Justice Quarterly, WA, a Longitudinal Analysis of Prisoner and Solitary Populations in Washington State, 2002-2017
Download original document:
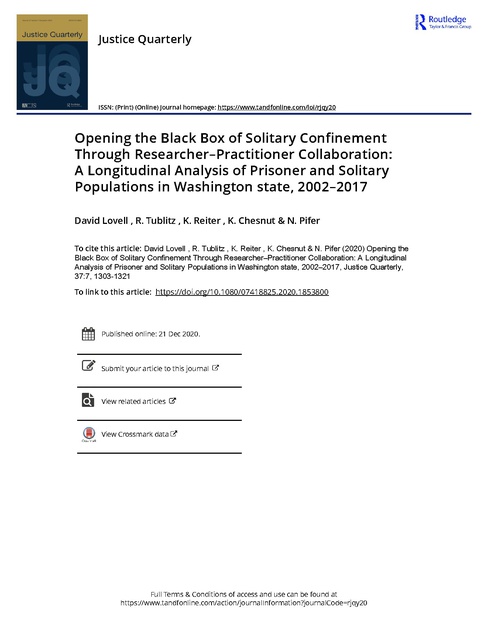
Document text
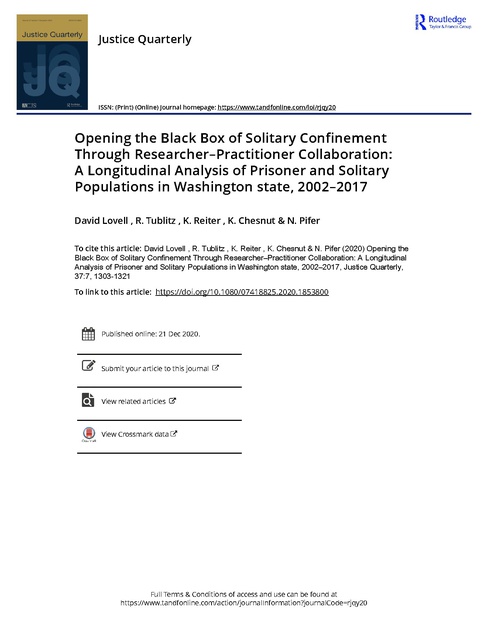
Document text
This text is machine-read, and may contain errors. Check the original document to verify accuracy.
Justice Quarterly ISSN: (Print) (Online) Journal homepage: https://www.tandfonline.com/loi/rjqy20 Opening the Black Box of Solitary Confinement Through Researcher–Practitioner Collaboration: A Longitudinal Analysis of Prisoner and Solitary Populations in Washington state, 2002–2017 David Lovell , R. Tublitz , K. Reiter , K. Chesnut & N. Pifer To cite this article: David Lovell , R. Tublitz , K. Reiter , K. Chesnut & N. Pifer (2020) Opening the Black Box of Solitary Confinement Through Researcher–Practitioner Collaboration: A Longitudinal Analysis of Prisoner and Solitary Populations in Washington state, 2002–2017, Justice Quarterly, 37:7, 1303-1321 To link to this article: https://doi.org/10.1080/07418825.2020.1853800 Published online: 21 Dec 2020. ~~ Submit your article to this journal r!1 View related articles ® View Crossmark data 8" 8" 8" CrossM<1rk Full Terms & Conditions of access and use can be found at https://www.tandfonline.com/action/journalInformation?journalCode=rjqy20 JUSTICE QUARTERLY 2020, VOL. 37, NO. 7, 1303–1321 https://doi.org/10.1080/07418825.2020.1853800 11) Check for updates Opening the Black Box of Solitary Confinement Through Researcher–Practitioner Collaboration: A Longitudinal Analysis of Prisoner and Solitary Populations in Washington state, 2002–2017 David Lovella, R. Tublitzb, K. Reiterb, K. Chesnutb,c and N. Piferd a School of Nursing, University of Washington, Seattle, WA, USA; bDepartment of Criminology, Law & Society, University of California, Irvine, CA, USA; cVera Institute of Justice, New York, NY, USA; d Criminology & Criminal Justice Program, University of Rhode Island, Kingston, RI, USA ABSTRACT ARTICLE HISTORY This article presents a rare longitudinal analysis of solitary confinement use in one state prison system: spanning 2002–2017 in the Washington Department of Corrections (DOC). An ongoing partnership with DOC officials facilitated methodological and conceptual improvements, allowing us to construct a dataset that provides a rich description of who is in solitary confinement, for how long, and why. Operationalizing solitary confinement as the intersection of the most serious custody status with the most restrictive housing location, we describe significant changes in ethnic composition and behavioral profiles of people in solitary confinement and in frequency and duration of solitary confinement use. These results suggest how particular policy interventions have affected the composition, numbers, and lengths of stay in solitary confinement. Combining longitudinal analysis and iterative engagement with DOC officials, we provide a roadmap for better understanding solitary confinement use in the United States now and in the future. Received 2 June 2020 Accepted 11 November 2020 KEYWORDS Restrictive housing; solitary confinement; prison; gangs Tens of thousands of prisoners across the United States experience solitary confinement annually (Association of State Correctional Administrators & the Arthur Liman Public Interest Program and Yale Law School (ASCA-Liman), 2015, 2018; Beck, 2015). Prisoners generally spend no more than an hour per day outside of cells the size of a wheelchairaccessible bathroom stall, and eat cold meals alone, with limited access to natural light, phones, family visits, or any human touch. Prisoners live not days, but months and years under such conditions. In tandem with mass incarceration, the use of solitary confinement expanded drastically across the United States in the 1980s and 1990s, often in modern, hyper-secure, “supermax” facilities (Reiter, 2016; Riveland, 1999; Sakoda & Simes, 2019). Though integral to incarceration since the prison was “born” and perpetually controversial (Foucault, 1977; Haney & Lynch, 1997; Rubin & Reiter, 2018; Smith, 2006), solitary CONTACT K. Reiter reiterk@uci.edu ß 2020 Academy of Criminal Justice Sciences 1304 D. LOVELL ET AL. Estimates of how many people experience solitary confinement annually range from 68,000 prisoners to 18% of all prisoners in the United States, or over 250,000 people (ASCA-Liman, 2015; Beck, 2015). To address definitional debates underlying conflicting estimates, Mears et al. recently suggested a four-dimensional conceptual framework – goal, duration, quality, and intentionality – to describe the constellation of factors that make up solitary confinement (or “restrictive housing”) practices (2019, p. 1434). The operational focus of our alternative approach allows us to bypass arguments about how to define solitary confinement, a conceptually and ethically controversial practice. Rather, our operational definition applies the near-universal correctional functions of classification and movement to identify the sites and subjects of solitary confinement from correctional tracking records. These methods permit consistent, robust analyses of who is subjected to solitary confinement and the association of this experience with institutional misconduct and other factors. Trajectories of solitary confinement placement confinement has come under renewed scrutiny in the last decade (Reiter, 2018; ASCALiman, 2015). Federal and state correctional systems have begun to experiment with mitigation and alternative programs. Here, we focus on a 15-year period during which the Washington Department of Corrections (DOC) attempted to confront these issues and ask whether and how a prison system might reduce its use of solitary confinement. The question of whether a prison system might change direction, including how the practice of solitary confinement might be constrained, has animated criminological scholarship over decades (Jacobs, 1977; Liebling, 1999; Petersilia, 1991; Reiter, 2016; Rhodes, 2004; Rubin & Reiter, 2018). A longitudinal, quantitative dataset with which to assess these questions, however, is rare. Our dataset, analyzed in collaboration with practitioner partners, allows us to look both at individual factors, such as how many gang members with violent infraction histories are placed in solitary confinement for how long in any given year, and at institutional factors, including demographic shifts and policy changes, which influence behavioral patterns (Haney, 2018; Liebling, 1999; Toch, 1977; Toch, Adams, & Grant, 1989). Where scholars have used point-in-time datasets to examine the relationship between individual and institutional factors in understanding the use and effects of solitary confinement, controversies abound over how to define and operationalize the practice (Kurki & Morris, 2001; Mears et al., 2019; Naday et al., 2008; Reiter, 2016). We identify which prisoners are subjected to the aversive conditions described above in terms of two factors: (1) whether they are living in units engineered to lock them down (location) and (2) the rules governing how long they stay, their conditions of confinement, and movement (custody status). Here, these measurement principles are applied to a rich administrative dataset to ask: (1) Who is in solitary confinement, for how long, and why? (2) How, if at all, do their individual characteristics, including ethnicity, gang status, and behavioral profiles change over time? (3) What patterns emerge from this analysis? We show how the distribution and extent of solitary confinement use in Washington has shifted with institutional vicissitudes in demographics, capacity, gang management policies, programming, and classification systems. ® 1305 Previous studies have reached conflicting conclusions about whether solitary confinement has a disparate impact on groups defined by race or ethnicity. Studies focusing on patterns in disciplinary infractions and solitary confinement placements over four to six years tend to find minimal disparities (Cochran, Toman, Mears, & Bales, 2018; Tasca & Turanovic, 