The Effects of Male Incarceration Dynamics on Acquired Immune Deficiency Syndrome Infection Rates among African American Women and Men, Chicago Journals, 2014
Download original document:
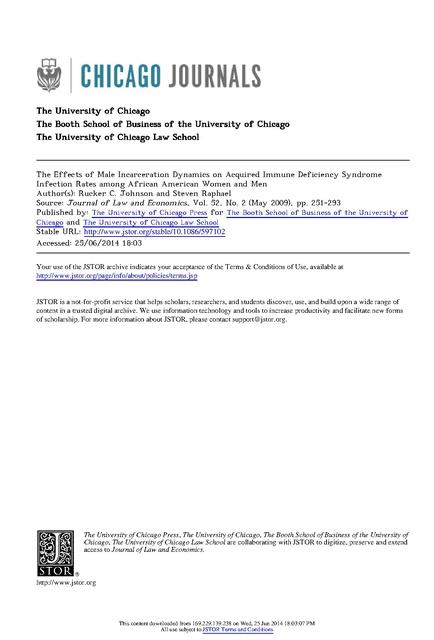
Document text
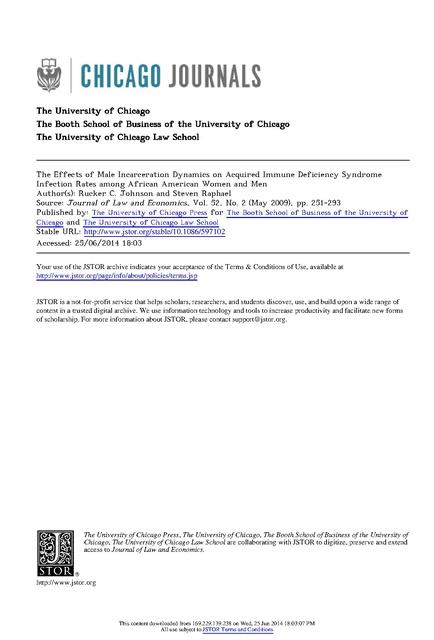
Document text
This text is machine-read, and may contain errors. Check the original document to verify accuracy.
The University of Chicago The Booth School of Business of the University of Chicago The University of Chicago Law School The Effects of Male Incarceration Dynamics on Acquired Immune Deficiency Syndrome Infection Rates among African American Women and Men Author(s): Rucker C. Johnson and Steven Raphael Source: Journal of Law and Economics, Vol. 52, No. 2 (May 2009), pp. 251-293 Published by: The University of Chicago Press for The Booth School of Business of the University of Chicago and The University of Chicago Law School Stable URL: http://www.jstor.org/stable/10.1086/597102 . Accessed: 25/06/2014 18:03 Your use of the JSTOR archive indicates your acceptance of the Terms & Conditions of Use, available at . http://www.jstor.org/page/info/about/policies/terms.jsp . JSTOR is a not-for-profit service that helps scholars, researchers, and students discover, use, and build upon a wide range of content in a trusted digital archive. We use information technology and tools to increase productivity and facilitate new forms of scholarship. For more information about JSTOR, please contact support@jstor.org. . The University of Chicago Press, The University of Chicago, The Booth School of Business of the University of Chicago, The University of Chicago Law School are collaborating with JSTOR to digitize, preserve and extend access to Journal of Law and Economics. http://www.jstor.org This content downloaded from 169.229.139.238 on Wed, 25 Jun 2014 18:03:07 PM All use subject to JSTOR Terms and Conditions The Effects of Male Incarceration Dynamics on Acquired Immune Deficiency Syndrome Infection Rates among African American Women and Men Rucker C. Johnson University of California, Berkeley Steven Raphael University of California, Berkeley Abstract This paper investigates the connection between incarceration dynamics and acquired immune deficiency syndrome (AIDS) infection rates, with particular emphasis on the black-white AIDS rate disparity. Using case-level U.S. data spanning 1982–96, we model the dynamic relationship between AIDS infection rates and the proportion of men in the age-, state-, and race-matched cohort that are incarcerated. We find strong effects of male incarceration rates on male and female AIDS rates. The dynamic structure of this relationship parallels the incubation time between human immunodeficiency virus infection and the onset of full-blown AIDS. These results persist after controlling for year fixed effects; a fully interacted set of age, race, and state fixed effects; crack cocaine prevalence; and flow rates in and out of prison. The results reveal that higher incarceration rates among black males over this period explain the lion’s share of the racial disparity in AIDS infection among women. 1. Introduction Coincident with the large increase in black male incarceration rates is a pronounced increase in acquired immune deficiency syndrome (AIDS) infections We are grateful to David Card, Ken Chay, Sheldon Danziger, William Dow, Robert Greifinger, Theodore Hammett, Harry Holzer, Matt Kahn, Lawrence Katz, Lars Lefgren, David Levine, David Newmark, John Karl Scholz, and Eugene Smolensky for their valuable input and seminar participants at the University of California at Berkeley, Harvard University, Princeton University, University of Michigan, University of Wisconsin–Madison, Yale University, New York University, Brown University, University of Maryland, the Population Association of America Economic Demography Workshop, and the Public Policy Institute of California for helpful comments and discussion. We also wish to thank Steven Levitt for sharing data on the prevalence of crack cocaine, Peter Bacchetti for sharing data on the acquired immune deficiency syndrome (AIDS) incubation period distribution, and Matthew McKenna of the Centers for Disease Control and Prevention for providing useful information about the data collection process for AIDS cases. We thank the Russell Sage Foundation for its financial support of this project. [Journal of Law and Economics, vol. 52 (May 2009)] 䉷 2009 by The University of Chicago. All rights reserved. 0022-2186/2009/5202-0011$10.00 251 This content downloaded from 169.229.139.238 on Wed, 25 Jun 2014 18:03:07 PM All use subject to JSTOR Terms and Conditions 252 The Journal of LAW & ECONOMICS among African American women and men. Between 1970 and 2000, the proportion of black men incarcerated increased from .03 to .08, with a much larger increase in the proportion that has ever been to prison. There was no comparable increase among non-Hispanic white men. Concurrently, the human immunodeficiency virus (HIV)/AIDS infection rate among African American women went from zero during the pre-epidemic period to an annual rate of 55 per 100,000 between 2000 and 2003, a figure 19 times higher than that for nonHispanic white women. For African American men, this rate exceeds 100 per 100,000, in contrast to less than 15 per 100,000 among non-Hispanic white men. Moreover, African Americans (12 percent of the overall population) accounted for half of the AIDS cases reported in 2002. Racial differences in HIV/AIDS infection rates are not well understood. Individual-level risk factors alone have proven inadequate to explain the substantial geographic heterogeneity in the diffusion patterns of the AIDS epidemic in the United States both between and within racial/ethnic groups. Researchers have yet to identify the mechanisms by which the AIDS epidemic transformed from one impacting almost exclusively young gay men to a disease increasingly transmitted through heterosexual sex that disproportionately afflicts minority women. In this paper, we investigate the potential connection between incarceration dynamics and AIDS infection rates. We estimate the effects of changes in male incarceration rates on male and female AIDS infection rates and assess the extent to which high levels of black male incarceration explain the black-white AIDS rate disparity. We hypothesize that changes in male incarceration rates alter HIV transmission risks within defined sexual relationship markets through a number of channels. In particular, male incarceration lowers the sex ratio (male to female), disrupts the continuity of heterosexual relationships, and increases the exposure of incarcerated men to high-risk sex amid a population with a high prevalence of HIV. All of these factors elevate an individual’s or group’s AIDS infection risk and should disproportionately affect the AIDS infection rates of black women and men. Following Charles and Luoh (2005), we exploit the fact that the overwhelming majority of sexual relationships, as well as marriages, occur between women and men of similar age, race/ethnicity, and geographic location (Laumann et al. 1994). To identify the effect of incarceration on AIDS infection rates, we exploit this social stratification and the tremendous variation in incarceration trends over the past 2 decades within these groups. We construct a panel data set of AIDS infection rates covering the period 1982–96 that varies by year of onset, mode of transmission, state of residence, age, gender, and race/ethnicity. Using data from the U.S. Census, we construct a conforming panel of current and cohort-specific lagged male and female incarceration rates. We use these panel data to model the dynamic relationship between male and female AIDS infection rates and contemporaneous and lagged changes in male incarceration rates within sexual relationship markets. The im- This content downloaded from 169.229.139.238 on Wed, 25 Jun 2014 18:03:07 PM All use subject to JSTOR Terms and Conditions Incarceration and AIDS Rates 253 pact of incarceration is identified from variation within sexual relationship markets over time. We find very strong effects of male incarceration rates on both male and female AIDS infection rates. The dynamic structure of this relationship—that is, the lagged effects of the proportion of males incarcerated—parallels the distribution of the incubation time between HIV infection and the onset of fullblown AIDS, with small effects for early lags and relatively large effects for later lags. These results are robust to explicit controls for (race-specific) year fixed effects and a fully interacted set of age, race, and state fixed effects. We find similar results in models estimated separately by racial group. The results are robust to controls for the onset of the crack epidemic and for controls for the degree of turnover in the prison population. The magnitudes of the results suggest that higher incarceration rates among black males explain the lion’s share of the black-white disparity in AIDS infection rates among both men and women. 