covering a broad geographic area. This suggests that strategies for providing services to the parolee population will need to be tailored by supervisorial district and SPA. In California, African-American and Latino parolees, in particular, tend to return to disadvantaged neighborhoods and communities, defined by high poverty rates, high unemployment rates, low educational attainment, and a higher share of households in which English is not the primary language. This suggests that reentry in these communites will be even more challenging in that the constellation of parolees’ needs for health care, housing, employment, and other services will be harder to meet. How do policymakers, public health and public safety officials, and communities begin to prioritize their efforts? When it comes to physical health conditions, we found that prisoners bear a high burden of chronic diseases, such as hypertension and asthma, and infectious diseases, such as hepatitis and tuberculosis—conditions that require regular use of health care to manage effectively. The chronic nature of these diseases suggests that access to care will be important, yet, in general, many parolees return to disadvantaged areas where there may be few community health care resources available. We also found that mental health care and substance abuse treatment needs appear to be even more pronounced than California prisoners’ physical health needs, suggesting that this is an important area on which to focus. Our analysis showed that there are three potential safety nets for the parolee population. Particularly in the area of mental health care and substance abuse treatment services, the safety net is made up of a patchwork of different networks of service providers. For parolees and com- Summary and Conclusions 145 munity organizations trying to serve this population, navigating this patchwork of services is likely a complex and challenging task. For example, the substance abuse treatment safety net encompasses distinct networks of providers that are contracted by either the state or counties to provide services. However, these networks tend to be small relative to the overall size of the parolee population. The Parolee Services Network (PSN) is an important means of providing alcohol and drug treatment to exoffenders upon their release from prison. However, funding for the PSN has remained fixed for a number of years while the size of the parolee population has increased. The Substance Abuse Coordinating Agencies (SASCA) also provide access to alcohol- and drug-treatment services, and, like the PSN, coordinate community-based treatment providers to provide services to the reentry population. However, similar to the PSN, SASCA covers only a small percentage (approximately 4 percent) of those released from prison (Walden House, undated). Although both the PSN and SASCA are important, it appears that Proposition 36 and other county alcohol- and drug-treatment services form the larger safety net for many parolees at the local level. There are also some limitations, however, given that Proposition 36 covers only parolees who re-offend and who are nonviolent drug offenders. Clearly, there is a need to consider how to better integrate these different networks of substance abuse services and assess where greater investment may be needed. Successful recovery requires positive coping skills, such as identifying “triggers,” organizing the day, and avoiding negative situations. Strong social and family support is an immeasurable advantage that many addicted offenders do not enjoy. Instead, they suffer weakened family ties and long-standing psychosocial problems that put them at great risk for relapse by virtue of their extreme socioeconomic deficits, exposure to drug-using associates, and other high-risk situations (Gorski et al., 1993). Relapse increases the recidivism risk for technical violations and new offenses, so it is not surprising that research shows that drug and property offenses are a key reason for the high recidivism rate in California. Investing in improving access to substance abuse treatment services in communities where parolees concentrate may yield long-term benefits when it comes to improving the chances of successful reentry, including positive treatment and criminal justice outcomes. Policymakers may also consider ways to improve access to county alcohol- and drug-treatment services. The CASC model adopted by Los Angeles County as part of Proposition 36 may be a useful way to assist parolees and community organizations in assessing treatment needs and triaging parolees to appropriate services. Like the substance abuse safety net, the mental health safety net also comprises a patchwork of networks that represent potential access for the reentry population. County mental health services and county contract services make up a large component of the potential safety net for the reentry population. In addition, Parole Outpatient Clinics (POCs) represent an important initial source of mental health care for parolees and, at least in one county we studied, serve as the gatekeepers to accessing county mental health services. Coupled with prerelease assessment, POCs have been shown to contribute to lower recidivism rates among mentally ill offenders (Farrabee, 2006). However, we found that there are relatively few POCs within counties and that many parolees have to travel far to access these services. Further, anecdotal information1 raises concerns that some POCs may see cutbacks in staffing, given the current economic crisis. 1 Personal communication, January 13, 2009. 146 Understanding the Health Implications of Prisoner Reentry in California, Phase I Report It is also important to assess policy and institutional barriers that may prevent access to needed services. One area worth assessing is the role of POCs as the gatekeepers to county mental health services. From a health services perspective, this may undermine parolee reentry efforts, given that some POCs are understaffed and have long waiting lists. Moreover, anecdotally, it appears that there is a strong disincentive for parolees to report mental health problems to their parole officer for fear of being labeled as a troublemaker or at high risk of recidivism. We will examine these types of policy and institutional barriers in more depth as part of the second phase of our study. With respect to the health care safety net, we found much variability in potential access to clinics and hospitals across and within counties. An important finding was the role that community clinics play in filling in geographic gaps in the safety net. Clearly, one strategy to improve accessibility in those areas with high concentrations of parolees would be to fund more community clinics. At the same time, in the current economic crisis, we expect safetynet providers to become even more stressed. As California considers options for reducing correctional costs by releasing more parolees or offenders without conditions of parole, the health care impact of these decisions will be important to assess. We expect that community clinics and hospitals, particularly in areas with high concentrations of parolees and where there are few other community resources, will be especially impacted. Strengths and Limitations of Our Approach This study represents a first attempt to examine in-depth the needs of the reentry population, identify communities with greater clustering of parolees, define the health care safety net for this population, and, finally, develop measures that summarize the accessibility of safety-net resources in communities where parolees are concentrated. Key innovations of this study include our efforts to define the safety net for parolees and develop measures of accessibility to various health care resources, including hospitals, clinics, mental health care, and substance abuse facilities. In defining the safety net for parolees, we focused on identifying the facilities that are likely to serve this population (e.g., MISP hospitals and clinics), rather than the facilities that are unlikely to be truly available to a largely disadvantaged population. In developing our accessibility measures, we made an effort to take into account the resources available at the relevant facilities (i.e., their capacity), the external demands imposed on them by the broader community, and distance. Mapping parolee clusters in the context of the safety net and their accessibility to health care resources is an important step not only in helping policymakers and practitioners understand the public health implications of reentry, but also in helping them best allocate and fund resources for this population. For example, we included in our maps supervisorial districts and, in the case of Los Angeles County, SPAs, to place these results in both a policy- and health-planning context. Our results serve to highlight which supervisorial districts in the four counties (and SPAs in Los Angeles County) are disproportionately affected by prisoner reentry and identify which of these areas have lower levels of accessibility to health care resources. We validated our approach to measuring accessibility by comparing how well our measures correlate with measures of medically underserved areas and populations in California. These results provide us with confidence that our measures are fairly robust and can serve as a useful planning tool. Summary and Conclusions 147 While we believe our approach and findings can inform policy and planning, we recommend the following steps to further enhance our understanding of the public health implications of prisoner reentry: • We recommend using the street addresses of parolees for more precision in identifying concentrations of parolees. More detailed data would allow policymakers to fine-tune their understanding of which communities are disproportionately affected by reentry and the health care capacity of these communities.2 • To better understand the health care needs of the parolee population and inform planning, we recommend undertaking a survey of inmates who are about to be released and tracking them over time. • We recommend improving data collection on capacity measures for the hospitals, clinics, and substance abuse, mental health, and other relevant facilities that represent the potential safety net for parolees, which will, in turn, improve the accuracy of accessibility measures. • We recommend establishing an expert panel to make recommendations on how to refine the definition of the safety net for this population to help policymakers better understand and facilitate care. Next Steps In the conceptual framework we discussed in Chapter Four, it is clear that health