Effects of Private Prison Confinement in MN on Offender Recidivism, MN DOC, 2013
Download original document:
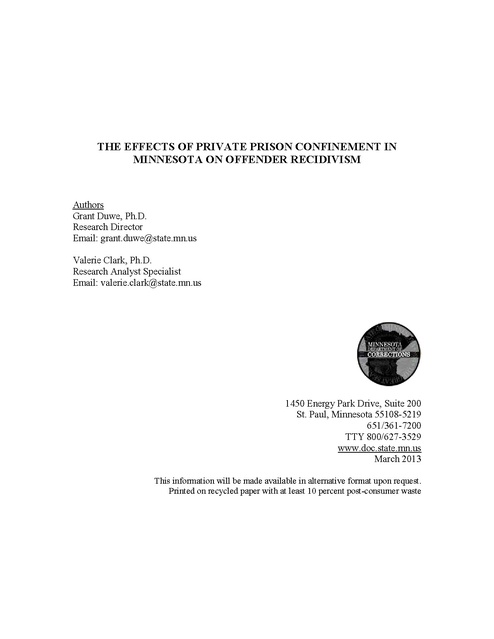
Document text
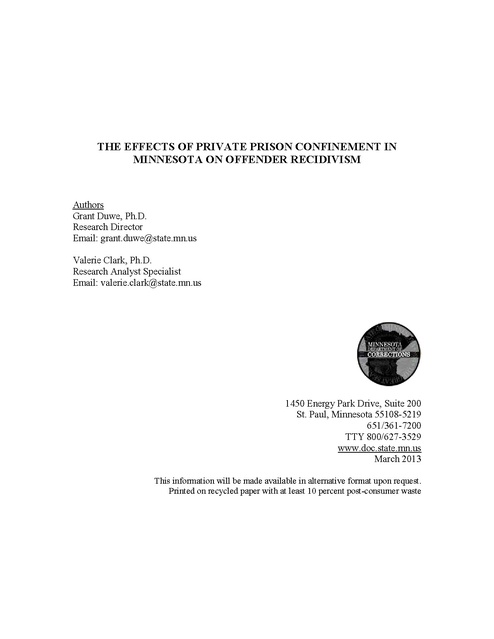
Document text
This text is machine-read, and may contain errors. Check the original document to verify accuracy.
THE EFFECTS OF PRIVATE PRISON CONFINEMENT IN MINNESOTA ON OFFENDER RECIDIVISM Authors Grant Duwe, Ph.D. Research Director Email: grant.duwe@state.mn.us Valerie Clark, Ph.D. Research Analyst Specialist Email: valerie.clark@state.mn.us 1450 Energy Park Drive, Suite 200 St. Paul, Minnesota 55108-5219 651/361-7200 TTY 800/627-3529 www.doc.state.mn.us March 2013 This information will be made available in alternative format upon request. Printed on recycled paper with at least 10 percent post-consumer waste Research Summary Evidence has been mixed as to whether private prisons are more effective than stateoperated facilities in reducing recidivism. This study analyzes whether private prison confinement in Minnesota has had an impact on recidivism by examining 3,532 offenders released from prison between 2007 and 2009. Propensity score matching was used to individually match a comparison group of 1,766 inmates who had only been confined in state-run facilities with 1,766 offenders who had served time in a private prison facility. Using multiple measures of recidivism and private prison confinement, 20 Cox regression models were estimated. The results showed that offenders who had been incarcerated in a private prison had a greater hazard of recidivism in all 20 models, and the recidivism risk was significantly greater in eight of the models. The evidence presented in this study suggests that private prisons are not more effective in reducing recidivism, which may be attributable to fewer visitation and rehabilitative programming opportunities for offenders incarcerated at private facilities. Introduction The number of private prisons has risen dramatically over the past three decades. Private corporations had only a limited role in corrections at the beginning of the 1980s, providing food service and healthcare, among other services (Selman and Leighton, 2010). By 2005, private corporations operated 415 prison facilities in addition to providing other prison services to public facilities (Stephan, 2008). Determinate sentencing practices and the War on Drugs led to unprecedented prison population growth, which outpaced the capacity of U.S. prisons (Selman and Leighton, 2010; Austin and Coventry, 2001). Thus, state and federal correctional agencies looked to private facilities for bed space. Additionally, the political climate during the 1980s fostered a movement towards privatizing many government functions, including corrections (Austin and Coventry, 2001). Between 2000 and 2005, the number of adult correctional facilities rose by nine percent, and private facilities accounted for nearly all of this increase. Of the 153 new prison facilities built during this time period, 151 were private (Stephan, 2008). State prisoners have accounted for most of the growth in the number of offenders housed in private prisons over the past decade (Sabol, Minton, and Harrison, 2007). Despite the rapid increase in the number of private facilities and privately housed offenders over the past three decades, inmates held in private facilities still make up only a small percent of all prisoners. Offenders confined in private facilities account for 8 percent of all prisoners; more than 16 percent of all federal prisoners are held in private facilities, while nearly 7 percent of all state prisoners are in private facilities (West, Sabol, and Greenman, 2010). 1 The debate over whether to privatize more prisons has become a fixture in many state budget negotiations. The main argument used in favor of prison privatization is that private institutions are more efficient. More than 30 cash-strapped states have turned to private prisons under the assumption that they provide the same services and produce better recidivism rates for less money than public facilities (Oppel, 2011). Proponents of private prisons have claimed as much as 20 percent in savings over state-run prisons. The difference in price, they argue, comes from the use of nonunion workers, which gives them more control over wages and benefits. Further, the desire to maintain high profit margins gives private prison corporations an incentive to act more efficiently (Cheung, 2004). Opponents of prison privatization argue that claims made in favor of this movement lack evidence (Government Accounting Office, 1996; Selman and Leighton, 2010; Cheung, 2004). Due to data limitations, as well as misleading use of data, few studies have provided convincing evidence to support the claim that private prisons provide the same or better services for less money (Government Accounting Office, 1996). All the while, private prisons generally accept only minimum or medium-security offenders with few or no health and behavioral problems (Oppel, 2011; Arizona Department of Corrections, 2011). In addition to the lack of savings, opponents of privatization cite evidence showing that private prisons are poorly run compared to public prisons (Cheung, 2004). Austin and Coventry (2001) found a higher rate of inmate-on-inmate and inmate-on-staff assaults at privately-run facilities compared to public facilities. However, other measures of inmate misconduct, including riots and other inmate-led disturbances, are comparable. 2 More recently, many states and the federal government have scaled back their reliance on private facilities (Cook, 2010; Shelden, 2010). Declining incarceration rates across several states has created less demand for bed space. Further, the Great Recession and budget crises have forced several states to crowd more prisoners into less space. Private facilities in a handful of states, including Minnesota, now sit vacant (Cook, 2010; Shelden, 2010). Present Study Prior to 2010, when prison population growth created shortages in prison beds at state facilities, the Minnesota Department of Corrections (MnDOC) frequently housed some of its inmates at the Prairie Correctional Facility (PCF) in Appleton, Minnesota. The facility, which opened in 1996, once held as many as 1,200 Minnesota state prisoners (Havens and Giles, 2009). Operated by Corrections Corporation of America (CCA), a private prison company, the PCF closed in February 2010 due, in part, to slowed growth in Minnesota’s prison population, which minimized the need to transfer offenders to nonMnDOC facilities. This study evaluated whether private prison confinement had an impact on recidivism among inmates released from Minnesota prisons between 2007 and 2009. Of the 9,535 offenders incarcerated in MnDOC facilities and released to the community during the 2007-2009 period, 1,766 (19 percent) spent at least a portion of their confinement at the PCF. Due to eligibility criteria offenders had to meet in order to be transferred to the PCF, propensity score matching was used to individually match the 1,766 offenders who spent time in the PCF with 1,766 offenders from the comparison group pool (N = 7,769) who spent their entire confinement period in state correctional 3 facilities. In analyzing the data, Cox regression was used to determine whether confinement at the PCF had an impact on recidivism. In the next section, this report reviews prior research that has compared recidivism outcomes and costs among private and public prisons. Next, it describes the private prison experience in Minnesota in greater detail. Following a description of the data and methods used in this study, the results from the statistical analyses are presented. This report concludes by discussing the implications of the findings for correctional policy and practice. Prior Research Comparing Public and Private Prisons Two major arguments made in favor of prison privatization—lower recidivism rates and lower costs—have not received thorough scholarly attention. There have been five major studies that compared the recidivism rates of public and private prisons (Lanza-Kaduce, Parker, and Thomas, 1999; Lanza-Kaduce and Maggard, 2001; Farabee and Knight, 2002; Bales et al., 2005; Spivak and Sharp, 2008) and one recent study that compared the costs (Arizona Department of Corrections, 2011). In two separate studies using the same data from Florida prisons, Lanza-Kaduce et al. (1999) and Lanza-Kaduce and Maggard (2001) found that offenders released from private prisons had a lower risk of recidivism compared to offenders released from public prisons. In the first study, the authors matched approximately 200 male prisoners from medium security public and private facilities. Individuals were matched based on race, type of offense, prior record, and age. Within 12 months of release, the private prisoners were less likely to be arrested, convicted of a new offense, and sent back to prison for a new offense compared to public prisoners. 