2. Incarceration and HIV/AIDS Transmission among Inmates and the Community How do changes in male incarceration rates affect the rate at which HIV/ AIDS propagates through a given population? A mechanical effect may occur through the incapacitation of a group of high-risk individuals. To the extent that prisons remove from society those whose behavior accelerates the spread of infectious diseases, an increase in incarceration may reduce the overall incidence of HIV/AIDS. Nonetheless, several factors associated with serving time are likely to elevate the risk of infection while incarcerated. To the extent that imprisonment independently elevates transmission risk, increases in incarceration rates may increase HIV infections among inmates as well as members of the communities from which they come and to which they return. Such adverse effects of incarceration are likely to occur through a number of channels. First, the prevalence of HIV infections in U.S. prisons is particularly high.1 Holding sexual practices constant, subjecting higher proportions of the population to higher prevalence environments should directly translate into higher rates of new infections. Second, the sexual activity that occurs in male prisons is particularly risky. Unprotected male homosexual activity is associated with by far the highest per1 The overall prevalence of the human immunodeficiency virus (HIV) is 2–3 percent among inmates but has been reported to be as high as 17.4 percent in some states (New York in 1993). Roughly one-fourth of those living with HIV in 1997 passed through a correctional facility in that year (Hammett, Harmon, and Rhodes 2002). According to a U.S. Department of Justice report (1993, p. 15), between November 1992 and March 1993, 11,500 AIDS cases were reported in federal prisons, and the number of new cases grew at a rate of approximately 50 percent a year between 1994 and 1997. Thomas and Moerings (1994) give reports from a number of other countries that highlight that HIV/AIDS in prison systems is of worldwide concern. This content downloaded from 169.229.139.238 on Wed, 25 Jun 2014 18:03:07 PM All use subject to JSTOR Terms and Conditions 254 The Journal of LAW & ECONOMICS contact probability of infection.2 Even if the frequency of sexual contact diminishes while incarcerated, the higher per-contact risk may elevate the overall periodic transmission rate.3,4 Third, the configuration of sexual relationship networks—who has sex with whom and over what time frame—in prison is likely to enhance the efficiency of HIV transmission. Sexual networks characterized by serial monogamy provide a less efficient environment for a communicable disease to spread. On the other hand, network configurations in which a small number of individuals have repeated sexual encounters with a large number of partners—either through rape, prostitution, or otherwise—more speedily connect members within a network through the high-risk central network member. A fourth avenue by which higher male incarceration rates may augment AIDS incidence is through the effect of incarceration on the number of lifetime sex partners and the likelihood of concurrency.5 Incarceration dynamics destabilize existing relationships. The typical U.S. prisoner serves a relatively short spell (a median of 2 years) followed by even shorter spells usually triggered by a parole violation (Raphael and Stoll 2004). The resultant periodic absences from nonincarcerated partners are likely to result in the formation of new relationships by the partners left behind, as well as new sexual relationships among inmates, thus increasing the total lifetime number of partners. Moreover, ancillary relationships may continue after an inmate is released and returns to previous partners, augmenting the extent of concurrency. Finally, a more subtle transmission mechanism may occur through the effect of incarceration on the market conditions within which sexual partners match. As inmates are overwhelmingly male and minority, incarceration disproportionately reduces the ratio of minority men to minority women. This scarcity of minority men improves their bargaining position in negotiating personal relationships while diminishing the ability of minority women to be discriminating in choice of partner and/or to negotiate safer sex practices. The improved terms of trade for men may also translate into men having to display less com2 Probabilistic computations presented in Johnson and Raphael (2006) indicate that the HIV transmission risk associated with one unprotected male homosexual experience with an infected partner (via anal receptive sex) is equivalent to the risk of 85 unprotected encounters with an HIVpositive woman. 3 Existing research suggests that between 20 (Tewksbury 1989) and 65 (Wooden and Parker 1982) percent of male inmates have sex while incarcerated. The National Health and Social Life Survey finds that 12.8 percent of former male inmates report “ever having sex with a man,” compared with 7.9 percent of all other men (Francis 2006). 4 A Bureau of Justice Statistics national survey of administrative records on sexual violence in prison estimates that there were 8,210 allegations of sexual violence in correctional facilities—3.2 allegations per 1,000 inmates (Beck and Hughes 2005). These estimates, however, are likely to be conservative, as victims may be reluctant to report such assaults to prison authorities. 5 Concurrency involves having more than one sexual partner and going back and forth between them. These factors are known to augment the risk of contracting and spreading sexually transmitted diseases (Morris 1997). This content downloaded from 169.229.139.238 on Wed, 25 Jun 2014 18:03:07 PM All use subject to JSTOR Terms and Conditions Incarceration and AIDS Rates 255 mitment or loyalty in seeking sexual relations, which results in less stable relationships.6 Thus, there are many pathways by which incarceration may impact the AIDS infection rates among former inmates and members of their sexual networks.7 The mechanisms noted above—incapacitation effects, elevated transmission rates while incarcerated, and effects of incarceration dynamics on the formation of new sexual relationships and concurrency—should disproportionately impact the African American community in the United States. Nearly one-fifth of black adult males in the United States have served time (Raphael 2005), compared with less than 3 percent of adults white males. The ratio of men to women among the noninstitutionalized population is markedly lower for non-Hispanic blacks than for non-Hispanic whites (Adimora and Schoenbach 2005). Whether these factors translate into greater AIDS infection rates among African Americans is the question to which we now turn. 3. Empirical Framework and Data Description With ideal data, we would model the effects of current and prior incarceration spells on the likelihood of becoming HIV positive by current and former inmates as well as by men and women within the same sexual relationship markets as current and former inmates. Thus, one might model group-level HIV incidence in year t as a function of the proportion of men who have ever served time in jail or prison. The “treatment” for incarcerated men can be viewed as exposure to a high-risk prison environment. For women, the treatment amounts to having men in their sexual relationship market exposed to higher transmission risks. Beyond these two effects, there may still be additional spillover effects on men and women who have never been to prison or who never have sex with a current or former inmate via secondary infections from those who have. The possibility of these secondary infections coupled with a time-served delay between changes in male incarceration and changes in female HIV infections suggests a dynamic relationship between incarceration and HIV incidence with lagged effects of the former on the latter. 6 Charles and Luoh (2005) show that higher rates of male imprisonment lower the likelihood that women marry, reduce the quality of their spouses when they do, and shift the gains from marriage away from women and toward men. In addition, low sex ratios are associated with higher rates of single motherhood, teen pregnancy (Sampson 1995), and incidence of syphilis (Kilmarx, Zaidi, and Thomas 1997) and gonorrhea (Thomas and Gaffield 2003). 