Estimating Gender Disparities in Federal Criminal Cases, Journal Article by Sonja Starr, 2012
Download original document:
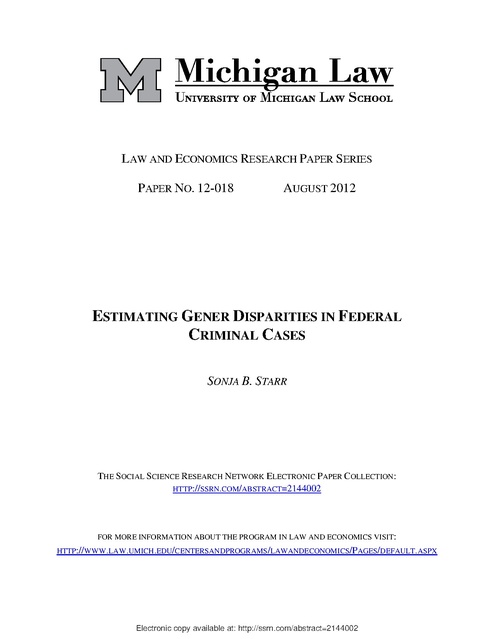
Document text
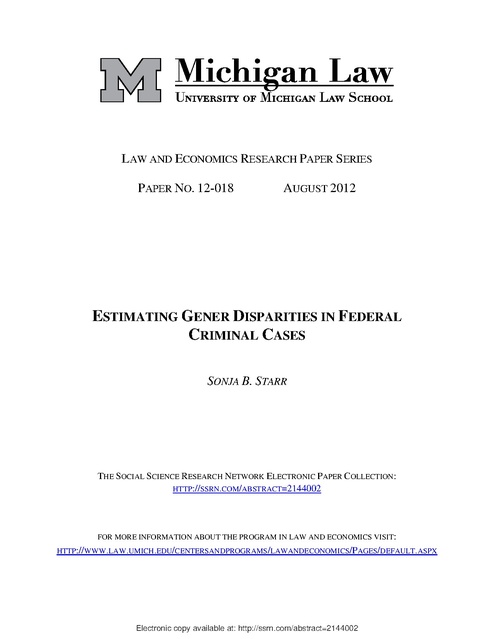
Document text
This text is machine-read, and may contain errors. Check the original document to verify accuracy.
LAW AND ECONOMICS RESEARCH PAPER SERIES PAPER NO. 12-018 AUGUST 2012 ESTIMATING GENER DISPARITIES IN FEDERAL CRIMINAL CASES SONJA B. STARR THE SOCIAL SCIENCE RESEARCH NETWORK ELECTRONIC PAPER COLLECTION: HTTP://SSRN.COM/ABSTRACT=2144002 FOR MORE INFORMATION ABOUT THE PROGRAM IN LAW AND ECONOMICS VISIT: HTTP://WWW.LAW.UMICH.EDU/CENTERSANDPROGRAMS/LAWANDECONOMICS/PAGES/DEFAULT.ASPX Electronic copy available at: http://ssrn.com/abstract=2144002 Estimating Gender Disparities in Federal Criminal Cases Sonja B. Starr* University of Michigan Law School sbstarr@umich.edu August 29, 2012 This paper assesses gender disparities in federal criminal cases. It finds large gender gaps favoring women throughout the sentence length distribution (averaging over 60%), conditional on arrest offense, criminal history, and other pre-charge observables. Female arrestees are also significantly likelier to avoid charges and convictions entirely, and twice as likely to avoid incarceration if convicted. Prior studies have reported much smaller sentence gaps because they have ignored the role of charging, plea-bargaining, and sentencing fact-finding in producing sentences. Most studies control for endogenous severity measures that result from these earlier discretionary processes and use samples that have been winnowed by them. I avoid these problems by using a linked dataset tracing cases from arrest through sentencing. Using decomposition methods, I show that most sentence disparity arises from decisions at the earlier stages, and use the rich data to investigate causal theories for these gender gaps. * Thanks to Ing-Haw Cheng, John DiNardo, Nancy Gertner, Sam Gross, Jim Hines, JJ Prescott, Eve Brensike Primus, Adam Pritchard, and Marit Rehavi for helpful comments and conversations, to Ryan Gersowitz, Michael Farrell, Seth Kingery, and Adam Teitelbaum for research assistance, and to participants in the Law and Economics Lunch, Fawley Lunch Workshop, and Criminal Justice Roundtable at the University of Michigan Law School, the University of Michigan Labor Lunch, and the Ninth Circuit Judicial Conference. Electronic copy available at: http://ssrn.com/abstract=2144002 Starr—Estimating Gender Disparities in Federal Criminal Cases Estimating Gender Disparities in Federal Criminal Cases Introduction In the United States, men are fifteen times as likely to be incarcerated as women are. But can this gap be explained by differences in criminal behavior or circumstances, or are courts or prosecutors treating genuinely equivalent cases differently on the basis of gender? The latter would violate the Constitution, undercut the criminal justice system’s punishment objectives, and contribute to the social consequences of demographically concentrated mass incarceration. So the reasons for the gender gap are of considerable legal and policy interest. This study explores them using a dataset that traces federal criminal cases from arrest through sentencing. I find that gender gaps widen at every stage of the justice process and that men and women ultimately receive dramatically different sentences. Existing studies of demographic disparities in criminal justice focus on narrow slices of the justice process in isolation. Most assess the judge’s final sentencing decision, controlling for conviction severity or “presumptive sentence” measures that are themselves produced by discretionary decisions and negotiations. Ignoring disparities in those earlier stages could bias sentencing disparity estimates, both because the key control variable is endogenous and because of sample selection from the winnowing of cases at each procedural stage. Current sentencing literature typically ignores this “funnel.” There is a small literature addressing disparities in prosecutorial decisons, but it addresses only certain pieces of the process and does not estimate their ultimate sentencing consequences. These limitations represent a surprising gulf between the quantitative empirical scholarship and the theoretical literature on the criminal justice system, which widely recognizes that sentencing is heavily shaped by prosecutors’ capacious charging and bargaining discretion. This study seeks to close this gap, using a multi-agency linked dataset that traces cases from arrest through sentencing. I estimate sentence outcomes conditioned on characteristics that are fixed near the beginning of the justice process, rather than near the end of it: the arrest offense, criminal history, and other prior characteristics. This approach generates a measure of the aggregate gender disparity introduced in the post-arrest justice process. I then use sequential decomposition methods to assess how much of this gap appears to be explainable by decision-making at each procedural stage. See Altonji, Bharadwaj, and Lange 2008; DiNardo, Fortin, and Lemieux 1996. In short, I ask: do otherwise-similar men and women who are arrested for the same crimes end up with the same punishments, and if not, at what points do their fates diverge? Although the arrest offense is not a perfect proxy for underlying criminal conduct, it is a big improvement on the highly endogenous controls used in current research. I also use estimation strategies—reweighting of the mean and the distribution—that offer a useful solution to a problem with which sentencing researchers have long struggled: how to treat non-prison sentences. The leading approach is a Two-Part Model that separates the incarceration decision from the length decision, but that introduces serious sample selection concerns if there is disparity in the first stage. The best solution is simply to treat sentencing as a single process and estimate disparities in all sentences, including the zeros. Doing so with reweighting rather than regression obviates the functional form concerns that underlie many researchers’ preference for the Two-Part Model. 1 Electronic copy available at: http://ssrn.com/abstract=2144002 Starr—Estimating Gender Disparities in Federal Criminal Cases The estimated gender disparities are strikingly large, conditional on observables. Most notably, treatment as male is associated with a 63% average increase in sentence length, with substantial unexplained gaps throughout the sentence distribution. These gaps are much larger than those estimated by previous research. This is because, as the sequential decomposition demonstrates, the gender gap in sentences is mostly driven by decisions earlier in the justice process—most importantly sentencing fact-finding, a prosecutor-driven process that other literature has ignored. But why do these disparities exist? Despite the rich set of covariates, unobservable gender differences are still possible, so I cannot definitively answer the causal question. However, several plausible theories have testable implications, and I take advantage of the unusually rich dataset to explore them. I find substantial support for some theories (particularly accommodation of childcare responsibilities and perceived role differences in group crimes), but that these appear only to partially explain the observed disparities. 1. Discretion and Gender Disparity in Criminal Justice 1.1. Sources of Discretion in the Federal Criminal Justice Process Just as the states do, the federal justice system gives enormous power to prosecutors. The United States in effect has a system of negotiated justice, and prosecutors hold most of the chips. They have broad discretion to choose charges from numerous overlapping criminal statutes, and then to determine the terms of plea deals. Plea-bargaining does not necessarily focus mainly on dropping of charges—indeed, the lead charge was dropped only 17% of the time in this study’s sample. The parties also often negotiate stipulations to key “sentencing facts”—for instance, the quantity of drugs trafficked or the defendant’s major or minor role in a conspiracy. The prosecutor also may make non-binding sentencing recommendations or request special leniency to reward cooperators. Federal sentencing is guided by two main legal frameworks. First, each criminal statute specifies a sentencing range. Most are broad and start at zero (for instance, 0-20 years), but some specify a “mandatory minimum.” Second, since 1987, the statutory sentencing constraints have been supplemented by much narrower ranges (for instance, 27 to 33 months) found in the U.S. Sentencing Guidelines. The Guidelines sought to reduce unwarranted disparities in sentencing, including gender disparities (see Breyer 1988), by constraining judicial discretion. They were mandatory until 2005, when the Supreme Court’s decision in United States v. Booker (543 U.S. 220) rendered them advisory. But advisory does not mean unimportant—judges are still required to calculate the Guidelines sentence, and most sentences are still within the Guidelines range (U.S. Sentencing Commission 2010). The Guidelines sentencing ranges are found in the cells of a grid, the two axes of which are the “offense level” and the defendant’s criminal history. Judges determine the offense level based on the crime(s) of conviction and the “sentencing facts.” Although judges have independent factfinding authority, in practice they usually defer to the plea agreement’s stipulations (Stith 2008; Schulhofer and Nagel 1997; Powell and Cimino 1995). One survey found that 92% of judges said their findings of fact diverge from the plea agreement either “infrequently” or “never” (Gilbert and Johnson 1996). Legal scholars widely agree that the Guidelines greatly empowered prosecutors because the sentence was now far more constrained by the charges of conviction and especially by the negotiated “sentencing facts” (Stith 2008; Bibas 2009). Prosecutors thus 2 Electronic copy available at: http://ssrn.com/abstract=2144002 Starr—Estimating Gender Disparities in Federal Criminal Cases could both threaten long sentences and virtually promise much lower ones in exchange for guilty pleas, and plea rates rose from 87% to 97%, where they remain today (Alschuler 2005; Miller 2004). Although Booker expanded judicial discretion, the continued high rate of Guidelines compliance means these sources of prosecutorial influence have not disappeared. In addition, prosecutors can still firmly bind judges using mandatory minimums. Prosecutors have a variety of incentives to balance, including career incentives that push toward maximizing sentences and resource constraints that discourage going to trial (see, for example, Baker and Mezzetti 2001; Easterbrook 1983). In addition, prosecutors may be affected by sympathy or a sense of fairness. Schulhofer and Nagel (1997) review federal prosecutors’ case files and find evidence of deliberate charge manipulation to avoid excessive sentences. Prosecutorial discretion is often described as the power not to seek to maximize punishment—to be selectively lenient (see Stith 2008). Although there may be good policy reasons for allowing such discretion, it is a potential source of unwarranted disparity if it is influenced by legally irrelevant factors such as gender. 1.2. Existing Empirical Research Existing studies of demographic disparities in criminal justice have typically focused on single stages of the criminal process in isolation—usually, the judge’s final sentencing decision. In the federal-court literature, the usual approach is to estimate gaps in sentence outcomes when controlling for the Guidelines offense level and the defendant’s criminal history. These two key controls are often combined into a “presumptive sentence,” usually the lower end of the Guidelines range (U.S. Sentencing Commission 2010), or into dummies for the Guidelines grid cell (see, for example, Mustard 2001). Similarly, state-level studies generally control for some measure of conviction severity as well as criminal history (see, for example, Steffensmeier, Kramer, and Streifel 1993). Studies of gender disparity that take this approach have usually found that women receive shorter sentences, conditional on observables. The size of this effect has varied considerably, even among studies that use federal data. Sarnikar et al. (2007) find about a 30% unexplained gender gap in sentence length, as did a prominent recent U.S. Sentencing Commission (2010) study. Many studies, however, have estimated considerably smaller disparities—for instance, Stacey and Spohn (2006), Schanzenbach (2005), and Mustard (2001) all find average gender gaps in sentence length of around 10%. The problem with the dominant approach is that the key control variable is itself the result of a host of discretionary decisions made earlier in the justice process, which these studies ignore. The resulting sentencing disparity estimates are potentially biased by the endogeneity of the key control variable as well as sample selection introduced by the dismissal of cases prior to sentencing. Although there have been occasional studies of pleabargaining disparities (see, for example, Spohn and Spears 1997; Shermer and Johnson 2010), they concern only certain bargaining outcomes, such binary measures of whether any charges were dropped, and ignore negotiation over sentencing facts, which is the key aspect of bargaining in the modern federal system. Moreover, without assessing disparities in prosecutor’s initial choice of charges, the charge-bargaining results are not very meaningful.1 1 Spohn, Gruhl, and Welch (1987) found gender disparities favoring women in the rate of filing felony charges in Los Angeles County, but did not analyze charge severity as an outcome. 3 Starr—Estimating Gender Disparities in Federal Criminal Cases Further, the plea-bargaining studies tend to assess that stage in isolation too, rather than assessing its ultimate sentencing-disparity consequences. 