4 In the second study, Lanza-Kaduce and Maggard (2001) used a longer follow-up period (four years). They replicated the results from the previous study using a relaxed standard of significance (p < .10) and only for one measure of recidivism (Bales et al., 2005). The authors found that private prisoners were less likely to be reincarcerated for either a new offense or a technical violation compared to public prisoners. Farabee and Knight (2002) found that the effects of public versus private prisons varied based on gender but not age. They followed nearly 9,000 inmates released from Florida prisons between 1997 and 2000 for up to three years. Recidivism was defined as a new conviction and reincarceration. They found that male prisoners released from public prisons did not have significantly different rates of recidivism for either measure of recidivism. Among female prisoners, on the other hand, releasees from private prisons were significantly less likely to be convicted of a new offense and less likely to be reincarcerated compared to releasees from public prisons. Male offenders between the ages of 18 and 24 released from public prisons did not have significantly different rates of recidivism compared to their counterparts in private prisons. Although the findings from the initial studies on Florida prisoners suggested that private prison confinement reduced recidivism for some offenders (Farabee and Knight, 2002; Lanza-Kaduce and Maggard, 2001; Lanza-Kaduce et al., 1999), Bales and colleagues (2005) reported that this effect washed out after making several methodological improvements, including a more accurate measurement of private prison exposure. Because many prisoners in states that have public and private facilities end up spending some time in both, Bales et al. (2005) measured the extent to which offenders were confined in public and private prisons. That is, they conducted multiple analyses 5 comparing groups based on where they were released and how much time they had spent in public and private facilities. They followed a base sample of approximately 80,000 Florida inmates for up to 60 months after release. Overall, Bales and colleagues (2005) found that private prison exposure had no effect on whether adult males, adult females, or youthful offenders were reconvicted of a felony or reincarcerated for a new offense. In the most recent study that compared the recidivism rates between public and private prisons, Spivak and Sharp (2008) found that inmates released from private prisons had higher rates of recidivism compared to releasees from public prisons. While the authors replicated the Bales et al. (2005) study by using data from more than 23,000 inmates released from prisons in Oklahoma, they also extended the literature by examining the proportion of time served in private and public facilities. Using a followup period that ranged between 36 and 84 months, Spivak and Sharp (2008) found that in most of the models they tested, inmates released from private prisons were more likely to return to prison compared to inmates released from public prisons. None of the above studies compared the costs of private prisons to that of public prisons. During the 1990s, the Government Accounting Office (GAO) (1991; 1996) released two reports that examined whether private prisons save states and the federal government money. After reviewing several unpublished studies that compared public and private prison costs across several states, the GAO concluded that there is no evidence that private prisons save money. Many of the studies they reviewed did not have reliable data, and some offered misleading information. Of the few studies that have provided conclusive evidence that private facilities save money over public facilities, the savings were negligible. A study by the Bureau of Justice Assistance that compared the 6 costs of private and public prisons found that, at best, private prisons provide a savings of only one percent compared to public facilities (Austin and Coventry, 2001). Moreover, findings from several meta-analyses have shown that private prisons are no more costeffective than public prisons (Pratt and Maahs, 1999; Lundahl, Kunz, Brownell, Harris, and Van Vleet, 2007). A recent report from the Arizona Department of Corrections revealed that the costs of private prisons are sometimes the same as, or greater than, the costs of running public facilities (Arizona Department of Corrections, 2011). Private prisons have either similar or higher costs in spite of the fact that in Arizona (and many other states) they take only the healthiest and most well-behaved inmates (Oppel, 2011). Moreover, private prisons usually offer less institutional programming and reentry services. The general public’s admiration of private enterprise and distrust of government contributes to a common assumption that private prisons are run more efficiently compared to public prisons (Spivak and Sharp, 2008). In other words, private prisons are thought to get the best results (lower recidivism) for the least amount of money. Although many observers believe this proposition, few studies have actually compared the costs and recidivism rates of public and private prisons, and the results have been mixed. The present study contributes to the small body of literature on the relationship between private prisons and recidivism in several ways. First, existing research has been conducted in states that rely heavily on private prisons. Here, however, we examine the performance of a private prison in a state—Minnesota—that has not historically relied on private prisons. Second, this study extends prior research by using propensity score matching to create a comparison group of offenders incarcerated only at state correctional 7 facilities. In doing so, we provide a counterfactual estimate of what would have likely happened to the offenders incarcerated at the PCF had they only been incarcerated at state correctional facilities. Third, existing research has measured recidivism as a reconviction, reincarceration for a new offense, or any return to prison. We build upon prior research by using four separate measures of recidivism (rearrest, reconviction, new offense reincarceration, and technical violation revocation) to assess private prison performance. Private Prison Confinement in Minnesota Beginning in the late 1990s, the MnDOC began transferring male prisoners to the PCF as a result of prison bed shortages created by prison population growth. The number of prisoners transferred to the PCF, however, began to increase dramatically in 2004. Indeed, the number of Minnesota offenders incarcerated at the PCF grew from less than 100 offenders on January 1, 2004, to nearly 1,200 (nearly 13 percent of the total prison population) by January 1, 2008 (Minnesota Department of Corrections, 2004; 2008). A little more than two years later, however, the MnDOC no longer housed any of its prisoners at the PCF due to its closure. The rise and fall in the number of Minnesota prisoners housed at the PCF was largely due to historic shifts in Minnesota’s prison population. On January 1, 2000, Minnesota’s prison population was a little more than 5,900 offenders (Minnesota Department of Corrections, 2000). Six years later, the prison population had grown by almost 50 percent, reaching nearly 8,900 offenders on January 1, 2006 (Minnesota Department of Corrections, 2006). Much of this growth was due to the creation of a felony driving while intoxicated (DWI) law in 2002 and a sharp increase in the number of inmates incarcerated for methamphetamine offenses. After the “meth boom” subsided 8 and the DWI offender