care access outcomes for the reentry population include both potential access and realized access. Potential access outcomes include the ones we measured here, in the first phase of our study, in terms of the accessibility and capacity of safety-net providers. Realized access outcomes include whether parolees actually access care in the safety-net facilities based on such measures as primary care visits, hospital and emergency department visits, preventive screening, infectious disease treatment and monitoring, psychiatric and drug treatment visits, preventable hospitalizations, and unnecessary delays in receiving care. However, for potential access to become realized access, a number of barriers facing parolees (related to policy, institutional, and individual factors) must be overcome. Further, the reentry population is a challenging population to treat, not only because parolees bring with them a complex set of physical, mental, and substance abuse health care needs, but also because they have a unique constellation of other issues, including homelessness, transience, low literacy, and high levels of risk-taking behavior. Combined, these factors have important implications for how health care providers reach out to this population and provide services to them. Phase I relied mostly on analyses of existing survey and administrative datasets and on GIS methods to map parolee clusters and define accessibility. In Phase II of the project, we will further explore the public health implications of prisoner reentry in California. To do so, we will perform in-depth case studies in three of the counties with the greatest concentration of parolees (Alameda, Los Angeles, and San Diego). We will conduct focus groups with newly 2 Although we had originally requested street addresses, the California Department of Corrections and Rehabilitation’s Office of Research elected—for confidentiality reasons—to provide only information on census tract location, zip code, and Thomas Brothers Guide map cell and to omit street addresses from the data file provided. 148 Understanding the Health Implications of Prisoner Reentry in California, Phase I Report released parolees and interview service providers. The focus groups will explore the issues and challenges that parolees face in meeting their health care needs and, in turn, how their health affects their ability to successfully address other basic needs and reintegrate with their families. The interviews with the service providers will explore the challenges that providers face in meeting the needs of the reentry population and the factors that have facilitated or hindered their ability to provide services to this population. Appendix A Parolee Clusters in Four California Counties, by Count of Parolees We mapped the concentration of parolees at the county level in two ways. First, we mapped the concentration of parolees using rate of return overlaid by the secondary clusters. Using rates of returns allowed us to identify which areas or neighborhoods in a county are the most burdened by returning prisoners. Second, we also mapped the concentration of parolees using actual counts overlaid by the secondary clusters. Counts of parolees will be useful for service providers and planners in determining the actual number of parolees in a given area, as shown here in Appendix A. In interpreting the maps showing the counts of parolees—Figures A.1 through A.4—it is necessary to keep in mind that census tracts in more rural areas tend to be larger than those in urban areas. Thus, the concentrations of parolees may appear to be larger in more rural areas. 149 150 Understanding the Health Implications of Prisoner Reentry in California, Phase I Report Figure A.1 Parolee Clusters in Alameda County, by Count of Parolees Parolee Clusters in Four California Counties, by Count of Parolees 151 Figure A.2 Parolee Clusters in Kern County, by Count of Parolees 152 Understanding the Health Implications of Prisoner Reentry in California, Phase I Report Figure A.3 Parolee Clusters in Los Angeles County, by Count of Parolees Parolee Clusters in Four California Counties, by Count of Parolees 153 Figure A.4 Parolee Clusters in San Diego County, by Count of Parolees Appendix B Methods In this appendix, we discuss the methods used in the analyses described in the body of this report. Comparison of Demographic Information for the Data Samples We used three different sources of information about parolees and inmates in our analyses: 1. The California Department of Corrections and Rehabilitation (CDCR) data file of parolees released in calendar years 2005 and 2006 from California state prisons. 2. The CDCR analysis sample we created of parolees with both a valid census tract and zip code during the observation period (2005–2006). 3. The Bureau of Justice Statistics (BJS) 2004 Survey of Inmates in State and Federal Correctional Facilities sample based on all state prison inmates in California (see BJS, 2009). Although we could map the residential census tract of parolees released from California prisons in 2005 and 2006, we had only basic demographic information on this group, such as race/ethnicity and age. We also had information on their criminal history, including their controlling offense, sentence, and previous criminal behavior. In this section, we briefly compare the available demographics and criminal history information for the CDCR parolees to those of the state inmates surveyed by the BJS. Table B.1 summarizes the demographic characteristics of parolees released in 2005–2006, the CDCR analytic sample that we used in our analyses in Chapters Three through Six, and the BJS survey sample that we used in Chapter Two to summarize the health care needs of the reentry population. Across the three data sources, we found that the distribution of parolees by race/ethnicity is similar. About a quarter of the parolees and prisoners are African-American, between 36 and 37 percent are Hispanic or Latino, and a third are white. In terms of gender, males account for roughly 90 percent of each sample. The BJS survey had a smaller percentage of females in its sample; however, this is less of a concern, because our analyses focus specifically on males. Across all three samples, the mean age was 35 years. 155 156 Understanding the Health Implications of Prisoner Reentry in California, Phase I Report Table B.1 Demographic Characteristics of All Parolees, Analytic Sample, and BJS Survey of State Prisoners (percent) Characteristic CDCR All Parolees CDCR Analysis Sample BJS Survey California Prison Inmates County Alameda 4.51 3.42 — Kern 3.61 4.32 — 27.93 26.01 — 6.49 7.11 — White 32.36 33.54 29.55 African-American 25.69 26.32 29.01 Latino 37.64 35.86 37.15 Other 4.31 4. 28 4.29 Los Angeles San Diego Race/ethnicity Gender Male 89.04 88.14 93.61 Female 10.96 11.86 6.39 Violent 23.13 23.14 48.38 Property 33.28 33.55 25.19 Drug 32.23 31.89 20.02 Other 11.37 11.42 6.41 Age in years (mean) 35.12 35.09 35.93 Sentence length (mean) 3.10 3.11 9.07 At least one prior arrest — — 85.81 Controlling offensea At least one prior incarceration Sample size (n) 48.98 49.78 58.14 176,618 137,305 1,757 Note: CDCR sample of all parolees represents the number of parolees released from California state prisons in calendar years 2005 and 2006. The CDCR analysis sample is based on the CDCR parolees for whom we had both a valid census tract and zip code for the observation period (2005–2006). The BJS survey sample is based on all state prison inmates who resided in California. a The controlling offense is the principal offense designated by the court as the base term. For the CDCR samples, the table reflects what is designated in the parolee’s data record. For the BJS survey sample, this measure represents inmates’ self-reported reason for incarceration. We did see some differences between the three samples in terms of average sentence length. For both the CDCR and BJS samples, this variable was length of the sentence imposed. BJS interviewed prisoners during their current incarceration; some prisoners had very long sentences, raising the overall mean for sentence length. Then again, parolees tend to be in and out Methods 157 of prison with shorter sentences, especially for revocations. Also, longer sentences are imposed for more serious offenses and may come with mandatory minimums, so those with longer sentences are likely to serve a higher percentage of their sentence. We also see some differences in the percentage of individuals who fall into the various categories of crime: violent, property, drug, and other. This may be related to the fact that the CDCR samples are based on an individual’s controlling offense, while the BJS sample’s data are self-reported. Finally, the BJS survey sample includes a somewhat higher percentage of individuals with prior incarcerations (58 percent), compared to roughly half of the parolee samples. Again, this may be related to the fact that we are comparing parolee data with data on prisoners who are currently incarcerated. Analysis of 2004 Survey of Inmates in State and Federal Correctional Facilities Although our focus is on understanding the socioeconomic characteristics, health care needs, and access to care of parolees, it is difficult to obtain detailed information on a large and representative sample of parolees nationally and in California. Instead, as a proxy, we used self-reported data from the 2004 Survey of Inmates in State and Federal Correctional Facilities conducted by BJS. All estimates in this report are based on the responses of individuals incarcerated in state prisons. In addition to national estimates, we constructed estimates for California (n = 1,757). Table B.1 summarizes how the survey respondents compare to the data available on parolees released in 2005–2006. Data are also tabulated for racial/ethnic groups in California: white, African-American, Latino, and other. We conducted descriptive analyses of the inmate survey, reporting both lifetime and current health, mental health, and substance abuse problems. To estimate the reported prevalence of symptoms of substance abuse and dependence, we used the methodology developed by BJS (Mumola and Karberg, 2006) for the 2004 Survey of Inmates in State and Federal Correctional Facilities. Survey respondents were categorized as dependent on or abusing drugs or alcohol based on criteria in the Diagnostic and Statistical Manual of Mental Disorders, fourth edition (DSM-IV) (see Appendix C). This report makes the following comparisons and assesses the statistical significance of any differences found between (1) U.S. males and California males and (2) California males by race/ethnicity. We report statistically significant differences (p ≤ 0.05) between U.S and California inmates and, for California only, between white and African-American inmates and between white and Latino inmates. Estimates for other race/ethnicity were often subject to small sample sizes. 