1.3. The Dataset This study uses data from four different federal sources: the U.S. Marshals’ Service (USMS), the Executive Office of U.S. Attorneys (EOUSA), the Administrative Office of the U.S. Courts (AOUSC), and the U.S. Sentencing Commission (USSC); the Bureau of Justice Statistics provided inter-agency linking files that allow cases to be traced from arrest through sentencing. The main sample consists of federal property and fraud crimes, drug crimes, regulatory offenses, and violent crimes sentenced between FY 2001 and FY 2009.2 Immigration cases, which have different stakes centering on deportation, were excluded. To reduce common support concerns, offense categories that were over 95% male were dropped: weapons, sex and pornography, conservation, and family offenses. The data include rich offense and offender information, including arrest offense (which USMS identifies with 430 codes),3 gender, race, age, marital status, district, citizenship, a string field describing the offense, criminal history, number of dependents, education, Hispanic ethnicity, counsel type, co-defendant information, and county. AOUSC also lists the initial and final charges ; these statutory sections then had to be coded on a numeric charge severity scale. I constructed three such scales based on combined severity of all charges: the statutory maximum, the statutory minimum, and a Guidelines-based measure. If the statute prescribed varying sentences depending on case facts, I used default assumptions grounded in legal research. For further details, see the Data Appendix. 2. Analysis and Results 2.1. Filing and Conviction-Stage Disparity This study principally focuses on whether male and female arrestees ultimately receive the same sentences, but a threshold question is whether they are equally likely to be sentenced at all. Disparities in charging and conviction rates are important outcomes in their own right, and also are potential sources of sample selection bias in the sentencing analysis. To be included in the sentencing data, defendants must first face charges before a district court judge—a close proxy for felony charges because misdemeanors are usually handled by magistrates. Second, defendants must be convicted of a non-petty offense: a felony or a Class A misdemeanor. Accordingly, I begin by estimating the probability of these events. Columns 1 and 2 of Table 2 report the “male” odds ratios from logistic regressions.4 Conditional on arrest offense, district, race, citizenship, and age (the variables observed for all arrested defendants), male arrestees face a modestly but significantly higher probability of a charge before a district judge: 92.2% for the average male and 90.7% for the equivalent 2 For the filing and conviction analyses, the sample consists of cases charged or disposed of during that period. grouped certain closely related codes and subdivided certain drug codes based on a separate drug-type field. There were 123 arrest offenses after this recoding, and the results are robust to use of the original codes. 4 Except where other clustering is noted, all standard errors are clustered on arrest offense and district (combined), due to concern that local crime patterns or the U.S. Attorney’s Office’s priorities might introduce correlations. Results are robust to clustering on arrest offense or district alone. 3 I 4 Starr—Estimating Gender Disparities in Federal Criminal Cases female.5 Conditional on the same variables plus multi-defendant case structure, male district court defendants are also significantly more likely to be convicted of a non-petty offense (93.2% versus 91.4%; Table 2, Col. 2).6 Sample selection bias from filing and conviction are likely to downward-bias the sentencing disparity estimates reported below, but fairly slightly, because these initial disparities affect relatively few cases. I therefore do not correct the sentencing-stage estimates below for sample selection at these threshold stages. 2.2. The “Two-Part Model” of Incarceration Probability and Sentence Length When estimating sentencing disparity, a threshold question is how to treat non-prison sentences such as probation or fines (18% of the sample). This question has been hotly debated in sentencing research. The leading practice is to break sentencing into two decision processes, each estimated parametrically: whether to order incarceration and, if so, for how long (see, for example, Berk 1983). The theory is that non-prison sentences are have no obvious “prison equivalent,” and moreover, some covariates might be more influential in the incarceration decision than the length decision or vice versa. A practical advantage is that constraining the length sample to positive-length cases allows log transformation without having to assign some arbitrary small value to the zeros.7 This is ideal because sentencing law is structured so that inputs to sentencing will generally have multiplicative effects—each Guidelines grid cell is a multiplier of the ones adjacent to it. Although I prefer a different approach (discussed below), for comparability to the current literature, I begin with estimates for this “Two-Part Model” (TPM). Table 2, Column 3 shows the results of a logistic regression of an incarceration indicator on gender, arrest offense, criminal history, district, race, age, education level, U.S. citizenship, and the multidefendant case flag. The average male in the sample faces an 86% probability of incarceration; comparable females are nearly twice as likely to avoid incarceration (74%). Conditional on incarceration, men receive sentences that are approximately 34% longer. The complication is that the gender disparity in the incarceration decision almost surely means that the length estimates are downward biased by sample selection.8 Criminologists have often responded to this problem with Heckman-style corrections (see Heckman et al. 1988; see Ulmer and Bradley [2006] for sentencing examples), but this approach is not ideal because there is no plausible exclusion restriction.9 In addition, the approach assumes that the estimand is the average treatment effect (the “ATE”) on the underlying population. In this context, that is a strange object: the gender disparity in prison sentence length that would be observed in a hypothetical world in which all defendants had to go to prison. This thought exercise is of improbable interest to policymakers. 5 This sample consisted of arrestees facing some charge. Cases that were entirely declined were dropped because they often represent unknown outcomes (transfers to other authorities or districts). When declinations citing a favorable reason (such as lack of evidence) are included as zeros, the gender disparity stays significant. 6 Petty offense convictions and jury acquittals are rare, so this disparity is driven by dismissals by prosecutors. 7 The resulting estimates would be extremely sensitive to the choice of small value. Note that there are also a very small number of life sentences, which I code as 540 months based on life expectancy data. 8 The direction of bias is clear because of the incarceration decision and the prison length decision are both driven by observable and unobservable factors affecting case severity. If selection-on-observables holds in the full sample, it almost surely will not hold in the sample of nonzero prison cases, because the incarceration regression indicates that conditional on the observed covariates, men are more likely to be incarcerated—that is, it takes less severe unobservables to push a given male case into the incarceration sample. 9 As Bushway, Johnson, and Slocum (2007) point out, the sentencing literature tends to ignore this problem. 5 Starr—Estimating Gender Disparities in Federal Criminal Cases If one is to follow the Two-Part Model at all, it is better instead to ask: If we went from treating everyone like women to treating everyone like men, (1) (2) what percentage of non-prison sentences would be replaced with prison, and among cases that already would have received prison sentences, how would the average length of those sentences change? More formally, the quantities of interest are: (1) E(PM|X) – E(PF|X) (2) E(YM|X, PF=1) - E(YF|X, PF=1) where P indicates a prison sentence, Y is prison sentence length, M and F denote the male and female treatment conditions, and X is the covariate distribution for the population noted.10 Object (2), in my view, is of more policy interest than the full-population ATE, requiring no speculation about a world in which probation and fines were not possible. With the estimand framed this way, the selection bias problem is not that the estimation sample contains too few females, but that it contains “extra” males who would not have been incarcerated if they were female. If it were possible to identify who those extra males were, OLS regression in a sample excluding them would be an unbiased estimator of object (2). Unfortunately, while the number of extra males can be readily estimated based on the incarceration logit,11 they cannot be identified; PF is unobserved for males (see Lee [2009], who discusses an analogous problem). In Table 3, I apply varying assumptions as to which males were marginal to produce different trimmed-sample estimates. Table 3, Column 1 replicates the “male” coefficient on log prison sentence length from the full-sample OLS regression. Because sample selection bias is almost surely downward, this should be treated as a lower bound on the true sentence length disparity within the pool of cases that would have been subject to incarceration regardless of gender. Column 2 provides something roughly approximating an upper bound, based on a nearworst-case assumption about selection bias. The Column 2 sample has trimmed the males with the lowest (most negative) individual influence on the “male” coefficient.12 In this case, the Column 2 length-disparity estimate is about 67%—approximately double the estimate for the untrimmed sample. Columns 3 and 4 of Table 3 show results for samples trimmed based 10 This notation assumes monotonicity, such that PM=1 whenever PF=1. This assumes gender monotonically affects incarceration probability, a reasonable assumption: being male greatly increased that probability in every one out of dozens of analyzed subsamples. 12 Lee (2009) proposes a similar trimming method for estimating bounds on the effect of a randomly assigned treatment when treatment monotonically affects attrition. In that case worst-case bounds can be more readily estimated; the trim that will raise the treatment effect estimate by the most is just the lower tail of the treated outcome distribution (see Lee 2009). The trim I conduct in Table 3, Column 2 is based on the same intuition. But rather than assuming random treatment, I assume selection on observables within the full sentenced sample, and use regression to estimate the number of “extra males” and to model the outcome. This assumption could certainly be challenged, as I discuss below, but it already underlies both parts of the TPM; my method simply gives a near-worst-case adjustment for the second-part estimate assuming that the first part is correct. When there are covariates, one cannot just trim the lower tail; rather, the trim is based on the observations’ influence on the partial effect of being male. Estimating a true upper bound would require trimming the group with the most negative joint influence on the “male” coefficient. Identifying that group is computationally impossible. But ranking observations by individual influence is easy and is, in practice, probably a “bad enough” assumption about sample selection to provide useful guidance as to its possible scope. 11 6 Starr—Estimating Gender Disparities in Federal Criminal Cases on a plausibly realistic (rather than worst-case) assumption about who the marginal males are. The assumption is simply that they are those with short sentences—that is, that gender is likelier to be the deciding factor in closer cases. The Column 3 sample trims the males with the very shortest nonzero sentences (one year or less), while the Column 4 sample picks them randomly from the bottom quarter of the distribution (two years or less). The estimates for these two trimmed samples are 63% and 47%, respectively. This trimming exercise is not meant to “correct” sample selection bias, but rather to provide a general sense of its possible magnitude. Unfortunately, the potential bias here is large, rendering the TPM not ideally informative. The TPM remains appealing when the disparity in incarceration probability is small, such that selection bias is likely minor; for this reason, Rehavi and Starr (2012a) used it to assess racial disparity. In the gender context, however, more useful guidance can be found using other methods. 2.3. Inverse Propensity-Score Weighting Estimates of Gender Disparities The sample selection problem described above would not exist but for the choice to model the determination of sentences as two distinct decision processes, a choice that is not compelled by theory.13 I propose a simpler approach: keeping non-prison sentences in the sample for the length-disparity estimates, and treating them as zeros. While the Two-Part Model dominates the sentencing literature, a substantial minority of the literature rejects it. Researchers following the minority approach typically instead treat sentencing as a single process in which the non-prison cases are censored, applying a Tobit model that estimates average disparity in an underlying latent variable (see Tobin 1958; see Sarnikar et al. [2007]; Bushway and Piehl [2001]; Kurlychek and Johnson [2004]; and Albonetti [1997] for sentencing examples). This approach avoids the sample selection concern, but raises other practical problems. The Tobit is not robust to violations of its assumptions of normality and homoskedasticity (see, for example, Arabmazar and Schmidt 1982; Cameron and Trivedi 2010)—and in this sample, specification tests for the Tobit are decisively failed. Moreover, while the Tobit allows researchers to avoid assigning a specific value to the non-prison sentences, they still must choose a censoring point below which their value is assumed to fall. This choice is arguably equally arbitrary, and if the length variable is log-transformed, it will have a big effect on the Tobit estimates.14 The approach I propose is conceptually simpler than either the Tobit approach or the Two-Part Model, and avoids the practical weaknesses of both. If incarceration disparities are the outcome of policy interest, then there is nothing unknown about the value of non-prison sentences: they are correctly valued at zero. The main practical drawback of including them is that it precludes log transformation, but this functional form concern is only a problem for parametric estimation. I instead estimate the average length disparity in months by inverse propensity score weighting (“IPW”), without specifying any functional relationship between 13 Bushway and Piehl (2001) provide strong reasons that a single-decision model (in particular, the Tobit) is a better fit to the Guidelines process, in which zeros are just values in the lower end of the sentencing grid. 14 For instance, using a lower limit of half a day in the the Tobit log prison model (and the same covariates as in the TPM above) produces a gender disparity estimate of 128%, while a limit of one month produces an estimate of 72%. Either limit is theoretically defensible, as are many others. While the very lowest observed nonzero sentence is one day, only 0.3% are below one month. One might reasonably set the limit to censor these cases, to avoid giving excessive weight to large multiplicative differences between trivially short sentences. 