population plateaued, the total prison population began growing at a much slower pace after 2006. Moreover, to accommodate the growth in the prison population, the MnDOC began expanding bed space capacity at the Minnesota Correctional Facility (MCF)-Faribault in 2008. As bed space shortages decreased in response to the lack of growth and the MCF-Faribault expansion, so, too, did the MnDOC’s need for PCF prison beds. Although a relatively large number of Minnesota prisoners were confined at the PCF, especially during the 2004-2009 period, offenders were required to meet a number of criteria in order to be transferred to the PCF. For example, the PCF did not accept any offenders over the age of 60, nor did it take any prisoners with serious medical conditions or mental health disorders. Further, the PCF excluded offenders whose custody-level classification was either secure or maximum, accepting only offenders who had a medium or minimum custody level classification. Given that a prisoner’s classification is determined largely by prior criminal history and behavior within the institution, minimum- and medium-level inmates tend to have less serious criminal histories and/or better behavior in prison than secure- and maximum-level offenders. Overall, Minnesota prisoners confined at the PCF were relatively healthy (mentally and physically), well-behaved inmates with limited criminal histories. Nevertheless, compared to confining an inmate at a MnDOC facility, it cost about the same to house an inmate at the PCF. For example, the MnDOC’s average marginal per diem during the 2004-2009 period was nearly $65, which is the same as the daily rate the MnDOC paid the PCF ($65) for housing an inmate (Minnesota Department of Corrections, 2012). 9 Although confinement costs for the PCF and MnDOC facilities were roughly the same, there were differences in the availability of programming. The PCF offered inmates chemical dependency treatment, adult basic education, and some types of vocational programming. While the MnDOC provided these types of programming, it also offered other programming opportunities such as EMPLOY (an employment reentry program), sex offender treatment, the Affordable Homes Program (a vocational program), and the Minnesota Comprehensive Offender Reentry Plan (MCORP). These programs have been shown to be effective in helping offenders find post-release employment (Duwe, 2012a; Duwe, 2012b; Northcutt Bohmert and Duwe, 2012) or reducing the risk of recidivism (Duwe, 2012a; Duwe, 2012b; Duwe and Goldman, 2009). Like MnDOC facilities, the PCF offered visitation privileges to inmates. It is worth noting, however, that Appleton (where the PCF is located) is about a three-hour drive from the Twin Cities (Minneapolis and St. Paul), a seven-county metropolitan area from which a majority of the prisoner population comes. Appleton is therefore about one hour farther from the Twin Cities than the most distant MnDOC facility at Moose Lake. Although it is unclear whether inmates were visited less frequently at the PCF due to the lack of PCF visitation data, distance is presumed to reduce the likelihood of visitation (Austin and Hardyman, 2004). Moreover, research on Minnesota prisoners has found that prison visitation has a significant, albeit modest, association with reduced recidivism (Duwe and Clark, 2011). Data and Methodology A retrospective quasi-experimental design was used to determine whether private prison confinement had an impact on recidivism. The population for this study consisted 10 of 9,535 Minnesota prisoners released between 2007 and 2009. This time period was selected because it captures most offenders who were confined at the PCF during its period of optimum use (2004-2009). Because the PCF housed only male offenders, female prisoners were excluded from this study. Similarly, this study excluded “shortterm offenders” who had a remaining term of imprisonment of 180 days or less at the time they were sentenced to prison. Between July 2003 and June 2009, short-term offenders were required to serve their prison time at a local jail. As a result, these offenders were not confined at a MnDOC facility, nor were they eligible for transfer to the PCF. Dependent Variable In this study, recidivism was defined as a 1) rearrest, 2) reconviction, 3) reincarceration for a new sentence, or 4) revocation for a technical violation. It is important to emphasize that the first three recidivism variables strictly measure new criminal offenses. In contrast, technical violation revocations (the fourth measure) represent a broader measure of rule-breaking behavior. Offenders can have their supervision revoked for violating the conditions of their supervised release. Because these violations can include activity that may not be criminal in nature (e.g., use of alcohol, failing a community-based treatment program, failure to maintain agent contact, failure to follow curfew, etc.), technical violation revocations do not necessarily measure reoffending. Recidivism data were collected on offenders through December 31, 2010. Given that the offenders in this study were released between January 2007 and December 2009, the follow-up time ranged from one year to four years with an average of 30 months. 11 Data on arrests and convictions were obtained electronically from the Minnesota Bureau of Criminal Apprehension. Reincarceration and revocation data were derived from the Correctional Operations Management System (COMS) database maintained by the MnDOC. The main limitation with using these data is that they measure only arrests, convictions or incarcerations that took place in Minnesota, although it is worth noting that interstate recidivism is relatively low (Langan and Levin, 2002). As a result, the findings presented later likely underestimate the true recidivism rates for the offenders examined here. To accurately measure the total amount of time offenders were actually at risk to reoffend (i.e., “street time”), it was necessary to account for supervised release revocations in the recidivism analyses. More specifically, for the three recidivism variables that strictly measure new criminal offenses (rearrest, reconviction, and new offense reincarceration), it was necessary to deduct the amount of time they spent in prison for technical violation revocations from their total at-risk period. Failure to deduct time spent in prison as a supervised release violator would artificially increase the length of the at-risk periods for these offenders. Therefore, to achieve a more accurate measure of “street time,” the time that an offender spent in prison as a supervised release violator was subtracted from his at-risk period, but only if it preceded a rearrest, a reconviction, a reincarceration for a new offense, or if the offender did not recidivate prior to January 1, 2011. To illustrate, if an offender was released on January 1, 2007, he would have had an at-risk period of 48 months given that December 31, 2010, was the end of the followup period. If it is assumed that this offender returned to prison for a technical violation 12 revocation on January 1, 2008, then his time to failure for a technical violation revocation would be 12 months. If it is further assumed, however, that this offender was imprisoned until June 30, 2008, for this technical violation revocation, our at-risk period calculations in the rearrest, reconviction, and reincarceration analyses took into account the six months this offender spent in prison from January 1-June 30, 2008. If this offender did not recidivate with a new criminal offense, then his total at-risk period would have been 42 months instead of 48. If, however, this offender was rearrested for a new offense on January 1, 2009, the time to rearrest would have been 18 months instead of 24. Independent Variables The main objective of this study is to evaluate the relationship between private prison confinement and recidivism. As a result, the 1,766 offenders who served time in the PCF were assigned a value of “1”, whereas the offenders in the comparison group were given a value of “0”. Similar to the approach taken by Bales et al. (2005), two additional measures of private prison exposure were created. These measures reflect the fact that many offenders are imprisoned at multiple facilities during their sentences and may not spend their entire terms of confinement in private facilities. For the first measure, Private Prison Time, the total number of days offenders were incarcerated at the PCF were counted. For the second measure, Private Prison Time Proportion, a variable was created that calculated the proportion of time served at the PCF. For example, if an offender’s term of imprisonment was 500 days and 200 of these days were served at the PCF, then the Private Prison Time Proportion for this offender would be 0.40. 