158 Understanding the Health Implications of Prisoner Reentry in California, Phase I Report Selection of the Parolee Sample Data on California parolees released in 2005–2006 were obtained from the CDCR.1 Although our original request was for the exact street address of each parolee, the CDCR’s Office of Research elected on confidentiality grounds to provide only information on parolees’ census tract location, zip code, and Thomas Brothers Guide map cell and to omit street address from the file. For our analysis, we focused on the census tract location for the geographic mapping of the distribution of parolees using the population-weighted centroid of each tract as the residential location. Using the census tract was better suited for our purposes than the other variables provided by the CDCR because census tracts are potentially small in urban areas, allowing us to capture any variation that exists, and because they mapped well to other data that we used to characterize the safety net and underlying demand for services in each of the counties. However, without the actual residential addresses of returning CDCR inmates, we could not validate their census tract geocoding or compute other aggregations of the data for cluster analysis or individual accessibility. We elected not to use the Thomas Brothers map cell variable for several reasons: (1) Thomas Brothers map cells are not based on the demographics of the area and can, thus, vary widely in size and composition by county; (2) census tracts are defined based on the demographics of an area and are, on average, smaller than or equal in size to Thomas Brothers map cells; and (3) approximately 40 percent of the sample was missing this information. To create the parolee analytic file, we first combined data from the two files provided by the CDCR. The first file (TERMGRUP) is essentially a log of all the times an individual enters or leaves prison for any reason. Using this file, we determined that 248,833 paroles occurred in 2005–2006. Some individuals were paroled more than once during this period, so we selected only their first 2005–2006 parole, giving us 176,618 parolees. The second file (TERMCASE) includes only records for new court commitments and returns to prison with a new sentence. Revocations of parole with no additional sentence, for example, would be included in the first file but not in the second file. TERMCASE includes information about the initial sentence, prior record, and demographic characteristics. By appending this information to that in the TERMGRUP file, we were able to analyze the demographic and other characteristics of the 2005–2006 parolees. We then utilized data on a log recorded by parole officers on all the places where parolees resided in 2005 and 2006 after being released from prison. From this file, we chose the earliest record in 2005–2006 that included a valid census tract number and appended it to the file created by merging the TERMGRUP and TERMCASE files. Of the total 176,618 cases in our file, 22 percent (n = 39,313) of cases did not have a valid Federal Information Processing Standard (FIPS) code. The FIPS county code is a five-digit code that uniquely identifies counties in the United States, with the first two digits representing the FIPS state code and the last three digits representing the county code. In addition, we used the six-digit FIPS census tract code and the concatenated FIPS codes to identify parolee locations by state, county, and census tract. In this way, we were able to find census tract numbers for 137,305 parolees. To describe 1 Focusing on parolees captured the majority of individuals released from prison. We did not include in our sample those individuals who were unconditionally released from prison (i.e., who served their entire sentence). In a typical year, approximately 97 percent of individuals released from California’s prisons are parolees; only 3 percent are released unconditionally. Methods 159 the characteristics of parolees, we used the entire sample of 176,618 parolees released in 2005 and 2006; however, all our maps are necessarily based on the subset of parolees (n = 137,305) for whom we had valid census tract data. Out of necessity, our analyses exclude the 22 percent of 2005–2006 parolees for whom we did not have valid census tract data. We compared the distribution and characteristics of the parolees who were included in the final analysis file with those who were excluded. Our analysis did not reveal any systematic differences across the two groups in terms of distribution by county or by race/ethnicity, which provides some evidence that the missing data do not undermine our analysis. Another potential source of error in mapping the geographic distribution of parolees is the method used by CDCR to assign census tract numbers. We conducted an analysis of the potential impact error that assigning census tract numbers might have on the geographic patterns we identified (see Appendix E). We concluded that, by including the cases with missing zip codes in our analyses, the overall pattern of clustering was not substantively biased by any potential errors in assignment of census tract information to those cases without zip codes. Many of the figures shown Chapters Five and Six are shaded based on the density of parolees. This, of course, can be done in many ways. We opted to shade each count and census tract based on the rate of returnees. For a county, the rate of returnees is the number of returnees per 1,000 parolees released in California. For a given census tract, we rescaled the measure by the county count. That is, the rate for each census tract is the number of parolees residing in that tract per 1,000 parolees residing in the county. For an alternate perspective, in Appendix A, we provide maps in which counties and census tracts are shaded based on the raw counts of parolees (rather than the rate). Cluster Analysis To identify “clusters” of parolees, using the 2005–2006 parolee data, we assigned parolees to the population-weighted centroids of their census tracts. To do so, we first took the total tracts in California (n = 7,049) and—using the BlockPop points (the block-level points that contain demographic information, with data provided by the software provider ESRI) within each tract—we created a population-weighted tract centroid based on the Pop2000 (2000 census population by block) variable. We used the BlockPop points to weigh the tract centroid based on population density. Using the tract centroids, we then geocoded the parolees using the tract variable, which basically allowed us to map out the parolee points by tract. We then used a nearest neighbor hierarchical (NNH) cluster routine to group parolees into clusters based on spatial proximity. Specifically, we ran these parolee tract centroids through a program called CrimeStat III®, which is used mainly to find data clusters. In conducting the NNH analysis, we set the search radius at 50 percent and the minimum cluster size at 30 (except for Kern County, where the minimum cluster size was set at 20) with one standard deviation.2 That is, we represented clusters on maps using one-standard deviation ellipses of the population-weighted census tract center points. The decision to use the 50percent search radius and minimum size criteria of at least 20 parolees was based on a sensitivity 2 For Alameda, Los Angeles, and San Diego counties, the minimum cluster size was set at 30. Because Kern County is less densely populated, we set the minimum cluster size at 20. 160 Understanding the Health Implications of Prisoner Reentry in California, Phase I Report analysis of cluster results for a limited set of possible criteria. The NNH cluster method identifies clusters regardless of which criteria are chosen. Thus, our aim in using the NNH method was to clearly delineate subcounty areas that include the largest proportion of parolees. To assign names to each of the clusters across California that we had identified with the NNH routine, we used the nearest zip code area to a given cluster. Specifically, we took the Zip Code polygon (also provided by ESRI) and aggregated zip codes based on the zip code area’s name. We then overlaid this with the raw count of parolees by census tract to get a better idea of the locations with the highest density of parolees in each zip code area to resolve ambiguous assignments or situations in which more than one cluster was closest to the same zip code area. To name the clusters at the county level, we used a two-step process: (1) a cluster that was mainly in one zip code area was given that zip name, and, (2) for clusters covering multiple zip code areas, we used the parolee density layer to name the cluster (i.e., if a cluster stretched across multiple zip names and had a relatively equal density of parolees, we used both zip names—e.g., Arvin/Lamont cluster in Kern County). We used a different approach for the Los Angeles zip code area only. We had a number of clusters in the Los Angeles zip code area and so we elected to use the urban neighborhood area name to get a finer naming scheme than the zip code could provide.3 That is, a large segment of the city of Los Angeles falls within a single zip code area; however, there are several officially recognized urban neighborhoods within that zip code area that would allow better precision in describing the location of the clusters (e.g., South Los Angeles, Southeast Los Angeles). Finally, in interpreting the maps showing concentrations of parolees, it is important to note that census tracts in more rural areas tend to be larger in size than those in urban areas. Thus, the concentrations of parolees may appear to be larger in more rural areas. Socioeconomic Analysis For the socioeconomic analysis in Chapter Three, we relied on census data to describe the demographic and socioeconomic characteristics of census tracts in California by race/ethnicity, educational attainment, poverty rate, and degree of linguistic isolation of households. We aggregated the census tracts into seven clusters with similar characteristics. To do so, we used a statistical cluster analysis approach to group the census tracts that were more similar to other census tracts in the same cluster than they were to other tracts outside the cluster. We used a nonhierarchical method known as k-medoids to cluster the tracts. The k-medoids method is an alternative to the more commonly used k-means and has the added benefit of being