7 Starr—Estimating Gender Disparities in Federal Criminal Cases the covariates and the outcome variable. I then extend this method to the distribution, allowing assessment of disparities in incarceration probability as well as other possible heterogeneity in gender effects on sentences of different lengths. The IPW estimates of average gender disparities in sentence length are given in Table 4. The probability of being male (E(M|Xi) for each observation (the “propensity score”) is first estimated by a logistic regression of “male” on the covariates X: gender, arrest offense, criminal history, race, age, education level, U.S. citizenship, and the multi-defendant case indicator.15 Estimates of average gender disparities are then produced via weighted regression where the weights are inverse functions of the propensity score. To refer to the estimands, I use the common language of “treatment effects,” where “treatment” refers to being male. But note that for these “effects” to be given a causal interpretation, one must assume there are no confounding variables; I return to this point below. In Column 1 of Table 4, I estimate the overall average gender disparity in sentence length conditional on the pre-charge covariates. This “average treatment effect” (ATE) represents the difference between two counterfactuals: the mean sentence if everybody were treated like males and the mean sentence if everybody were treated like females (see DiNardo 2002).16 Table 4, Columns 4 and 7 reflect separate estimates of the average effects of gender disparity on male and female sentences. The “average treatment effect on the treated” (TOT) reflects the estimated effect of being male on male sentences, and is estimated by comparing the observed male average to a reweighted female average (Col. 4).17 After this reweighting, the female endowments of covariates are similar to those of the males, so the reweighted female mean can be interpreted as a counterfactual mean if males were treated like females. The “average treatment effect on the untreated” (TUT) is conversely estimated by reweighting the males, and represents the counterfactual increase in sentence if females were treated like males (Col. 7). As Table 4 shows, even after reweighting, the average gender gaps in sentence length are strikingly large. The overall average disparity (the ATE) in Column 1 is 23 months, which translates into a 63% increase in sentence length. When measured in months, gender appears to have a bigger effect on males than females (compare Columns 4 and 7): being male increases male sentences by 25 months, and would increase female sentences by 15 months. But this difference is mostly because of a higher baseline average: in percentage terms, the TOT and TUT are not very different (64% versus 61%). A drawback of propensity score reweighting is its vulnerability to the problem of limited overlap between the male and female samples (see Busso, DiNardo, and McCrary 2008). Although the large sample size reduces this concern, women are only 19% of the sample and are thinly represented in certain offenses and high criminal history categories.18 The reweighting of the female distribution risks giving unduly high weight to women with unusual covariate values. In Table 4, Columns 2 and 5, I report the ATE and TOT for a 15 District fixed effects, which were included in the Two-Part Model, are not included in the weights. When reweighting, parsimony makes it easier to balance the most important variables, and gender composition does not vary much by district in any event. The results are robust to including the districts. 16 The weights are given by 1/(1-E(M|Xi)) for female observations and 1/ E(M|Xi) for males, before rescaling to average 1 (see Busso, DiNardo, and McCrary 2008). 17 The weights are E(M|Xi )/(1-E(M|Xi) for female observations, before rescaling to average 1. 18 See Figure 1a for the propensity score distribution. 8 Starr—Estimating Gender Disparities in Federal Criminal Cases sample that eliminates those problematic covariate combinations by trimming extreme propensity score values (see, for example, Heckman et al. 1998).19 The drawback with this method is that the sample to which the estimates apply is not very intuitively or transparently defined. In Columns 3 and 6, I report the ATE and TOT for an alternate sample that excludes the highest three criminal history categories.20 Both trimming strategies produce gender disparity estimates that are fairly similar in percentage terms to the full-sample estimates (compare Columns 1 through 3 and Columns 4 through 6). I report only the full-sample results for the TUT (the effect of gender on women), because estimating it depends on reweighting only the males, and no males have propensity scores anywhere near zero. For this reason, as I proceed below to analyze the gender disparity in more detail, I focus on the counterfactual effects if women were treated like men. The effects of gender on men and women are of equal policy interest, but analyzing the TUT is simpler because the full sample can be used without limited-overlap concerns. Table 5 accordingly shows TUT estimates for subsamples and alternate specifications. Column 1 replicates the main estimate from Table 4 for comparison purposes. Columns 2 and 3 show estimates for two large offense-type categories: drug offenses (Column 2) and property, fraud, and regulatory offenses (Column 3). In percentage terms the effects are similar. The disparity is likewise almost identical in percentage terms before and after the watershed Booker decision (Columns 4 and 5).21 It is smallest for non-parents and largest for single parents (51.6% versus 67.3%; compare Columns 6-8). It is larger for defendants in multi-defendant cases than for sole defendants (66% vs. 51.2%, Columns 910), much larger among blacks than non-blacks (74% vs. 51.1%, Columns 11-12), and slightly larger in states without federal women’s prisons (Columns 13-14). Many of these subsample comparisons are useful in assessing possible causal theories for the unexplained gender gap, and they will be further addressed in the Discussion. The remainder of Table 5 shows the robustness of the TUT estimates to alternate specifications of the gender-propensity model. Columns 15 and 16 show that the TUT is unchanged by the addition of a set of flags for case characteristics mentioned in a text field based on the arresting officers’ notes (in 2001-2007, the years the field is available). The flags are for mentions of guns, other weapons, drug seizures, official victims, minor victims, conspiracy and racketeering. Columns 17 through 20 show that the estimates are robust to adding controls for marital and parental status and defense counsel type. Disparities decline slightly when controlling for pleas and time elapsed before conviction (Col. 21). The gender disparities in drug cases decline slightly when drug quantity seized at arrest, as recorded in the EOUSA investigation files, is added to the controls. This check could only be performed for arrests before 2004 because of data limitations (compare Columns 22 and 23).22 19 The propensity-score cutoff (approximately 0.93) is optimized to minimize variance (see Crump et al. 2009). The trim drops about 4% of women and 21% of men from the sample. 20 The main sample already excludes the most male-dominated crime categories. Adding the criminal history constraint does not entirely eliminate the limited overlap problem, but mitigates it considerably (see Figure 1b). 21 This does not preclude the possibility that Booker changed disparities; this analysis does not seek to disentangle Booker’s causal effects from longer-term trends. 22 Results are also robust to the use of the original ungrouped arrest codes; the addition of district controls, Hispanic ethnicity, and county-level controls for poverty rate, unemployment, per capita income, and crime 9 Starr—Estimating Gender Disparities in Federal Criminal Cases Finally, a comparison of Column 1 and Column 24 of Table 5 illustrates the importance of the choice to condition on arrest offense rather than on the end result of sentencing fact-finding. The Column 24 reweighting substitutes the final Guidelines offense level instead of the arrest offense, and the estimated disparity is reduced by 63%. This comparison suggests that by conditioning on an endogenous variable and ignoring gender disparities introduced earlier in the justice process, the current literature may have substantially understated the size of the gender gap. In Figure 2, I extend the reweighting method to estimate the effect of gender on the distribution of sentences for females following the method proposed by DiNardo, Fortin, and Lemieux (1996). The white and black bars reflect the observed distribution of sentence lengths for male and female defendants, respectively; non-prison sentences have their own bin and need not be assigned a numeric value. The checkered bars represent the counterfactual distribution if females were treated like males. Comparison of the checkered to the black bars shows large unexplained gaps throughout the distribution. The unexplained gap in the share sentenced to non-prison sentences (about 11 percentage points) is similar to the regression estimate in Table 2. The gap is not confined to the low end—the whole reweighted male distribution is shifted to the right relative to the female distribution. 2.4. Decomposing the Gender Gaps The estimates presented above represent the aggregate disparities introduced throughout the post-arrest justice process, raising the further question of when in the justice process those disparities emerge. Table 6 shows a sequential decomposition of the observed average gender disparity into components explainable by pre-charge covariates and by each subsequent stage of the process: charging, charge-bargaining, sentencing fact-finding, and sentencing. The method is a sequence of inverse-propensity score reweightings, in which new variables are added to the propensity score estimation at each step (see, for example, Altonji, Bharadwaj, and Lange 2008; DiNardo, Fortin, and Lemieux 1996). In this part of the analysis, data limitations require separate assessment of drug and non-drug cases. For non-drug crimes, the initial and final charges were coded with the statutory minimum, maximum, and Guidelines measures described above. But in drug cases, the AOUSC charge data are too ambiguous to permit that coding; the same statutory subsections encompass a vast array of drug types, quantities, and sentences. The only usable measure of statutory severity available for drug cases is the mandatory minimum for the crime of conviction, which the Sentencing Commission records. Thus, in drug cases I cannot disentangle the effects of initial charging and subsequent charge-bargaining. The mandatory minimum variable represents the combined effect of those stages. The non-drug decomposition is shown in Panel A of Table 6. Column 1 shows the raw observed gender gap to be decomposed. In Column 2, the men have been weighted based on pre-charge covariates. Columns 3, 4 and 5 sequentially add the initial charge severity measures, the conviction measures, and the final offense level (the product of sentencing fact-finding). The drug decomposition (Panel B) has one stage fewer: the conviction mandatory minimum substitutes for the separate charging and conviction variables. The explanatory value attributed to each stage is the change in the unexplained rate; and various exclusions from the sample: cases in which the indictment was issued before the arrest, cases from the South, and arrests by each of the two enforcement agencies (the FBI and the DEA). 