2018), while point-in-time comparisons of demographics of solitary confinement units with general population (GP) units consistently find non-white prisoners over-represented in solitary confinement (Reiter, 2012; Schlanger, 2012). A recent study analyzed a survey that asked state prison systems to self-report solitary confinement and gang-affiliated populations; prisoners classified as gang members were over-represented in solitary confinement across the United States (Pyrooz & Mitchell, 2020). The study does not mention race, but others have noted the longstanding ties between race and gangs in US prisons (Berger 2014; Bloom & Martin, 2013; Reiter 2016), strengthening Pyrooz and Mitchell’s recommendation to “integrate measures of gang affiliation into correctional research” (2019, p. 22), as we do in our analysis. The relationship between solitary confinement and institutional order is also contested (Briggs, Sundt, & Castellano, 2003; Lovell, Johnson, & Cain, 2007). One recent study among men in a three-year cohort in a mid-western DOC found that disciplinary segregation was associated with a greater probability of misconduct (Labrecque & Smith, 2019), but another study, among men in a two-year cohort in the Oregon DOC, found that disciplinary segregation was not a significant predictor of subsequent institutional misconduct (Lucas & Jones, 2019). Our dataset permits an evaluation of longer-term patterns of misconduct, in and out of solitary settings. One recent study expanded the usual short periods of analysis described in preceding studies about both race and misconduct, using nearly a decade (1987–1996) of data from Kansas: a prison system small enough (5–7000 prisoners) to allow tracing of bed-level data to examine individual correlates of solitary confinement placement, such as race, and also patterns in frequency and duration of solitary confinement over time (Sakoda & Simes, 2019). Our study takes an even broader scale approach: examining populations in and out of solitary confinement over 15 years, with 15,000 or more prisoners per cohort, following particular individuals and groups over decades of criminal and correctional history. Attending to broader institutional forces at play over our study period is critical to our approach. Lynch recently argued that in studies of sentencing, findings are often “operationalized as a single end-stage outcome that is unmoored from the social, organizational, and institutional forces that help produce a class of defendants to be sentenced” (Lynch, 2019, p. 1159). This critique could just as readily be applied to studies of solitary confinement (Cochran et al., 2018; Logan et al., 2017) in which disparities in outcomes and differences in personal and behavioral characteristics of prisoners are analyzed with limited attention to institutional patterns such as fluctuations in bed capacity, shifts in demographic make-up, and reforms or retrenchments in policies governing solitary confinement placement and release. Our longitudinal dataset allows us to generate individual-level and aggregate statistics on histories and outcomes during incarceration, and to place findings in the context of broader institutional forces shaping those patterns. JUSTICE QUARTERLY ® 1306 D. LOVELL ET AL. 1 In a timely example of how relevant the analysis in the instant study is, DOC research staff recently noted that they “had some concerns” with these numbers as originally reported and have revised them upwards, re-calculating that, in 2015, 3.4% of the state prison population was in “restrictive housing” according to the ASCA-Liman Definition, and, in 2017, 4.1% of the state prison population was in “restrictive housing” by this definition. E-mail communication with DOC Department of Research, dated Sept. 25 and Sept. 28, 2020, on file with authors. The ASCA-Liman report defines “restrictive housing” as “separating prisoners from the general population and holding them in cells for an average of 22 or more hours per day for 15 continuous days or more.” This analysis draws on a longitudinal administrative record set of the entire DOC population on six evenly-spaced snapshot intervals (July 1, 2002, 2005, 2008, 2011, 2014, and 2017): subject-level demographic records (N ¼ 57,130), and event-level records of admissions and releases (266,266), prison sentences (230,833), custody assignments (1.2 million), infractions (630,088), and inter-facility movements (2.4 million). Discussions with DOC research office partners about how best to meet the data Data and methods The administrative dataset analyzed here was collected as part of a multi-method project, also using ethnographic, interview, and archival data, to evaluate solitary confinement use over time in Washington DOC (Reiter et al., 2020). This project extends a decades-long collaborative relationship between researchers and DOC: first between the University of Washington (UW) and DOC through the Mental Health Collaboration (Allen, Lovell, & Rhodes, 2001); later in a UW-led multi-method systematic survey of Washington’s solitary confinement population in 1999–2000 (Lovell, 2008; Lovell, Cloyes, Allen, & Rhodes, 2000; Rhodes, 2004); and finally, in this study, replicating and extending the 2000 study in collaboration with an original member of both previous studies. In rates of overall incarceration and solitary confinement use, Washington DOC is below average: it has the 12th lowest rate of incarceration among the states (Kaeble & Cowhig, 2018), and as of 2018, its reported proportion of population in “restrictive housing” (2.3%) was half the national average (4.5%) (ASCA-Liman, 2018, p. 13).1 In terms of willingness to collaborate with researchers, however, Washington DOC is above average: current and former DOC leadership have agreed there are knowledge gaps around solitary confinement, invited scholars and advocates alike to analyze and critique policies in order to address these gaps, and participated actively in collaborations: both facilitating access to the administrative data underlying the analyses presented here and helping to interpret results. In particular, Eldon Vail and Dan Pacholke, nationally recognized correctional policy experts, led Washington DOC during part of our study period and consulted with us on interpretation of findings. Research about solitary confinement use has been produced through practitioner–researcher collaborations in a number of states, including Colorado (O’Keefe et al. 2011), Florida (Mears & Bales, 2009), Kansas (Sakoda & Simes, 2019), and Oregon (Pyrooz, Labrecque, Tostlebe, & Useem, 2020). Few, however, have attempted the quantitative and qualitative depth of this project, which is more comparable to the New York studies of Toch and colleagues (Toch, 1977; Toch et al., 1989), conducted as the new “supermax” era was coming upon us in the 1980s, or the California studies by Petersilia on re-entry and community supervision (Petersilia 2009). Ours represents an intergenerational academic–practitioner collaboration spanning both eras. ® 1307 In Washington DOC policy (2020: 320.250), maximum custody status is the highest level of custody classification. Maximum custody prisoners are assessed in formal hearings to pose a sufficient risk to safety – whether their own or others’ – to warrant holding them for an extended period in a maximum-security location, isolated by architecture, procedure, and staffing. As legal expert Fred Cohen notes, maximum custody is a risk-based classification, justified as a preventive measure rather than a punitive sanction (Cohen, 2008). In Washington DOC, prisoners first enter solitary confinement through short-term administrative segregation (Ad-Seg) placements, usually awaiting adjudication following an infraction. Infraction of a specific prison rule may result in a disciplinary hearing and the sanction of a disciplinary segregation (DSeg) placement. Alternatively, multiple infractions, other behavior patterns, or an extended stay in administrative segregation may lead to a re-classification as maximum custody (Max). Terminology needs of our study, exemplifying our