7 A few studies attempt to measure the rate at which HIV is transmitted in prisons. One strand of this literature estimates the rate at which new inmates seroconvert (test negative on entering prison and positive at a follow-up date) while incarcerated (Brewer et al. 1988; Horsburgh et al. 1990; Castro et al. 1994; Macalino et al. 2004). A second strand assesses the degree to which longterm prisoners who had been incarcerated since before the start of the AIDS epidemic become infected with the HIV virus (Mutter, Grimes, and Labarthe 1994; Krebs and Simmons 2002). The subset of these studies that tabulate annual transmission rates suggest transmission rates per year served on the order of .1 to .5 percent, a figure roughly 7 to 35 times the rate of transmission for the nation overall. This content downloaded from 169.229.139.238 on Wed, 25 Jun 2014 18:03:07 PM All use subject to JSTOR Terms and Conditions 256 The Journal of LAW & ECONOMICS Unfortunately, representative data on HIV infections do not exist for the United States. However, nearly complete national data on the universe of advanced-stage HIV infections in the United States are publicly available. Thus, in this paper we model the determinants of AIDS (or advanced-stage HIV) infections. One benefit of analyzing the determinants of AIDS cases rather than early-stage HIV (which is often asymptomatic) is that it minimizes the differences in reporting rates across groups that are simply an artifact of differential interaction with the health care system, resulting in differences in early detection. A further complication, however, is that the average lag for a model of AIDS cases is likely to be larger than the comparable lag for HIV infections because of reasons beyond the factors already noted. For both genders, variance in the AIDS incubation distribution—where incubation is defined as the time between HIV infection and the development of a measurably suppressed immune system—will induce a lag between any incarceration-induced infections and newly diagnosed AIDS cases. Estimates of the cumulative distribution function (CDF) of incubation for the pre-1996 period8 reveal sharp increases in the proportion developing full-blown AIDS starting 3 years after seroconversion and a flattening of the CDF at around 10 years after infection (Bacchetti 1990; Brookmeyer 1991; U.K. Register of HIV Seroconverters Steering Committee 1998).9 Thus, our model specification must account for the likely long lag function relating changes in incarceration to changes in AIDS infection rates. Following Charles and Luoh (2005), our empirical strategy builds on the fact that the overwhelming majority of marriages occur between men and women of similar age, race/ethnicity, and geographic location. Moreover, these endogamous patterns mirror the stratification of sexual relationships along these lines, thus creating sharp and distinct sexual relationship markets (Laumann et al. 1994). We exploit this empirical regularity and the substantial variation in the incarceration trends over this period that occur within these demographic groups. Accordingly, we define sexual relationship markets by the interaction of race, state of residence, and age. We use the proportion of men incarcerated to capture the proportion of the relationship market’s population at risk in a given year. We then model AIDS incidence as a function of contemporaneous and lagged changes in the fraction of men incarcerated at the relationship market level. 8 The AIDS incubation period was altered considerably by the introduction of antiretroviral drugs in 1996, with the variance increasing considerably along with the median and mean time to the development of symptoms. For this reason, our empirical tests focus on the pre-1996 period. 9 These estimates suggest that roughly one-quarter of HIV-positive individuals develop AIDS within 6 years, one-half within 9 years, and three-quarters within 12 years. For women who are at risk of infection via heterosexual relationships with former inmates, time served also induces a lag between men becoming infected while incarcerated and the ultimate infection of female partners. There is evidence that people are most infectious in the first few months after becoming infected and again when the disease develops into AIDS (Jaquez et al. 1994). This content downloaded from 169.229.139.238 on Wed, 25 Jun 2014 18:03:07 PM All use subject to JSTOR Terms and Conditions Incarceration and AIDS Rates 257 Specifically, our principal estimates come from estimation of the regression 冘 13 AIDSRatersat p tp0 冘 13 qmt IM rsa,t⫺t ⫹ qft IFrsa,t⫺t tp0 (1) ⫹ drsa ⫹ l rt ⫹ fst ⫹ pat ⫹ Rsat, where r indexes racial/ethnic groups, s indexes state of residence, a indexes age groups, and t indexes year of infection. The variable AIDSRatersat measures the number of new AIDS cases diagnosed per 100,000 individuals from race group r, age group a, in state s, during year t; IM rsa,t⫺c provides the male incarceration rate (defined as the proportion incarcerated at a point in time for the given year) for the demographic group rsa for the contemporaneous year of infection and for 13 lagged years; IFrsa,t⫺c provides the comparable incarceration rates for women; drsa denotes a complete set of sexual relationship market fixed effects defined by the interaction of race, state of residence, and age; l rt denotes a complete set of race-specific year effects; fst denotes a complete set of statespecific year effects; pat provides a complete set of age-specific year effects; and rsat is the random error term. We also include a set of educational attainment indicators (high school dropout, high school graduate, some college, college graduate [the reference category]) as additional controls in all models. Finally, the parameters qmt and qft provide the coefficients on the contemporaneous and lagged incarceration rates and provide the principal parameters of interest. An important aspect of the specification of equation (1) is the fact that we are specifying the contemporaneous and lagged male and female incarceration rates to be cohort consistent. This means that we model AIDS infection rates for a specific gender and sexual relationship market as a function of the contemporaneous incarceration rates and the lagged incarceration rates for the specific group. Thus, the 1990 infection rate for black women between 30 and 34 in Georgia is modeled as a function of the current incarceration rate for 30–34year-old black men in Georgia, the 1989 incarceration rate for 29–33-year-old black men in Georgia, the 1988 incarceration rate for 28–32-year-old black men in Georgia, and so on. The inclusion of sexual market fixed effects adjusts for time-invariant marketspecific characteristics, such as drug use prevalence or behavioral norms, factors that are otherwise difficult to quantify. Allowing for race-specific, age-specific, and state-specific individual year effects controls for race- and age-specific trends that might exist in AIDS incidence at the national level and overall trends that may vary by state. Collectively, the inclusion of the sexual relationship market effects and the various time effects means that we are identifying the effect of incarceration on AIDS infection rates using variation in both series occurring within sexual relationship networks after accounting for race, age, and state-level time trends in both variables. We estimate equation (1) using weighted least squares, where we weight by the population size of each group defined by race, state, age, and year. Finally, to ensure that our statistical inferences are robust This content downloaded from 169.229.139.238 on Wed, 25 Jun 2014 18:03:07 PM All use subject to JSTOR Terms and Conditions 258 The Journal of LAW & ECONOMICS to serial correlation in the error term, we calculate the variance-covariance matrix of our parameter vector, allowing for nonzero covariance across observations within sexual relationship markets. We estimate separate models by gender. Since sexually transmitted AIDS infection is the hypothesized chief mechanism linking incarceration and AIDS, we also estimate the equation separately for new AIDS infections contracted through heterosexual (for women) and homosexual (for men) sex, in addition to estimating models for overall AIDS infection rates. Given the high degree of correlation between current and lagged incarceration rates, we use a third-order polynomial distributed (Almon) lag for both male and female incarceration rates to reduce multicollinearity problems and yet allow a fairly flexible structure on the shape of the lag distribution. Our modeling of the lag structure is guided by the medical and epidemiological evidence regarding the pre-1996 incubation period (which suggests no more than two inflections in the incubation probability distribution function). We tested alternative lag lengths and higher order polynomials, but none significantly improved the fit of the model. We further constrain the lag coefficients to zero for those whose transmission effects correspond with time periods that predate the AIDS epidemic (that is, before 1980). For example, for AIDS rates in 1985 we constrain all coefficients on lagged incarceration rates in excess of 5 years to zero; for AIDS rates in 1986 we constrain all coefficients on lagged incarceration rates in excess of 6 years to zero, and so on. These constraints essentially mean that later lags are identified using fewer years of data and AIDS infection rates occurring later in the panel. This specification of the distributed-lag model parallels that of Pakes and Griliches (1984) and Andrews and Fair (1992) in other applications. A detailed discussion of our data is provided in the Appendix. Here we provide a brief overview of our data sources and the manner in which we construct our panel. We use data from the 2001 Centers for Disease Control and Prevention (CDC) AIDS Public Information Data Set (PIDS) as well as the 1980, 1990, and 2000 5-Percent Public Use Microdata Samples (PUMS) from the U.S. Census of Population and Housing. The AIDS PIDS database provides case-level information on all known AIDS cases measured by the national AIDS surveillance system. To construct AIDS infection rates, we first tabulate the total number of newly diagnosed AIDS cases by the state of