10 Starr—Estimating Gender Disparities in Federal Criminal Cases gender gap when one adds that stage’s measures. What remains after the final reweighting is attributed to the sentencing decision. In the last two lines of each panel, I express each component as percentages of the raw observed gender gap and of the gender gap that was unexplained by the pre-charge covariates. That is, the last line decomposes the gender disparity that appears to be introduced during the criminal justice process. This method of decomposition is path-dependent: explanatory value is preferentially attributed to the covariates that are added first. Path-dependence is often a drawback to sequential decomposition, because in many contexts, when multiple correlated covariates together explain a certain portion of an outcome gap, there is no theoretical reason to “blame” one over the others (see Fortin, Lemieux, and Firpo 2011; DiNardo, Fortin, and Lemieux 1996). But here path-dependence is desirable, because the justice process is itself path-dependent: earlier decisions constrain later ones.23 The decomposition tracks the divergence of men’s and women’s fates as the process advances, so it would not make sense to attribute to a later stage a disparity that already existed. When there is a natural ordering like this, sequential decomposition is appropriate (see Altonji, Bharadwaj, and Lange 2008). The decompositions show that significant new disparity favoring women is introduced at every stage of the justice process, but sentencing fact-finding is especially crucial. In non-drug cases, an eight-month gender gap remained unexplained after reweighting by arrest offense and the other pre-charge covariates—this is the gap attributed to the justice process as a whole. Initial charging and charge-bargaining contribute about 9% and 4% of the gap, respectively; Guidelines fact-finding explains 60%, leaving 27% for the final sentencing stage to explain. In drug cases, the mandatory minimum can explain one third of the 23-month gender gap attributed to the justice process. Guidelines fact-finding can explain 29.5%, leaving 37% attributed to the final sentencing decision. In Figures 3a through 3d, I show a similar sequential decomposition of the sentencing distributions (see DiNardo, Fortin, and Lemieux 1996). Figure 3a shows the distribution of non-drug sentences observed for males and females and, between them, the distributions produced by the same series of reweightings described above. Each step in the sequence makes the male distribution look somewhat more like the female. Figure 3b presents these results in a way that (while it does not show the underlying distributions) allows the procedural sources of the gaps in the distribution to be more readily discerned. The full height of each bar represents the gap in the cumulative distribution at the denoted sentence threshold after reweighting by the pre-charge covariates—that is, the gap in the probability of getting a sentence exceeding the threshold. The patterned sections decompose these gaps into charging, charge-bargaining, fact-finding, and sentencing components. Figures 3c and 3d repeat these exercises for drug cases. The decompositions again show the central role of sentencing fact-finding, especially in explaining gaps higher in the length distribution. Judges’ final sentencing decisions appear to be more important in explaining disparities at the lower end, particularly in the incarceration decision (Figs. 3b, 3d). Because fact-finding and Guidelines departures are both stages in which men’s and women’s outcomes appear to diverge substantially, it is worth inquiring whether any particular findings of fact and departures appear to be key factors. Table 7 shows the 23 For instance, the initial charges define the range of possible outcomes to charge-bargaining; charges are almost never added (and in most cases are not dropped). 11 Starr—Estimating Gender Disparities in Federal Criminal Cases explanatory value attributed to each of several findings and departures when they are added to the mean decompositions from Table 6. These variables were not added sequentially with one another because there is no natural ordering among them; each was added independently. If they are correlated, the sum of the shares reported likely overstates their collective importance.24 Each share is thus best interpreted as the maximum the variable can explain. The factors listed in Table 7 were assessed because they are factors that one might expect to vary by gender. Their relevance to possible causal theories for gender disparity are addressed in the Discussion below. Other than the factors analyzed here, sentencing factfinding involves a vast array of context-specific inquiries. Likewise, other stated reasons for departures vary widely, and are often vague, such as “the interests of justice.” 3. Discussion The unexplained gender disparities identified above are large—much larger than those estimated via the prevailing method of conditioning on presumptive sentence. The key interpretive question is why these gaps exist—and, in particular, whether unobserved differences between men and women might justify them. One cannot instrument for inborn traits or manipulate them, so estimation of demographic disparities always risks omitted variables bias, and one must be cautious about inferring gender discrimination. Still, some often-advanced causal theories have testable implications. In this Part, I consider the leading theories suggested in the literature and in my informal conversations with criminal lawyers. 3.1. Unobserved differences in offense severity. One obvious question is whether the crimes differ in ways not captured by the arrest offense codes. The arrest offense is not a perfect proxy for underlying criminal conduct, and if it overstates the severity of female conduct relative to that of men, that might explain some of the observed disparity. In particular, one might wonder whether the disparities introduced at sentencing fact-finding merely represent the process’s proper accounting for nuance differences in facts within offense categories, which is, after all, fact-finding’s purpose. Unobserved differences naturally cannot be ruled out, but there are good reasons to doubt that they explain much of the observed disparity. First, the observable covariates are detailed, capturing considerable nuance. They include not just the 430 arrest codes and the multi-defendant flag (a proxy for group criminality, an important severity criterion), but also additional flags based on the written offense description (see Table 4, Rows 15-16). Second, the disparities are similar across all case types (and across arresting agencies), suggesting it is not a matter of a few crimes being “worse” when men commit them. Such differences would have to be prevalent across a variety of crimes and agencies to explain the result. Third, there is some reason to believe unobserved divergences between the arrest offense and actual criminal conduct may bias disparity estimates downward. If police tend to treat men more harshly, one might expect them to record arrest offenses that overstate men’s culpability relative to women’s. The empirical evidence on gender and policing is limited. Traffic stop studies reach divergent conclusions about whether there is bias against men (compare Rowe 2009 with Persico and Todd 2006), but at least do not suggest bias against women. A study covering a wider range of crimes (Stolzenberg and D’Alessio (2004)) found 24 This is almost surely the case with the fact-finding results in drug cases, where the shares reported in Table 7 add up to slightly more than the total months of disparity attributed to fact-finding in Table 6. 12 Starr—Estimating Gender Disparities in Federal Criminal Cases that other factors equal, reported crimes with female offenders are substantially less likely to lead to arrests, results that they interpret to show police leniency toward women. Nonetheless, there are some easily imaginable differences between male and female cases that might not be observed. For instance, men might well commit violent crimes with greater force, a difference not fully captured by the arrest code (beyond the labeling of some assaults as “aggravated”). There are fewer obvious potential differences in property, regulatory, or drug offenses, but perhaps women might commit smaller-scale offenses. Scale is captured to some degree by the arrest offense codes (for instance, pickpocketing versus vehicle theft), but not entirely—for instance, wire fraud could be in any amount. Findings of fact on loss value appear capable of explaining up to 20% of the otherwise-unexplained gap in non-drug crimes (Table 7). Unfortunately, there is no way to tell how much of that factfinding difference reflects true underlying differences in the facts. With respect to drug quantity, the data are more informative. Drug quantity and type determine eligibility for mandatory minimums, which explain 29.5% of the post-arrest gender gap in drug cases (Table 6); related Guidelines adjustments can explain a further 3% (Table 7).25 For arrests before FY 2004, the drug quantity and type seized at arrest is recorded in the EOUSA investigation file. Within that pool, there are substantial gender disparities in the drug quantity found at the sentencing stage, even after controlling for drug quantity at arrest and the other standard covariates. The estimated gender gap in sentences in pre-2004 drug cases is only slightly reduced by adding arrest-stage drug quantity controls to the reweighting (Table 5, Cols. 22-23). These findings suggest that quantity findings at sentencing diverge from the underlying facts in ways that differ by gender. Another key factor affecting drug sentencing is the “safety valve” loophole built into the drug mandatory minimum statutes and the related Guidelines safety valve. The safety valves can explain up to 9% of the sentence gap in drug cases, and one might wonder whether this reflects “real” case differences. Eligibility for the safety valve is defined by statute, and cases can be coded as seemingly eligible or not based on the case’s observed characteristics: criminal history, certain offense features, lack of aggravating role, and lack of obstruction. Conditional on apparent eligibility, women are significantly more likely to get safety-valve reductions. This is only suggestive evidence of disparate treatment, however, because the observables do not perfectly track the eligibility requirements.26 3.2. The “girlfriend theory.” In group offenses, another factor affecting culpability is relative role. Women might be viewed as minor players—perhaps mere accessories of their male romantic partners. Prosecutors and judges may consider such women less dangerous, less morally culpable, or useful sources of testimony. While leniency may be appropriate in such cases (see Raeder 25 Drug quantity findings drive both the application of mandatory minimums and the more nuanced gradations under the Guidelines. The 3% figure in Table 7 reflects only the latter component: the additional gender disparity explained by quantity findings after mandatory minimums had already been accounted for. 26 The key source of discretion in safety valve application is the prosecutor’s choice whether to characterize the defendant as having been fully truthful in describing the crime (see 18 U.S.C. 3553(e)). Beyond the absence of obstruction and the presence of acceptance-of-responsibility reductions, discussed above, the data do not provide a way to assess whether the defendant was in fact truthful. 13 Starr—Estimating Gender Disparities in Federal Criminal Cases [2006]), some lawyers I spoke to suggested that such perceptions are not always justified by the facts; in cases involving couples, it may just be assumed that the female is the “follower.” The data provide no way to test whether role perceptions are well founded, but they do suggest that they can partially explain the gender gap. Other than its implications for cooperation departures, the “girlfriend theory” has two testable implications: first, the gender gap should be larger in multi-defendant cases, and second, part of it should be attributable to sentencing adjustments for role in the offense. Both predictions are supported by the data. The gender gap is significantly larger in multi-defendant cases: 66% compared to 51% (Table 5). Approximately 14% of the otherwise-unexplained disparity in non-drug cases and 20% in drug cases can potentially be explained by role adjustments (Table 7). The girlfriend theory appears to explain part, but not most, of the gender gap; it is hard for it to explain the large disparities that persist even in single-defendant cases.27 3.3. Parental responsibilities. Another possibility is that prosecutors and/or judges worry about the effect of maternal incarceration on children. The estimates are robust to controls for marital status and number of dependents, but these variables do not capture all differences in care responsibilities, including custody status. Other research shows that female defendants are far more likely than men to have primary or sole custody, and incarcerating women more often results in foster care placements (see Hagan and Dinovitzer [1999] for a review of the literature; Koban 1983). In an experiment asking judges to give hypothetical sentences based on short vignettes, Freiburger (2010) found that mentioning childcare reduced judges’ probability of recommending prison, but mentioning financial support for children did not. The childcare theory suggests that one would expect to see the largest gender disparities among single parents, and the smallest among defendants with no children. That expectation is borne out by the data: compare Table 5, Columns 6-8. The TUT estimate is still over 50% among childless defendants, however, so the childcare theory appears not to fully explain the gender gap, but it probably explains part of it.28 On the other hand, the decompositions in Table 7 indicate that, at most, between 1% and 2% of the sentencing gap can be explained by disproportionate invocation of the official “family hardship” departure in the Sentencing Guidelines. Women in the sample receive that departure at three times the rate of men: 2.4% of cases versus 0.8%. But because the departure is so rare for both genders, it cannot explain much of the overall disparity. This is presumably because it requires “extraordinary circumstances,” and judges typically hold that single parenthood does not suffice (see U.S.S.G. 5H1.6; Raeder 2006). Likewise, the main federal sentencing statute, 18 U.S.C. 3553, does not mention family hardship, and the Guidelines affirmatively instruct that family ties are “not ordinarily relevant.” Federal sentencing law is not designed to provide much accommodation for defendants’ children. In short, the family status-gender interaction appears to be more substantial than the one formal legal mechanism for accommodating family hardship can explain. Prosecutors 27 The formal departure for duress or coercion (U.S.S.G. 5K2.12), while given to women at five times the rate of men (0.4% versus 0.08%), is far too rare to be a significant explanation for the gender gap. 28 The gender gap is also slightly smaller in states with federal women’s prisons (see Table 5, Columns 13-14), which may suggest that judges do not want to move women far from their families, although this is not a dramatic difference and other characteristics of those seven states might explain it. 14 Starr—Estimating Gender Disparities in Federal Criminal Cases and/or judges seem to use their discretion to accommodate family circumstances in sub rosa ways—but not for male defendants. Among single men, conditional on observables, having children significantly increases sentences, and among married men, children make no significant difference. There are many competing arguments concerning whether family status is a proper sentencing consideration (see, for example, Markel, Collins, and Leib 2007), and I will not address them here. However, if family hardship is a legitimate consideration, one might expect it to play at least some role in men’s cases as well. Numerous studies have suggested that paternal incarceration harms children even when the father was already a noncustodial parent (see Hagan and Dinovitzer [1999] for a review). 