academic–practitioner collaboration, led to two major expansions of the scope and power of this dataset. First, to assess how solitary confinement populations had changed since the 2000 UW study, we requested archival information on prisoners in any form of solitary confinement on our snapshot dates. Lacking ready capacity to identify these prisoners, DOC offered to provide data for all prisoners in custody on these dates, leaving it to us to identify who was in solitary confinement and when. Our willingness to pick our own apples from the DOC data tree led to a 30-fold expansion of our subject pool, permitting longitudinal comparisons between solitary confinement and GP prisoners. Second, DOC provided us all Washington prison sentences in the entire history of prisoners in our vastly expanded dataset, rather than only the index offense data we had requested. Although information about currently active convictions accompanies prisoners as they move through DOC, retrospectively retrieving links between court and correctional records is complicated by the multiplicity of charges, sentencing policies, and admission statuses that may apply. Recognizing a systematic problem when we showed them a pattern of missing data, DOC provided the entire prison conviction history for the 57,000 prisoners in our expanded subject population, allowing us both to identify the most serious current offense and to provide a consistent measure of prisoners’ criminal histories. Source data were compiled cohort by cohort, applying uniform coding procedures to compile event-level data into a subject-level dataset. We computed the facility location and custody status of every prisoner in the system throughout each admission, length of stay (LOS) at each location, and subject-level summaries of numbers and rates of relevant events, such as infractions. Compilation codes were tested and modified until they yielded consistent and plausible counts and summary statistics (e.g. no negative values for LOS or rates) across all prisoners in six snapshot cohorts. We also use some inferential statistics (e.g. chi-square and t-tests) in the analyses we present to test for differences across cohorts and groups. JUSTICE QUARTERLY ® 1308 D. LOVELL ET AL. 2 Intra-facility housing changes and periods spent in recently decommissioned internal solitary confinement units are better captured in our related, intensive field study dataset of 106 solitary confinement prisoners (Reiter et al., 2020). To contextualize findings on the size and characteristics of Washington’s solitary confinement population, we first describe overall patterns in the state prison population between 2002 and 2017. Table 1 displays counts and demographic, crime type, sentence length, and gang affiliation characteristics for the entire prison population incarcerated on each of the six snapshot dates. Washington State’s prison population grew by 13%, despite changes in sentencing policy (SHB2338, 2002) that were expected to reduce imprisonment by lessening penalties and providing treatment Results In DOC, Intensive Management Units (IMUs) are the most secure housing facilities. The term “supermax” is not a category of institution in DOC; instead the state has five IMUs, located at Clallam Bay Corrections Center (CC), Monroe CC, Washington CC (“Shelton”), Stafford Creek CC, and the Washington State Penitentiary (called “Walla Walla” or the “concrete mama” (Hoffman & McCoy, 2018)). IMUs feature distinct security perimeters with advanced technology for controlling entrances, gates, and doors; strict procedures for prisoner movement; and no normal occasions for prisoners to share space with others unless shackled. Though exact conditions (like cell size and degree of access to natural light) vary across IMUs, the uniformly restrictive conditions impose intense isolation (often for extended periods of time) comparable to conditions in other state supermaxes. IMUs are adjacent to the “main institution” (a correctional center or complex may have multiple facilities, or stand-alone buildings, sharing a common Superintendent) to allow escorting prisoners on foot without delay. As a Lieutenant at Shelton said during a prison visit: “Nothing happens fast around here except going to the IMU.” Transfers between facilities are recorded in DOC’s movement records, allowing us to identify who was placed in IMUs and for how long. Transfers in and out of cells within a facility, however, are recorded as housing changes: likely 50 million in number for our subjects, vastly exceeding our and DOC’s ability to retrieve and compile, absent unlimited resources.2 Therefore, inter-facility movement records in our data do not capture prisoners isolated on Ad-Seg or D-Seg status (Ad/DSeg status) inside a main institution. Importantly, Ad/DSeg prisoners, who were living under comparably stringent conditions as IMU-Max prisoners, in two decrepit segregation units within the main institutions at two of Washington’s oldest prisons – Walla Walla and Monroe – are not captured in our data. These two units, with a combined capacity of 250, closed in 2011, but were replaced (and then some) by 200 new IMU beds at each prison. Our inability to identify all such Ad/DSeg prisoners through movement records requires caution in how the terms “IMU” versus “solitary confinement” are used in our findings. Because of this limitation, we center our trend and comparative analyses on the maximum custody group, who are reliably identified over the entire course of our study period and whose long-term presence in maximum security settings raises the sharpest ethical issues (Lovell, 2014). ® 63% 19% 10% 8% 8% 92% 19% 33% 29% 20% 2005 44% 20% 18% 18% 0% 62% 19% 11% 9% 8% 92% 17% 32% 28% 23% 2008 46% 20% 19% 15% 0% 60% 19% 12% 9% 8% 93% 16% 34% 25% 25% 2011 46% 20% 20% 14% 0% 61% 18% 13% 9% 8% 92% 13% 35% 26% 27% 2014 JUSTICE QUARTERLY 42% 17% 17% 23% 1% 5% 10% 9% 2% 74% 17,625 101.7 120.4 6% 10% 8% 2% 75% 17,288 99.8 117.3 6% 9% 6% 2% 78% 17,308 94.8 112.1 5% 9% 5% 1% 80% 16,852 89.1 107.1 Cohort Table 1. Washington DOC population characteristics, 2002–2017. 2002 Age at snapshot (in years) 18–25 21% 26–35 33% 36–45 29% Over 45 17% Gender Female 7% Male 93% Race/ethnicity White, Non-Hispanic 60% Black, Non-Hispanic 21% Hispanic 12% Other/Unknown 7% Most serious offense at conviction Violent, non-sex 41% Sex 17% Property 15% Drug/other 25% Missing 2% Sentence length (in months) Mean 87.9 Standard deviation 104.8 Gang affiliation by racial/ethnic STG White 5% Black 9% Hispanic 4% Other 1% No gang affiliation 81% Total prison population 15,907 1309 2017 11% 34% 27% 28% 8% 92% 60% 18% 14% 9% 48% 19% 19% 13% 0% 100.9 124.6 5% 10% 9% 2% 74% 17,943 3 General crime types were derived from DOC codes in the administrative data. Violent, non-sex offenses include murder, manslaughter, robbery, and assault; sex offenses include rape, sexual assault, child molestation, and failure to register as a sex offender; property crimes include arson, burglary, theft, forgery, trafficking, and possession of stolen property; drug crimes include manufacturing, delivering or possession with intent to distribute, and possession of a controlled substance. 