13 The independent, or control, variables included in the statistical models were those that were not only available in the COMS database but also might have an impact on recidivism and PCF selection. Table 1 describes the covariates used in the statistical Table 1. Logistic Regression Model for Private Prison Selection Predictors Predictor Description Coefficient Minority Age at Release (years) Prior Supervision Failures Prior Convictions LSI-R Score Mental Health Medical Health Offense Type Property Drugs Other Criminal Sexual Conduct Felony DWI New Court Commitment Metro Commit Length of Stay (months) Supervision Type ISR CIP Work Release Discharge Release Year Constant N Log-likelihood Nagelkerke R2 Minority = 1; White = 0 Offender age in years at time of release from prison Number of prior revocations while under correctional supervision Number of prior felony convictions, excluding index conviction(s) Most recent Level of Service Inventory-Revised (LSIR) score prior to release Mental health problems (major mental illness, significant mood disorder, brain injury or is intellectually impaired) = 1; no critical mental problems = 0 Medical health problems (visual impairment, speech impairment, mobility impairment, hearing loss, paraplegic, etc.) = 1; no medical health problems = 0 Person offense serves as the reference Property offense = 1; non-property offense = 0 Drug offense = 1; non-drug offense = 0 Other offense = 1; non-other offense = 0 Sex offense = 1; non-sex offense = 0 Felony DWI offense = 1; non-Felony DWI offense = 0 New commitment = 1; probation or release violator = 0 Twin Cities metropolitan area = 1; Greater Minnesota =0 Number of months between prison admission and release dates Supervised release serves as the reference Intensive supervised release (ISR) = 1; non-ISR = 0 Challenge Incarceration Program (CIP) = 1; non-CIP = 0 Work Release = 1; non-Work Release = 0 Discharge = 1; released to correctional supervision = 0 Year in which first released from prison for instant offense ** p < .01 * p < .05 0.032 -0.010** Standard Error -0.084** 0.026 0.010 0.008 -0.033** 0.005 -0.019 0.074 -0.279** 0.061 0.434** 1.131** 0.676** 0.111 0.099 0.108 -1.057** 1.398** 0.189 0.122 0.827** 0.074 -0.038 0.060 0.012** 0.001 -0.275** 0.092 0.160 0.218** 0.398 0.105 0.079 0.268 0.162** -327.425 9,535 7968.569 0.187 0.035 69.581 models. Several measures commonly associated with recidivism risk were used, including the offender’s race, age, number of prior supervision failures and prior 14 0.062 0.003 convictions, and Level of Service Inventory- Revised (LSI-R) score (Gendreau, Little, & Goggin, 2006). Because private prisons, including the PCF, often do not accept inmates with certain mental and medical conditions (Arizona Department of Corrections, 2011; Oppel, 2011), several measures pertaining to medical and mental health were included. Similar studies on private prison confinement and recidivism have found that offense type (e.g., person offense, drug offense), length of sentence, and post-release supervision significantly affect the outcome measure (Bales et al., 2005; Spivak & Sharp, 2008). Thus, these measures were included in the analyses. Prior research has shown that admission type (New Commit), county of commitment (Metro), and length of stay are significant predictors of recidivism for Minnesota prisoners (Duwe, 2010; Duwe and Clark, 2011), which is why they were included in this study. Measures relating to participation in institutional programming (e.g., chemical dependency treatment), visitation, or institutional discipline were excluded because these data were not available for offenders while they were housed at the PCF. Propensity Score Matching PSM is a method that estimates the conditional probability of selection to a particular treatment or group given a vector of observed covariates (Rosenbaum & Rubin, 1985). The predicted probability of selection, or propensity score, is typically generated by estimating a logistic regression model in which selection (0 = no selection; 1 = selection) is the dependent variable while the predictor variables consist of those that theoretically have an impact on the selection process. Once estimated, the propensity scores are then used to match individuals who entered treatment with those who did not. 15 Thus, an advantage with using PSM is that it can simultaneously “balance” multiple covariates on the basis of a single composite score. In matching private prison offenders with public prison offenders on the conditional probability of entering the PCF, PSM reduces selection bias by creating a counterfactual estimate of what would have happened to the private prison offenders had they not been housed at the PCF. PSM has several limitations, however, that are worth noting. First, and foremost, because propensity scores are based on observed covariates, PSM is not robust against “hidden bias” from unmeasured variables that are associated with both the assignment to treatment and the outcome variable. Second, there must be substantial overlap among propensity scores between the two groups in order for PSM to be effective (Shadish, Cook & Campbell, 2002); otherwise, the matching process will yield incomplete or inexact matches. Finally, as Rubin (1997) points out, PSM tends to work best with large samples. Although somewhat limited by the data available, an attempt was made to address potential concerns over unobserved bias by including as many theoretically-relevant covariates (20) as possible in the propensity score model. In addition, this study later demonstrates there was substantial overlap in propensity scores between the treated and untreated offenders. Further, the sample size limitation was addressed by assembling a large number of cases (N = 9,535) on which to conduct the propensity score analyses. Matching Private and Public Prison Inmates Propensity scores were calculated for the 1,766 private prison participants and the 7,769 non-participants in the comparison group pool by estimating a logistic regression model in which the dependent variable was private prison incarceration. The predictors 16 were the 20 control variables used in the statistical analyses (see Table 1). The results show a number of factors that predicted whether offenders were incarcerated at the PCF. The Nagelkerke R2 value of 0.187 suggests the model explained nearly 19 percent of the variability in entering the PCF. In Table 1, the results show that the odds of serving time at the PCF were significantly greater for younger offenders, offenders with fewer prior supervision failures, offenders with lower LSI-R scores, offenders with fewer medical health problems, and offenders admitted to prison as a new court commitment. Regarding offense type, offenders incarcerated for property, drug, DWI, and other offenses were significantly more likely to be housed at the PCF than offenders imprisoned for a person offense. Sex offenders, however, were significantly less likely to be housed at the PCF. Regarding post-release supervision, we also see that offenders confined at the PCF were significantly less likely to be intensively supervised, although they were more likely to be placed on work release. Lastly, release year was positively associated with entering the PCF, which reflects the fact that the number of offenders entering the facility increased over the period examined in this study. As shown in Table 2, the difference in mean propensity score between private and public prison offenders was statistically significant at the .01 level. Still, there was substantial overlap in propensity scores. Indeed, the vast majority of offenders in both groups (93 percent for private prison and 98 percent for public prison) had propensity scores less than 0.50. After obtaining propensity scores for the 