residence, race, age, gender, and year of diagnosis. We then use data from the 1980, 1990, and 2000 U.S. Census PUMS to estimate the national population corresponding to each state # race # age # gender # year cell for each census year and linearly interpolate population estimates for intercensus years. These two variables are then used to tabulate an AIDS diagnoses per 100,000 individuals. We employ four race/ethnicity categories: non-Hispanic white, non-Hispanic black, non-Hispanic Asian, and Hispanic. We use nine of the 10 age groupings from the AIDS PIDS data.10 The introduction and widespread use of medical 10 The age ranges describing each infected individual refer to age at infection and are 20–24, 25–29, This content downloaded from 169.229.139.238 on Wed, 25 Jun 2014 18:03:07 PM All use subject to JSTOR Terms and Conditions Incarceration and AIDS Rates 259 therapies, particularly medical advances introduced since 1996, have altered or elongated the lagged structure of the relationship between incarceration and AIDS incidence. Thus, we focus on the period from 1982 to 1996. One problem with the AIDS PIDS data concerns the ability to identify the state of residence at the time of diagnosis. To protect confidentiality, roughly 15 percent of AIDS cases observed over this period lack subregion (East, West, South, Midwest) geographic identifiers. Thus, the infections rates in our panel data set are estimated using only 85 percent of the total number of AIDS cases recorded in the United States, encompassing data from 38 states plus Washington, D.C.11 To make use of all cases, we also estimated the models using the fourcategory region of residence to define geographic location. The results are qualitatively and numerically similar to what we present below. With 15 years, 39 states, four race/ethnicity groups, and nine age groups, the dimensions of the panel define 21,060 individual cells for each gender.12 Figures 1 and 2 present our estimates of the annual newly diagnosed AIDS cases (expressed per 100,000) for men and women for 1982–2000.13 Figure 1 reveals that black men are newly diagnosed with AIDS at a rate that is between 3 and 9 times the comparable rate for white men (with the larger figures pertaining to the latter periods). The rate of new AIDS cases for black women is between 12 and 24 times the annual rate of new diagnoses for white women. To estimate male and female incarceration rates, we use the group-quarters identifier included in the PUMS data. This variable identifies individuals residing in nonmilitary institutions (inmates of federal and state prisons, local jail inmates, residents of inpatient mental hospitals, and residents of other nonaged institutions). We use this variable as our principal indicator of incarceration. For the census years 1980, 1990, and 2000, we measure the contemporary incarceration rate for each demographic group defined by state of residence, age group, race/ethnic group, and gender as the proportion of the members of the demographic cell that is institutionalized. For noncensus years, we linearly interpolate the incarceration rate using the estimated rates for the 2 years bracketing the year in question.14 Our model also requires that we estimate lagged incar30–34, 35–39, 40–44, 45–49, 50–54, 55–59, 60–64, and 65 and older. We drop the latter category since many of those 65 and older defined as institutionalized in the census are in nursing homes. 11 The 12 states with missing disaggregated AIDS case-level information are Alaska, Iowa, Idaho, Maine, Mississippi, Montana, North Dakota, New Hampshire, South Dakota, Vermont, West Virginia, and Wyoming. There are also missing state identifiers for some AIDS cases in small rural areas disproportionately in the South. 12 For cells with a positive population estimate and no new AIDS cases, we set the AIDS infection rate to zero. After omitting those cells in which the population estimates from the census are zero, there are 21,018 observations for men and women. 13 For the descriptive statistics in Figures 1–4, we use all AIDS cases recorded in the AIDS Public Information Data Set (PIDS) data set, since the analysis is at the national level. The model estimates that follow are based on the 85 percent of cases in which we can identify the state of residence. 14 We also estimated our models using the time path of the overall state incarceration rates between census years to nonlinearly interpolate group-specific incarceration rates. The results using this alternative are similar to the results presented here. This content downloaded from 169.229.139.238 on Wed, 25 Jun 2014 18:03:07 PM All use subject to JSTOR Terms and Conditions Figure 1. Annual newly diagnosed AIDS cases, men ages 20–64 260 This content downloaded from 169.229.139.238 on Wed, 25 Jun 2014 18:03:07 PM All use subject to JSTOR Terms and Conditions Figure 2. Annual newly diagnosed AIDS cases, women ages 20–64 261 This content downloaded from 169.229.139.238 on Wed, 25 Jun 2014 18:03:07 PM All use subject to JSTOR Terms and Conditions 262 The Journal of LAW & ECONOMICS Table 1 Change in the Proportion of Men Incarcerated, 1982–96 Category Ages 20–64: White Black Hispanic Other Ages 25–34: White Black Hispanic Other 10th 25th 50th 75th 90th ⫺.003 .005 .001 ⫺.009 0 .015 .007 ⫺.001 .002 .035 .012 .002 .006 .05 .016 .004 .009 .064 .02 .01 .001 .03 .005 ⫺.001 .004 .038 .012 .001 .006 .049 .013 .002 .008 .064 .017 .006 .012 .072 .021 .011 Note. Values are percentiles of the distribution of change across all age-state cells within racial groups. The distributions are weighted by the average of the 1982 and 1996 male population of each cell. ceration rates for each demographic group defined by our panel data set. Using a procedure similar to that for estimating contemporary incarceration rates, we construct 13 cohort-specific lagged incarceration rates, where the age bracket and year are adjusted for the lag length. Again, the details of this imputation are discussed at length in the Appendix. 4. Descriptive Statistics and Preliminary Analysis Our empirical strategy relies heavily on the variation occurring within relationship markets and how this within-group variation differs across groups. In addition, the model as laid out in equation (1) imposes, a priori, several functional form restrictions. In this section, we provide a descriptive analysis of the data with an eye on demonstrating the great heterogeneity in the time path of incarceration and AIDS infection rates for our defined relationship markets. We also provide some simple descriptive analysis showing the within-group relationship between these variables, descriptive evidence pertaining to the dynamics of the relationship between incarceration and AIDS, and some simple falsification tests that probe the reasonableness of some of our specification choices. 4.1. Variation in Incarceration and AIDS Infections Table 1 presents key percentiles of the distribution of the total change in incarceration rates between 1982 and 1996 across states for men between the ages of 20–64 and 25–34, respectively. The changes in Table 1 highlight the considerable geographic variation across states in male incarceration growth and sharp between-group differences in incarceration growth. While incarceration rates among young men increased for all groups, young black men exhibited markedly higher increases, followed by young Hispanic men and small increases for young white men. Within racial groups there is substantial variation in incarceration rate levels This content downloaded from 169.229.139.238 on Wed, 25 Jun 2014 18:03:07 PM All use subject to JSTOR Terms and Conditions Incarceration and AIDS Rates 263 Figure 3. Cross-state distributions of AIDS infection rates, men ages 30–44 and changes. For example, in 1996 the state-level incarceration rate for young black men varied from roughly .08 at the 10th percentile to .13 at the 90th. Moreover, the distribution of the changes reveals a change at the 90th percentile (7.2 percentage points) nearly 2.5 times the change at the 10th percentile. As it pertain to AIDS infections, the tails of these distributions are particularly important since a small minority of individuals who engage in high-risk behaviors (or are exposed to high-risk environments) contribute a disproportionate share of HIV infections. Similar disparities and large cross-state variation are also evidenced in AIDS infection rates. Figure 3 displays mean AIDS incidence per 100,000 as well as key percentiles of the cross-state distributions of this variable by race for men between 30 and 44 years of age (the age groups with a particularly high incidence level at the height of the epidemic). Figure 4 presents comparable figures for women. By 1996, the average AIDS infection rate for black men in this age group was 280, compared with 50 and 160 for non-Hispanic whites and Hispanics, respectively. Similar yet proportionally larger racial disparities emerge for women, with overall averages of 125, 10, and 35 cases per 100,000 among black, white, and Hispanic women, respectively. The tremendous cross-state variation in the extent of the AIDS epidemic (as evidenced by the difference between the 90th and 10th percentiles) within racial groups is equally