4 To avoid confusion, we follow DOC’s terminology with the term “Hispanic,” which DOC codes separately from race as “Hispanic Origin” (Y/N); but we apply these data to define mutually exclusive categories: “White, non-Hispanic” includes any individual whose race is listed as White and who is not classified as Hispanic Origin; “Black, nonHispanic” includes any individual whose race is listed as Black and not identified as Hispanic; “Hispanic” includes any individual whose ethnicity is listed as Hispanic or Latino, regardless of any other racial identification; “Other/ Unknown” includes any individual whose race is listed as Asian/Pacific Islander, Native American/American Indian, Other, Unknown and whose ethnicity is not Hispanic. alternatives for drug-related offenses. The proportion of prisoners incarcerated for drug or other offenses declined substantially, while those incarcerated for violent, non-sexual offenses increased by nearly 17% between 2002 and 2017 (p < 0.001).3 Reflecting the shift toward more violent offenses, average sentence lengths increased significantly, as did the average age of prisoners. The proportion of Hispanic prisoners increased by 17%, while the proportion of Black, non-Hispanic prisoners decreased by 16% (p < 0.001), and White, non-Hispanic representation remained stable.4 Affiliation with security threat groups (STG) or prison gangs, increased as well: in 2017, over one in four prisoners (26%) was identified as a member of an STG, up from 19% in 2002. The growth of gang affiliation was not equally distributed across racial Source: Authors’ calculations. Washington State Department of Corrections. ® 1310 D. LOVELL ET AL. 5 Rates of gang affiliation by racial/ethnic group were generated by dividing the total number of members in each racial/ethnic group identified as an STG member by the total number of prisoners of each racial/ethnic group. Table 1 displays the STG membership by racial/ethnic affiliation of STGs, grouped from detailed STG data provided by DOC. STGs identified as “White” affiliated included Biker, Skinhead, White Supremacist and Security Threat Concern; ~o, “Black” affiliated included Black Gangster Disciples, Blood, Crip, and Vice Lord; “Hispanic” affiliated included Norten ~o, Paisas, La Fuma, Cuban, and Hispanic-Other; “Other” affiliated included Asian and Other. Suren 6 Our original analysis identified an even larger proportion of prisoners in this “Other-Max” group; our practitioner collaborators thought more than 10% was an unlikely proportion of prisoners to be assigned max custody status but still awaiting placement in an IMU or similar facility. We then further evaluated whether some of those “OtherMax” prisoners were housed out-of-state. Indeed, when we examined individual cases in the original movement files, we found this was true, leading us to better specify and exclude those prisoners in our sample, of any custody status, who were housed out of state. Table 2 presents trends in solitary confinement use by both custody status (classification) and location (facility). We distinguish four groups either classified at the highest custody level (Maximum, labeled “Max”), or located in the most restrictive locations (IMUs). At the center of our analysis are prisoners both classified Max and housed in IMUs (denoted by IMU-Max). Next are prisoners who have not been reclassified Max, but are housed in IMUs for administrative or disciplinary segregation (IMU-Ad/DSeg). Third, for treatment purposes, some Max prisoners are housed at the special offender unit (SOU) at Monroe, designed to address serious behavioral health needs, or at the inmate transitional pod (ITP) at Clallam Bay, a program-focused unit for prisoners transitioning out of solitary confinement (denoted by Max-Tx). Finally, a residual group of Max prisoners could not be assigned a facility type because, on the snapshot date, they were on hospital or court release, or awaiting transfers to an IMU, SOU, or ITP (Other-Max).6 Solitary confinement use (in IMU-Max, IMU-Ad/Dseg, and Total IMU) far outpaces population growth over our study period in the state, growing at least 130% (in IMUMax), compared to a 13% growth in the state prison population. As explained earlier, IMU-Max represents a clearly defined population, with reliable snapshot counts for prisoners subjected to long-term solitary confinement over the entire study period, but it excludes prisoners in Ad/DSeg either in the IMU, or in other within-facility units, not identifiable in the between-facility movement records we analyze. Figure 1 illustrates differences in rates and patterns of growth in IMU-Max and total prison populations, accompanied by changes in average LOS for the IMU-Max group on their snapshot date assignments. One-day counts capture those physically held in IMUs on snapshot dates, and demonstrate that a small, but increasing proportion of Washington’s prison population was held in solitary confinement across snapshots, in both IMU-Max and IMU-Ad/DSeg groups. One-day counts, however, do not account for movement in and out of IMUs Disentangling the solitary population and ethnic groups.5 While rates of gang affiliation for White, non-Hispanic prisoners remained relatively low over the 15-year period, gang affiliation among prisoners of color increased substantially: between 2002 and 2017, the proportion of Black, nonHispanic prisoners classified as gang-affiliated rose from 35% to 41%; for Hispanic prisoners, from 28% to 53%, a sharp increase with substantial consequences for solitary confinement practices. ® Table 2. Solitary confinement in Washington State, 2002–2017. Cohort 2002 2005 2008 2011 2014 2017 % Num. % Num. % Num. % Num. % Num. % Custody and confinement level IMU-Max IMU-Ad/DSeg Max-Tx Other-Max General population Out of state/unknown Total IMUb Total maximum custodyc 149 105 18 34 15,499 102 254 201 0.9% 0.7% 0.1% 0.2% 97.4% 0.6% 1.6% 1.3% 228 144 50 55 16,270 105 372 333 1.4% 0.9% 0.3% 0.3% 96.5% 0.6% 2.2% 2.0% 338 337 44 11 16,438 140 675 393 2.0% 1.9% 0.3% 0.1% 95.0% 0.8% 3.9% 2.3% 472 177 35 27 16,440 137 649 534 2.7% 1.0% 0.2% 0.2% 95.1% 0.8% 3.8% 3.1% 283 291 42 20 16,893 96 574 345 1.6% 1.7% 0.2% 0.1% 95.8% 0.5% 3.3% 2.0% 342 260 52 18 17,121 150 602 412 1.9% 1.4% 0.3% 0.1% 95.4% 0.8% 3.4% 2.3% Cumulative days spent in IMU (any custody status)d Mean (St. Dev.) Not placed in IMU 1–45 days 46–90 days 91–365 days 366 days or more (>1 year) At least 1 day in IMU 43.1 12,062 2128 499 728 490 3845 (211.5) 75.8% 13.4% 3.1% 4.6% 3.1% 24.2% 47.6 12,673 2344 487 755 593 4179 (230.3) 75.2% 13.9% 2.9% 4.5% 3.5% 24.8% 56.2 12,533 2606 583 890 695 4774 (256.8) 72.4% 15.1% 3.4% 5.1% 4.0% 27.6% 74.6 12,120 2535 610 1041 981 5167 (302.7) 70.1% 14.7% 3.5% 6.0% 5.7% 29.9% 80.4 11,863 2854 810 1050 1048 5762 (327.1) 67.3% 16.2% 4.6% 6.0% 5.9% 32.7% 82.4 11,847 2985 928 1075 1108 6096 (330.0) 66.0% 16.6% 5.2% 6.0% 6.2% 34.0% (136.2) (124.6) 15,907 306.0 116.9 (239.2) (121.2) 16,852 283.9 90.6 (192.9) (116.9) 17,308 347.7 127.8 (273.2) (138.5) 17,288 325.8 66.4 (316.7) (77.9) 17,625 214.0 70.9 Days in IMU by custody and confinement level at snapshot date: Mean (St. Dev.) IMU-Max IMU-Ad/DSeg Total prison population 227.0 114.7 (129.6) (79.6) 17,943 ® 1311 Source: Authors’ calculations. Washington State Department of Corrections. Changes in the use of local segregation for disciplinary and administrative purposes (outside of IMUs, for prisoners classified lower than Max Custody) likely affect the counts of IMUAd/DSeg populations, particularly in early cohort years. b Total IMU is the sum of all prisoners living in IMU units on July 1st, including (i) IMU-Max, those on maximum custody housed in IMUs, and (ii) IMU-Ad/DSeg, those who are housed in IMUs on lower custody levels, including administrative segregation, disciplinary segregation and awaiting hearings. c Total Maximum Custody consists of three groups, all classified as maximum custody: (i) those housed in IMUs (IMU-Max), (ii) those in SOU or ITP units (Max-Tx), and (iii) those located elsewhere (Other-Max). d Days spent in IMU represents cumulative days spent in IMU until the snapshot date for all prisoners, regardless of custody classification, during their current prison admission. a JUSTICE QUARTERLY Num. 1312 9 D. LOVELL ET AL. 350 300 0 "' 0 "'e 250 0 ,l: "C OJ) u" 200 .c " 150 OJ) ~" ~ 100 i::: 50 0 2002 (=100) - 2005 2008 2011 2014 2017 Snapshot Year !MU-Max Population - - !M U-Max LOS - Total Prison Population Figure 1. Percentage change in IMU-Max population, IMU-Max length of stay (LOS), and total prison population (indexed at 2002), Washington DOC, 2002–2017. at other points. To better understand both the prevalence and duration of placement in solitary, we used event-level movement information to calculate the cumulative amount of time each prisoner spent in solitary confinement from admission to snapshot date. Over the study period, a majority of prisoners in DOC in each snapshot cohort were never placed in solitary confinement, but a substantial and growing proportion