9,535 offenders, a “greedy” matching procedure that utilized a without replacement method was used to match the private 17 Table 2. Propensity Score Matching and Covariate Balance for Private Participation Variable Sample Propensity Score Total Matched Total Matched Total Matched Total Matched Total Matched Total Matched Total Matched Total Matched Total Matched Total Matched Total Matched Total Matched Total Matched Total Matched Total Matched Total Matched Total Matched Total Matched Total Matched Total Matched Total Matched Minority Age at Release (Years) Prior Supervision Failures Prior Convictions LSI-R Score Mental Health Medical Health Property Drugs Other Criminal Sexual Conduct Felony DWI New Court Commit Metro Length of Stay (Months) ISR CIP Work Release Discharge Release Year Total Private = 1,766 Total Public = 7,769 Matched Private = 1,766 Matched Public = 1,766 Private Mean 28.56% 28.56% 47.06% 47.06% 35.03 35.03 0.74 0.74 4.89 4.89 23.82 23.82 18.40% 18.40% 35.84% 35.84% 13.93% 13.93% 40.49% 40.49% 14.04% 14.04% 2.15% 2.15% 15.80% 15.80% 81.20% 81.20% 50.06% 50.06% 29.46 29.46 13.02% 13.02% 13.36% 13.36% 19.65% 19.65% 1.13% 1.13% 2008.09 2008.09 18 Public Mean 16.23% 28.54% 49.30% 46.15% 34.89 35.23 1.21 0.71 5.05 4.79 27.22 23.43 26.08% 19.00% 46.09% 36.75% 21.11% 12.85% 22.14% 39.64% 15.25% 15.06% 10.22% 2.55% 7.50% 16.25% 61.17% 80.18% 54.00% 50.74% 21.79 30.03 26.73% 14.78% 4.85% 14.27% 11.38% 18.23% 1.24% 1.02% 2007.96 2008.08 Bias (%) 75.04 0.12 3.66 1.49 1.19 1.74 28.98 2.05 3.19 2.06 39.31 4.64 15.47 1.26 17.22 1.55 15.92 2.57 32.09 1.42 2.81 2.37 31.18 2.19 20.28 1.00 38.41 2.12 6.44 1.11 30.45 1.95 29.85 4.20 22.83 2.16 18.11 2.94 0.84 0.87 13.21 1.01 Bias Reduction -99.84% -59.35% 45.60% -92.93% -35.43% -88.19% -91.86% -91.02% -83.83% -95.59% -15.59% -92.99% -95.05% -94.49% -82.76% -93.60% -85.94% -90.52% -83.74% 3.08% -92.32% t test p Value 0.00 0.96 0.09 0.59 0.61 0.52 0.00 0.40 0.15 0.47 0.00 0.09 0.00 0.67 0.00 0.58 0.00 0.35 0.00 0.61 0.20 0.39 0.00 0.44 0.00 0.71 0.00 0.44 0.00 0.69 0.00 0.53 0.00 0.13 0.00 0.44 0.00 0.28 0.72 0.74 0.00 0.82 prison offenders with the public prison inmates. Private prison offenders were matched to public prison offenders who had the closest propensity score (i.e., “nearest neighbor”) within a caliper (i.e., range of propensity scores) of 0.10. Matches were found for all 1,766 private prison offenders. Table 2 presents the covariate and propensity score means for both groups prior to matching (“total”) and after matching (“matched”). In addition to tests of statistical significance (“t test p value”), Table 2 provides a measure (“Bias”) developed by Rosenbaum and Rubin (1985) that quantifies the amount of bias between Bias = 100(X t - X c ) ( S t2 + S c2 ) 2 the treatment and comparison samples (i.e., standardized mean difference between samples), where X t and St2 represent the sample mean and variance for the treated offenders and X c and S c2 represent the sample mean and variance for the untreated offenders. If the value of this statistic exceeds 20, the covariate is considered to be unbalanced (Rosenbaum & Rubin, 1985). As shown in Table 2, the matching procedure reduced the bias in propensity scores between the private and public prison offenders by 99 percent. Whereas the p value was 0.00 in the unmatched sample, it was 0.96 in the matched sample. In the unmatched sample, there were nine covariates that were significantly imbalanced (i.e., the bias values exceeded 20). But in the matched sample, covariate balance was achieved given that no covariates had bias values greater than 20. For example, in the unmatched sample, the average LSI-R score for the 1,766 offenders in the private prison group was 23.82 versus an average score of 27.22 for the 7,769 offenders in the comparison group pool. The difference in LSI-R scores between the two groups was statistically significant, 19 and the bias value was 39.31. After the matching procedure was used, however, the average LSI-R score for the 1,766 offenders in the comparison group was 23.43, which yielded a bias value of 4.64 and a t test value no longer significant at the .05 level. Analysis In analyzing recidivism, survival analysis models are preferable in that they utilize time-dependent data, which are important in determining not only whether offenders recidivate but also when they recidivate. As a result, this study uses a Cox regression model, which uses both “time” and “status” variables in estimating the impact of the independent variables on recidivism. For the analyses presented here, the “time” variable measures the amount of time from the date of release until the date of first rearrest, reconviction, reincarceration, technical violation revocation, or December 31, 2010, for those who did not recidivate. The “status” variable, meanwhile, measures whether an offender recidivated (rearrest, reconviction, reincarceration for a new crime, and technical violation revocation) during the period in which he was at risk to recidivate. In the analyses presented below, Cox regression models were estimated for each of the four recidivism measures. Results Recidivism rates are presented in Table 3 for offenders housed in the PCF (private), offenders in the matched comparison group housed only in MnDOC facilities (public), and all offenders housed only in MnDOC facilities (public comparison group pool). The results show that the 7,769 offenders in the public comparison group pool had higher rates for all four recidivism measures than the 1,766 offenders housed at the PCF. Yet, after balancing the two groups on factors related to PCF selection and/or recidivism 20 risk, the results indicate that the private prison offenders had slightly higher recidivism rates than those in the public prison comparison group. Table 3. Recidivism Rates by Private and Public Prison Recidivism Private Public Rearrest Reconviction New Offense Reincarceration Technical Violation Revocation N 46.5% 31.0% 14.0% 33.2% 1,766 41.4% 25.8% 13.0% 31.0% 1,766 Public Comparison Pool 54.5% 37.0% 18.7% 39.9% 7,769 Although these findings provide little indication as to whether private prison confinement had a significant impact on recidivism, the observed recidivism differences between the private and public prison offenders may be due to other factors such as time at risk, amount of time incarcerated at a private facility, and proportion of prison term served at a private facility. To statistically control for the impact of these other factors on reoffending, Cox regression models were estimated for each private prison measure across the four measures of recidivism. To determine model fit, the assumptions that the hazards are proportional and that the relationships between the log hazard and covariates are nonlinear were tested. Inspection of the residuals revealed that each of the Cox regression models estimated in this study adequately fit the data. The Impact of Private Prison on Recidivism The results in Table 4 indicate that, controlling for the effects of the other independent variables in the statistical model, offenders housed at the PCF for any period of time had a significantly greater hazard ratio for two of the four recidivism measures examined. In the rearrest model, the hazard ratio for the private prison variable was 21 1.133, which indicates that private prison confinement significantly increased the risk of rearrest by 13 percent. In the reconviction model, the hazard ratio for the private prison variable was 1.221, which suggests that private prison confinement increased the risk of reconviction by 22 percent. Private prison confinement did not have a significant impact on either new offense reincarceration or reincarceration for a technical violation revocation. Table 4. Cox Regression: Impact of Any Private Prison on the Hazard of Recidivism Rearrest Reconviction Reincarceration Private Prison Minority Age at Release (years) Prior Supervision Failures Prior Convictions LSI-R Score Mental Health Medical Health Offense Type Property Drugs Other Criminal Sexual Conduct Felony DWI New Court Commitment Metro Length of Stay (months) Supervision Type ISR CIP Work Release Discharge Release Year Supervised Release Revocations N ** p < .01 * p < .05 Hazard Ratio 1.133* 1.278** 0.961** 1.169** 1.067** 1.028** 1.064 1.147* SE 0.051 0.056 