less influenced by extreme values or outliers within a cluster. The tracts were grouped into a predetermined number of clusters that were usually arbitrarily chosen. We chose seven socioeconomic clusters to keep the number manageable and because we believed that seven was a reasonable number of clusters that could be easily mapped and still convey the distinct characteristics of each cluster. We standardized all variables using the z-score. The k-medoid method involved creating seven clusters and searching for tracts that were similar to the median value for each cluster relative to other clusters. The solution was derived through an optimization algorithm that iteratively changed the cluster median as better values 3 This enabled us to distinguish, for example, between the South Los Angeles and Southeast Los Angeles clusters. Methods 161 were obtained. We used an SAS® statistical software cluster procedure to run the cluster analysis and assign each tract in California to a cluster. Developing Measures of Accessibility To understand the interaction between prisoner reentry and the different types of safety nets beyond simple mapping, we generated quantitative measures of accessibility. These measures took into account the supply of what we considered to be the relevant facilities comprised by the health care safety net and the potential demands placed on it by those who may not have the ability to pay for services. Such measures needed to take into consideration how existing safety-net facilities are geographically distributed and the competition for services based on the socioeconomic context to which parolees return. Several studies have identified the importance of distance to health care services in terms of utilization of those services (Hadley and Cunningham, 2004; Fortney et al., 1999; and Lovett et al., 2002). Thus, to measure the access to care of returning CDCR inmates, we need a measure that would consider the context in which these individuals reside. Moreover, the measure could not be based solely on geographic proximity, as is commonly the case when analysts measure the number of available health care providers and potential demands within some distance threshold or buffer around the area. An assessment of the potential demand placed on a facility should encompass demands from outside the circumscribed area to provide a more accurate picture of accessibility. For instance, accessibility is not only composed of how far one has to travel, but also how long it takes to get an appointment or the wait time once one has arrived at the facility. The key assumption that makes such a measure possible is that, within some threshold distance, residents of an area are more likely to view the nearest facilities as being fully available for their use. If this assumption is valid, then the accessibility of services provided by the facility can be measured as the ratio of supply to demand within a single buffer or catchment. In this view, accessibility is determined by the level of potential demand and the supply—the capacity of the facility—that is available to handle that demand. In reality, this assumption is often an invalid one. Health care facilities are not fully available to serve residents within a circumscribed area or catchment, because they also serve nearby areas that are outside the buffer or catchment for a single tract or catchment. We addressed this in two ways. First, as discussed previously, we looked only at facilities that are likely to provide services to individuals who have difficulty paying or are likely to be uninsured. Second, to encompass the notion of competition for facility services, we needed a method that would allow us to incorporate potential demand from surrounding areas in addition to the buffer area of the tract. The term accessibility refers to the relative ease by which locations of activities, such as work, shopping, and health care, can be reached from a given location (BTS, 1997). Because we did not have access to data that could reveal actual access and utilization of health care services by individuals in the state of California, we settled for a measure of accessibility from a single point in an area—in this case, census tracts. To improve our estimate of the approximate central location of residents, we used a population-weighted geographic center point, or centroid, rather than just the geometric center point of census tracts, as is commonly done. The subtract populations that we used for weighting the centers of tracts were derived from blocklevel 2000 census data. Moreover, to create a measure of accessibility that is easily interpretable 162 Understanding the Health Implications of Prisoner Reentry in California, Phase I Report given the available data, we decided to use an approach that would compute a ratio of supply to potential demand for each census tract in California. Allard (2004) observed that the supply of social services is greater in central city areas where poverty is most concentrated, but the potential demand for such services is also greater. We adopted Allard’s notion of potential demand as the total population living in households with incomes below the federal poverty level (FPL). The method that we used for calculating the accessibility of health care safety-net facilities to census tracts in California is a GIS-based method called the “two-step floating catchment area” (2SFCA) method. The measure is a gravity-type measure in that it incorporates distance, supply, and demand. However, it computes a more easily interpretable measure of accessibility than do traditional gravity models. The 2SFCA approach was initially developed by Radke and Mu (2000) by computing the ratio of suppliers to residents in a prespecified service area centered at each service providers’ location and then summing the ratios for residents living in areas where different providers’ services overlap. Radke and Mu used Euclidean, or straight-line, distances and an overlaying service-area circle to create a set of decomposed areas of overlapping circles. Rather than using Radke and Mu’s decomposition method, we adopted Wang and Minor’s (2002) use of travel-time catchment areas of irregularly shaped polygons and Luo and Wang’s (2003) two-step method to consider all potential demands on a facility as opposed to those only from within the circular catchment area. The method was implemented using ESRI’s ArcGIS™ 9.3 and Network Analyst extension to compute distance matrixes with total travel time in minutes from each population-weighted tract centroid to all health safetynet facilities. The two-step algorithm took the following form: • Step 1: For each safety-net facility, j, search all population locations, k, that are within a threshold travel time, d 0 , from location j, and compute the supply-to-demand ratio: Sj Rj = ∑ { k ∈ d kj ≤ d o } Pk , where Pk is the population of tract k whose centroid falls within the catchment, S j is the capacity or supply measure associated with the facility at location j, and d kj is the travel time between k and j. • Step 2: For each population location, i, search all facility locations, j, that are within the threshold travel time, d 0 , from location i, and sum the facility-to-population ratios, R j , at these locations: AiF = ∑ { j ∈ d ij ≤d 0 } Rj = ∑ { j ∈ d ij ≤d 0 Sj } ∑ { k ∈ d kj ≤d 0 } Pk , where AiF represents the accessibility at resident location i based on the 2SFCA method, R j is the facility-to-population ratio at facility location j, whose centroid falls within the catchment centered at i, and d ij is the travel time between i and j. A larger value for AiF indicates a better accessibility at a location relative to other locations in the study area. Methods 163 We wrote Visual Basic® for Applications scripts to implement the 2FCSA method using ESRI’s ArcObjects application programming interface libraries. Essentially, the method requires computing the distance matrix from all tracts in an area and then selecting all population centroids within the threshold travel time (10 minutes drive time) of each health facility location.4 We calculated the ratio of supply to demand for each facility. Facilities that were missing full-time equivalent (FTE) data were dropped from the analysis. For some facility types (mental health and substance abuse) for which we did not use a capacity measure, we simply counted the facility as a single unit of supply. Thus, the ratio was interpreted as facilities per potential demands. The next step reused the same distance matrix to determine for each population centroid the number of facilities within the threshold travel time. The sum of the supply-to-demand ratios of the selected facilities was the computed accessibility for the tract. The resulting accessibility for the tract should be viewed as an average accessibility measure of all persons within the tract, and it incorporates all demands made on the facility from the surrounding area—not just from the centroid-based buffer area of each tract. Tracts whose buffer areas (within the travel-time threshold) overlapped with others in the catchment of the facility were factored in as well. For example, a facility with a relatively high capacity may be located in tract A with high potential demand. However, it was also within the travel-time threshold of other nearby tracts with high potential demand; thus, the net result was that the accessibility for tract A may be lower because of the competing demands on the facility. In the maps, we summarized the results by quartile, with the lowest quartile (shaded light green on the maps) representing the lowest level of accessibility, and the highest quartile (shaded dark green) representing the highest level of accessibility. The quartiles for the accessibility measures were based on 25-percent intervals of the population demand versus supply in a specific county. The quartiles were equal-sized bins, and each bin had the same number of census tracts. The quartile cutoffs were based on the distribution at the county level and were internal to a particular county. However, the accessibility values (low, medium-low, mediumhigh, and high, as indicated by different shades of green in the accessibility maps) were comparable across counties. In mapping the different types of safety-net facilities, we showed only what was available within a county’s border. The exception was in calculating the accessibility measure for hospitals, in which we included the census tract centroids and hospitals in contiguous/surrounding counties when determining potential demand, because county boundaries are less meaningful in determining access to hospital services. We did not take this approach for clinics because of the challenges in collecting reliable data on these facilities. Also, clinics tend to have smaller catchment areas than hospitals. Therefore, our maps for clinics likely underestimate the accessibility for some areas along the borders of our study counties. Similarly, for mental health and substance abuse treatment facilities, we 4 To construct the 10-minute travel-time areas around both weighted census tract centroids and health care facilities, we used the Network Analyst extension in the ArcGIS Desktop package. The 10-minute travel-time areas were constructed using a digital representation of the street network attributed with characteristics such as posted speed limits and the length of each segment of roadway. Our analysis did not include any measures of traffic congestion or multimodal networks (public transit, rail, or off-road bicycle networks). We used the posted speed limits and length of each road segment to determine the amount of time required to traverse a given road segment. The software used these parameters to construct an area/polygon of the reachable area within a 10-minute travel time from a given destination point. 