of prisoners had spent time in these units. The proportion of prisoners spending at least one day in an IMU between their prison admission and snapshot dates had increased from 24.2% in 2002 to 34% in 2017. Prisoners in 2002 spent an average of 6 weeks in IMUs from admission to snapshot; by 2017, time spent in IMU increased significantly to an average of 12 weeks (p< 0.001). Changes in mean values are skewed by a few outliers, who have spent their entire (long or life) prison sentences in an IMU, beginning decades before and extending through the study period. To counter the skew, we binned cumulative days in IMU into distinct groups: 0 days, 1–45 days, 46–90 days, 91 days to 1 year, and over 1 year.7 Pooling across all cohorts, we find that more than half of those who spent at least one day in an IMU stayed for between 1 and 45 days, cumulatively. The second largest group (18.6%) cumulatively spent between three months and one year in solitary confinement, and a substantial proportion (16.5%) of those placed in an IMU spent more than 1 year there. The changing distribution of cumulative time spent in IMUs reinforces the finding that average time spent in solitary increased over the study period. More prisoners spent at least one day in IMU, and proportions of prisoners in each cumulative LOS group increased substantially, led by those spending between 46 and 90 days and those spending more than one year in IMU. In total, our data 7 Here, the 45-day cut point reflects institutionally-mandated administrative hearings required to extend or release an individual from administrative segregation. Likewise, for those classified as Max, (re-)classification reviews only happen every 6–12 months, as reflected in the overall longer mean lengths of stay for IMU-Max, as opposed to IMU-Ad/DSeg groups. Both represent examples of policies driving patterns in lengths of stay. JUSTICE QUARTERLY 1313 8 This analysis uses the person (in custody as of the snapshot date) as the unit of analysis. Even if a single person has multiple stays in an IMU during the current admission up to the snapshot date, they would be counted only once as “having spent at least one day in an IMU.” We further examined the average percentage of days spent in an IMU out of the total number of days in prison up to the snapshot date for each cohort, finding an increasing proportion of prison time spent in IMUs across the cohorts. While not presented here in detail, this finding reinforces the trends in the cumulative time spent in IMU and average LOS analyses. 9 Unlike the cumulative days in IMU calculations, the average length of stay by classification and confinement levels presented here do not cumulate days in IMU facilities. Here, each placement in a distinct IMU facility is analyzed as a separate placement term. Thus, if one prisoner is placed in IMU facility A, and subsequently moved to IMU facility B, the length of stay in each placement will be counted separately. (To the extent individuals have consecutive stays across multiple IMUs, then, these numbers might undercount average lengths of total stay.) Length of stay is calculated from admission date in the current incarceration up until the snapshot date. 10 The general population (GP) excludes: prisoners housed in IMUs, prisoners with a max custody classification held in other locations (i.e. those in SOU, ITP, or “Other Locations”), prisoners held out of state, and prisoners whose locations or custody statuses were unknown. Table 3 compares demographic, criminal history, gang status, and behavioral histories of IMU-Max and GP prisoners across snapshots,10 showing significant differences between these groups. In both populations, White, non-Hispanic prisoners represented the largest group. However, compared to the GP, prisoners of Hispanic ethnicity were substantially over-represented in IMU-Max, while White, non-Hispanic prisoners are under-represented (p < 0.001). Black, non-Hispanic people were slightly underrepresented among IMU-Max prisoners, relative to their presence in the GP. These disparities diverge over time: the proportion of Hispanic prisoners in the IMU-Max population increased by nearly 34% between 2002 and 2017, while the proportions of all other racial and ethnic groups decreased. IMU-Max prisoners have more serious conviction and in-prison misconduct histories than GP prisoners. Across cohorts, nearly three-quarters (73%) of IMU-Max prisoners were convicted of non-sexual violent offenses, compared with just 44% of GP prisoners. The IMU-Max group were also first convicted of prison-eligible offenses at a younger age, on average, than those in the GP (p < 0.001). Further, in-prison misconduct rates were higher and more serious for the IMU-Max group: annual infraction rates for these prisoners were more than double GP rates, and IMU-Max prisoners committed far more violent infractions and staff assaults than those in GP The maximum custody IMU population demonstrate a greater prevalence of IMU placement across the population over time, and an increasing proportion of prison time spent in IMUs.8 In addition to examining cumulative days spent in IMU for the full prison population, we also calculated mean lengths of stay (LOS) in IMUs for both the IMU-Max and IMU-Ad/DSeg groups.9 Both groups spent substantial amounts of time in IMU settings, although, as expected, those in IMU-Max had markedly longer stays in IMU than the IMU-Ad/DSeg group. Across the study period, average time in IMU-Max ranged from 7 to 12 months, compared to 2 to 4 months for the IMU-Ad/DSeg group. The mean LOS for IMU-Max fluctuated: generally increasing until 2011, followed by a decline through 2017 to a level just below the mean LOS in 2002 (Figure 1). For the IMU-Ad/DSeg group, mean LOS dropped even more substantially after 2011. Changes in average LOS for both groups were a factor in periods of growth in total IMU populations prior to 2008, as well as in declines of IMU populations after 2011. ® 1314 Table 3. Comparison of IMU-Max and General Prison populations, Washington DOC, 2002–2017. Cohort 2002 Background characteristics Age at snapshot (years)a 18–25 36% 26–35 40% 36–45 17% Over 45 7% Race/ethnicitya Black, non-Hispanic 19% Hispanic 20% Other/unknown 13% White, non-Hispanic 48% Most serious offense at convictiona Violent, non-Sex 68% Sex 15% Property 8% Drug/other 9% Missing 1% Age of first conviction (years) a Under 18 12% 18–25 69% Over 25 20% In-prison behavioral profile Gang affiliation by racial/ethnic STGa White 14% Black 22% Hispanic 21% Other 3% No gang affiliation 40% Annual infraction ratea Mean 8.3 St. Dev. 7.6 Violent infractionsa Mean 4.0 St. Dev. 5.8 Staff assaultsa Mean 1.2 St. Dev. 3.3 Total population 149 2005 Gen. Pop. IMU-Max 2008 Gen. Pop. IMU-Max 2011 Gen. Pop. IMU-Max 2014 Gen. Pop. IMU-Max ® 2017 Gen. Pop. IMU-Max Gen. Pop. 21% 33% 29% 17% 24% 40% 22% 13% 19% 32% 29% 20% 31% 43% 15% 12% 16% 32% 29% 23% 24% 45% 18% 13% 15% 34% 26% 25% 19% 41% 20% 19% 13% 34% 26% 27% 20% 47% 20% 13% 11% 34% 27% 29% 21% 11% 7% 60% 16% 22% 8% 55% 19% 10% 8% 63% 15% 30% 6% 49% 19% 10% 9% 62% 20% 29% 7% 44% 19% 12% 9% 61% 14% 37% 5% 44% 18% 12% 9% 62% 17% 27% 9% 47% 18% 13% 9% 60% 41% 17% 16% 25% 2% 66% 14% 10% 9% 0% 42% 17% 17% 23% 1% 70% 9% 14% 7% 0% 43% 20% 19% 18% 0% 74% 11% 11% 4% 0% 45% 21% 19% 16% 0% 78% 8% 10% 4% 0% 45% 20% 20% 14% 0% 75% 7% 11% 7% 0% 48% 20% 20% 13% 0% 4% 45% 51% 9% 69% 22% 3% 45% 52% 10% 69% 21% 3% 45% 52% 10% 65% 25% 3% 46% 51% 8% 67% 25% 3% 46% 51% 8% 69% 23% 3% 45% 52% 4% 9% 4% 1% 81% 21% 14% 22% 1% 43% 5% 9% 4% 1% 81% 20% 12% 39% 1% 28% 5% 9% 5% 2% 79% 15% 14% 33% 3% 36% 5% 10% 7% 2% 76% 15% 11% 40% 4% 31% 5% 10% 8% 2% 75% 14% 16% 32% 4% 33% 4% 10% 8% 2% 76% 1.3 2.4 5.1 7.8 1.1 1.8 5.3 5.4 1.1 2.0 4.2 4.9 1.0 1.7 4.7 5.9 1.0 1.8 4.9 6.7 1.1 1.9 0.5 1.5 3.3 4.5 0.4 1.4 3.3 4.2 0.5 1.5 3.0 4.0 0.5 1.6 3.3 4.3 0.5 1.6 3.0 3.4 0.5 1.6 0.1 0.4 15,499 0.7 2.2 228 0.0 0.4 16,270 0.7 2.0 338 0.0 0.4 16,438 0.7 2.1 472 0.1 0.5 16,440 0.8 2.5 283 0.1 0.5 16,893 0.6 2.0 342 0.1 0.5 17,121 Source: Authors’ calculations. Washington State Department of Corrections. a Statistically