striking. For example, in 1996 the infection rate for black men between 30 and 44 in the state at the 90th percentile of the cross-state distribution is nearly 4 times the comparable rate of the state at the 10th percentile. The comparable 90/10 ratio for black women 30–44 years of age in 1996 is nearly 10. This content downloaded from 169.229.139.238 on Wed, 25 Jun 2014 18:03:07 PM All use subject to JSTOR Terms and Conditions 264 The Journal of LAW & ECONOMICS Figure 4. Cross-state distributions of AIDS infection rates, women ages 30–44 4.2. Correlations between AIDS Infections, Contemporary, and Lagged Incarceration Rates We proceed with a simple graphical analysis of the relationship between changes in incarceration and changes in AIDS infections. We present simple contemporaneous and lagged correlations and provide a first-pass analysis of the timing of the relationship between change in incarceration and change in AIDS infections. To do so, we divide the 15-year period spanning 1982–96 into three 5-year periods of the epidemic: 1982–86 are the early years, 1987–91 are the middle years, and 1992–96 are the peak years. For each period and each sexual relationship market (defined by race, age, and state), we calculate the total number of new AIDS cases (per 100,000) over the 5-year period as well as the corresponding 5-year change, the one-period-lagged 5-year change, and the twoperiods-lagged 5-year change in male incarceration rates. The one-period- and two-periods-lagged changes are calculated for the same cohort—that is, when the individuals were 5 and 10 years younger, respectively. Figure 5 presents scatter plots of cumulative new male infections per 100,000 over the 5-year peak period (1992–96) against the contemporaneous, oncelagged, and twice-lagged changes in incarceration rates. Figure 6 presents the comparable scatter plots for women. For both male and female AIDS infection rates, there is no visible relationship between contemporaneous changes in incarceration and AIDS infections. However, we find that the lagged 5-year changes in incarceration rates are more strongly associated with changes in the number This content downloaded from 169.229.139.238 on Wed, 25 Jun 2014 18:03:07 PM All use subject to JSTOR Terms and Conditions Figure 5. Effects of changes in male incarceration rates on new AIDS infections in men, 1992–96 This content downloaded from 169.229.139.238 on Wed, 25 Jun 2014 18:03:07 PM All use subject to JSTOR Terms and Conditions Figure 6. Effects of changes in male incarceration rates on new AIDS infections in women, 1992–96 This content downloaded from 169.229.139.238 on Wed, 25 Jun 2014 18:03:07 PM All use subject to JSTOR Terms and Conditions Incarceration and AIDS Rates 267 Table 2 New AIDS Cases (per 100,000) Reported in 1987–91 and 1992–96 Men Incarceration Rate Male: DContemporaneous DCohort-consistent 5-year lagged (1) (3) ⫺1,026 (1,292) (4) ⫺363 (1,268) 1,997 (3,498) 55,228** (4,763) 55,473** (4,756) 17,456** (2,104) 17,488** (2,089) ⫺385 (4,976) . . . ⫺5,739** (1,795) . . . DCohort-consistent 5-year lagged . . . R N (2) 1,906 (3,416) Female: DContemporaneous 2 Women .266 3,120 ⫺2,408 (7,584) .266 3,117 . . . .243 3,119 1,501 (1,919) .247 3,117 Note. Incarceration rate changes are expressed in decimal form; for example, .01 represents a 1-percentagepoint change. Standard errors are in parentheses. All regressions include a constant term. ** Statistically significant at the 1% level. of new AIDS cases than are the contemporaneous 5-year changes.15 We find the strongest effect for the two-period-lagged change in incarceration rates. The larger effects of changes in lagged incarceration rates are most clear in the regression results presented in Table 2. Here the dependent variable is the cumulative new AIDS infections over 5-year periods for the latter two periods (1987–96), while the explanatory variables include the contemporaneous and the once-lagged 5-year changes in male incarceration simultaneously. For the male AIDS infection rate model, we find no significant effect of the contemporaneous 5-year change in male incarceration yet strong significant effects of the one-period-lagged change. The same holds true when the dependent variable is changed to female AIDS infection rates. These patterns are quite suggestive of incarceration-induced infections in that we should not expect to see a contemporaneous effect since few HIV-positive transmissions develop into fullblown AIDS in the first several years after acquiring HIV. We should, however, see effects emanating from changes in male incarceration that occurred in previous time periods, and indeed, that is the pattern of effects we find. This simple exercise suggests two falsification tests we can perform that should yield no evidence of incarceration effects, assuming these relationships are not spurious. The first involves testing whether lagged increases in female incarceration rates are independently associated with female AIDS infection rates. The rationale for this test builds on the fact that the transmission risk faced by imprisoned women should be negligible, and thus the incapacitation effect should 15 For both men and women, the slope coefficients on the contemporaneous and lagged incarceration changes are statistically distinguishable from one another at the 1 percent level of confidence. This content downloaded from 169.229.139.238 on Wed, 25 Jun 2014 18:03:07 PM All use subject to JSTOR Terms and Conditions 268 The Journal of LAW & ECONOMICS clearly dominate. Thus, if one were to find significant positive effects of female incarceration rates on AIDS infections, one might be concerned that changes in incarceration may serve as a proxy for changes in drug use or some other behavior. This first specification test is displayed in columns 2 and 4 of Table 2. While the principal test involves the model in which the female infection rate is the dependent variable, we present results for male AIDS infections as well. The contemporaneous change in female incarceration rates exhibits a negative and significant effect on the female AIDS infection rate, while the once-lagged change is statistically insignificant. Note that the effects of the male incarceration variables on female infections are robust to inclusion of these additional variables. In the male infection rate model, the female incarceration variables are both statistically insignificant, while the results for the male incarceration variables are not altered by their inclusion. The second falsification test considers whether lagged changes in incarceration rates that correspond to periods that predate the AIDS epidemic (for our purposes, before 1982) are associated with subsequent increases in AIDS infection rates. For the early and middle periods of the epidemic, we can define lagged 5-year changes in incarceration rates that predate the AIDS epidemic. We should see no significant effects of changes in incarceration rates during these periods (occurring before 1982) and significant effects of changes in incarceration rates occurring during the epidemic (1982 and later). Note that since we constrain the effects of incarceration rates before 1980 to zero in our specification of equation (1), this also serves as a specification check for our more detailed model results presented below. Since we cannot estimate incarceration rates for the 1970s because of data constraints,16 here we use data from the Bureau of Justice Statistics (BJS) to measure the contemporaneous and lagged changes in incarceration rates. Again, we use 5-year cumulative AIDS cases but measured at the state level rather than the state # age # race level. We use the same three 5-year time periods (early, middle, and peak) and define three time periods over which changes in incarceration rates are measured: contemporaneous (t ⫺ 5 to t), once lagged (t ⫺ 10 to t ⫺ 5), and twice lagged (t ⫺ 15 to t ⫺ 10). Define the dimensions t p (early, middle, peak) and a dimension s corresponding to state. We use these data to estimate the model ChangeAIDSst p ast ⫹ x # earlyst ⫹ f # middlest ⫹ b # DIncarcerationcontemporaneous st ⫹ d # DIncarcerationstoncelagged ⫹ g # DIncarcerationoncelagged # earlyst st (2) ⫹ k # DIncarcerationtwicelagged ⫹ p # DIncarcerationsttwicelagged # earlyst st ⫹ l # DIncarcerationsttwicelagged # middlest ⫹ st, 16 The 1970 Public Use Microdata Samples is a 1 percent sample, less than one-fifth the size of the 1980 sample. Incarceration rates at the sex, race, age, and state levels are quite imprecise for many groups. For this reason, we focus on state-level data here. This content downloaded from 169.229.139.238 on Wed, 25 Jun 2014 18:03:07 PM All use subject to JSTOR Terms and Conditions Incarceration and AIDS Rates 269 Table 3 Estimated Effects of Changes in Incarceration Rates on Cumulative AIDS Infections (per 100,000) by Period Contemporaneous Once lagged Twice lagged Early Middle Peak 143.44 (87.77) 172.71a (451.60) 20.21a (389.14) 143.44 (87.77) 463.16* (188.50) 625.13a (458.49) 143.44 (87.77) 463.16* (188.50) 852.11** (250.97) Note. Standard errors are in parentheses. Contemporaneous and lagged effects are estimates from the coefficients of the model specified in equation (2). a Predates the AIDS