3.4. Cooperativeness. Another often-advanced theory is that female defendants receive leniency because they are more cooperative with the government. These data provide, at best, limited support for that theory. Conditional on observables, women are modestly but significantly more likely to receive downward departures for cooperation in another case (20% versus 17%), have higher guilty plea rates (97.5 vs. 96.2%), and have their cases resolved about two weeks sooner on average (a 10% difference). But the interpretation of these differences is not clear. Plea rates, timing, and cooperation are all endogenous, turning on the deals being offered. Moreover, women could be being rewarded more for the same level of cooperation; the actual assistance they provide is unobserved. On all four charge- and conviction-severity scales, women receive modestly but significantly larger charge reductions in plea-bargaining than men do, and far more favorable findings of fact, suggesting that they may be offered better factual stipulations. If women really are inherently more cooperative (or risk-averse), one might think prosecutors could get away with offering them lesser discounts, and still induce frequent guilty pleas. Yet the opposite appears to be true. Whatever the merits of these indicators of cooperativeness, they seem to explain only fairly modest portions of the gender gap. Adding a plea and elapsed-time indicator to the reweighting reduces the unexplained disparity by about 8% (Table 5, Col. 21). Disparities in departures for cooperation can explain up to 9% of the otherwise-unexplained gap in drug cases, but no significant share in non-drug cases (Table 7). In addition, the “acceptance of responsibility” reduction and the obstruction of justice enhancement do not explain any substantial portion of the gender gap; in non-drug cases these offset one another, while in drug cases neither is significant (Table 7). Unlike that of the family hardship departure, the limited explanatory power of these adjustments and departures cannot be attributed to rarity or tight legal constraints—all are very common. Formal mechanisms for recognizing women’s purportedly greater cooperativeness are readily available, and yet they explain only a modest share of the disparity in drug cases and none in non-drug cases. 3.5. Mental health, addiction, abuse, and other sympathetic life circumstances. Another theory is that female defendants may have more troubled life circumstances, such as poverty, mental illness, addiction, and abuse histories. If so, they may be perceived as less morally culpable or as candidates for rehabilitation. Criminal defendants often come from difficult backgrounds. This could well be disproportionately true for females; perhaps because women more rarely commit crime, those who do are likelier to be in the upper tail of the life-hardship distribution. Prisoner studies show more self-reported mental illness and prior abuse among women. See James and Glaze (2006); Harlow (1999). 15 Starr—Estimating Gender Disparities in Federal Criminal Cases Socioeconomic status is not unobserved, however, and does not seem to explain the gender gap. The main specification includes education, and the results are robust to adding county-level socioeconomic controls and defense counsel type (a strong proxy for poverty). But mental health, addiction, and abuse are not observable unless judges cite them as the basis for a departure. The Guidelines permit departures for “unusual” mental and emotional conditions (U.S.S.G. 5H1.3) and for “significantly reduced mental capacity” (U.S.S.G. 5K2.13). They prohibit departuers for “disadvantaged upbringing” (U.S.S.G. 5H1.12) and in most cases for addiction (U.S.S.G. 5H1.4), although judges have more flexibility to disregard these restrictions after Booker. Together, all such cited bases for departures explain only between 1 and 2% of the otherwise-unexplained gap in sentence length; they are too rare too explain more. If prosecutors or judges take such factors into account in informal ways (as they seem to with family hardship, above), it would be unobservable. 3.6. Race-Gender Interactions. Columns 11-12 of Table 5 show that the gender gap is substantially larger among black than non-black defendants (74% versus 51%). The race-gender interaction adds to our understanding of racial disparity: racial disparities among men significantly favor whites,29 but among women, the race gap in this sample is insignificant (and reversed in sign). The interaction also offers another theory for the gender gap: it might partly reflect a “black male effect”—a special harshness toward black men, who are by far the most incarcerated group in the U.S. This possibility is not really an “explanation” for the gender gap, much less a reason to worry less about it—but it might cause policymakers to understand it differently, as an issue of intersectional race-gender disparity. This theory only goes so far, however—the gender gap even among non-blacks is over 50%, far larger than the race gap among men. 3.7. Gender discrimination: preference-based and statistical. Although several of the factors above appear to explain portions of the gender gap, that gap is large enough that it is plausible that gender discrimination also contributes. If so, several types of discrimination could be at play. The theoretical literature suggests “chivalry” and “paternalism” (see, for example, Franklin and Fearn [2008]). Another theory is selective sympathy: perhaps circumstances like family hardship or “bad influence” appear more sympathetic when it is women who are in them. Psychology experiments have found that attributions of blame and credit are often filtered through expectations that males are “agentic” and active and women are “communal” and passive (see Eagly, Wood, and Diekman [2000] for a review). If so, prosecutors or judges might more readily credit societal or situational explanations for females’ crimes than for males.’ Statistical discrimination is also possible. Perhaps the likeliest such mechanism is that prosecutors or judges might assume men are more dangerous than women. Studies generally find that women have lower recidivism rates, though some of the difference may be explained by characteristics that this study controls for (see Gendreau, Little, and Goggin [1996] for a meta-analysis). I do not have recidivism data to test whether statistical discrimination might be “rational” here. Note that if recidivism risk perceptions are based on individual information about the offender (not based on gender), then it is perfectly permissible to consider them. But punishment decisions based on statistical generalizations 29 Rehavi and Starr (2012) explore these more extensively, finding a 10% unexplained disparity. 16 Starr—Estimating Gender Disparities in Federal Criminal Cases about men and women are unconstitutional. The Supreme Court has repeatedly ruled that reliance on gender stereotypes is impermissible even if those stereotypes are statistically well founded (see J.E.B. v. Alabama ex rel T.B., 511 U.S. 127 [1994]). Conclusion This study finds dramatic unexplained gender gaps in federal criminal cases. Conditional on arrest offense, criminal history, and other pre-charge observables, men receive 63% longer sentences on average than women do. Women are also significantly likelier to avoid charges and convictions, and twice as likely to avoid incarceration if convicted. There are large unexplained gaps across the sentence distribution, and across a wide variety of specifications, subsamples, and estimation strategies. The data cannot disentangle all possible causes of these gaps, but they do suggest that certain factors (such as childcare and offense roles) are partial but not complete explanations, even combined. These estimates are much larger than those of prior studies, which have probably substantially understated the sentence gap by filtering out the contribution of pre-sentencing discretionary decisions. In particular, this study highlights the key role of sentencing factfinding, a prosecutor-dominated stage that existing disparity research ignores. Mandatory minimums—prosecutors’ most powerful tools—are also important contributors to gender gaps in drug sentencing. Understanding the relative roles of prosecutors and judges is important. Gender disparities have been cited to support constraints on judicial discretion, including when the Sentencing Guidelines were adopted. But such constraints typically empower prosecutors, so if prosecutors drive disparities, they could backfire. Policymakers might simply be untroubled by leniency toward women. They are a small minority of defendants, and when disparities favor traditionally disempowered groups, they might raise fewer concerns. But the gender disparity issue need not be framed in terms of how women are treated. One could ask: why are men treated so harshly, if women are (apparently) treated otherwise? It is hard to dismiss this question as trivial: over two million American men are behind bars. While males generally are not a disadvantaged group, men in the criminal justice system generally are; they are mostly poor and disproportionately nonwhite. The especially high rate of incarceration of men of color is a serious social concern, and gender disparity is one of its key dimensions. From this perspective, one might think differently about some of the possible explanations for the gender gap. Most defendants of both genders have suffered serious hardship, have mental health or addiction issues, have minor children, and/or have “followed” others onto a criminal path. Sentencing law provides very limited formal mechanisms to account for such factors—which is probably why, with women, they appear to mostly be considered sub rosa. If prosecutors, judges, and legislators are comfortable with those factors playing a role in the sentencing of women, then perhaps it is worth explicitly reconsidering their place in criminal sentencing more generally. 17 Starr—Estimating Gender Disparities in Federal Criminal Cases Reference List Albonetti, Celesta A. 1997. “Sentencing Under the Federal Sentencing Guidelines.” Law and Society Review 31:601–634. Altonji, Joseph G., Prashant Bharadwaj, and Fabian Lange. 2008. “Changes in the Characteristics of American Youth: Implications for Adult Outcomes.” Working Paper no. 13883. National Bureau of Economic Research, Cambridge, Mass. Alschuler, Albert W. 2005. “Disparity: The Normative and Empirical Failure of the Federal Guidelines.” Stanford Law Review 58:85-118. Arabmazar, Abbas, and Peter Schmidt. 1982. “An Investigation of the Robustness of the Tobit Estimator to Non-Normality.” Econometrica 50:1055-63. Ashcroft, John. 2003. “Department Policy Concerning Charging Offenses, Disposition of Charges, and Sentencings.” Memorandum, September 22. Baker, Scott, and Claudio Mezzetti. 2001. “Prosecutorial Resources, Plea Bargaining, and the Decision to Go to Trial.” Journal of Law, Economics, and Organization 17:149-67. Berk, Richard A. 1983. “An Introduction to Sample Selection Bias in Sociological Data.” American Sociological Review 48:386–98. Bibas, Stephanos. 2009. “Prosecutorial Regulation Versus Prosecutorial Accountability.” University of Pennsylvania Law Review 157:959-1016. Breyer, Stephen. 1988. “The Federal Sentencing Guidelines and the Key Compromises Upon Which They Rest.” Hofstra Law Review 17:1-50. Bushway, Shawn, and Anne Morrison Piehl. 2001. “Judging Judicial Discretion: Legal Factors and Racial Discrimination in Sentencing.” Law and Society Review 35:733–67. Bushway, Shawn, Emily Owens, and Anne Morrison Piehl. 2012. “Sentencing Guidelines and Judicial Discretion: Quasi-experimental Evidence from Human Calculation Errors.” Journal of Empirical Legal Studies 9:291-319. Bushway, Shawn, Brian D. Johnson, and Lee Ann Slocum. 2007. “Is the Magic Still There? The Use of the Heckman Two-Step Correction for Selection Bias in Criminology.” Journal of Quantitative Criminology 23:151-78. Busso, Matias, John DiNardo, and Justin McCrary. 2009. “Finite Sample Properties of Semiparametric Estimators of Average Treatment Effects.” Working paper. University of Michigan, Ann Arbor, Mich. Cameron, Colin, and Pravin K. Trivedi. 2010. Microeconometrics Using Stata, Revised Edition. College Station: Tex.: Stata Press. Crump, Richard K., V. Joseph Hotz, Guido W. Imbens, and Oscar A. Mitnik. 2009. “Dealing With Limited Overlap in Estimation of Average Treatment Effects.” Biometrika 96:187-99. DiNardo, John. 2002. “Propensity Score Reweighting and Changes in Wage Distributions,” Working paper. University of Michigan, Ann Arbor, Mich. 18 Starr—Estimating Gender Disparities in Federal Criminal Cases DiNardo, John, Nicole M. Fortin, and Thomas Lemieux. 1996. “Labour Market Institutions and the Distribution of Wages, 1973-1992: A Semiparametric Approach.” Econometrica 64:1001-46. Eagly, Alice H., Wendy Wood, and Alice B. Diekman. 