significant differences between IMU-Max and general population (Gen. Pop.) at p < 0.001 (for categorical, chi square; for numeric, and t-test). D. LOVELL ET AL. IMU-Max JUSTICE QUARTERLY 1315 11 Violent infractions include seven infraction types: aggravated assault on another offender, fighting, possession of a weapon, aggravated assault on a staff member, sexual assault of a staff member, assault on another offender, sexual assault of another offender, and assault on a staff member. Our findings draw on an especially robust dataset, including: (1) multiple individual characteristics, like gang status and infraction rates, each one of which has constituted the sole focus of previous analyses; (2) snapshot data that covers both the entire prison population and each individual’s entire criminal and incarceration history; and (3) a 15-year period of analysis over six snapshot dates, a longer time period than in previous studies of solitary confinement. Such a rich dataset makes a succinct analysis of a subset of findings challenging to present. Here, we focus on our analytic methods, an overview of the characteristics of people in and out of solitary confinement, and overall patterns in solitary confinement use. First, we measure the sites, subjects, and varieties of solitary confinement in terms of the intersection of location and custody status. This operational taxonomy, along with the prisoner characteristics associated with solitary confinement placements, was achieved by developing an extensive population analysis script that compiled a correctional dataset tracking events, movements, and dispositions into an analytic dataset permitting analysis of patterns of prisoner behavior and facility placements over time. Our multi-generational researcher-practitioner collaboration with Washington DOC facilitated both obtaining and interpreting this data. In turn, we hope our operational taxonomy will facilitate more precise measurements of solitary confinement use, applicable and comparable across the vicissitudes of different correctional systems’ varied labels for security levels, housing locations, and solitary confinement practices (Mears et al., 2019). Second, we provide an overview and comparison of characteristics of people in solitary confinement, focusing on the specifically targeted IMU-Max group to provide a Discussion (p < 0.001).11 Nevertheless, serious misconduct appeared to decline substantially across IMU-Max prisoner snapshots (but not for GP), with average annual infraction rates among IMU-Max prisoners falling from 8.3 in 2002 to 4.9 in 2017 (p < 0.001), average numbers of violent infractions decreasing from 4 to 3 (p < 0.05), and average numbers of staff assaults decreasing from 1.2 to 0.6 (p < 0.05). Gang members were substantially over-represented in IMU-Max compared to GP (66% to 22%, pooled across all snapshot years). While the prevalence of gang membership grew in both groups over time, patterns of gang affiliation across racial-ethnic sub-categories behaved differently within the IMU-Max and GP groups. Among GP prisoners, the proportion of those affiliated with Hispanic gangs grew by 118% from 2002 to 2017; among IMU-Max prisoners, Hispanic gang membership grew substantially (55%), but at a lower rate than in the GP. Black gang membership, on the other hand, grew by just 7% in the GP, but fell by 24% among IMU-Max prisoners. Explaining these patterns is outside the scope of the present analysis, but the scale of divergence in patterns across both racial-ethnic sub-categories of gang affiliates and GP and IMU-Max populations merits future attention. ® 1316 D. LOVELL ET AL. clear contrast to GP prisoners. Over time, the average IMU-Max prisoner was increasingly likely to be older, Hispanic, convicted of a violent offense, and gang affiliated, but decreasingly likely to have assaulted a staff member. Like Pyrooz and Mitchell (2020), we find gang members over-represented in solitary confinement relative to their representation in the general prison population. We also find that Hispanic prisoners are increasingly over-represented in solitary confinement, providing evidence of the racially disproportionate impact of solitary confinement (Reiter, 2012; Sakoda & Simes, 2019; Schlanger, 2012). Our longitudinal analysis shows this disproportion steadily increasing over time, at a faster rate than gang membership in the general prison system, which increased only slightly over our period of analysis. As in other studies finding misconduct associated with solitary confinement placement (Labrecque & Smith, 2019), we find that prisoners in solitary confinement have significantly and consistently higher annual infraction, violent infraction, and staff assault rates than GP prisoners. However, all three measures of infractions, despite remaining fairly stable throughout the system, generally declined in IMU-Max over time. Rendering population patterns visible also renders visible new questions about what combination of individual behavior patterns and institutional policies produce the changes we see. Have IMU-Max prisoners become less violent and dangerous? Have institutional policies about identifying gang members and behavioral or affiliation criteria for max custody changed? When the UW solitary confinement study was conducted 20 years ago, pioneering experiments in relaxing the stringency of solitary confinement conditions and supporting prisoners in changing course had begun at Shelton (Rhodes, 2004); at that time, Washington DOC leaders justified IMU placements as a necessary response to White Supremacist groups, and IMU reforms focused on mitigating organized attacks and challenges to correctional authority by these groups. The late 2010s brought another round of reforms attempting to relax the stringent conditions of solitary confinement; this time factional rivalries among gang-affiliated Hispanic prisoners first justified IMU placements and then became the focus of reform efforts (Warner, Pacholke, & Kujath, 2014). This relationship between shifts in prison population demographics, behavior patterns, and correctional attention to specific sub-categories of gangs perceived as particularly dangerous deserves further analysis, but identifying the relevant trends, as we do here, is a first step. Third, we see changing patterns in solitary confinement use over time. Overall, the prevalence and duration of solitary confinement grew across Washington’s prison population between 2002 and 2017. The raw numbers and rates of both Max custody status prisoners and prisoners in IMU locations more than doubled from 2002 to 2017. And an increasing proportion of people throughout the system experienced solitary confinement: in 2017, more than 1 in 3 prisoners had spent at least a day in solitary compared to 1 in 4 in 2002. This trend echoes and quantifies Sakoda & Simes’ argument that solitary confinement is a “normal event during imprisonment” (2019: 2). Although rates of solitary confinement use increased overall, average LOS in solitary confinement (which peaked in 2011 in tandem with the peak years of solitary confinement use in Washington) decreased. By 2017, average LOS on IMU-Max and IMU-Ad/ DSeg (along with the standard deviations) were the shortest they had been in the state since 2002. This analysis reveals that Washington DOC had some success in ® 1317 The research presented here utilized a confidential data file from the Washington Department of Corrections. This study would not have been possible without the support of the research and correctional staff in the Washington DOC, especially Eldon Vail, Bernard Warner, Dan Pacholke, Dick Morgan, Jody Becker-Green, Steve Sinclair, Paige Harrison, Vasiliki GeorgoulasSherry, Bruce Gage, Ryan Quirk, and Tim Thrasher. Formerly of the University of Washington, Lorna Rhodes served as a project mentor, and L. Clark Johnson provided critical advice at early Acknowledgments reducing its use of solitary confinement from peak levels, and especially in shortening LOS in these conditions. But what forces facilitated or constrained these reductions? The dramatic