164 Understanding the Health Implications of Prisoner Reentry in California, Phase I Report took into account only those facilities within a county’s border and, again, may have underestimated the accessibility for some areas along the borders of our study counties. Appendix C Detailed Results Tables Used in Estimating Prevalence of Symptoms of Drug Abuse and Dependence and Mental Health Problems In this appendix, we present detailed results tables used in estimating the prevalence of symptoms of drug abuse and dependence and the prevalence of mental health problems. Table C.1 presents data on the prevalence of symptoms of drug abuse and dependence among inmates based on the DSM-IV criteria and the methodology developed by BJS (Mumola and Karberg, 2006) for the 2004 Survey of Inmates in State and Federal Correctional Facilities (see BJS, undated). The BJS survey included questions designed to measure the prevalence of substance abuse or dependence as defined in the DSM-IV. Abuse symptoms included repeated drug use in hazardous situations or recurrent occupational, educational, legal, or social problems related to drug use. Inmates reporting any of these symptoms in the 12 months prior to their admission to prison met the abuse criteria. Dependence criteria covered a range of symptoms, including behavioral, cognitive, and physiological problems. Inmates reporting three or more symptoms in the 12 months before their admission to prison met the dependence criteria (Mumola and Karberg, 2006). 165 166 Understanding the Health Implications of Prisoner Reentry in California, Phase I Report Table C.1 Symptoms of Drug Abuse and Dependence (percent) By Race/Ethnicity (California) Total U.S. Total California White AfricanAmerican Latino Other Failure to fulfill major role obligations 25.46 30.29 38.96 20.89 32.94 11.85 Continued use in hazardous situations 34.06 37.66 49.29 27.60 37.87 24.81 Drug-related legal problems 22.81 29.88 38.34 22.07 30.86 16.64 Recurrent social or interpersonal problems 43.98 48.40 60.61 39.14 48.25 29.80 Symptom Symptoms of drug abuse Symptoms of drug dependence Tolerance 32.92 38.63 50.66 30.22 37.56 23.17 Withdrawal 27.84 35.55 45.37 22.59 39.85 18.84 Compulsive use 31.35 35.93 45.66 26.42 37.90 16.63 Impaired control 31.44 38.68 46.59 31.34 40.12 22.35 Time spent obtaining, using, recovering 28.87 34.48 44.83 25.98 34.82 18.87 Neglect of activities 25.57 30.42 39.06 22.28 31.72 15.51 Continued use despite problems 34.76 41.61 55.00 32.06 40.83 22.26 Source: BJS 2004 inmate survey. Note: Sample includes male inmates incarcerated in state prisons only. Statistical significance is denoted by bold (California versus United States; race/ethnicity comparisons are African-American, Latino, and other versus white). Detailed Results Tables 167 Table C.2 presents patterns of drug use measured as lifetime prevalence, regular use, and use in the month prior to arrest. It shows both overall use and the use of specific substances by each demographic group. Table C.2 Patterns of Drug Use as Measured by Lifetime Prevalence, Regular Use, and Use in Month Prior to Arrest (percent) By Race/Ethnicity (California) Total U.S. Total California White AfricanAmerican Latino Other 83.24 84.96 90.00 85.48 82.46 69.56 Marijuana/hashish 78.11 77.65 83.11 78.38 74.21 66.12 Cocaine/crack 46.06 48.90 54.78 44.12 50.41 28.19 Heroin/opiates 22.91 28.42 38.35 15.23 32.56 12.95 Depressants 21.02 19.81 34.89 10.39 17.11 4.29 Stimulants 27.94 46.67 72.98 17.95 49.60 34.12 22.79 43.33 68.71 15.59 46.03 32.46 Hallucinogens 32.74 43.58 58.25 33.81 42.07 23.13 Inhalants 13.61 20.27 25.44 9.72 24.23 20.97 2.43 1.65 4.11 0.79 0.59 0.00 69.00 72.64 80.78 72.80 68.25 55.24 Marijuana/hashish 59.80 57.01 61.77 60.94 52.02 42.10 Cocaine/crack 28.99 27.53 29.13 30.16 26.14 11.73 Heroin/opiates 12.60 15.65 18.76 6.12 21.03 10.85 9.66 7.01 13.20 2.91 5.96 1.71 17.26 32.74 55.96 7.37 34.61 28.24 14.25 30.08 51.73 6.84 31.43 26.67 13.45 15.60 17.94 14.53 14.43 17.16 Inhalants 4.55 6.66 9.44 2.35 8.21 3.35 Other drugs 1.05 0.38 1.03 0.27 0.00 0.00 55.77 58.31 64.32 55.31 59.08 31.56 Marijuana/hashish 41.23 36.98 35.65 42.07 36.10 20.09 Cocaine/crack 20.71 15.98 11.39 20.98 16.23 11.73 7.84 9.35 9.67 2.88 14.58 4.95 Type of Drug Lifetime use Any drug Methamphetamine Other drugs Regular use Any drug Depressants Stimulants Methamphetamine Hallucinogens Month before arrest Any drug Heroin/opiates 168 Understanding the Health Implications of Prisoner Reentry in California, Phase I Report Table C.2—Continued By Race/Ethnicity (California) Total U.S. Total California White AfricanAmerican Latino Other 5.15 2.92 6.12 0.80 2.19 1.71 11.73 25.75 43.57 5.27 29.40 9.76 10.40 23.93 40.79 4.75 27.11 9.76 Hallucinogens 6.07 5.45 4.15 7.89 4.83 3.35 Inhalants 1.03 1.78 2.06 1.05 2.14 1.68 Other Drugs 0.59 0.22 0.74 0.00 0.00 0.00 Type of Drug Depressants Stimulants Methamphetamine Source: BJS 2004 inmate survey. Note: Sample includes male inmates incarcerated in state prisons only. Statistical significance is denoted by bold (California versus United States; race/ethnicity comparisons are African-American, Latino, and other versus white). Detailed Results Tables 169 In Chapter Three, we examined the mental health problems presented by inmates. Those designations were based on DSM criteria constructed from inmates’ responses to specific questions about their well-being. Responses to individual criteria are presented in Table C.3. Table C.3 Responses to Individual Questions on Mental Health That Served as Input for DSM-IV Category Assignment (percent) By Race/Ethnicity (California) Symptom Total U.S. Total California White AfricanAmerican Latino Other Major depressive or mania symptoms Persistent sad, numb, or empty mood 31.74 30.44 33.29 32.83 26.83 27.26 Loss of interest or pleasure in activities 34.73 31.89 35.12 37.08 25.56 31.38 Increased or decreased appetite 31.45 27.16 24.98 34.19 24.40 20.12 Insomnia or hypersomnia 38.95 35.87 38.20 39.92 31.32 33.52 Psychomotor agitation or retardation 38.79 38.94 43.60 41.32 33.33 40.85 Feelings of worthlessness or excessive guilt 34.10 33.95 35.36 41.78 26.99 33.67 Diminished ability to concentrate or think 27.55 26.21 29.76 28.38 22.78 18.37 Ever attempted suicide 11.76 11.34 15.33 10.40 8.97 11.67 Persistent anger or irritability 37.00 37.10 41.28 43.75 30.01 27.28 Increased/decreased interest in sexual activities 33.95 32.41 32.52 43.07 24.71 28.82 11.49 11.55 8.60 17.45 9.76 8.31 7.57 10.24 8.85 14.87 8.23 6.78 Psychotic disorder symptoms Delusions Hallucinations Source: BJS 2004 inmate survey. Note: Sample includes male inmates incarcerated in state prisons only. Statistical significance is denoted by bold (California versus United States; race/ethnicity comparisons are African-American, Latino, and other versus white). Appendix D Additional Results for Physical Health and Mental Health Problems In this appendix, we include additional findings on the health problems of California inmates that were not presented in Chapter Two. Here, we present the survey findings for dental problems, reported disability, and mental health treatment for all inmates. Dental Problems Oral health can have long-term implications for physical health. Some studies have reported associations between oral infections and diabetes, heart disease, and strokes (U.S. Department of Health and Human Services, 2000). Dental problems also can affect social functioning; for example, missing teeth can have a negative effect on an individual’s appearance and ability to find employment. According to the Surgeon General’s report Oral Health in America, important disparities remain. Although there have been gains in oral health status overall, non-Hispanic blacks, Hispanics, and American Indians and Alaskan Natives generally have the poorest oral health of any racial/ethnic groups in the United States (U.S. Department of Health and Human Services, 2000). The prevalence of dental problems among the inmate population is substantial (Table D.1). Nationally, half of the surveyed inmates reported a dental problem (50 percent). Reports by California inmates were similar (48 percent). There were no statistically significant differences across racial/ethnic groups among California inmates. Among those who reported a dental problem, California inmates were less likely than prison inmates nationally to have Table D.1 Dental Problems and Treatment Since Admission (percent) By Race/Ethnicity (California) Total U.S. Total California White AfricanAmerican Latino Other Current dental problem 50.41 48.45 50.92 51.91 44.29 45.49 Saw a physician for dental problem (among those reporting current dental problem) 85.68 79.09 79.85 78.50 78.23 85.19 Dental Health Status Source: BJS 2004 inmate survey. Note: Sample includes male inmates incarcerated in state prisons only. Statistical significance is denoted by bold (California versus United States; race/ethnicity comparisons are African-American, Latino, and other versus white). 