2000. “Social Role Theory of Sex Differences and Similarities: A Current Appraisal.” 123-174 in The Developmental Social Psychology of Gender, edited by Thomas Eckes and Hanns Trauter. Sussex: Psychology Press. Easterbrook, Frank H. 1983. “Criminal Procedure as a Market System.” Journal of Legal Studies 12:289-332. Fortin, Nicole, Thomas Lemieux, and Sergio Firpo. 2011. “Decomposition Methods in Economics.” In Handbook of Labor Economics, vol. 4, 1-102, edited by Orley Ashenfelter and David Card. Amsterdam: Elsevier. Franklin, Cortney A., and Noelle E. Fearn. 2008. “Gender, Race, and Formal Court DecisionMaking Outcomes: Chivalry/Paternalism, Conflict Theory, or Gender Conflict?” Journal of Criminal Justice 36:279-90. Freiburger, Tina L. 2010. “The Effects of Gender, Family Status, and Race on Sentencing Decisions.” Behavioral Sciences and the Law 28:378-95. Gendreau, Paul, Tracy Little, and Claire Goggin. 1996. “A Meta-Analysis of the Predictors of Adult Offender Recidivism: What Works!” Criminology 34:575-608. Gilbert, Scott A., and Molly T. Johnson. 1996. “The Federal Judicial Center’s 1996 Survey of Judicial Experience.” Federal Sentencing Reporter 9:87-93. Hagan, John, and Ronit Dinovitzer. 1999. “Collateral Consequences of Imprisonment for Children, Communities, and Prisoners.” Crime and Justice: A Review of Research 26:121-62. Harlow, Caroline Wolf. 1999. “Prior Abuse Reported by Inmates and Probationers.” Bureau of Justice Statistics Report, NCJ 172879. Heckman, James, Hidehiko Ichimura, Jeffrey Smith, and Petra Todd. 1998. “Characterizing Selection Bias Using Experimental Data.” Econometrica 66:1017-98. James, Doris J., and Lauren Glaze. 2006. “Mental Health Problems of Prison and Jail Inmates.” Bureau of Justice Statistics Report, NCJ 213600. Koban, L. 1983. “Parents in Prison: A Comparative Analysis of the Effects of Incarceration on the Families of Men and Women.” Research in Law, Deviance, and Social Control 5:171-83. Kurlychek, Megan C., and Brian D. Johnson. 2004. “The Juvenile Penalty: A Comparison of Juvenile and Young Adult Sentencing Outcomes in Criminal Court.” Criminology 42:485-515. Lee, David S. 2009. “Training, Wages, and Sample Selection: Estimating Sharp Bounds on Treatment Effects.” Review of Economic Studies 76:1071-1102. 19 Starr—Estimating Gender Disparities in Federal Criminal Cases Markel, Dan, Jennifer M. Collins, and Ethan J. Leib. 2007. “Privilege or Punish: Criminal Justice and the Challenge of Family Ties.” University of Illinois Law Review 2007:1148-1228. Miller, Marc L. 2004. “Domination and Dissatisfaction: Prosecutors as Sentencers.” Stanford Law Review 56:1211-69. Mustard, David B. 2001. “Racial, Ethnic, and Gender Disparities in Sentencing: Evidence from the U.S. Federal Courts.” Journal of Law and Economics 44:285-314. Persico, Nicola, and Petra E. Todd. 2006. “Generalizing the Hit Rates Test For Racial Bias in Police Enforcement, With an Application to Vehicle Searches in Wichita.” The Economic Journal 116:F351-F367. Powell, William J., and Michael T. Cimino. 1995. “Prosecutorial Discretion Under the Federal Sentencing Guidelines: Is the Fox Guarding the Hen House?” West Virginia Law Review 97:373-95. Raeder, Myrna S. 2006. “Gender-Related Issues in a Post-Booker Federal Guidelines World.” McGeorge Law Review 37:691-756. Rehavi, M. Marit, and Sonja Starr. 2012. “Racial Disparity in Federal Criminal Charging and its Sentencing Consequences.” Working Paper no. 12-002. University of Michigan Law and Economics, Empirical Legal Studies Center, Ann Arbor, Mich. Rowe, Brian. 2009. “Gender Bias in the Enforcement of Traffic Laws: Evidence Based on a New Empirical Test.” Unpublished manuscript. University of Michigan, Department of Philosophy, September. Sarnikar, Supriya, Todd Sorensen, and Ronald L. Oaxaca. 2007. “Do You Receive a Lighter Prison Sentence Because You Are a Woman? An Economic Analysis of Federal Criminal Sentencing Guidelines.” Working paper no. 2870. Institute for the Study of Labor (IZA), Bonn, Germany. Schanzenbach, Max M. 2005. “Racial and Gender Disparities in Prison Sentences: The Effect of District-Level Judicial Demographics.” Journal of Legal Studies 34:57-92. Schulhofer, Stephen J., and I. H. Nagel. 1997. “Plea Negotiations Under the Federal Sentencing Guidelines.” Northwestern University Law Review 91:1284-1316. Scott, Ryan W. 2012. “Inter-Judge Sentencing Disparity After Booker: A First Look.” Stanford Law Review 63:1-66. Shermer, Lauren O’Neill, and Brian Johnson. 2010. “Criminal Prosecutions: Examining Prosecutorial Discretion and Charge Reductions in U.S. Federal District Courts.” Justice Quarterly 27:394-430. Spohn, Cassia, John Gruhl, and Susan Welch. 1987. “The Impact of the Ethnicity and Gender of Defendants on the Decision to Reject or Dismiss Felony Charges.” Criminology 25:175-92. Spohn, Cassia, and Jeffrey W. Spears. 1997. “Gender and Case Processing Decisions.” Women and Criminal Justice 8:29-59. 20 Starr—Estimating Gender Disparities in Federal Criminal Cases Stacey, Ann Martin, and Cassia Spohn. 2006. “Gender and the Social Costs of Sentencing: An Analysis of Sentences Imposed on Male and Female Offenders in Three U.S. District Courts.” Berkeley Journal of Criminal Law 11:43-75. Steffensmeier, Darrell, John Kramer, and Cathy Streifel. 1993. “Gender and Imprisonment Decisions.” Criminology 31:411-46. Stolzenberg, Lisa, and Stewart J. D’Alessio. 2004. “Sex Differences in the Likelihood of Arrest.” Journal of Criminal Justice 32:443-54. Stith, Kate. 2008. “The Arc of the Pendulum: Judges, Prosecutors, and the Exercise of Discretion.” Yale Law Journal 117:1420-97. Tobin, James. 1958. “Estimation of Relationships for Limited Dependent Variables.” Econometrica 26:24-36. Ulmer, Jeffrey T., and Mindy S. Bradley. 2006. “Variation in Trial Penalties Among Serious Violent Offenses.” Criminology 44:631-70. U.S. Sentencing Commission. 2010. Demographic Differences in Federal Sentencing Practices: An Update of the Booker Report’s Multivariate Regression Analysis. 21 Starr—Estimating Gender Disparities in Federal Criminal Cases Table 1 SUMMARY STATISTICS District court defendants sentenced for non-petty crimes: Male White Black Other Race Age (Years) U.S. Citizen Non-Parent Married Parent Single Parent Multi-Defendant Case Education: HS Dropout HS Diploma GED/Vocational College Criminal History: Category 1 (low) Category 2 Category 3 Category 4 Category 5 Category 6 (high) Offense Category: Property/Fraud Regulatory Drug Violent Sentenced to Prison Prison Sentence Length (Months) Prison Sentence Length (If Incarcerated) All arrestees in filing-stage sample Filed in District Court All district-court defendants in conviction-stage sample Convicted (Non-Petty) (1) Mean (2) Female Mean (3) Male Mean (4) Observations 0.808 0.646 0.310 0.044 34.1 73.7 0.368 0.300 0.333 0.473 0 0.652 0.295 0.053 34.5 82.6 0.374 0.244 0.383 0.472 1 0.645 0.313 0.042 34.0 71.6 0.366 0.313 0.321 0.473 231,694 231,694 231,694 231,694 231,694 231,694 187,651 187,651 187,651 231,694 0.418 0.213 0.130 0.239 0.342 0.236 0.123 0.300 0.436 0.208 0.132 0.224 231,694 231,694 231,694 231,694 0.565 0.106 0.127 0.066 0.038 0.097 0.737 0.093 0.091 0.034 0.018 0.028 0.524 0.109 13.6 0.074 0.043 0.114 231,694 231,694 231,694 231,694 231,694 231,694 0.282 0.055 0.590 0.073 0.818 56.9 69.5 0.468 0.054 0.446 0.032 0.639 25.2 39.5 0.237 0.055 0.625 0.083 0.861 64.4 74.8 231,694 231,694 231,694 231,694 231,617 231,617 161,032 0.919 0.905 0.922 386,205 0.928 0.913 0.932 286,709 22 Starr—Estimating Gender Disparities in Federal Criminal Cases Male Black Other Age Table 2 REGRESSION ESTIMATES OF MEAN GENDER DISPARITIES IN CASE PROCESSING* (1) (2) (3) (4) Filing in District Non-Petty Conviction Incarceration Log Prison Length Court (Odds Ratios) (Odds Ratios) (Odds Ratios) (If Incarcerated) Coefficient SE Coefficient SE Coefficient SE Coefficient SE 1.213*** .044 1.293*** .029 2.193*** .052 0.347*** .014 1.023 .045 0.919** .025 0.909*** .023 0.063*** .012 1.544** 1.009*** .201 .002 0.928 0.989*** .043 .001 0.929 1.001 .050 .001 0.0170 0.0063*** .029 .000 1.480** .215 1.061 .035 0.674*** .027 -0.037* .016 0.680*** .020 1.115*** .031 0.158*** .017 Ed. 2: HS Grad 0.864*** .020 -0.0205* .008 Ed. 3: GED 0.902*** .026 0.0217** .007 0.944* .027 0.001 .008 Crim. His. Cat. 2 2.165*** .070 0.261*** .015 Crim. His. Cat. 3 3.525*** .124 0.364*** .015 Crim. His. Cat. 4 7.336*** .370 0.511*** .016 Crim. His. Cat. 5 11.573*** .820 0.650*** .017 U.S. citizen Multi-defendant Ed. 4: College Crim. His. Cat. 6 N 379,148 282,938 19.424*** 1.238 231,613 0.944*** .014 189,498 NOTE. – Ed. Cat. = educational category; Crim His. Cat. = criminal history category. Odds ratios/coefficients are from logistic and OLS regressions that also include arrest-offense and district fixed effects. *Standard errors clustered on arrest-district, respectively. *p.<0.05; **p<0.01; ***p<0.001. 23 Starr—Estimating Gender Disparities in Federal Criminal Cases Table 3 POSSIBLE EFFECTS OF SAMPLE SELECTION ON ESTIMATION OF DISPARITY IN NON-ZERO PRISON SENTENCES: COMPARISON OF TRIMMED-SAMPLE ESTIMATES* (1) (2) (3) (4) Coefficient SE Coefficient SE Coefficient SE Coefficient SE Male 0.347*** .014 0.669*** .020 0.629*** .018 0.497*** .014 Sample Trim Untrimmed Influence-Based Shortest Random Short N 189,498 166,586 166,586 166,586 NOTE. – This table compares the "male" coefficient from Table 2, Column 4 to estimates for the same regression in samples that have male observations dropped so that the gender ratio in the trimmed sample matches the counterfactual ratio predicted by the Table 2, Column 3 regression if males were, conditional on observables, incarcerated only at the rate of women. The samples in Columns 2-4 are trimmed based on differing assumptions about which males are on the incarceration margin. Column 2 trims the male cases with the most negative individual influence on the "male" coefficient, Column 3 trims those with the shortest nonzero sentences, and Column 4 trims randomly from the male cases that have sentences no longer than 24 months. *Standard errors are clustered on the offense-district. *p<0.05, **p<0.01, ***p<0.001 24 Starr—Estimating Gender Disparities in Federal Criminal Cases Table 4 AVERAGE GENDER DISPARITIES IN PRISON SENTENCE LENGTH (INCLUDING ZEROS): INVERSE PROPENSITYSCORE REWEIGHTING ESTIMATES* Treatment Average Treatment Effect on (Treated=Male) Treatment on Treated (Men) Women (1) (2) (3) (4) (5) (6) (7) 23.23*** 17.60*** 17.29*** 25.13*** 18.67*** 18.55*** 15.34*** Male (2.716) (1.923) (1.373) (2.908) (1.936) (1.409) (1.701) 36.58*** 29.76*** 27.85*** 39.28*** 31.57*** 30.98*** 25.20*** Constant (3.393) (2.986) (2.254) (3.505) (2.985) (2.183) (2.472) 63.5 59.1 62.1 64.0 59.1 59.9 60.9 Percent Sample N Full 231,582 PS Trim 190,535 Low CH 173,407 Full 231,582 PS Trim 190,535 Low CH 184,787 Full 231,582 NOTE. – Columns 1-3 show the average increase in sentence in months associated with changing all cases from the female to the male treatment condition, estimated by inverse propensity-score reweighting. Covariates used to estimate propensity scores are arrest offense, criminal history, education, age, race, U.S. citizenship, and multi-defendant case flag. Column 1 shows full-sample results. The Column 2 sample is trimmed to eliminate extreme propensity score values (p(m)>.93), and the Column 3 sample is limited to cases in criminal history categories 1-3. For the same samples, Columns 4-6 shows the "average treatment effect on the treated" (men) obtained by comparing the observed male average to the reweighted female average. Column 7 shows the counterfactual "average treatment effect on the untreated" (women) obtained by comparing the observed female average to the reweighted male average, for the full sample. The "constant" line is the average in the female treatment condition and the "percent" line expresses the treatment effect as a percent of this female average. *Standard errors are clustered on the strata within which propensity scores are balanced. *p<0.05, **p<0.01, ***p<0.001. 25 Starr—Estimating Gender Disparities in Federal Criminal Cases Table 5 ALTERNATE SAMPLES AND SPECIFICATIONS: INVERSE PROPENSITY-SCORE REWEIGHTING ESTIMATES, TREATMENT ON UNTREATED (WOMEN)* (1) (2) (3) (4) (5) (6) Married Sample Main Drug Prop./ Reg. Non-Parent Single Parent Parent Male 15.34*** 23.35*** 5.975*** 12.82*** 13.40*** 17.63*** (1.70) 25.20*** (2.472) 60.9 231,582 (1.115) 40.00*** (2.064) 58.4 136,730 (0.408) 11.01*** (0.893) 54.3 77,989 (1.717) 24.85*** (3.154) 51.6 68,890 (1.877) 22.60*** (2.531) 59.3 56,085 (2.608) 27.26*** (3.749) 67.3 62,419 (7) (8) (12) Post-Booker (10) Sole Defendant (11) Pre-Booker (9) MultiDefendant Black Non-Black 14.65*** (1.855) 23.81*** (2.554) 61.5 109,663 15.89*** (1.961) 26.46*** (3.067) 60.1 121,883 21.42*** (2.421) 32.43*** (2.90) 66.0 109,487 9.599*** (1.553) 18.73*** (2.99) 51.2 121,875 17.52*** (2.645) 23.68*** (3.80) 74.0 71,737 13.20*** (1.087) 25.83*** (1.947) 51.1 159,801 (13) (14) (15) (16) (17) (18) States w/ W. Pris. 14.45*** (1.626) 25.79*** (2.78) 56.0 91,470 States w/o W Pris. 15.59*** (2.149) 24.81*** (2.96) 62.8 139,932 Police Notes Rec'd 15.75*** (1.665) 26.44*** (2.71) 59.6 134,613 Police Notes Flags 15.57*** (1.606) 26.44*** (2.78) 58.9 134,613 Family Rec'd 15.07*** (1.842) 25.22*** (2.777) 59.8 187,553 Family Added 15.19*** (1.407) 25.23*** (2.339) 60.2 187,549 (19) (20) (21) (22) (23) (24) Counsel Rec'd Counsel Added Plea/Time Added Drug Qty Rec'd Drug Qty Ctrl. Presumpt. Sentence Percent 15.33*** (1.531) 26.70*** (2.224) 57.4 14.83*** (1.351) 26.70*** (2.521) 55.5 14.06*** (1.607) 25.20*** (2.523) 55.8 19.28*** (1.943) 33.20*** (2.060) 58.1 17.83*** (1.720) 33.20*** (2.372) 53.7 5.661*** (0.748) 25.20*** (4.218) 22.5 N 135,471 135,470 231,582 37,074 37,074 231,617 Constant Percent N Sample Male Constant Percent N Sample Male Constant Percent N Sample Male Constant NOTE. – The constant reflects the observed female average sentence length in months for the designated sample (including zeros) and the "male" coefficient is the average additional sentence length predicted if these cases were treated as male, based on inverse propensity score reweighting of the observed male sentences using the same covariates as in Table 4 except as noted. Standard errors are clustered on the strata within which propensity scores are balanced. *p<0.05, **p<0.01, ***p<0.001. 