shifts we document in both numbers of people in solitary confinement and durations of stays – without any associated dramatic shifts in the usually assumed behavioral predictors of solitary confinement, like overall institutional rates of gang membership or violent infractions – suggest the influence of other institutional factors (cf. Lynch, 2019). While additional analysis is needed, we can, thanks to our iterative conversations with DOC officials, suggest two institutional factors that influenced rates and durations of solitary confinement use during periods of abrupt change: bed capacity increases and local-level rehabilitative programming changes. First, between 2000 and 2008, while DOC’s expanding capacity was continually outpaced by population growth (despite legislative changes intended to reduce imprisonment, Washington State Institute for Public Policy [WSIPP], 2006), IMU capacity in Washington expanded by 520 beds. Three years later, in 2011, both IMU-Max counts and average LOS peaked. Both then decreased in tandem with decreasing IMU capacity: down 212 beds as of 2017, as some units were re-purposed for other special groups, such as parole violators, and managed with far less restrictive protocols. While the relationship between capacity, IMU counts, and LOS deserves its own focused analysis, we have taken the first step by identifying relevant trends. These findings suggest that constraining capacity is likely a key to long-term reductions in solitary confinement, along with reducing LOS and rate of assignments into maximum security settings like IMUs. Second, between 2011 and 2014, Washington DOC built upon previous, local initiatives at Clallam Bay and Walla Walla IMUs, embarking on an effort to “reinvent what segregation can be”: partnering with Vera Institute of Justice, eliminating some aversive disciplinary policies, and introducing facility-specific missions and group rehabilitative programming across IMUs (Neyfakh, 2015). Both the temporary drop in IMU-Max populations in 2014, and the more sustained decreases in average LOS for this population between 2011 and 2017 are tied to these interventions. The correctional population analysis presented in this study exemplifies an approach to research and collaboration suited to improving the ability of corrections systems to track changes in prisoner characteristics, LOS, and overall rates of placement in various forms of solitary confinement. Rendering such patterns visible strengthens researcher–practitioner collaboration, revealing in Washington’s case what is working, i.e. sustained reductions in lengths of solitary confinement stays; and what is not working, i.e. less sustained reductions in rates of solitary confinement use. By displaying institutional patterns, our collaborative research findings also suggest avenues of analysis to improve outcomes for prisoners and in prison settings. JUSTICE QUARTERLY ® 1318 D. LOVELL ET AL. Natalie Pifer, JD, PhD, is an Assistant Professor of Criminology and Criminal Justice at the University of Rhode Island, 510 Chafee Hall, Kingston, RI 02881. Email: npifer@uri.edu. She Kelsie Chesnut, MA, ABD, is a Research Associate at the Vera Institute of Justice, Center on Sentencing and Corrections, 634 S Spring Street, #300A, Los Angeles CA 90014. Email: kchesnut@vera.org. She studies criminal justice reform, the impact and translation of policy into practice, and is especially interested in correctional staff’s role in policy reform. Her work has appeared in American Journal of Public Health, Injury Prevention, and The Annual Review of Law and Social Science. Keramet Reiter, JD, PhD is an Associate Professor, at the University of California, Irvine, Department of Criminology, Law & Society, 3373 Social Ecology II, Irvine, CA 92697. E-mail: reiterk@uci.edu. She is the project PI, studies prisons, prisoners’ rights, and the impact of prison and punishment policy on individuals, communities, and legal systems, especially the history and uses of long-term solitary confinement in the United States and internationally. Her work has appeared in the American Journal of Public Health, Law & Society Review, and Punishment & Society, and she is the author of two books: 23/7: Pelican Bay Prison and the Rise of Long-Term Solitary Confinement (Yale University Press, 2016), and Mass Incarceration (Oxford University Press, 2017). Rebecca Tublitz, MPP is a doctoral student at the University of California, Irvine, Department of Criminology, 2340 Social Ecology II, Irvine, CA 92697. Email: rtublitz@uci.edu. She studies the impact of criminal justice and corrections reforms and is particularly interested in how actors across the criminal justice system respond to policy interventions. David Lovell, PhD (philosophy, University of Wisconsin); MSW (social work, University of Washington), Research Associate Professor Emeritus, University of Washington, Child, Family, and Community Health Nursing. In 1982–1983, he was philosopher-in-residence with the Connecticut Department of Correction. His writing on prisons, mental illness, solitary confinement, and ethics centers on processes and outcomes in prison and the community, and has appeared in Psychiatric Services, Crime and Delinquency, Law and Human Behavior, and Criminal Justice and Behavior as well as Correctional Mental Health Report and Correctional Law Reporter. He is the author of the entry on solitary confinement in J. Bumgarner, C. Lewandowski (Eds.), Criminal Justice in America: The Encyclopedia of Crime, Law Enforcement, Courts, and Corrections. Notes on contributors This work was supported by the Langeloth Foundation and approved by the Institutional Review Board at the University of California, Irvine (HS 2016-2816). Funding None of the authors have conflicts of interest to declare. Disclosure statement stages of data compilation. At the University of California, Irvine, Keely Blissmer helped to compile the literature review; Dallas Augustine, Melissa Barragan, Pasha Dashtgard, Gabriela Gonzalez, and Justin Strong all participated in data collection and analysis at various stages of this project. Note: The views expressed here are those of the authors and do not necessarily represent those of the Washington DOC or other data file contributors. Any errors are attributable to the authors. ® 1319 Allen, D. G., Lovell, D. G., & Rhodes, L. A. (2001). Correctional mental health: A research agenda. In J.J. Fitzpatrick, P.A. White (Eds), Psychiatric mental health nursing research digest (pp. 180–184). New York, NY: Springer. Association of State Correctional Administrators and the Arthur Liman Public Interest Program, Yale Law School (ASCA-Liman) (2015). Time-In-Cell: The ASCA-Liman 2014 National Survey of Administrative Segregation in Prison. Retrieved from https://law.yale.edu/sites/default/files/area/ center/liman/document/asca-liman_administrativesegregationreport.pdf Association of State Correctional Administrators and the Arthur Liman Public Interest Program, Yale Law School (ASCA-Liman) (2018). Reforming restrictive housing: The 2018 ASCA-Liman nationwide survey of time-in-cell. Report issued by the Association of State Correctional Administrators (ASCA) & the Liman Center for Public Interest Law at Yale Law School. Retrieved from https://law.yale.edu/sites/default/files/area/center/liman/document/asca_liman_ 2018_restrictive_housing_released_oct_2018.pdf Beck, A. J. (2015). Use of restrictive housing in U.S. prisons and jails, 201112. Washington, DC: Bureau of Justice Statistics, Government Printing Office. Retrieved from https://www.bjs.gov/ content/pub/pdf/urhuspj1112.pdf. Berger, D. (2014). Captive nation: Black prison organizing in the civil rights era. Chapel Hill: University of North Carolina Press. Bloom, J., & Martin, W. E. (2013). Black against empire: The history and politics of the Black Panther party. Berkeley: University of California Press. Briggs, C. S., Sundt, J. L., & Castellano, T. C. (2003). The effect of supermaximum security prisons on aggregate levels of institutional violence. Criminology, 41(4), 1341–1376. doi:10.1111/j. 1745-9125.2003.tb01022.x Cochran, J. C., Toman, E. L., Mears, D. P., & Bales, W. D. (2018). Solitary confinement as punishment: examining in-prison sanctioning disparities. Justice Quarterly, 35(3), 381–411. doi:10. 