171 172 Understanding the Health Implications of Prisoner Reentry in California, Phase I Report seen a physician while incarcerated. Among California inmates, there was no statistically significant difference by race/ethnicity in the percentage of individuals with a dental problem or who had received treatment since admission. Disability There is no single definition of disability, but, in general, it refers to any characteristics of the body, mind, or senses that, to a greater or lesser extent, affect a person’s ability to engage independently in some aspects of day-to-day life. In 2000, 18.6 percent of the U.S. population between the ages of 16–64 years had some kind of disability (U.S. Department of Health and Human Services, 2007). Inmates reported a number of physical and other types of disabilities that could limit functioning or require assistance. Overall, more than one-third of inmates reported a disability (35 percent), and about 18 percent considered themselves to be disabled (Table D.2). The most common type of disability reported was impaired sight (10 percent), though other disabilities were also reported: hearing (7 percent), learning (13 percent), speech (4 percent), and mobility (3 percent) impairments. In addition, 18 percent of inmates reported that they required special education. Interestingly, only half as many inmates (18 percent versus 35 percent) reported that they considered themselves disabled. There were no statistically significant differences between U.S. and California inmates, with the exception of special education, which was significantly lower in California (15 percent). But California inmates were nevertheless significantly more likely than all U.S. inmates to consider themselves disabled (21 percent versus 18 percent). Table D.2 Disability Rates (percent) By Race/Ethnicity (California) Disability Any disability Sight Hearing Learning Speech Special education Need aid Consider self disabled Total U.S. Total California White AfricanAmerican Latino Other 34.63 32.87 37.13 35.56 27.64 31.97 10.17 10.00 8.52 12.35 9.56 8.30 6.94 6.34 6.22 5.53 6.60 10.09 13.18 13.44 18.70 13.28 10.04 8.54 3.85 3.81 1.79 4.72 4.13 8.41 18.48 15.18 15.60 18.86 12.67 10.16 2.54 3.38 4.32 4.56 1.97 1.71 17.74 21.21 23.64 27.37 15.14 17.04 Source: BJS 2004 inmate survey. Note: Sample includes male inmates incarcerated in state prisons only. Statistical significance is denoted by bold (California versus United States; race/ethnicity comparisons are African-American, Latino, and other versus white). Additional Results for Physical and Mental Health Problems 173 There were few differences across race/ethnicity in California. Latino males in California were less likely to report a learning disability relative to their white counterparts (10 percent versus 19 percent). Latinos were also less likely to consider themselves disabled (15 percent versus 21 percent). Treatment for Mental Illness The results are qualitatively similar for mental health treatment received by inmates with a previous history of mental illness (see Chapter Two, Table 2.14) and by all inmates (Table D.3). The disparity for Latinos is stronger, but it is likely driven, in part, by a lower prevalence of reported mental illness. Table D.3 Mental Health Treatment Among All Inmates (percent) By Race/Ethnicity (California) Total U.S. Total California White AfricanAmerican Latino Other Received any mental health treatment 29.51 31.35 43.27 33.54 21.45 23.66 Had overnight hospital stay 11.46 10.20 14.05 12.52 5.52 10.12 Used prescribed medications 22.21 23.66 33.56 25.31 15.61 16.92 Had professional mental health therapy 20.95 21.26 30.15 23.03 13.63 16.89 3.50 4.21 5.95 5.79 1.81 3.36 Treatment Ever received treatment Used other mental health treatment Received treatment during the year before or since admission Received any mental health treatment 21.13 22.42 29.75 26.06 14.50 18.60 Had overnight hospital stay 5.15 4.95 6.26 6.79 2.54 5.09 Used prescribed medications 16.42 18.37 25.47 20.65 11.56 15.26 Had professional mental health therapy 13.89 13.48 16.63 16.91 8.67 11.84 2.14 2.46 2.82 3.92 0.98 3.37 Used other mental health treatment Received treatment after admission Received any mental health treatment 17.81 19.15 23.81 23.39 13.03 13.51 Had overnight hospital stay 3.02 3.03 3.60 4.09 1.95 1.68 174 Understanding the Health Implications of Prisoner Reentry in California, Phase I Report Table D.3—Continued By Race/Ethnicity (California) Total U.S. Total California White AfricanAmerican Latino Other Used prescribed medications 13.81 15.92 20.56 18.77 10.87 10.17 Had professional mental health therapy 11.67 11.00 13.57 13.68 7.49 6.75 1.79 2.08 2.04 3.66 0.98 1.68 Treatment Used other mental health treatment Source: BJS 2004 inmate survey. Note: Sample includes male inmates incarcerated in state prisons only. Statistical significance is denoted by bold (California versus United States; race/ethnicity comparisons are African-American, Latino, and other versus white). Appendix E Sensitivity Analysis of Missing Zip Code Data and Alternate Accessibility Measures for Hospitals and Clinics In this appendix, we discuss our approach for conducting our sensitivity analysis of the missing zip code data for alternate accessibility measures for hospitals and clinics. Sensitivty Analysis of Missing Zip Code Data A potential source of error in calculating the geographic analysis results from the method used by the CDCR was in assigning census tract numbers. In creating the 11-digit FIPS code, the CDCR used the zip code as a control variable. For cases that were missing a zip code, the CDCR took the first random location of the street address that came up in its search and assigned it a FIPS. We know that this introduced some error into the assignment of tract location, particularly in cases in which the street name was a commonly used one (e.g., Main Street). To assess how much error this may have introduced into the geocoding of parolee locations, we compared the distribution of parolees in the state by county, mapping cases with a zip code versus cases that were missing a zip code. Overall, 21 percent (n = 29,292) of the geocoded cases were missing a zip code. Figure E.1 shows two maps of the parolee rates of return per 1,000 population by county, calculated using cases with a zip code and then with missing a zip code. If the cases with missing zip code data were concentrated in certain counties, this would suggest possible bias in the pattern identified. Comparing the concentration of parolees across counties using all the geocoded cases (see Figure 3.1, Chapter Three), we see that simply using the cases with a zip code to calculate parolee rates of return would yield a higher estimate for some counties in central and Southern California (Fresno, Tulare, Kern, and San Bernardino counties) and in several smaller counties in Northern California (Yuba, Yola, and Sacramento counties), but it would result in a lower estimate for one rural Northern California county (Humboldt). However, the overall pattern of parolee rates of return in California by county remains the same as the distribution shown in Figure 3.1, in which we included all of the cases that could be geocoded. If we compare the concentration of parolees using the cases that were missing zip code data, we see that the parolee rate of return increases for Alameda County and some of the rural counties in Northern California,1 but it decreases for three Northern California counties (San Joaquin, Madera, and Monterey) and one Southern California county (San Diego). 1 The other Northern California counties were Del Norte, Humboldt, Siskiyou, Butte, Lake, Contra Costa, Solano, and Stanislaus. 175 176 Understanding the Health Implications of Prisoner Reentry in California, Phase I Report Figure E.1 Comparison of Parolee Rates of Return, by County, Using Cases with Zip Code Data Versus Cases Missing Zip Code Data The prevalence of missing zip codes differed across counties. Table E.1 summarizes the results of this comparison to the statewide average for the four counties that are the focus of this study. In our sample, Alameda County had approximately 30 percent of the parolees with missing zip codes, compared to the statewide average of 21 percent. By comparison, San Diego County and Kern County had only 12 percent and 8 percent of parolees, respectively, with missing zip codes. Los Angeles County’s rate of missing zip codes was very similar to the state average, approximately 23 percent. This is not surprising, given that Los Angeles comprises a large share of the state parolees. Table E.1 Distribution of Cases with Missing Zip Codes, State and County Levels Total Parolees Parolees Missing Zip Code Percent of Parolees Missing Zip Code Alameda 4,689 1,421 30 Kern 5,936 499 8 35,710 8,350 23 San Diego 9,782 1,185 12 California 137,305 29,292 21 County Los Angeles Sensitivity Analysis 177 Because Alameda County had 9 percent more cases with missing zip code data than the statewide average, we also were concerned that this might affect the clustering pattern seen in this county. So, we examined whether the 30 percent of parolees with missing zip codes may affect the clusters of parolees identified. Specifically, we looked for differences in cluster patterns in the county, first creating clusters using the cases with zip codes and then overlaying these clusters with clusters created using only the cases that were missing zip codes. We also examined different minimum cluster sizes (15, 20, 25, and 30) to see how the cluster pattern might change. The areas of Alameda County that had cases with missing zip codes were Berkeley, Emeryville, and Oakland.2 We found that, regardless of the cluster size used, the clusters identified in these areas using the cases with or without zip codes overlapped extensively. Thus, we are confident that, by including the cases with missing zip codes in our analyses, the overall pattern of clustering is not substantively biased by any potential errors in assignment of census tract information to those cases without zip codes. Alternate Accessibility Measures for Hospitals and Clinics Our measures of accessibility for hospitals and clinics used FTE as a proxy for a facility’s capacity. Because some hospitals and clinics were missing capacity data, in these instances, we had to drop the facilities from our calculation of the accessibility measures. To assess the effect of missing capacity data, we conducted a sensitivity analysis, examining whether the accessibility patterns within a county changed when using instead a simple count of facilities rather than capacity in calculating an alternate measure of capacity. This is equivalent to an unweighted accessibility measure versus one weighted by capacity. In Figures E.2 through E.9, we present the maps showing the alternate measures using the simple count of these types of facilities in calculating accessibility. 