26 Starr—Estimating Gender Disparities in Federal Criminal Cases Table 6 SERIAL DECOMPOSITION OF AVERAGE GENDER DISPARITY BY PROCEDURAL SOURCES: IPW ESTIMATES OF TREATMENT ON UNTREATED (WOMEN) Unexplained Gap (Months) [1] No Controls (Total Gap) 26.90*** Non-Drug Cases (Observed Female Mean: 13.27 Months) [4] Add [2] Pre[3] Add Conviction Charge Charge Sev. Sev. [5] Add Controls Measures Measures Fact-finding 7.89*** 7.18*** 6.88*** 2.13*** Remainder (Attrib. to Sentencing) N/A (0.37) (0.31) (0.30) (0.29) (0.27) As % of Female Mean 202 59.5 54.1 51.8 16.1 N/A Share Explained by This Stage 19.01*** 0.71*** 0.30*** 4.75*** 2.13*** N/A (0.29) (0.08) (0.05) (0.13) (0.27) N/A 70.7 2.6 1.1 17.7 7.9 N/A N/A 9.0 3.8 60.2 27.0 This Stage As % of Total Gap This Stage as % of Disparity in Justice Process Drug Cases (Observed Female Mean: 40.04 Months) Remainder (Attrib. to Sentencing) [1] No Controls (Total Gap) 38.92*** [2] Pre-Charge Controls 23.38*** [3] Add Conviction Mand. Min. 15.57*** [4] Add Fact-finding 8.67*** (0.42) (0.38) (0.35) (0.29) As % of Female Mean 97.2 58.4 38.9 21.7 N/A Share Explained by This Stage 15.54*** 7.81*** 6.90*** 8.67*** N/A (0.30) (0.22) (0.20) (0.29) This Stage As % of Total Gap N/A 39.9 20.1 17.7 22.3 N/A N/A 33.4 29.5 37.1 Unexplained Gap (Months) This Stage as % of Disparity in Justice Process N/A NOTE. – Column 1 shows the average observed male-female sentence gap in months, while Column 2 shows the gap when males are reweighted on the inverse propensity score using the pre-charge covariates from Table 4. In the other columns, additional covariates have been added sequentially. In Panel A, Column 3 adds the mandatory minimum, statutory maximum, and guidelines sentence associated with the initial charges; Column 4 further adds the same measures for the charges of conviction; and Column 5 further adds the final Guidelines offense level. In Panel B, Column 3 adds the mandatory minimum at conviction, and Column 4 further adds the final offense level. The "Share Explained by This Stage" row is based on the reduction of the unexplained relative to the preceding step, and the rows below it express this share as a percentage of the total gap and the gap unexplained by pre-charge covariates. The last column in each panel ("Share Remaining") expresses the residual unexplained in the preceding column, which is attributed to the final sentencing decision, in percentage terms, showing that the percentages the decomposition attributes to the procedural stages sum to 100%. *Standard errors are bootstrapped (500 replications). *p<0.05, **p<0.01, ***p<0.001. 27 Starr—Estimating Gender Disparities in Federal Criminal Cases Table 7 SHARE OF MEAN GENDER GAP EXPLAINED BY SPECIFIC FINDINGS OF FACT AND DEPARTURES: IPW DECOMPOSITION OF TREATMENT ON UNTREATED (WOMEN)* Findings of Fact: Aggravating/Mitigating Role Acceptance of Responsibility Obstruction of Justice Loss Amount Non-Drug Crimes (Gap Unexpl. by Pre-Charge Controls: 7.9 Months) Months Share of Gap (%) Drug Crimes (Gap Unexpl. By Pre-Charge Controls: 23.4 Months) Months Share of Gap (%) 1.138*** (0.062) -0.261*** (0.037) 0.228*** (0.042) 1.585*** (0.065) 4.578*** (0.128) 0.039 (0.094) 0.076 (0.070) 14.4 -3.3 2.9 20.1 Drug Quantity N/A Drug Safety Valves (Guidelines/Mand Min Waiver) Departures: Family Ties Substantial Assistance/ Cooperation Mental Health/Abuse/Addiction N/A 0.123*** (0.018) 0.069 (0.037) 0.136*** (0.024) 1.6 0.9 1.7 N/A 0.740*** (0.103) 2.074*** (0.111) 0.287*** (0.032) 2.141*** (0.108) 0.235*** (0.030) 19.6 0.2 0.3 N/A 3.2 8.9 1.2 9.2 1.0 NOTE. – Incremental reductions in unexplained disparity when particular findings of fact or departures are added to the IPW reweightings in Table 6. Findings of fact are added to weights that already include all variables through the conviction stage as noted in Table 6. Departures are added to weights that also included the final Guidelines offense level. *Standard errors are bootstrapped (500 replications). Because these figures are based on adding each of these variables independently (rather than together or sequentially), their collective explanatory power may be overstated if the variables are collinear with one another. *p.<0.05, **p<0.01, ***p<0.001. 28 Starr—Estimating Gender Disparities in Federal Criminal Cases FIGURES Figure 1a. – Distribution of Gender Propensity Scores for Full Sample Figure 1b. - Distribution of Gender Propensity Scores for Low Criminal History Sample 29 Starr—Estimating Gender Disparities in Federal Criminal Cases Figure 2. - Gender Disparities in the Sentencing Distribution: Females vs. Reweighted Males 0.4 Share of Distribution 0.35 Observed Male 0.3 Reweighted Male 0.25 Observed Female 0.2 0.15 0.1 0.05 0 NP <12 12 to 36 36 to 60 60 to 84 84 to 150 >=150 Sentence (Months) 30 Starr—Estimating Gender Disparities in Federal Criminal Cases Figure 3a. - Sequential Reweighting of the Sentencing Distribution: Non-Drug Cases 0.6 Observed Male Pre-Chg Controls Share of Distribution 0.5 + Chg Controls 0.4 + Conv Ctrls + Factfinding 0.3 0.2 0.1 0 NP <12 12 to 24 24 to 36 36 to 60 60 to 120 >120 Sentence (Months) Figure 3b. - Decomposition of Gender Gaps in the Sentencing Distribution by Procedural Percentage Point Gap in Prob. of Exceeding Threshold (Females vs. Reweighted Males) Source: Non-Drug Cases 0.14 Sentencing 0.12 Factfinding 0.1 0.08 Charge-Bargaining Charging 0.06 0.04 0.02 0 Any Pris 12 24 36 60 Sentence Length Threshold (Months) 120 31 Starr—Estimating Gender Disparities in Federal Criminal Cases Figure 3c. - Sequential Reweighting of the Sentencing Distribution: Drug Cases Share of Distribution 0.3 Observed Male 0.25 Pre-Chg Controls + Mand Min 0.2 +Factfinding 0.15 Observed Female 0.1 0.05 0 NP <24 24 to 48 48 to 72 72 to 120 120 to 180 >=180 Sentence (Months) Figure 3d. – Decomposition of Gender Gaps in the Sentencing Distribution by Procedural Percentage Point Gap in Prob. of Exceeding Threshold (Females v. Reweighted Males) Source: Drug Cases 0.2 0.18 0.16 0.14 0.12 0.1 0.08 0.06 0.04 0.02 0 Sentencing Factfinding Mand Min Any Pris 24 48 72 120 180 Sentence Length Threshold (Months) 32 Starr—Estimating Gender Disparities in Federal Criminal Cases DATA APPENDIX 1. The Linked Dataset This project is based on a linked, multi-agency dataset from four federal agencies: the U.S. Marshals’ Service (USMS), the Executive Office for the U.S. Attorneys (EOUSA), the Administrative Office of the U.S. Courts (AOUSC) and the U.S. Sentencing Commission (USSC).30 These datasets are collected by the Bureau of Justice Statistics (BJS) and, pursuant to security conditions, made available to researchers via the National Archive of Criminal Justice Data along with linking files that allow records to be linked at an individual level across the agencies.31 Together, these files trace cases from arrest through sentencing. USMS collects information upon booking of arrestees in federal custody, based on arrest-stage information drawn from law enforcement. Their data include arrest offense, race, age, gender, marital status, a written offense description based on information from law enforcement, U.S. citizenship status, arrest date, the federal judicial district, and the arresting agency. EOUSA collects investigation- and case-related data for prosecutors; its fields were used to determine whether arrestees were charged before a district judge and for information on the type and quantity of drugs seized in arrests. Data on the initial and final charges in the case (and their disposition) as well as the number of co-defendants, defense counsel type, and the county of the offense came from the AOUSC, which compiles district court records. The USSC provides information recorded by judges on the sentence, the mandatory minimum applicable at sentencing, the defendant’s criminal history, education level, number of dependents, and Hispanic ethnicity, as well as rich detail on the specific findings of “sentencing facts” entered by judges as well as the reasons given for departure from the Sentencing Guidelines range. The linking algorithm is dyadic and includes both inter- and intra-agency links, because EOUSA and AOUSC each have multiple kinds of files. There are two possible linking pathways that incorporate all the relevant fields. The first runs from USMS to the EOUSA suspect investigation file to the EOUSA “cases terminated” file to the AOUSC “cases terminated” file to the USSC. The second runs from USMS to the EOUSC suspect investigation file to the EOUSA “cases filed” file to the AOUSC “cases filed” file to the AOUSC “cases terminated” file to the USSC. The sample for the sentencing analysis is limited to cases that linked all the way through one of these two pathways. Link-through rates were 81% (USMS to EOUSA investigation files),32 93% (EOUSA to AOUSC, among 30 The underlying linked dataset is the same as that used in a related paper on racial disparity by Rehavi and Starr (2012). However, the samples are constructed differently, in part because of different common-support concerns; this study uses more years of data, includes different case types, and includes all federal districts. This study also includes a number of additional covariates. 31 Descriptions of the files are at http://www.icpsr.umich.edu/icpsrweb/content/NACJD/guides/fjsp.html. 32 The lower link rate at this stage is probably because there are substantive reasons cases might not link through, in addition to failures of the linking algorithm. Cases would not link through if they were immediately either declined or transferred to some other authority (before opening a suspect investigation file). i Starr—Estimating Gender Disparities in Federal Criminal Cases cases filed in district court only), and 90% (AOUSC to USSC, among cases with convictions of non-petty offenses only), and did not significantly differ by gender.33 2. Sample Restrictions 2.1. Timing The main sentencing sample consisted of cases sentenced between October 1, 2000 through September 30, 2009. The analyses of filing and conviction rates required case initiation (arrest or opening of the EOUSA investigation file, whichever is later) or disposition, respectively, during the same period. 2.2. Case Type Immigration cases were excluded because their stakes typically center on deportation rather than sentencing and because they often are handled via a very different fast-track process.34 In order to achieve better overlap between the male and female samples, I also excluded several case categories in which the arrestees were over 95% male: sex and pornography-related offenses (except for prostitution), weapons offenses, conservation offenses (mainly illegal hunting and fishing), and family offenses (mainly failure to pay child or spousal support). The remaining case types were property and fraud offenses, regulatory offenses (excluding those mentioned above), non-sexual violent crimes, and drug offenses. All case type exclusions were based on the USMS arrest code. Defining the sample based on the arrest stage data alone avoided potentially serious sample selection issues that could have emerged had the exclusions been based on the prosecutor's discretionary decisions. The USMS codes are based on the principal arrest offense and may exclude some secondary criminal conduct (although in most cases, because concurrent sentencing is the default rule, secondary conduct will not affect the sentence). While virtually nobody in the sample was convicted of any immigration, sex/pornography, family, or conservation offenses, overlap between weapons cases and other cases is more common: about 6% of the sample was convicted of a weapons charge. The presence of weapons in violent crimes is often captured by the arrest code, and their presence in any kind of case is often captured by the police-notes-based description field that I use in robustness checks. Cases with arrest codes indicating a reason for detention other than a criminal offense (material witness warrants and violations of the conditions of parole or probation) were also excluded from the sample. 3. Construction of Key Independent Variables 3.1. Demographics Gender, race, U.S. citizenship, marital status, and age are recorded by USMS. Race is coded as white, black, Asian, Native, and Other/Unknown; the last three groups together constitute only 4% of the sample, and I combined them. USSC provides number of dependents, education level, and Hispanic ethnicity (ethnic Hispanics are overwhelmingly coded as white in the race data). Marital status, number of dependents, and Hispanic ethnicity are sometimes missing and are included only in robustness checks. Also as a 33 Rates of filing in district court and conviction of non-petty offenses are outcomes assessed in the paper; cases that drop out of the sample due to non-filing, dismissal, or acquittal do not reflect linking failures. 34 Citizenship was included as a covariate, and non-citizens were excluded in robustness checks, because deportation is also an important concern when non-citizens are charged even in non-immigration cases. ii Starr—Estimating Gender Disparities in Federal Criminal Cases robustness check, the county fields in AOUSC were linked to county level unemployment, poverty, and wage data from the Census Bureau and to crime data from the FBI’s Uniform Crime Reporting Program. 