0.004 0.022 0.007 0.004 0.066 0.056 Hazard Ratio 1.221** 1.108 0.960** 1.183** 1.055** 1.032** 1.101 1.207** 1.202 0.977 1.005 0.510* 0.987 1.060 1.352** 0.994** 0.100 0.091 0.096 0.299 0.110 0.068 0.055 0.001 0.922 0.538** 0.883 1.415 0.943 0.829** 3,532 0.083 0.106 0.070 0.209 0.034 0.051 SE 0.065 0.069 0.004 0.026 0.009 0.005 0.080 0.069 Hazard Ratio 1.078 1.242* 0.963** 1.281** 1.033** 1.037** 1.558** 1.604** 1.338* 1.024 1.092 0.565 1.166 1.175 1.262** 0.992** 0.124 0.116 0.121 0.392 0.139 0.084 0.068 0.002 0.820 0.457** 0.854 2.116** 0.960 0.978 3,532 0.106 0.143 0.087 0.226 0.045 0.051 SE Revocation 0.096 0.102 0.006 0.033 0.012 0.008 0.106 0.099 Hazard Ratio 1.050 1.608** 0.973** 1.162** 1.018* 1.034** 1.337** 1.258** 0.060 0.065 0.004 0.024 0.009 0.005 0.074 0.064 1.593** 0.941 0.852 0.830 1.442 1.794** 1.277* 0.986** 0.178 0.174 0.184 0.525 0.200 0.131 0.100 0.003 1.017 0.997 1.141 1.788** 1.362* 0.969 1.093 0.999 0.122 0.109 0.112 0.210 0.128 0.077 0.064 0.001 0.654** 0.342** 0.973 3.670** 1.029 0.867* 3,532 0.163 0.253 0.125 0.264 0.068 0.067 1.718** 1.185 1.673** 0.091 0.116 0.079 0.990 0.039 3,494 The results also showed the hazard ratio was significantly greater for younger offenders (all four measures), prior supervision failures (all four measures), prior convictions (all four measures), offenders with higher LSI-R scores (all four measures), 22 SE offenders with medical concerns (all four measures), minority inmates (three measures), offenders committed from the Metro area (three measures), shorter lengths of stay in prison (three measures), offenders with mental health concerns (two measures), inmates who were released to no supervision (two measures), property offenders (two measures), new court commitments (new offense reincarceration), sex offenders (technical violation revocations), DWI offenders (technical violations revocations), offenders placed on work release (technical violation revocations), and those released to ISR (technical violation revocations). The risk (hazard) of revocation for a technical violation was significantly lower, however, for CIP offenders (three measures), supervised release revocations (two measures), sex offenders (rearrest), and offenders placed on ISR (new offense reincarceration). Of the 1,766 offenders who were confined at the PCF, their lengths of stay at that facility ranged from one day to 2,912 days (about eight years) (SD = 8.61). The average length of stay at the PCF for these offenders was 349 days (almost a year), whereas the median length of stay was 295 days. In Table 5, the results that examined the impact of total time spent at the PCF (in days) on the four recidivism measures are presented. The findings indicate that private prison had a significant effect on only one of the four recidivism measures, increasing the hazard for reconviction. More specifically, one additional day in confinement at the PCF increased the risk of reconviction by 1.3 percent. Because the findings for the other covariates are similar to those presented in Table 4, they will not be repeated here. 23 Table 5. Cox Regression: Impact of Time in Private Prison on the Hazard of Recidivism Rearrest Reconviction Reincarceration Revocation Private Prison Time (Days) Minority Age at Release (years) Prior Supervision Failures Prior Convictions LSI-R Score Mental Health Medical Health Offense Type Property Drugs Other Criminal Sexual Conduct Felony DWI New Court Commitment Metro Length of Stay (months) Supervision Type ISR CIP Work Release Discharge Release Year Supervised Release Revocations N ** p < .01 * p < .05 Hazard Ratio 1.003 1.276** 0.961** 1.169** 1.067** 1.028** 1.064 1.149* SE 0.003 0.056 0.004 0.022 0.007 0.004 0.066 0.056 Hazard Ratio 1.013** 1.104 0.959** 1.185** 1.056** 1.032** 1.105 1.217** 1.196 0.965 0.992 0.507* 0.983 1.062 1.355** 0.994** 0.100 0.091 0.096 0.299 0.110 0.068 0.055 0.001 0.916 0.547** 0.885 1.430 0.941 0.830** 3,532 0.083 0.107 0.070 0.208 0.034 0.051 SE 0.004 0.069 0.004 0.026 0.009 0.005 0.080 0.069 Hazard Ratio 1.001 1.240* 0.963** 1.282** 1.033** 1.038** 1.557** 1.603** 1.322* 1.008 1.067 0.566 1.152 1.175 1.268** 0.991** 0.124 0.116 0.121 0.392 0.139 0.085 0.068 0.002 0.818 0.479** 0.859 2.131** 0.954 0.982 3,532 0.106 0.143 0.087 0.226 0.045 0.051 SE 0.007 0.102 0.006 0.033 0.012 0.008 0.106 0.099 Hazard Ratio 1.001 1.607** 0.973** 1.162** 1.018* 1.034** 1.336** 1.259** 0.004 0.065 0.004 0.024 0.009 0.005 0.074 0.064 1.593** 0.933 0.848 0.829 1.437 1.796** 1.280* 0.986** 0.178 0.174 0.184 0.525 0.200 0.131 0.100 0.003 1.015 0.993 1.137 1.782** 1.361* 0.969 1.093 0.999 0.122 0.109 0.112 0.210 0.128 0.077 0.064 0.001 0.649* 0.348** 0.974 3.702** 1.028 0.867* 3,532 0.163 0.252 0.125 0.263 0.068 0.067 1.714** 1.189 1.675** 0.091 0.116 0.079 0.990 0.039 3,494 The 1,766 offenders housed at the PCF spent, on average, 40 percent of their term of imprisonment at that facility. The median proportion of time served at the PCF was 38 percent. The minimum percentage of time served at PCF was less than one percent, whereas the maximum was 92 percent (SD = 0.23). In Table 6, the results from the Cox regression models that estimated the impact of proportion of prison time served at the PCF on the four recidivism measures are presented. The proportion of prison time served at the PCF significantly increased the hazard by 21 percent for rearrest and 52 percent for reconviction. Put another way, the greater the proportion of private prison 24 SE Table 6. Cox Regression: Impact of Private Prison Proportion on the Hazard of Recidivism Rearrest Reconviction Reincarceration Revocation Private Prison Time Proportion Minority Age at Release (years) Prior Supervision Failures Prior Convictions LSI-R Score Mental Health Medical Health Offense Type Property Drugs Other Criminal Sexual Conduct Felony DWI New Court Commitment Metro Length of Stay (months) Supervision Type ISR CIP Work Release Discharge Release Year Supervised Release Revocations N ** p < .01 * p < .05 Hazard Ratio 1.210* 1.276** 0.961** 1.169** 1.067** 1.028** 1.068 1.152* SE 0.095 0.056 0.004 0.022 0.007 0.004 0.066 0.056 Hazard Ratio 1.520** 1.108 0.959** 1.184** 1.056** 1.032** 1.109 1.219** 1.198 0.974 0.999 0.511* 0.992 1.061 1.355** 0.995** 0.100 0.091 0.096 0.300 0.110 0.068 0.055 0.001 0.923 0.547** 0.886 1.418 0.943 0.829** 3,532 0.083 0.106 0.070 0.208 0.034 0.051 SE 0.116 0.069 0.004 0.026 0.009 0.005 0.080 0.069 Hazard Ratio 1.107 1.241* 0.963** 1.281** 1.034** 1.038** 1.559** 1.606** 1.332* 1.029 1.090 0.571 1.184 1.180 1.267** 0.992** 0.124 0.116 0.121 0.392 0.139 0.084 0.068 0.002 0.823 0.475** 0.858 2.054** 0.958 0.980 3,532 0.106 0.143 0.087 0.226 0.045 0.051 SE 0.175 0.102 0.006 0.033 0.012 0.008 0.106 0.099 Hazard Ratio 1.056 1.607** 0.973** 1.162** 1.019* 1.034** 1.337** 1.259** 0.116 0.065 0.004 0.024 0.009 0.005 0.074 0.064 1.593** 0.938 0.851 0.831 1.445 1.798** 1.279* 0.986** 0.178 0.174 0.184 0.525 0.201 0.131 0.100 0.003 1.015 0.995 1.139 1.787** 1.363* 0.969 1.093 0.999 0.122 0.109 0.112 0.210 0.128 0.077 0.064 0.001 0.651** 0.348** 0.974 3.658** 1.028 0.867* 3,532 0.163 0.252 0.125 0.264 0.068 0.067 1.715** 1.190 1.675** 0.091 0.116 0.079 0.990 0.039 3,494 confinement time, the greater the risk of rearrest and reconviction. Proportion of confinement time at the PCF did not have a significant effect, however, on the other two recidivism measures (new offense reincarceration and technical violation revocations). In an effort to address concerns that the three private prison measures used above were insufficient to examine the effects of private prison confinement, two additional sets of analyses were conducted. Private prison confinement was limited to a) prisoners who served at least one year at the PCF and b) offenders whose percentage of time served at the PCF was at least 50 percent of their total confinement time. Next, propensity score 25 SE Table 7. Cox Regression: Impact of Private Prison Time in Excess of One Year on the Hazard of Recidivism Rearrest Reconviction Reincarceration Revocation Private Prison Time (> 1 year) Minority Age at Release (years) Prior Supervision Failures Prior Convictions LSI-R Score Mental Health Medical Health Offense Type Property Drugs Other Criminal Sexual Conduct Felony DWI New Court Commitment Metro Length of Stay (months) Supervision Type ISR CIP