2 The other areas in Alameda County did not have cases with missing zip code data. 178 Understanding the Health Implications of Prisoner Reentry in California, Phase I Report Figure E.2 Map of Primary Care Clinics Using Alternate Accessibility Measure, Alameda County Sensitivity Analysis Figure E.3 Map of Hospitals Using Alternate Accessibility Measure, Alameda County 179 180 Understanding the Health Implications of Prisoner Reentry in California, Phase I Report Figure E.4 Map of Primary Care Clinics Using Alternate Accessibility Measure, Kern County Sensitivity Analysis Figure E.5 Map of Hospitals Using Alternate Accessibility Measure, Kern County 181 182 Understanding the Health Implications of Prisoner Reentry in California, Phase I Report Figure E.6 Map of Primary Care Clinics Using Alternate Accessibility Measure, by SPA, Los Angeles County Sensitivity Analysis Figure E.7 Map of Hospitals Using Alternate Accessibility Measure, by SPA, Los Angeles County 183 184 Understanding the Health Implications of Prisoner Reentry in California, Phase I Report Figure E.8 Map of Primary Care Clinics Using Alternate Accessibility Measure, San Diego County Sensitivity Analysis Figure E.9 Map of Hospitals Using Alternate Accessibility Measure, San Diego County 185 References AddictionsandRecovery.org, “The Medical Definition of Addiction,” Web page, last updated May 9, 2009. As of May 15, 2009: http://www.addictionsandrecovery.org/definition-of-addiction.htm Allard, Scott W., “Access to Social Services: The Changing Urban Geography of Poverty and Service Provision,” Washington, D.C.: Brookings Institution, Center on Urban and Metropolitan Policy, August 2004. American Psychiatric Association, Diagnostic and Statistical Manual of Mental Disorders, 4th ed., text rev., Washington, D.C., 2000. Andersen, Ronald M., “Behavioral Model of Families’ Use of Health Services,” Chicago: Center for Health Administration Studies, University of Chicago, Research Series No. 25, 1968. Andersen, Ronald, and John F. Newman, “Societal and Individual Determinants of Medical Care Utilization in the United States,” Milbank Memorial Fund Quarterly, Vol. 51, No. 1, 1973, pp. 95–124. Bailey, Amanda, and Joseph M. Hayes, “Who’s in Prison? The Changing Demographics of Incarceration,” in Hans P. Johnson, ed., California Counts: Population Trends and Profiles, Vol. 8, No. 1, San Francisco, Calif.: Public Policy Institute of California, August 2006. As of May 21, 2009: http://www.ppic.org/main/publication.asp?i=701 Binswanger, Ingrid A., Mark F. Stern, Richard A. Deyo, Patrick J. Heagerty, Allen Cheadle, Joann G. Elmore, and Thomas D. Koepsell, “Release from Prison: A High Risk of Death for Former Inmates,” New England Journal of Medicine, Vol. 356, No. 2, January 11, 2007, pp. 157–165. BJS—see Bureau of Justice Statistics. BLS—see Bureau of Labor Statistics. Brady, Kathleen T., Marcia L. Verduin, and Bryan K. Tolliver, “Treatment of Patients Comorbid for Addiction and Other Psychiatric Disorders,” Current Psychiatric Reports, Vol. 9, No. 5, October 2007, pp. 374–380. BTS—Bureau of Transportation Statistics. Bureau of Justice Statistics, U.S. Department of Justice, Survey of Inmates in State and Federal Correctional Facilities, 2004, codebook, Ann Arbor, Mich.: Inter-University Consortium for Political and Social Research, undated. ———, “Corrections Statistics,” last updated April 29, 2009. As of May 15, 2009: http://www.ojp.gov/bjs/correct.htm Bureau of Labor Statistics, U.S. Department of Labor, “Employment Status of the Civilian Noninstitutional Population by Sex, Race, Hispanic or Latino Ethnicity, Marital Status, and Detailed Age, 2005 Annual Averages,” 2005. As of May 15, 2009: http://www.bls.gov/lau/table14full05.pdf Bureau of Transportation Statistics, U.S. Department of Transportation, Transportation Statistics Annual Report 1997, Washington, D.C., BTS97-S-01, 1997. As of May 21, 2009: http://www.bts.gov/publications/transportation_statistics_annual_report/1997/ 187 188 Understanding the Health Implications of Prisoner Reentry in California, Phase I Report Cabassa, Leopoldo J., Luis H. Zayas, and Marissa C. Hansen, “Latino Adults’ Access to Mental Health Care: A Review of Epidemiological Studies,” Administration and Policy in Mental Health and Mental Health Services Research, Vol. 33, No. 3, May 2006, pp. 316–330. Cahill, Meagan, and Nancy LaVigne, “Residential Mobility and Drug Use Among Parolees in San Diego County, California, and Implications for Policy,” in Yonette F. Thomas, Douglas Richardson, and Ivan Cheung, eds., Geography and Drug Addiction, New York: Springer, 2009, pp. 85–116. California Department of Alcohol and Drug Programs, “Reports and Documents,” catalog of reports from various years, undated. As of May 15, 2009: http://www.adp.cahwnet.gov/ParoleeServices/reports.shtml ———, “PSN Annual Statewide Summary Report,” 2005–2006. As of May 15, 2009: http://www.adp.cahwnet.gov/ParoleeServices/pdf/PSNAnnualSummaryReport-FY2005-06.pdf ———, “Parolee Services Network,” fact sheet, April 2009. As of May 15, 2009: http://www.adp.ca.gov/FactSheets/ParoleeServicesNetwork.pdf California Department of Corrections and Rehabilitation, “Recidivism Rates within One and Two Year Follow-up Periods for All Felons Paroled to California Supervision California Department of Corrections and Rehabilitation Released from Prison for First Time in 2003,” March 22, 2006. As of January 29, 2009: http://www.cdcr.ca.gov/Reports_Research/Offender_Information_Services_Branch/Annual/RECID2/ RECID2d2003.pdf ———, “California’s One-Year Recidivism Rate the Lowest Since 1979,” press release, April 10, 2006. As of January 29, 2009: http://www.cdcr.ca.gov/News/2006_Press_Releases/press20060410.html ———, Offender Information Services Branch, Prison Census Data as of December 31, 2007, Sacramento, Calif., February 2008. As of May 21, 2009: http://www.cdcr.ca.gov/Reports_Research/Offender_Information_Services_Branch/Annual/Census/ CENSUSd0712.pdf California Department of Finance, California Current Population Survey Report: March 2006, Sacramento, Calif., September 2007. California HealthCare Foundation, “County Programs for the Medically Indigent in California,” Oakland, Calif., August 2006a. As of May 15, 2009: http://www.chcf.org/topics/view.cfm?itemID=123106 ———, Medically Indigent Service Program Profiles, Oakland, Calif., August 2006b (revised November 2006). As of May 15, 2009: http://www.chcf.org/documents/policy/CountyPrgrmsMedicallyIndigentMISP.pdf California Legislative Analyst’s Office, California’s Criminal Justice System: A Primer, Sacramento, Calif., January 31, 2007. As of May 21, 2009: http://www.lao.ca.gov/LAOApp/PubDetails.aspx?id=1543 California Office of Statewide Health Planning and Development, Healthcare Workforce Development Division, “Medically Underserved Areas and Populations,” Web page, December 2007. As of May 21, 2009: http://www.oshpd.state.ca.us/HWDD/MUA_MUP.html California Prison Health Care Services, “About Us,” Web page, undated. As of May 15, 2009: http://www.cphcs.ca.gov/about.aspx CDCR—see California Department of Corrections and Rehabilitation. Council of Community Clinics, “About Council of Community Clinics,” Web page, undated. As of May 15, 2009: http://www.ccc-sd.org/about.aspx Council of State Governments, Criminal Justice/Mental Health Consensus Project, Lexington, Ky., June 2002. As of May 15, 2009: http://consensusproject.org/the_report/downloads References 189 County of Los Angeles Department of Public Health, “Service Planning Areas—What is a SPA?” Web site, undated. As of May 15, 2009: http://www.lapublichealth.org/spa/spawhat.htm Davidson, Pamela L., Ronald M. Andersen, Roberta Wyn, and E. Richard Brown, “A Framework for Evaluating Safety-Net and Other Community-Level Factors on Access for Low-Income Populations,” Inquiry, Vol. 41, No. 1, Spring 2004, pp. 21–38. Davis, Lois M., M. Rebecca Kilburn, and Dana Schultz, Reparable Harm: Assessing and Addressing Disparities Faced by Boys and Men of Color in California, Santa Monica, Calif.: RAND Corporation, MG-745-TCE, 2009. As of May 15, 2009: http://www.rand.org/pubs/monographs/MG745/ Davis, Lois M., and Sharon Pacchiana, “Health Profile of the State Prison Population and Returning Offenders: Public Health Challenges,” Journal of Correctional Health Care, Vol. 10, No. 3, April 2004, pp. 303–331. Durose, Matthew R., and Patrick A. Langan, “Felony Sentences in State Courts, 2004,” Bureau of Justice Statistics Bulletin, July 2007. As of May 21, 2009: http://www.ojp.usdoj.gov/bjs/pub/pdf/fssc04.pdf Expert Panel on Adult Offender and Recidivism Reduction Programming, Report to the California State Legislature: A Roadmap for Effective Offender Programming in California, Sacramento, Calif.: California Department of Corrections and Rehabilitation, 2007. As of January 29, 2009: http://www.cdcr.ca.gov/News/2007_Press_Releases/docs/ExpertPanelRpt.pdf Farabee, David, “Evaluation of California’s Mental Health Services Continuum Program for Parolees,” Corrections Today Magazine, Vol. 68, No. 7, December 2006. pp. 38–41. Fortney, John, Kathryn Rost, Mingliang Zhang, and James Warren, “The Impact of Geographic Accessibility on the Intensity and Quality of Depression Treatment,” Medical Care, Vol. 37, No. 9, September 1999, pp. 884–893. GAO—see U.S. Government Accountability Office. Gardiner, Christine Lynn, From Inception to Implementation: How SACPA Has Affected the Case Processing and Sentencing of Drug Offenders in One California County, doctoral dissertation, University of California, Irvine, July 2008. Gelberg, Lillian, Ronald M. Andersen, and Barbara D. Leake, “The Behavioral Model for Vulnerable Populations: Application to Medical Care Use and Outcomes for Homeless People,” Health Services Research, Vol. 34, No. 6, February 2000, pp. 1273–1302. Gorski, Terence T., John M. Kelley, Lisa Havens, and Roger H. Peters, Relapse Prevention and the SubstanceAbusing Criminal Offender: An Executive Briefing, Rockville, Md.: Substance Abuse and Mental Health Services Administration, Technical Assistance Publication Series 8, 1993. Grattet, Ryken, Joan Petersilia, and Jeffrey Lin, Parole Violations and Revocations in California, Irvine, Calif.: University of California, Irvine, October 13, 2008. As of January 29, 2009: http://ucicorrections.seweb.uci.edu/files/ Parole%20Violations%20and%20Revocations%20in%20California.pdf Hadley, Jack, and Peter Cunningham, “Availability of Safety Net Providers and Access to Care of Uninsured Persons,” Health Services Research, Vol. 39, No. 5, October 2004, pp. 1527–1547. Hammett, Theodore M., Patricia Harmon, and William Rhodes, “The Burden of Infectious Disease Among Inmates and Releasees from Correctional Facilities,” in The Health Status of Soon-To-Be-Released Inmates: A Report to Congress, Vol. 2, Chicago: National Commission on Correctional Health Care, May 2002. As of May 15, 2009: http://www.ncchc.org/pubs/pubs_stbr.vol2.html Hammett, Theodore M., Cheryl Roberts, and Sophia Kennedy, “Health-Related Issues in Prisoner Reentry,” Crime and Delinquency, Vol. 47, No. 3, July 2001, pp. 390–409. 