3.2. Arrest Offense There are 430 unique arrest offenses listed in the USMS data. The original arrest offense codes included many very similar offense descriptions, including some that were slightly more detailed versions of others (for instance, “vehicle theft” and “vehicle theft by bailee”). Often the more detailed ones were rarely used. Therefore, the smallest categories were combined with others that could describe the same legal offense.35 In addition, I subdivided some of the drug arrest offense codes based on the drug type information in the EOUSA investigation-stage file. This is because many drug cases are simply given the arrest code “dangerous drugs,” and because the cocaine arrest codes combine crack and powder, which have different sentencing schemes. There were 123 resulting arrest-code groups. The results are robust to the use of the original offense codes. Note that the drug offense codes do not specify quantity. The drug quantity at arrest (in addition to type) is usually identified by the EOUSA investigation-stage file; however, the quantity field is unreliable starting in FY 2004.36 Therefore, the main analyses do not include quantity in the controls, but robustness checks confined to FY 2001-03 do. To enable quantity comparisons across drug types, quantities were translated into “marijuana equivalents” according to the conversion tables in the Sentencing Guidelines. 3.3. Criminal History Criminal history data are only available in the USSC data and are accordingly only available for those sentenced for guideline offenses. The variable used was the defendant's criminal history category, which ranges from 1 to 6 and forms the basis of the Guidelines sentencing grid. In 0.2% of the sentencing sample, this field was originally missing but could be calculated based on another Sentencing Commission field called "criminal history points," according to the rules laid out in the Guidelines. 3.4. Charge Severity Measures The raw charge and conviction data are the statutory provisions under which the defendant was charged and convicted. These provisions had to be translated into measures of charge severity in order to assess the contribution of charging and conviction severity to sentence disparities.37 On the basis of legal research, I identified the statutory maximum and 35 No single number defined what categories were small enough to be combined, because the combination depended on the legal assessment that the crimes were sufficiently similar. 36 There are drastic changes in the apparent quantity distribution in this field from 2003 to 2004 as well as large inconsistencies in quantity between this field and the sentencing-stage quantities recorded by USSC beginning in 2004. EOUSA adopted a new data entry system in 2004, and it seems apparent that the problem is with this system; unfortunately the inconsistencies appeared neither to be uniformly applicable nor confined to particular drug types or districts, so there is no way to identify which cases are problematic. 37 While the AOUSC data include a “severity” field, which is ostensibly based on the statutory maximum, it is not very useful because appears to automatically be based on the very highest maximum contained anywhere in the statute cited, even when that maximum is only triggered by an exceptional circumstance that rarely applies. For instance, charges under 18 U.S.C. § 1347 (health care fraud) are coded by AOUSC as having a statutory maximum of life, even though that maximum only applies when the fraud leads to a death. iii Starr—Estimating Gender Disparities in Federal Criminal Cases minimum sentence, and the Guidelines-recommended sentence associated with each combination of charges and convictions. Because the cited statutory provisions sometimes contain varied sentencing schemes depending on the facts of the case, I researched the most common ways in which these statutes are charged in order to be able to make realistic assumptions in the face of such ambiguities. When possible, ambiguities were resolved by reference to the other charges in the case, when the legal elements of those charges revealed additional facts that the prosecutor must have been alleging. For instance, suppose Charge 1 is a burglary offense that usually has a maximum sentence of 10 years, but has a 20-year maximum if someone is seriously injured in the course of the burglary. Charge 2 is an aggravated assault charge, with a 15-year maximum, in which aggravated assault is defined to require that serious injury be proven. Because Charge 2’s presence indicates that the prosecutor was alleging serious injury, the maximum sentence for Charge 1 is raised to 20 years. Implementing this approach required constructing a number of flags for every federal criminal statute, a complicated statutory interpretation task. The flags indicated whether certain facts were elements of the crime: death, injury, serious injury, drug crime, sex crime, fraud, official victim, minor victim, terrorist motive, an assault, use of a weapon, use of a gun specifically, a “crime of violence,” obstruction of justice, taking a person for ransom, and whether the crime was a predicate offense for the crime of felony murder. Statutes also had to be coded to reflect adjustments to the statutory or guidelines sentences that would be triggered by the presence of particular facts as identified by the flags for the other charges in the case. Remaining ambiguities were resolved according to default assumptions that varied between the severity measures.38 Constructing a measure of the Guidelines sentence involved additional challenges. First, the statutory provisions cited by AOUSC had to be matched to corresponding Sentencing Guidelines. The actual Guidelines range calculated by the judge is not solely determined by the charges; rather, it is heavily driven by sentencing fact-finding. However, the point of the charge-severity measures is to distinguish the effect of charging and conviction severity itself from that of sentencing fact-finding. Thus, the Guidelines-based measures of charge and conviction severity represent base offense levels determined solely by what the prosecutor charged (or what the defendant was convicted of), that is, the elements of the crime. It is based on applying the Guidelines assuming the elements of all charges brought were proven, but no additional findings of fact were made at sentencing. The Guidelines define the “offense level”—a severity scale running from 1 to 43— associated with each offense. In order for the units of this measure to be comparable to the other metrics, this offense level had to be converted into an implied sentence length in months. Under the Guidelines, offense levels translate mechanically into sentence ranges based on a grid, with criminal history as the other axis. The same column (Column 6) was used for the translation in all cases, such that the charging and conviction measures are blind to the defendant’s actual criminal history—they reflect charge severity alone, and criminal history is a separate covariate. The number of months used was the low end of the range in the applicable grid cell. 38 A detailed spreadsheet showing these flags and other details on coding choices is available on request. iv Starr—Estimating Gender Disparities in Federal Criminal Cases Once the severity of the individual charges were coded, they were combined into total severity measures for all charges. In general, the severity of federal cases is determined by the most serious charge alone, because concurrent sentencing is the default rule. Thus, secondary charges affected the charge severity measures only when one of the charging statutes was an offense specifying that consecutive sentencing was required. As described above, however, information drawn from secondary charges could be used to adjust the coding of the primary charge. This approach to combining charges follows the method specified in the Sentencing Guidelines (see U.S.S.G. § 5G1.2). Two final adjustments were then made. First, the statutory minimum and the sum of the individual-charge maximums were imposed as lower and upper constraints, respectively, on the Guidelines sentence, which also tracks sentencing law (see U.S.S.G. § 5G1.2). Second, zeros on the statutory maximum, guidelines, and mean sentence scales were replaced with half a month—half of the lowest nonzero values otherwise calculated—to reflect the fact that no criminal charge truly has zero severity, even if no incarceration is imposed. This adjustment affected only 0.05% of cases for the statutory maximum measure, 0.2% of cases for the guidelines measure, and 0.5% for the mean sentence measure. The mandatory minimum measure was turned into an indicator variable for whether there was any nonzero mandatory minimum and (for alternate specifications) a categorical variable designating whether the mandatory minimum was 0, less than 10 years, and 10 years or more. Similar variables were constructed based on the actual mandatory minimum of conviction recorded at the sentencing stage in the USSC data. 3.5. Conviction and Sentence Outcomes A dummy variable for whether the defendant was convicted of a non-petty offense was constructed based on AOUSC records. Non-petty offenses are those carrying more than six months as a statutory maximum, so the classification of offenses is based on the statutory maximum measure described above. Conviction of a non-petty offense is a prerequisite for inclusion in the Sentencing Commission data. Sentence data were drawn from the Sentencing Commission and are therefore only available for those convicted of offenses covered by the sentencing guidelines. Sentence lengths were truncated at 540 months, and life sentences were given that value. This length is longer than the highest non-life statutory maximum found in federal law (480 months), and corresponds approximately to the remaining life expectancy of an American of the sampleaverage age. Only 0.7% of sentenced cases were affected by this truncation. v Starr—Estimating Gender Disparities in Federal Criminal Cases Data Sources U.S. Census Bureau, 2000. “Census of Population and Housing, Summary File 3.” http://www.census.gov/census2000/sumfile3.html (last updated October 13, 2011). U.S. Department of Justice. Office of Justice Programs. Bureau of Justice Statistics. 2009. Federal Justice Statistics Program: Paired-Agency Linked Files, 2009. Ann Arbor, MI: Inter-university Consortium for Political and Social Research. ICPSR Study Number 30701-v3 (2011-11-11). U.S. Department of Justice. Office of Justice Programs. Bureau of Justice Statistics. Federal Justice Statistics Program: Arrests and Bookings for Federal Offenses, 2000-2009. Ann Arbor, MI: Inter-university Consortium for Political and Social Research. ICPSR Study Numbers 24126-v2 (2011-03-08), 24145-v2 (2011-03-08), 24164.v2 (2011-0308), 24181.v2 (2011-03-08), 24216.v2 (2011-03-08), 24199.v2 (2011-03-08), 24211.v2 (2011-03-08), 24226.v2 (2011-03-08), 24231.v2 (2011-03-08), 29428.v2 (2011-03-08), 30794-v1 (2011-07-22). Original Data Source: U.S. Marshals’ Service (“USMS”). U.S. Department of Justice. Bureau of Justice Statistics. Federal Justice Statistics Program: Suspects in Federal Criminal Matters Concluded, 2000-2009 [United States]. Ann Arbor, MI: Inter-university Consortium for Political and Social Research. ICPSR Study Numbers: 24120-v2 (2011-03-08), 24139.v2 (2011-03-08), 24158.v2 (2011-03-08), 24175.v2 (2011-03-08), 24193.v2 (2011-03-08), 24210.v2 (2011-03-08), 24225.v2 (2011-03-08), 29424.v2 (2011-03-08), 30790.v1 (2011-06-03). Original Data Source: Executive Office of U.S. Attorneys (“EOUSA Matters Out”). U.S. Department of Justice. Bureau of Justice Statistics. Federal Justice Statistics Program: Defendants Charged in Criminal Cases Filed in District Court, 2000-2009 [United States]. Ann Arbor, MI: Inter-university Consortium for Political and Social Research. ICPSR Study Numbers: 24121-v2 (2011-03-08), 24140.v2 (2011-03-08), 24159.v2 (2011-03-08), 24176.v2 (2011-03-08), 24194.v2 (2011-03-08), 24211.v2 (2011-03-08), 24226.v2 (2011-03-08), 29426.v2 (2011-03-08), 30791.v1 (2011-06-03). Original Data Source: Executive Office of U.S. Attorneys (“EOUSA Cases In”). U.S. Department of Justice. Bureau of Justice Statistics. Federal Justice Statistics Program: Defendants in Federal Criminal Cases -- Terminated, 2000-2009 [United States]. Ann Arbor, MI: Inter-university Consortium for Political and Social Research. ICPSR Study Numbers: 24122-v2 (2011-03-08), 24141.v2 (2011-03-08), 24160.v2 (2011-03-08), 24177.v2 (2011-03-08), 24195.v2 (2011-03-08), 24212.v2 (2011-03-08), 24227.v2 (2011-03-08), 29433.v2 (2011-03-08), 30792.v1 (2011-06-03). Original Data Source: Executive Office of U.S. Attorneys (“EOUSA Cases Out”). U.S. Department of Justice. Bureau of Justice Statistics. Federal Justice Statistics Program: Defendants in Federal Criminal Cases Filed in District Court, 2000-2009 [United States]. Ann Arbor, MI: Inter-university Consortium for Political and Social Research. ICPSR Study Numbers: 24114-v2 (2011-03-08), 24133.v2 (2011-03-08), 24152.v2 (2011-03-08), 24169.v2 (2011-03-08), 24186.v2 (2011-03-08), 24204.v2 (2011-03-08), 24221.v2 (2011-03-08), 29402.v2 (2011-03-08), 30781.v1 (2011-06-03). Original Data Source: Administrative Office of the U.S. Courts (“AOUSC Cases In”). vi Starr—Estimating Gender Disparities in Federal Criminal Cases U.S. Department of Justice. Bureau of Justice Statistics. Federal Justice Statistics Program: Defendants in Federal Criminal Cases in District Court -- Terminated, 2000-2009 [United States]. Ann Arbor, MI: Inter-university Consortium for Political and Social Research. ICPSR Study Numbers: 24115-v2 (2011-03-08), 24134.v2 (2011-03-08), 24153.v2 (2011-03-08), 24170.v2 (2011-03-08), 24187.v2 (2011-03-08), 24205.v2 (2011-03-08), 24222.v2 (2011-03-08), 29242.v2 (2011-03-08), 30784.v1 (2011-0603). Original Data Source: Administrative Office of the U.S. Courts (“AOUSC Cases Out”). U.S. Department of Justice. Bureau of Justice Statistics. Federal Justice Statistics Program: Defendants Sentenced Under the Sentencing Reform Act, 2001-2009 [United States]. Ann Arbor, MI: Inter-university Consortium for Political and Social Research. ICPSR Study Numbers: 24127-v2 (2011-03-08), 24146.v2 (2011-03-08), 24165.v2 (2011-0308), 24182.v3 (2011-03-08), 24200.v3 (2011-03-08), 24217.v3 (2011-03-08), 24232.v2 (2011-03-08), 29381.v2 (2011-03-08), 30795.v1 (2011-06-06). Original Data Source: U.S. Sentencing Commission (“USSC”). U.S. Department of Justice. Office of Justice Programs. Federal Bureau of Investigation. Uniform Crime Reporting Program Data: County-Level Detailed Arrest and Offense Data, 2007-2009. Ann Arbor, MI: Inter-university Consortium for Political and Social Research. ICPSR Study Numbers: 30763-v1 (2012-01-25), 27644-v1 (2011-04-21), 25114-v1 (2009-07-31). vii