epidemic. * Statistically significant at the 5% level. ** Statistically significant at the 1% level. where earlyst and middlest are dummy variables corresponding to the early and middle periods of the epidemic. The regression specification allows the effects of lagged incarceration rates to differ when the change occurs during the preAIDS period. For example, the effect of the once-lagged change for the early period of the epidemic (a change in incarceration rates during 1977–81) is given by the sum of the coefficients d and g, while the once-lagged effects for the middle and peak periods is given by d alone. The effects of the twice-lagged changes in incarceration rate are given by k ⫹ p for the early period, k ⫹ l for the middle period, and k for the peak period. Our falsification test involved testing whether the once-lagged effects for the early period and the twice-lagged effects for the early and middle period are zero. To isolate the tests of the significance of changes in incarceration rates during the pre-AIDS period, we impose two constraints on the model in equation (2) to simplify the specification. First, the contemporaneous effects are constrained to be equal across the three periods. Second, the once-lagged effects are constrained to be equal for the middle and peak periods. Table 3 presents estimates of the contemporaneous, once-lagged, and twicelagged incarceration effects for the three 5-year periods. The results show an insignificant once-lagged effect of a change in incarceration for the early period, while the once-lagged effect is positive and significant for the middle and peak periods. The results also show insignificant twice-lagged effects for the early and middle periods, with a significant and large twice-lagged effect for the peak period. Thus, we find no evidence of significant effects of changes in male incarceration rates occurring during the pre-epidemic period on later AIDS infections. However, we find large significant effects of changes occurring during the AIDS epidemic. These dynamic patterns and these specification checks lend support to our specification of the dynamic model in equation (1). 5. Empirical Results from the Dynamic Regression Models In this section we present various estimates of the dynamic model of AIDS transmission presented in equation (1). Our goals are twofold. First, we aim to This content downloaded from 169.229.139.238 on Wed, 25 Jun 2014 18:03:07 PM All use subject to JSTOR Terms and Conditions 270 The Journal of LAW & ECONOMICS estimate the overall dynamic relationship between incarceration rates and AIDS infection rates among men and women. Second, we wish to use these results to provide a statistical accounting of the fraction of the racial differences in AIDS infection rates attributable to differences in incarceration rates. 5.1. Controlling for Incarceration and the Overall Race/Ethnic Differences in Infection Tables 4 and 5 present preliminary estimates of the lagged effects of incarceration on AIDS incidence using a restrictive version of the model in equation (1). Table 4 models the AIDS infection rate for men. For each dependent variable, the table presents two specifications: (1) a model including race, year, state, and age effects, and (2) a model with all of these fixed effects plus the contemporaneous and 13 years of lags of the incarceration rate for men and the comparable incarceration rates for women. Included in all models is a set of educational attainment indicators as additional controls. For each model, we display the results for the race dummies and the male incarceration variables only, to conserve space. Column 1 indicates an average black-white difference of 78 incidents per 100,000 over the course of the panel among men. The comparable Hispanicwhite and other race-white differentials are 41 and ⫺9, respectively. Controlling for incarceration rates eliminates the black-white and the Hispanic-white differences and slightly widens the other-white difference in infection rates. There are no measurable effects of contemporaneous incarceration rates, while the magnitude of the lagged effects increase with time (becoming significant at lag year 4, reaching a maximum at lag year 10, and remaining significant through lag year 13). Columns 3 and 4 reproduce these models for homosexually contracted AIDS incidence. The race effects presented in column 3 are considerably smaller than the effects presented in column 1. This is consistent with the fact that transmission through homosexual contact is a proportionally less important avenue of transmission for black men relative to white men. Nonetheless, the average annual infection rates for black men are considerably higher than those for white men (by roughly 36 per 100,000), while the transmission rates for Hispanic men are not significantly different than those of white men. For the black-white difference, controlling for incarceration reduces the coefficient on the black dummy from roughly 36 to ⫺8. The lag coefficients on the male incarceration rates parallel those in column 2 with two important differences. First, the magnitudes of the lag coefficients are considerably smaller. Second, the contemporaneous incarceration effect is positive. Given that only a small fraction of those who contract HIV develop AIDS within the same year of being infected, any contemporaneous effects are likely to be driven by something other than transmission while incarcerated. For This content downloaded from 169.229.139.238 on Wed, 25 Jun 2014 18:03:07 PM All use subject to JSTOR Terms and Conditions Table 4 Male Incarceration Rates and Racial/Ethnic Differences in AIDS Infection Rates among Men, 1982–96 AIDSrsat from Any Source Variable Black Hispanic Asian Male incarceration ratersat Male incarceration ratersat⫺1 Male incarceration ratersat⫺2 Male incarceration ratersat⫺3 Male incarceration ratersat⫺4 Male incarceration ratersat⫺5 Male incarceration ratersat⫺6 Male incarceration ratersat⫺7 Male incarceration ratersat⫺8 Male incarceration ratersat⫺9 Male incarceration ratersat⫺10 Male incarceration ratersat⫺11 Male incarceration ratersat⫺12 Male incarceration ratersat⫺13 Homosexually Contracted AIDSrsat (1) (2) (3) (4) 77.5021** (7.6323) 41.1514** (12.0986) ⫺8.7383 (7.5900) 14.0109 (10.2900) 10.0242 (9.9014) ⫺33.8370** (6.3124) ⫺.3626 (1.3827) ⫺.6083 (.6654) ⫺.3429 (.6144) .3207 (.7415) 1.2695⫹ (.7543) 2.3904** (.6730) 3.5705** (.5976) 4.6967** (.6287) 5.6560** (.7445) 6.3355** (.8382) 6.6221** (.8316) 6.4029** (.7421) 5.5647** (.8814) 3.9947* (1.6478) 35.6578** (4.1719) 10.6819 (7.2132) ⫺29.8663** (5.4176) ⫺7.9931⫹ (4.1828) ⫺8.2479 (6.9309) ⫺40.3626** (5.1110) 2.3335** (.5918) .8995** (.2686) .1639 (.2427) ⫺.0116 (.3034) .2344 (.3106) .7635** (.2711) 1.4373** (.2254) 2.1172** (.2233) 2.6648** (.2641) 2.9416** (.2969) 2.8092** (.2821) 2.1292** (.2237) .7629* (.2986) ⫺1.4280* (.6626) Note. The reference category is white. All models include controls for education, year, state, and age group. Columns 2 and 4 estimate constrained 13-year distributed lag models, using a third-order polynomial to represent the lag weights. These models include the same series of lagged female incarceration rates as shown for men; coefficient estimates on these variables are suppressed. All regressions are weighted by cell frequency. Robust standard errors (clustered on race # state # age) are in parentheses. N p 21,060. ⫹ Significant at the 10% level. * Significant at the 5% level. ** Significant at the 1% level. This content downloaded from 169.229.139.238 on Wed, 25 Jun 2014 18:03:07 PM All use subject to JSTOR Terms and Conditions Table 5 Male Incarceration Rates and Racial/Ethnic Differences in AIDS Infection Rates among Women, 1982–96 AIDSrsat from Any Source Variable Black Hispanic Asian Male incarceration ratersat Male incarceration ratersat⫺1 Male incarceration ratersat⫺2 Male incarceration ratersat⫺3 Male incarceration ratersat⫺4 Male incarceration ratersat⫺5 Male incarceration ratersat⫺6 Male incarceration ratersat⫺7 Male incarceration ratersat⫺8 Male incarceration ratersat⫺9 Male incarceration ratersat⫺10 Male incarceration ratersat⫺11 Male incarceration ratersat⫺12 Male incarceration ratersat⫺13 Heterosexually Contracted AIDSrsat (1) (2) (3) (4) 21.8181** (2.6804) 5.1703 (4.1633) 10.5511** (2.3292) ⫺8.8896* (3.8945) ⫺8.4996* (4.0854) ⫺2.4866 (2.1424) .0120 (.5441) .2716 (.2420) .5137* (.2472) .7393* (.3157) .9490** (.3283) 1.1436** (.3016) 1.3239** (.2784) 1.4906** (.2954) 1.6445** (.3425) 1.7863** (.3807) 1.9168** (.3842) 2.0367** (.3752) 2.1468** (.4818) 2.2478** (.8331) 7.5808** (.8331) 2.2380 (1.5878) 3.5738** (.7532) ⫺5.7682** (1.5327) ⫺3.5064* (1.7122) ⫺1.5494⫹ (.8862) .2758 (.2144) .3002** (.0887) .3208** (.0984) .3397** (.1286) .3590** (.1320) .3807** (.1171) .4071** (.1029) .4401** (.1083) .4819** (.1291) .5345** (.1467) .6001** (.1495) .6807** (.1481) .7785** (.1978) .8954* (.3487) Note. The reference category is white. All models include controls for education, year, state, and age group. Columns 2 and 4 estimate constrained 13-year distributed lag models, using a third-order polynomial to represent the lag weights. These models include the same series of lagged female incarceration rates as shown for men; coefficient estimates