190 Understanding the Health Implications of Prisoner Reentry in California, Phase I Report Health Resources and Services Administration, U.S. Department of Health and Human Services, “Shortage Designation: HPSAs, MUAs and MUPs,” undated. As of May 21, 2009: http://bhpr.hrsa.gov/shortage/muaguide.htm Heiser, Bill, and Junious Williams, Reentry Healthcare in Alameda County, Executive Summary: Initial Assessment and Recommendations of the Alameda County Reentry Task Force, Oakland, Calif.: Urban Strategies Council, May 29, 2008. As of May 15, 2009: http://www.urbanstrategies.org/documents/ReentryHealthTaskForceExecutiveSummary_FINAL_001.pdf HRSA—see Health Resources and Services Administration. Hughes, Timothy, and Doris James Wilson, “Inmates Returning to the Community After Serving Time in Prison,” Characteristics of Releases: Bureau of Justice Statistics Reentry Trends in the U.S., October 2002. Jacob and Valeria Langeloth Foundation, Philanthropic Opportunities in Correctional Health Care, View Associates, 2006. As of May 15, 2009: https://www.langeloth.org/events/Philanthropic%20Opportunities%20in%20Correctional%20Health%20 Care.pdf James, Doris J., and Lauren E. Glaze, Mental Health Problems of Prison and Jail Inmates, Washington, D.C.: Bureau of Justice Statistics, U.S. Department of Justice, September 2006. As of May 21, 2009: http://ojp.usdoj.gov/bjs/pub/pdf/mhppji.pdf Kokkevi, Anna, and Costas Stefanis, “Drug Abuse and Psychiatric Comorbidity,” Comprehensive Psychiatry, Vol. 36, No. 5, September–October 1995, pp. 329–337. Link, Bruce G., Ezra Susser, Ann Stueve, Jo Phelan, Robert E. Moore, and Elmer Struening, “Lifetime and Five-Year Prevalence of Homelessness in the United States,” American Journal of Public Health, Vol. 84, No. 12, December 1994, pp. 1907–1912. Lovett, Andrew, Robin Haynes, Gisela Sünnenberg, and Susan Gale, “Car Travel Time and Accessibility by Bus to General Practitioner Services: A Study Using Patient Registers and GIS,” Social Science and Medicine, Vol. 55, No. 1, July 2002, pp. 97–111. Luo, Wei, and Fahui Wang, “Measures of Spatial Accessibility to Health Care in a GIS Environment: Synthesis and a Case Study in the Chicago Region,” Environment and Planning B, Vol. 30, No. 6, 2003, pp. 865–884. Lynch, James P., and William J. Sabol, Prisoner Reentry in Perspective, Crime Policy Report 3, Washington, D.C.: Urban Institute, September 2001. As of May 15, 2009: http://www.urban.org/publications/410213.html Mallik-Kane, Kamala, and Christy A. Vischer, Health and Prisoner Reentry: How Physical, Mental, and Substance Abuse Conditions Shape the Process of Reintegration, Washington, D.C.: Urban Institute Justice Policy Center, February 2008. As of May 15, 2009: http://www.urban.org/UploadedPDF/411617_health_prisoner_reentry.pdf Maruschak, Laura M., “HIV in Prisons, 2000,” Bureau of Justice Statistics Bulletin, October 2002. As of May 21, 2009: http://www.ojp.usdoj.gov/bjs/pub/pdf/hivp00.pdf McMurray, Harvey L., “High Risk Parolees in Transition from Institution to Community Life,” Journal of Offender Rehabilitation, Vol. 19, Nos. 1–2, 1993, pp. 145–161. Mumola, Christopher J., Substance Abuse and Treatment, State and Federal Prisoners, 1997, Washington, D.C.: Bureau of Justice Statistics, U.S. Department of Justice, January 1999 (revised March 11, 2009). As of May 15, 2009: http://www.ojp.usdoj.gov/bjs/pub/pdf/satsfp97.pdf ———, Incarcerated Parents and Their Children, Washington, D.C.: Bureau of Justice Statistics, U.S. Department of Justice, 2000. As of May 15, 2009: http://www.ojp.usdoj.gov/bjs/pub/pdf/iptc.pdf References 191 Mumola, Christopher J., and Jennifer C. Karberg, Drug Use and Dependence, State and Federal Prisoners, 2004, Washington, D.C.: Bureau of Justice Statistics, U.S. Department of Justice, October 2006 (revised January 19, 2007). As of March 1, 2009: http://www.ojp.usdoj.gov/bjs/pub/pdf/dudsfp04.pdf National Commission on Correctional Health Care, The Health Status of Soon-to-Be-Released Inmates: A Report to Congress, Vol. 1, Chicago, March 2002. As of May 21, 2009: http://www.ncchc.org/pubs/pubs_stbr.vol1.html NCCHC—see National Commission on Correctional Health Care. Newman, Anabel, Prison Literacy: Implications for Program and Assessment Policy, Philadelphia, Pa.: National Center for Adult Literacy, 1993. OSHPD—see California Office of Statewide Planning and Development. Park, Annie, Nancy Watson, and Lark Galloway-Gilliam, South Los Angeles Health Equity Scorecard, Los Angeles, Calif.: Community Health Councils, December 2008. As of May 15, 2009: http://www.chc-inc.org/userimages/South%20LA%20Scorecard.pdf Pauly, Mark V., and José A. Pagán, “Spillovers and Vulnerability: The Case of Community Uninsurance,” Health Affairs, Vol. 26, No. 5, September–October 2007, pp. 1304–1314. Petersilia, Joan, “When Prisoners Return to the Community: Political, Economic and Social Consequences,” Sentencing and Corrections Issues for the 21st Century: Papers from the Executive Sessions on Sentencing and Corrections, No. 9, November 2000. As of May 15, 2009: http://www.ncjrs.gov/pdffiles1/nij/184253.pdf ———, Understanding California Corrections, Berkeley, Calif.: California Policy Research Center, University of California, May 2006. As of May 14, 2009: http://www.ucop.edu/cprc/documents/understand_ca_corrections.pdf Radke, John, and Lan Mu, “Spatial Decomposition, Modeling and Mapping Service Regions to Predict Access to Social Programs,” Geographic Information Sciences, Vol. 6, 2000, pp. 105–112. Regier, D. A., M. E. Farmer, D. S. Rae, B. Z. Locke, S. J. Keith, L. L. Judd, and F. K. Goodwin, “Comorbidity of Mental Disorders with Alcohol and Other Drug Use: Results from the Epidemiologic Catchment Area (ECA) Study,” Journal of American Medical Association, Vol. 264, No. 19, November 21, 1990, pp. 2511–2518. Rural Policy Research Institute, Demographic and Economic Profile: California, Columbia, Mo., July 2006. As of May 3, 2009: http://www.rupri.org/Forms/California.pdf Saviano, Elizabeth C., and Margie Powers, California’s Safety-Net Clinics: A Primer, Oakland, Calif.: California HealthCare Foundation, November 2005. Shuter, Jonathan, “Communicable Diseases in Inmates: Public Health Opportunities,” in The Health Status of Soon-to-Be-Released Inmates: A Report to Congress, Vol. 2, Chicago: National Commission on Correctional Health Care, May 2002. As of May 21, 2009: http://www.ncchc.org/pubs/pubs_stbr.vol2.html Steinhauer, Jennifer, “A City Where Hospitals Are as Ill as the Patients,” New York Times, June 5, 2008. As of February 24, 2009: http://www.nytimes.com/2008/06/05/us/05southla.html Sugita, Wayne K., Acting Director, Alcohol and Drug Program Administration, County of Los Angeles Department of Public Health, “Notice of Intent, Request for Proposals for Parolee Service Networks,” memo May 8, 2008. As of February 2, 2009: http://www.publichealth.lacounty.gov/adpa/grant/NOI-RFPforPSN.pdf Travis, Jeremy, But They All Come Back: Facing the Challenges of Prisoner Reentry, Washington, D.C.: Urban Institute Press, 2005. 192 Understanding the Health Implications of Prisoner Reentry in California, Phase I Report Travis, Jeremy, Elizabeth Cincotta McBride, and Amy L. Solomon, Families Left Behind: The Hidden Costs of Incarceration and Reentry, policy brief, Washington, D.C.: Urban Institute Justice Policy Center, June 2005. As of May 21, 2009: http://www.urban.org/UploadedPDF/310882_families_left_behind.pdf Travis, Jeremy, and Michelle Waul, “Prisoners Once Removed: The Children and Families of Prisoners,” in Jeremy Travis and Michelle Waul, eds., Prisoners Once Removed: The Impact of Incarceration and Reentry on Children, Families, and Communities, Washington, D.C.: Urban Institute Press, 2003. Treviño, Fernando M., and Mario I. Rendón, “Mental Illness/Mental Health Issues,” in Carlos W. Molina and Marilyn Aguirre-Molina, eds., Latino Health in the US: A Growing Challenge, Washington D.C.: American Public Health Association, 1994, pp. 447–475. Tuttle, Rochelle, and Lucien Wulsin, Jr., California’s Safety Nets and the Need to Improve Local Collaboration in Care for the Uninsured: Counties, Clinics, Hospitals, and Local Health Plans, Santa Monica, Calif.: Insure the Uninsured Program, October 2008. As of May 21, 2009: http://www.itup.org/Reports/Statewide/Safetynet_Report_Final.pdf U.S. Census Bureau, The 2008 Statistical Abstract: The National Data Book, Washington, D.C., 2008. As of May 21, 2009: http://www.census.gov/compendia/statab/ ———, State and County Quickfacts, “Alameda County, California,” last updated May 5, 2009a. As of May 15, 2009: http://quickfacts.census.gov/qfd/states/06/06001.html ———, State and County Quickfacts, “Kern County, California,” last updated May 5, 2009b. As of May 15, 2009: http://quickfacts.census.gov/qfd/states/06/06029.html U.S. Department of Health and Human Services, Office of the Surgeon General, Oral Health in America: A Report of the Surgeon General, Rockville, Md.: U.S. Department of Health and Human Services, National Institute of Dental and Craniofacial Research, National Institutes of Health, 2000. As of May 15, 2009: http://www.surgeongeneral.gov/library/oralhealth/ ———,“The Surgeon General’s Call to Action to Improve the Health and Wellness of Persons with Disabilities,” fact sheet, last revised January 4, 2007. As of February 25, 2009: http://www.surgeongeneral.gov/library/disabilities/calltoaction/factsheetwhatwho.html U.S. Government Accountability Office, Drug Offenders: Various Factors May Limit the Impacts of Federal Laws That Provide for Denial of Selected Benefits, Washington, D.C., GAO-05-238, September 2005. As of May 21, 2009: http://www.gao.gov/new.items/d05238.pdf Urban Institute, The Public Health Dimensions of Prisoner Reentry: Addressing the Health Needs and Risks of Returning Prisoners and Their Families, Meeting Summary, National Reentry Roundtable Meeting, Los Angeles, Calif., December 11–12, 2002. As of May 14, 2009: http://www.urban.org/publications/410920.html Visher, Christy A., and Kamala Mallik-Kane, “Reentry Experiences of Men with Health Problems,” in Robert Greifinger, ed., Public Health Behind Bars: From Prisons to Communities, New York: Springer, 2007, pp. 434–460. Walden House, “SASCA,” Web page, undated. As of May 21, 2009: http://www.waldenhouse.org/services/substanceabuse.html Wang, Fahui, and W. William Minor, “Where the Jobs Are: Employment Access and Crime Patterns in Cleveland,” Annals of the Association of American Geographers, Vol. 92, No. 3, September 2002, pp. 435–450. Western, Bruce, Jeffrey R. Kling, and David F. Weiman, The Labor Market Consequences of Incarceration, Princeton, N.J.: Princeton University Industrial Relations Section, Working Paper No. 450, January 2001.