1080/07418825.2017.1308541 Cohen, F. (2008). Penal isolation: beyond the seriously mentally ill. Criminal Justice and Behavior, 35(8), 1017–1047. doi:10.1177/0093854808317569 Foucault, M. (1977). Discipline and punish: The Birth of the prison. New York: Pantheon Books. Haney, C. (2018). The psychological effects of solitary confinement: A systematic critique. Crime and Justice, 47(1), 365–416. no. doi:10.1086/696041 Haney, C., & Lynch, M. (1997). Regulating prisons of the future: A psychological analysis of supermax and solitary confinement. NYU Review of Law & Social Change, 23, 477–570. Hoffman, E., & McCoy, J. (2018). Concrete mama: Prison profiles from Walla Walla. Seattle, WA: University of Washington Press. Kaeble, D., & Cowhig, M. (2018). Correctional populations in the United States, 2016 (Vol. 25121). Washington, DC: US Department of Justice, Bureau of Justice Statistics. Kurki, L., & Morris, N. (2001). The purposes, practices, and problems of supermax prisons. Crime and Justice, 28, 385–424. doi:10.1086/652214 Labrecque, R. M., & Smith, P. (2019). Assessing the impact of time spent in restrictive housing confinement on subsequent measures of institutional adjustment among men in prison. Criminal Justice and Behavior , 46(10), 1445–1455. doi:10.1177/0093854818824371 Liebling, A. (1999). “Doing research in prison: Breaking the silence?”. Theoretical Criminology, 3(2), 147–173. doi:10.1177/1362480699003002002 References studies criminal justice reforms and is especially interested in how changes to policing and punishment practices are developed and implemented. Her work has appeared in the American Journal of Public Health, Law & Social Inquiry, and the Cambridge Handbook on Policing in the United States. JUSTICE QUARTERLY ® 1320 D. LOVELL ET AL. , P.-P. (2017). Logan, M. W., Dulisse, B., Peterson, S., Morgan, M. A., Olma, T. M., & Pare Correctional shorthands: Focal concerns and the decision to administer solitary confinement. Journal of Criminal Justice, 52, 90–100. doi:10.1016/j.jcrimjus.2017.08.007 Lucas, J. W., & Jones, M. A. (2019). An analysis of the deterrent effects of disciplinary segregation on institutional rule violation rates. Criminal Justice Policy Review, 30(5), 765–787. doi:10.1177/ 0887403417699930 Lovell, D. G. (2008). Patterns of disturbance in a supermax population. Criminal Justice and Behavior, 35(8), 985–1004. doi:10.1177/0093854808318584 Lovell, D. G. (2014). Isolation vignettes: Practical applications of strict scrutiny. The Correctional Law Reporter, 26(1), 3. Lovell, D. G., Cloyes, K. C., Allen, D. G., & Rhodes, L. A. (2000). Who lives in super-maximum custody? A Washington State study. Federal Probation, 64(2), 33–38. Lovell, D. G., Johnson, C., & Cain, K. C. (2007). Recidivism of supermax prisoners in Washington state. Crime & Delinquency, 53(4), 633–656. doi:10.1177/0011128706296466 Lynch, M. (2019). Focally concerned about focal concerns: A conceptual and methodological critique of sentencing disparities research. Justice Quarterly, 36(7), 1148–1175. doi:10.1080/ 07418825.2019.1686163 Mears, D. P., Hughes, V., Pesta, G. B., Bales, W. D., Brown, J. M., Cochran, J. C., & Wooldredge, J. (2019). The new solitary confinement? A conceptual framework for guiding and assessing research and policy on “Restrictive housing”. Criminal Justice and Behavior, 46(10), 1427–1444. doi:10.1177/0093854819852770 Mears, D. P., & Bales, W. D. (2009). Supermax incarceration and recidivism. Criminology, 47(4), 1131–1166. doi:10.1111/j.1745-9125.2009.00171.x Naday, A., J.D. Freilich, & J. Mellow (2008). “The Elusive Data on Supermax Confinement.” Prison Journal, 88(1): 69–93. National Institute of Justice (NIJ) (2016). Restrictive housing in the U.S.: Issues, challenges, and future directions. Washington, DC: National Institute of Justice, https://www.ncjrs.gov/pdffiles1/ nij/250315.pdf. Neyfakh, L. (2015). What do you do with the worst of the worst? Slate. Retrieved from https:// slate.com/news-and-politics/2015/04/solitary-confinement-in-washington-state-a-surprising-andeffective-reform-of-segregation-practice.html. O’Keefe, M. L., Klebe, K. J., Stucker, A., Sturm, K., & Leggett, W. (2011). One year longitudinal study of the psychological effects of administrative segregation. Washington, DC: National Criminal Justice Research Service, National Institute of Justice, www.ncjrs.gov/pdffiles1/nij/grants/ 232973.pdf. Petersilia, J. (1991). Policy relevance and the future of criminology. Criminology, 29(1), 1–15. doi: 10.1111/j.1745-9125.1991.tb01056.x Petersilia, J. (2009). When prisoners come home: Parole and prisoner re-entry. New York, NY: Oxford University Press. Pyrooz, D. C., & Mitchell, M. M. (2020). The use of restrictive housing on gang and non- gang affiliated inmates in U.S. prisons: Findings from a national survey of correctional agencies. Justice Quarterly, 37(4), 590–615. doi:10.1080/07418825.2019.1574019 Pyrooz, D. C., Labrecque, R. M., Tostlebe, J. J., & Useem, B. (2020). Views on COVID-19 from inside prison: Perspectives of high-security prisoners. Justice Evaluation Journal, 3(2), 294–306. doi: 10.1080/24751979.2020.1777578 Reiter, K. (2012). Parole, snitch, or die: California’s supermax prisons and prisoners. Punishment & Society, 14(5), 1987–2007, 530–563. Reiter, K. (2016). 23/7: Pelican Bay prison and the rise of long-term solitary confinement. New Haven: Yale University Press. Reiter, K. (2018). After solitary confinement. Studies in Law, Politics and Society, 77, 1–29. Reiter, K., Ventura, J., Lovell, D., Augustine, D., Barragan, M., Blair, T., Chesnut, K., Dashtgard, P., Gonzalez, G., Pifer, N., & Strong, J. (2020). Psychological distress in solitary confinement: Symptoms, severity, and prevalence, United States, 2017-18. American Journal of Public Health, 110(S1), S56–S56. doi:10.2105/AJPH.2019.305375 ® 1321 Rhodes, L. A. (2004). Total confinement: Madness and reason in maximum security. Berkeley, CA: University of California Press. Riveland, C. (1999). Supermax prisons: Overview and general considerations. Washington, DC: National Institute of Corrections. Retrieved from http://static.nicic.gov/Library/014937.pdf. Rubin, A. T., & Reiter, K. (2018). Continuity in the face of penal innovation: Revisiting the history of American Solitary Confinement. Law & Social Inquiry, 43(04), 1604–1632. doi:10.1111/lsi.12330 Sakoda, R. T., & Simes, J. T. (2019). Solitary confinement and the U.S. Prison Boom. Criminal Justice Policy Review. doi:10.1177/0887403419895315 Schlanger, M. (2012). Prison segregation: Symposium introduction and preliminary data on racial disparities. Michigan Journal of Race & Law, 18, 241. Smith, P. S. (2006). The effects of solitary confinement on prison inmates: A brief history and review of the literature. Crime and justice, 34, 441–528. State of Washington. (1993). SHB1765. Tasca, M., & Turanovic, J. (2018). Examining race and gender disparities in restrictive housing placements. National Institute of Justice W.E.B. Du Bois Program of Research on Race and Crime Project Summary, Doc 252062 No. https://www.ncjrs.gov/pdffiles1/nij/grants/252062.pdf. Toch, H. (1977). Living in prison: The ecology of survival. New York, NY: Free Press. Toch, H., Adams, K. W., & Grant, D. (1989). Coping: Maladaptation in prisons. Washington, DC: American Psychological Association. Warner, B., Pacholke, D., & Kujath, C. (2014). Operation place safety: First year in review. Washington, DC: Washington State Department of Corrections. Retrieved from https://www. doc.wa.gov/docs/publications/reports/200-SR002.pdf. Washington State Institute for Public Policy (WSIPP) (2006). Evidence-based public policy options to reduce future prison construction, criminal justice costs, and crime rates. Retrieved from https://www.wsipp.wa.gov/ReportFile/952/Wsipp_Evidence-Based-Public-Policy-Options-to-ReduceFuture-Prison-Construction-Criminal-Justice-Costs-and-Crime-Rates_Full-Report.pdf. JUSTICE QUARTERLY ®