on these variables are suppressed. All regressions are weighted by cell frequency. Robust standard errors (clustered on race # state # age) are in parentheses. N p 21,051. ⫹ Significant at the 10% level. * Significant at the 5% level. ** Significant at the 1% level. This content downloaded from 169.229.139.238 on Wed, 25 Jun 2014 18:03:07 PM All use subject to JSTOR Terms and Conditions Incarceration and AIDS Rates 273 Table 6 Effect of Male Incarceration Rates on AIDS Infection Rates among Men and Women, 1982–96 Men (N p 21,060) Male Incarceration Ratersat Variable Contemporaneous year (t) Lag year t⫺1 Lag year t⫺2 Lag year t⫺3 Lag year t⫺4 Lag year t⫺5 Lag year t⫺6 Lag year t⫺7 Lag year t⫺8 Lag year t⫺9 Lag year t⫺10 Lag year t⫺11 Lag year t⫺12 Lag year t⫺13 1.3950 .4672 .0730 .1207 .5184 1.1743⫹ 1.9967** 2.8936** 3.7734** 4.5442** 5.1142** 5.3915** 5.2844** 4.7012* (1.7869) (.9358) (.7604) (.8256) (.7937) (.6573) (.5386) (.5958) (.7793) (.9302) (.9583) (.8875) (1.0547) (1.9139) Women (N p 21,051) Male Incarceration Ratersat .2836 (.9859) .6427 (.4837) .9140** (.3328) 1.1118** (.3897) 1.2509** (.4210) 1.3455** (.3989) 1.4104** (.3643) 1.4601** (.3678) 1.5090** (.4168) 1.5717** (.4708) 1.6628** (.4940) 1.7967** (.5012) 1.9881** (.6178) 2.2514* (1.0064) Female Incarceration Ratersat 1.7925 1.0156 .5333 .2806 .1924 .2036 .2491 .2639 .1829 ⫺.0591 ⫺.5270 ⫺1.2861 ⫺2.4013 ⫺3.9377 (2.3014) (1.2746) (1.2094) (1.3121) (1.2282) (1.0122) (.8734) (1.0011) (1.2655) (1.4457) (1.4348) (1.3591) (1.8791) (3.4936) Note. The dependent variable is AIDSrsat from any source. Constrained 13-year distributed lag models are estimated using a third-order polynomial to represent the lag weights. These models include the same series of lagged female incarceration rates as shown for men and controls for education and year; coefficient estimates on these variables are suppressed. All models include sexual relationship market fixed effects (race # state # age group), race-specific year effects (year # race), age-group-specific year effects (year # age group), and state-specific year effects (year # state). All regressions are weighted by cell frequency. Robust standard errors (clustered on race # state # age) are in parentheses. ⫹ Significant at the 10% level. * Significant at the 5% level. ** Significant at the 1% level. example, a contemporaneous effect may be indicative of an effect of wide-scale testing of the incarcerated on the number of new diagnoses. Table 5 presents comparable regression results for women. For AIDS cases transmitted by any source, there are large average black-white differentials in the annual average infection rate, even after controlling for education. The blackwhite difference in incidence for women is on the order of 22. This difference is smaller than those observed for men, which reflects the relatively lower infection rates among women. The inclusion of the incarceration rate variables completely eliminates the positive black-white differential in female infection rates. Concerning the lag coefficients on male incarceration rates, there is no measurable effect of the contemporaneous incarceration rate and lagged effects that increase monotonically with the lag length. The results in regressions (3) and (4) modeling heterosexually transmitted infections are similar. Adding the incarceration variables to the specification again eliminates the black-white differential. In fact, the black-white differential becomes negative and significant, which suggests that, holding incarceration rates constant, black women are infected at a lower rate than white women. The shape This content downloaded from 169.229.139.238 on Wed, 25 Jun 2014 18:03:07 PM All use subject to JSTOR Terms and Conditions 274 The Journal of LAW & ECONOMICS of the lag function is similar to that observed for the model using the overall AIDS infection rate, although the coefficients are smaller. 5.2. Allowing for Sexual Relationship Market Fixed Effects Table 6 presents estimates of the lagged effects of male incarceration rate on AIDS infection rates for men and women using the full specification from equation (1). For the male infection rate model, we report only the coefficients on the contemporaneous and lagged male incarceration rates. For the female infection rate model, we report the coefficients for both the set of male incarceration variables and the set of female incarceration variables. For the male infections model, the parameter estimates of the lag coefficients are quite similar to the parameter estimates from the lag coefficients using the somewhat restrictive model in Table 4. There is little evidence of a positive contemporaneous effect of incarceration on male AIDS infections or of effects of the first four lags. The lag coefficients become positive and significant at the fifth lag, increase through the 11th year, decline thereafter, and remain significant through the 13th year. Similarly, the results for women are not appreciably altered by the inclusion of the more liberal set of fixed effects. The lag structure using this more complete specification is nearly identical to that from the restrictive model presented in Table 5. Interestingly, we find no evidence of positive effects of contemporaneous and lagged female incarceration rates on female AIDS infections. These results are consistent with the more informal falsification test presented in the previous section. The lag structures revealed in Tables 4–6 (as well as in the less formal graphical analysis of the previous section) suggest that the effects of male incarceration on AIDS incidence do not surface for several years and increase considerably over an 11-year period for men and over at least a 13-year period for women. Factors that may be driving these delayed responses include delays between prison entry and infection, the known incubation delay between seroconversion and becoming severely immunocompromised, and time delays between male prison admission, female infections, and any other secondary infections that may occur. Thus, for both men and women, the expected patterns of the lagged effects of incarceration parallel the incubation distribution of the disease but with additional delays. In other words, the lag structure should peak later than the peak in the incubation distribution because of factors that cause delay between an increase in incarceration rates and a new HIV infection. To assess whether this is the case, Figure 7 plots the lagged coefficients on male incarceration rates from the models in Table 6 along with two alternative estimates of the probability distribution functions of the incubation period between seroconversion and the onset of AIDS. The first incubation distribution is calculated using the United Kingdom AIDS registry and pertains to HIV infections in the United Kingdom occurring prior to 1996 (U.K. Register of This content downloaded from 169.229.139.238 on Wed, 25 Jun 2014 18:03:07 PM All use subject to JSTOR Terms and Conditions Figure 7. Effects of male incarceration on AIDS rates: 13-year distributed lag model This content downloaded from 169.229.139.238 on Wed, 25 Jun 2014 18:03:07 PM All use subject to JSTOR Terms and Conditions 276 The Journal of LAW & ECONOMICS Seroconverters Steering Committee 1998).17 The second incubation distribution estimate comes from an analysis of the incubation period among homosexual men in San Francisco during the pre-1996 period (Bacchetti 1990). On the basis of both incubation period distribution estimates, the probability of acquiring advanced-stage HIV (following seroconversion) increases in each of years 1 through 7, reaching a peak likelihood in the seventh year and declining thereafter. By comparison, the lagged effects of male incarceration on overall AIDS infections for men follow a similar shape, although they are delayed by an additional 4 years (with a peak at the 11th lag). For women, the delay appears to be greater, as the lag coefficients increase through the 13-year period, which suggests a maximum effect beyond the lag length allowed in our panel regressions. 5.3. Simulating the Effect of Racial Differences in Incarceration on AIDS Infection Rates The results in Tables 4 and 5 indicate that racial differences in incarceration rates largely explain the sizable overall black-white differential in annual AIDS infection rates in models in which the racial differential is constrained to being constant through time. However, the more complete model specification results in Table 6 allow for a more detailed decomposition of the time path of this differential. Using these latter models, we calculate the counterfactual blackwhite difference in AIDS infection rate that would have occurred had black male incarceration rates equaled white male incarceration rates. We do so by subtracting the predicted AIDS differential caused by male differences in incarceration rates from the overall black-white difference in AIDS infection rates.18 Figure 8 displays the actual black-white differential in overall AIDS incidence among men along with the predicted black-white differentials after accounting 17 The figure in the graph smooths the raw estimate of the probability distribution function reported by the U.K. Register of Seroconverters Steering Committee (1998) using a third-order polynomial regression of the infection probability on the time since seroconversion. 