Work Release Discharge Release Year Supervised Release Revocations N ** p < .01 * p < .05 Hazard Ratio 1.000 1.172 0.954** 1.230** 1.066** 1.024** 1.007 1.331** SE 0.004 0.089 0.006 0.036 0.013 0.007 0.117 0.089 0.941 0.959 1.045 0.403 0.903 1.275* 1.298** 0.996 0.165 0.141 0.149 0.595 0.182 0.121 0.087 0.002 0.965 0.587* 0.908 1.464 0.967 0.814* 1,406 0.137 0.258 0.110 0.388 0.055 0.088 Hazard Ratio 1.012* 1.071 0.958** 1.238** 1.072** 1.029** 0.950 1.345** SE 0.005 0.112 0.007 0.045 0.016 0.008 0.144 0.110 Hazard Ratio 1.007 1.360 0.954** 1.431** 1.066** 1.045** 1.482 1.696** 1.016 0.782 0.922 0.228 0.687 1.393* 1.164 0.197 0.176 0.188 1.018 0.228 0.153 0.108 0.994* 0.746 0.535 0.980 2.306* 0.981 1.017 1,406 0.003 0.178 0.371 0.135 0.395 0.074 0.090 SE 0.008 0.183 0.012 0.059 0.024 0.014 0.204 0.176 Hazard Ratio 1.000 1.395** 0.971** 1.188** 1.019 1.035** 1.341* 1.119 SE 0.005 0.106 0.007 0.042 0.017 0.008 0.129 0.107 1.881 1.284 1.379 1.435 1.427 3.098** 1.270 0.352 0.333 0.348 1.058 0.390 0.291 0.177 1.128 0.958 1.229 1.493 1.462 0.999 1.026 0.194 0.171 0.175 0.379 0.209 0.137 0.102 0.996 0.814 0.937 1.283 1.658 0.881 0.807 1,406 0.005 0.290 0.610 0.215 0.726 0.123 0.124 1.001 1.850** 1.115 1.552** 0.002 0.147 0.289 0.125 1.049 0.065 1,392 analyses were conducted to create a comparison group for the 703 offenders who served at least one year at the PCF and another comparison group for the 625 offenders whose percentage of time served at the PCF was 50 percent or higher. After creating comparison groups that were not significantly different from these two private prison groups, Cox regression models were estimated for both private prison measures among the four recidivism outcomes, resulting in eight additional models. Consistent with the results shown above, the results show that private prison confinement increased the hazard of recidivism for all eight models. The effects were 26 Table 8. Cox Regression: Impact of Private Prison Time (50% or more) on the Hazard of Recidivism Rearrest Reconviction Reincarceration Revocation Private Prison Time > 50% Minority Age at Release (years) Prior Supervision Failures Prior Convictions LSI-R Score Mental Health Medical Health Offense Type Property Drugs Other Criminal Sexual Conduct Felony DWI New Court Commitment Metro Length of Stay (months) Supervision Type ISR CIP Work Release Discharge Release Year Supervised Release Revocations N ** p < .01 * p < .05 Hazard Ratio 1.281* 1.078 0.963** 1.216** 1.038** 1.026** 1.090 1.383** SE 0.124 0.092 0.005 0.034 0.013 0.007 0.113 0.090 Hazard Ratio 1.614** 0.947 0.964** 1.283** 1.028 1.029** 0.914 1.475** 1.147 1.004 1.157 0.249 1.130 1.335* 1.328** 0.991** 0.147 0.142 0.138 0.726 0.220 0.119 0.092 0.003 1.056 0.572* 0.917 1.909** 1.043 0.877 1,250 0.139 0.250 0.115 0.240 0.058 0.087 SE 0.151 0.112 0.007 0.038 0.015 0.008 0.137 0.109 Hazard Ratio 1.448 1.076 0.969** 1.299** 1.020 1.050** 1.196 2.146** 1.055 0.960 1.034 0.356 0.990 1.565** 1.352** 0.988** 0.177 0.172 0.170 0.736 0.276 0.143 0.112 0.004 0.841 0.407* 0.965 2.811** 1.152 1.061 1,250 0.178 0.374 0.139 0.255 0.073 0.084 SE 0.235 0.174 0.010 0.048 0.021 0.013 0.187 0.167 Hazard Ratio 1.073 1.696** 0.969** 1.232** 1.006 1.023** 1.432** 1.272* 0.156 0.116 0.007 0.039 0.017 0.009 0.136 0.115 2.163* 1.358 1.409 1.097 2.304 2.436** 1.421* 0.986* 0.308 0.309 0.309 0.793 0.434 0.240 0.176 0.007 1.343 1.043 1.233 2.080 2.060** 1.099 0.951 1.000 0.191 0.192 0.187 0.444 0.254 0.144 0.114 0.003 0.640 0.570 1.010 7.434** 1.265* 0.948 1,250 0.312 0.613 0.226 0.325 0.118 0.110 1.969** 1.124 1.860** 0.159 0.290 0.134 1.011 0.069 1,218 statistically significant, however in only three of the eight models. Total private prison time served (i.e., 12 months or more) significantly increased the hazard of reconviction (see Table 7), whereas the percentage of private prison time served measure (i.e., 50 percent or more) significantly increased the risk of rearrest and reconviction (see Table 8). Conclusion The findings showed that private prison incarceration was associated with a greater risk of recidivism in all 20 Cox regression models that were estimated. This 27 SE association was statistically significant, however, in only 8 of the 20 models. In particular, all five private prison measures significantly increased the risk of reconviction, whereas three measures (any private prison, private prison time proportion, and offenders who served at least half of their time in the PCF) had a significant effect on rearrest. None of the private prison variables, however, had a significant impact on either reincarceration measure (new offense reincarceration and technical violation revocation). While the findings suggest that time spent at the PCF did not have a beneficial impact on recidivism outcomes, it should be emphasized that PCF confinement did not significantly increase risk consistently across all recidivism measures examined. Moreover, the magnitude of increased recidivism risk was relatively modest (13 percent for rearrest and 22 percent for reconviction) in the models that analyzed any exposure to private prison. Findings from the three most recent evaluations (including this one) on prisoners in three different states suggest that confinement in private prisons does not lead to improved recidivism outcomes. For example, in the most rigorous study on Florida prisoners to date, Bales and colleagues (2005) found no difference in recidivism between public and private facilities. Spivak and Sharp (2008), meanwhile, reported that private prison confinement among Oklahoma prisoners was significantly associated with increased recidivism in most of the models they estimated. Like Spivak and Sharp (2008), we found that private prison confinement significantly increased offender recidivism risk in some, but not all, of the models that were tested. The recidivism results observed for private prisons may be attributable to a lack of visitation and rehabilitative programming in comparison to state-operated facilities. Although PCF programming and visitation data were not available, which is perhaps the 28 most significant limitation with this study, the PCF offered fewer programming opportunities for offenders compared to MnDOC facilities. To be sure, the PCF provided offenders with programming to address chemical dependency, educational, and vocational needs. The MnDOC facilities, however, offered a greater variety of programming, some of which has been demonstrated to increase employment (Duwe, 2012b; Northcutt Bohmert and Duwe, 2012) and lower recidivism (Duwe, 2012a; Duwe, 2012b; Duwe and Goldman, 2009). Moreover, even though the PCF provided offenders with visitation opportunities, the facility’s distance from Minnesota’s major population center (Twin Cities) may have resulted in fewer visits, at least in comparison to MnDOC facilities. Given that visitation has a significant, although modest, impact on recidivism (Duwe and Clark, 2011), fewer visits may have contributed to slightly higher recidivism rates for those who were confined at the PCF. A cost-benefit analysis was outside the scope of this evaluation, although it is worth reiterating that the daily rate the MnDOC paid the PCF was roughly the same as the MnDOC’s marginal per diem. As such, the PCF did not incarcerate these offenders less expensively than the MnDOC. Yet, due to PCF eligibility criteria, the MnDOC confined offenders who are, compared to those placed at the PCF, generally more expensive to incarcerate (i.e., older, less healthy, and more likely to have behavioral problems). Moreover, the higher recidivism rates observed among the PCF offenders relative to the comparison group may have resulted in more crimes committed postrelease, producing increased reincarceration and victimization costs to the state. Confining offenders at the PCF, then, may have ultimately cost the state more money than if they had been able to remain at a MnDOC facility. 29 As noted earlier, however, there was no room for these offenders at MnDOC facilities. When prison bed shortages arise, corrections agencies generally have three options: 1) construct a new facility, 2) add bed space capacity to an existing facility and/or 3) transfer inmates to private or public (local, state, or federal) correctional facilities where space is available. Whereas the first two options are geared towards addressing anticipated long-term population growth, transferring offenders to other correctional facilities provides a viable alternative to address rapid, short-term growth. In 2008, the MnDOC began to gradually expand the bed space capacity at the MCFFaribault to accommodate increases in the prison population over the long term. Prior to achieving a more long-lasting solution, however, the PCF provided the MnDOC with a release valve to help manage prison bed space shortages in the interim. As this evaluation suggests, private prisons can offer correctional agencies a valuable resource—prison bed space—during periods of sharp population growth. The value of this resource declines, however, if bed space is available in state-operated facilities. More specifically, the evidence from this evaluation and prior studies indicates that private prisons are not a superior alternative to state-run prisons. The findings from this study suggest, if anything, that private prisons produce slightly worse recidivism outcomes among the healthiest and best-behaved inmates for the same amount of money. Given the small number of existing studies, however, more research is needed from other states that utilize private prisons to draw a more definitive conclusion regarding the relative effectiveness of private and public prisons. To that end, it is recommended that future research should not only compare recidivism outcomes between offenders confined at private and state-operated prisons, but that it should also link these 30 outcomes to the provision of programming at both types of facilities. Future studies should also take the costs of recidivism into account when examining the cost effectiveness of private and public prisons. 31 References Arizona Department of Corrections (2011). FY 2010 Operating Per Capita Cost Report: Cost Identification and Comparison of State and Private Contract Beds. Phoenix, AZ: Arizona Department of Corrections, Bureau of Planning, Budget and Research. Austin, J., & Coventry, G. (2001). Emerging Issues on Privatized Prisons. Monograph. Washington, DC: U.S. Department of Justice, Office of Justice Programs, Bureau of Justice Assistance. Austin, J., & Hardyman, P.L. (2004). The Risks and Needs of the Returning Prisoner Population. Review of Policy Research, 21, 13-29. Bales, W.D., Bedard, L.E., Quinn, S.T., Ensley, D.T., & Holley, G.P. (2005). Recidivism of Public and Private State Prison Inmates in Florida. Criminology and Public Policy, 4, 57-82. Cheung, A. (2004). Prison Privatization and the Use of Incarceration. Washington, DC: The Sentencing Project. Cook, N. (2010). “How the Recession Hurts Private Prisons.” Newsweek, Accessed August 11th, 2011. (http://www.thedailybeast.com/newsweek/2010/06/30/howthe-recession-hurts-private-prisons.html). Duwe, G. (2010). Prison-based chemical dependency treatment in Minnesota: An outcome evaluation. The Journal of Experimental Criminology 6: 57-81. Duwe, G. (2012a). Evaluating the Minnesota Comprehensive Offender Reentry Plan (MCORP): Results from a randomized experiment. Justice Quarterly, 29: 347383. Duwe, G. (2012b). The benefits of keeping idle hands busy: The impact of a prisoner reentry employment program on post-release employment and offender recidivism. Crime & Delinquency. DOI: 10.1177/0011128711421653. Duwe, G., & Clark, V. (2011). Blessed be the social tie that binds: The effects of prison visitation on offender recidivism. Criminal Justice Policy Review doi: 10.1177/0887403411429724 32 Duwe, G., & Goldman, R. (2009). The impact of prison-based treatment on sex offender recidivism: Evidence from Minnesota. Sexual Abuse: A Journal of Research and Treatment, 21, 279-307. Farabee, D., & Knight, K. (2002). A comparison of public and private prisons in Florida: During- and post-prison performance indicators. Los Angeles, CA: Query Research. Gendreau, P., Little, T. & Goggin, C. (2006). A Meta-Analysis of the Predictors of Adult Offender Recidivism: What Works! Criminology, 34(4), 575-608. Government Accounting Office. (1991). Private Prisons: Cost Savings and BOP’s Statutory Authority Need to be Resolved. Report to the Chairman, Subcommittee on Regulation, Business Opportunities and Energy, Committee on Small Business, House of Representatives. Washington, DC: General Government Division, General Accounting Office. Government Accounting Office. (1996). Private and Public Prisons: Studies Comparing Operational Costs and/or Quality of Service. Report to the Subcommittee on Crime, Committee on the Judiciary, House of Representatives. Washington, DC: General Government Division, General Accounting Office. Havens, C., & Giles, K. (2009). “Minnesota may use private prison in Appleton.” Star Tribune, Accessed August 11th, 2011. (http://www.startribune.com/local/east/72081417.html). Langan, P.A., & Levin, D.J. (2002). Recidivism of Prisoners Released in 1994. Washington, DC: U.S. Department of Justice, Bureau of Justice Statistics. Lanza-Kaduce, L., & Maggard, S. (2001). The long-term recidivism of public and private prisoners. Unpublished paper presented at the National Conference of the Bureau of Justice Statistics and Justice Research and Statistics. Lanza-Kaduce, L., Parker, K., & Thomas, C.W. (1999). “A Comparative Recidivism Analysis of Releasees from Private and Public Prisons.” Crime & Delinquency, 45, 28-47. Lundahl, B., Kunz, C., Brownell, C., Harris, N., & Van Vleet, R. (2007). Privation privatization: A meta-analysis of cost effectiveness and quality of confinement indicators. Salt Lake City, UT: Utah Criminal Justice Center. 33 Minnesota Department of Corrections (2000). Adult Inmate Profile (January 1, 2000). http://www.doc.state.mn.us/aboutdoc/stats/adultprofile1-1-00.htm. Minnesota Department of Corrections (2004). Adult Inmate Profile (January 1, 2004). http://www.doc.state.mn.us/aboutdoc/stats/pdf/AdultProfile01-01-2004.pdf. Minnesota Department of Corrections (2006). Adult Inmate Profile (January 1, 2006). http://www.doc.state.mn.us/aboutdoc/stats/documents/January2006ProfileCard_fi nal.pdf. Minnesota Department of Corrections (2008). Adult Inmate Profile (January 1, 2008). http://www.doc.state.mn.us/aboutdoc/stats/documents/AdultInmateProfile01-012008.pdf. Minnesota Department of Corrections (2012). Financial Services Unit. Northcutt Bohmert, M., & Duwe, G. (2012). Minnesota’s Affordable Homes Program: Evaluating the effects of a prison work program on recidivism, employment and cost avoidance. Criminal Justice Policy Review, 23, 327-351. Oppel, Richard A. 2011. “Private Prisons Found to Offer Little in Savings.” New York Times, Accessed May 18th, 2011. (http://www.nytimes.com/2011/05/19/us/19prisons.html). Pratt, T.C. & Maahs, J. (1999). Are private prisons more cost-effective than public prisons? A meta-analysis of evaluation research studies. Crime & Delinquency, 45, 358-371. Rosenbaum, P.R., & Rubin, D.B. (1985). Constructing a control group using multivariate matched sampling methods that incorporate the propensity score. The American Statistician, 39, 33-38. Rubin, D.B. (1997). Estimating causal effects from large data sets using propensity scores. Annals of Internal Medicine, 127, 757-763. Sabol, W.J., Minton, T.D., & Harrison, P.M. (2007). Prison and Jail Inmates at Midyear 2006. Bulletin. Washington, DC: U.S. Department of Justice, Office of Justice Programs, Bureau of Justice Statistics. Selman, D., & Leighton, P. (2010). Punishment for Sale: Private Prisons, Big Business, and the Incarceration Binge. Lanham, MD: Rowman & Littlefield Publishers. 34 Shadish, W.R., Cook, T.D., & Campbell, D.T. (2002). Experimental and quasiexperimental designs for generalized causal inference. Boston: Houghton Mifflin. Shelden, R. (2010). The Prison Industry. Research Brief. San Francisco, CA: Center on Juvenile and Criminal Justice. Spivak, A.L., & Sharp, S.F. (2008). Inmate Recidivism as a Measure of Private Prison Performance. Crime & Delinquency, 54, 482-508. Stephan, J.J. (2008). Census of State and Federal Correctional Facilities, 2005. Report. Washington, DC: U.S. Department of Justice, Office of Justice Programs, Bureau of Justice Statistics. West, H.C., Sabol, W.J., & Greenman, S.J. (2010). Prisoners in 2009. Bulletin. Washington, DC: U.S. Department of Justice, Office of Justice Programs, Bureau of Justice Statistics. 35