18 To illustrate this decomposition, here we present a simplified version of equation (1). Suppose that AIDS infection rates depend on a set of sexual relationship market fixed effects, race-, age-, and state-specific year effects, and the contemporaneous incarceration for males only (the decomposition can be easily extended to the dynamic model we estimate in Tables 4–6). In other words, we would estimate the equation AIDSrast p aras ⫹ grt ⫹ dat ⫹ vst ⫹ bMIrast ⫹ rast. Taking expectations of this equation conditional on race p B and t p t0 and allowing the subscript Bt0 to denote this conditional expectation gives the expression AIDSBt0 p aBt0 ⫹ gBt0 ⫹ dBt0 ⫹ vBt0 ⫹ bMIBt0 , where the first fixed effect is the average network effect for blacks, the second effect is the black time effect for the given year, the following fixed effect is the average age-time effect for blacks, and the remaining provides the average state effect for blacks. If we take a similar expectation for whites and subtract this expectation from that for blacks, we get the final expression AIDSBt0 ⫺ AIDSWt0 p (aBt0 ⫺ aWt0) ⫹ (gBt0 ⫺ gWt0) ⫹ (dBt0 ⫺ dWt0) ⫹ (vBt0 ⫺ vWt0) ⫹ b(MIBt0 ⫺ MIWt0). The first term in the decomposition provides the average black-white differential for the whole panel, the second difference provides the additional period-specific difference, the third term provide the differential attributable to difference in the age distribution, while the next term provides the portion of the differential attributable to difference in the geographic distribution. The final term provides the portion of the difference in AIDS infection rates attributable to racial difference in incarceration rates. The tabulations in Figures 8 and 9 show the overall unadjusted differential (the left-hand side of this equation) as well as the overall differential less the final component of the decomposition due to incarceration. This content downloaded from 169.229.139.238 on Wed, 25 Jun 2014 18:03:07 PM All use subject to JSTOR Terms and Conditions Figure 8. Black-white differential in overall AIDS rates for men This content downloaded from 169.229.139.238 on Wed, 25 Jun 2014 18:03:07 PM All use subject to JSTOR Terms and Conditions 278 The Journal of LAW & ECONOMICS for black-white differences in male incarceration rates. Figure 9 presents the comparable series for women. Figure 8 reveals that racial differentials in incarceration rates explain little of the racial differentials early in the epidemic but account for a proportionally increasing share as we progress through the time period of the panel. In the latter years of the panel, racial differences in incarceration rates account for between 70 and 100 percent of the black-white differences in AIDS infection rates. For women, Figure 9 reveals that accounting for the effect of racial differences in male incarceration rates yields negative black-white differentials in overall AIDS infection rates. In other words, the model predicts that if black male incarceration rates had been at the lower level experienced by whites, black women would have been infected with AIDS at a rate that fell short of that for white women between 1982 and 1996. 6. Probing the Robustness of the Results Thus far, we have documented strong partial correlations between the rate at which men and women become infected with full-blown AIDS and lagged values of the incarceration rate for males in one’s demographic group. These correlations persist with controls for education and when we focus only on variation occurring within sexual relationship markets over time and after removing race-, age-, and state-specific year-to-year changes in both AIDS infection and incarceration rates. These partial correlations are highly significant, and the implied lagged effects of incarceration parallel estimates of the pre-1996 AIDS incubation period distribution. Moreover, the effect sizes suggest that much of the racial differential in AIDS infection rates is attributable to historical differences in the rates at which black men are incarcerated. In this section, we probe the robustness of our results to a number of our specification choices. While we discuss many specification tests, we present results for only a select set in order to conserve space. 6.1. Robustness to the Linear Interpolation of Incarceration Rates As noted above, in constructing our panel, we linearly interpolate contemporaneous and lagged incarceration rates for noncensus years. We reestimated all of these results using a panel in which incarceration rates in noncensus years are estimated using the time path of state-level incarceration rates (taken from BJS data) to nonparametrically apportion the decade-to-decade change across intercensus years.19 The correlation between the linearly interpolated incarceration rates and this more flexible alternative interpolation of noncensus years was high at .93. The results from these models were nearly identical to the results 19 For example, if the aggregate incarceration pattern in Minnesota increased linearly between 1980 and 1990, we would linearly interpolate for noncensus years for the detailed demographic subgroups in Minnesota, but if New Jersey’s incarceration time pattern instead exhibited nonlinearities, increasing at a decreasing rate during the decade of the 1980s, then we would use that structure to connect the end points of the demographic subgroups that reside in New Jersey. This content downloaded from 169.229.139.238 on Wed, 25 Jun 2014 18:03:07 PM All use subject to JSTOR Terms and Conditions Figure 9. Black-white differential in overall AIDS rates for women This content downloaded from 169.229.139.238 on Wed, 25 Jun 2014 18:03:07 PM All use subject to JSTOR Terms and Conditions 280 The Journal of LAW & ECONOMICS presented above. We also estimated our models using current and lagged incarceration data varying at the state-year level, which are not linearly interpolated. These results are also very similar and will be discussed in greater detail. 6.2. Race-Specific Estimates of the Lag Functions Equation (1) constrains the effects of incarceration on AIDS to be similar across racial groups. In Table 7, we relax this constraint. The table presents results from models in which the dependent variables are now race/ethnicity specific. The only notable departures from the constrained results presented in Table 6 are the somewhat weaker lagged effects for white women. The lagged effects are particularly strong for all minority male and female groupings presented and are quite close to the constrained results used to simulate racial differences above. 6.3. Allowing the Effects to Vary by Age Group We estimated separate models in which the effects were permitted to differ between younger (less than 45) and older groups but were constrained to be constant across racial groups. We find somewhat larger male incarceration effects on AIDS incidence for younger age groups. This result is to be expected since the rate of partner change, which affects the transmission speed of HIV, is greater at younger ages. 6.4. Allowing for Alternative Cross-Gender Age Matching We reestimated the incarceration rate lag functions using alternative panel data sets in which female race # state # age groups were matched to men from similar race # state groups but who were either older (by one or two 5-year age groupings) or younger (by one or two age groupings). The rationale of this test is that since sexual transmission is the primary pathway linking male incarceration dynamics to female AIDS infection rates, we would not expect increases in young men’s incarceration rates to have large consequences for much older women’s or much younger women’s AIDS incidence. Indeed, this is the case, as we find much smaller and, in most cases, insignificant effects of male incarceration rates on female AIDS infection rates for incompatible relationship age-matched groups (results available on request). The largest male incarceration effects are found on female AIDS incidence in the same 5-year age-range-matched group. 6.5. Allowing for Alternative Cross-Gender Race Matching As a falsification exercise, we reestimated regression models of AIDS infection rates among black women and added contemporaneous and lagged white male incarceration rates to our usual model specification. The rationale of this test is that since sexual transmission is the primary pathway linking male incarceration dynamics to female AIDS infection rates, we would not expect increases in white men’s incarceration rates to have large consequences for black women’s AIDS This content downloaded from 169.229.139.238 on Wed, 25 Jun 2014 18:03:07 PM All use subject to JSTOR Terms and Conditions This content downloaded from 169.229.139.238 on Wed, 25 Jun 2014 18:03:07 PM All use subject to JSTOR Terms and Conditions White Black 7.1189⫹ 1.1031 (4.2533) (2.9350) 1.4404 ⫺.5296 (3.1245) (1.7257) ⫺1.4508 ⫺1.3093 (2.6186) (1.1760) ⫺2.0901 ⫺1.3810 (2.2911) (1.0452) ⫺1.0130 ⫺.8892 (1.9221) (.9780) 1.2453 .0213 (1.5398) (.8509) 4.1492** 1.2056⫹ (.7236) (1.3452) 7.1634** 2.5191** (1.4905) (.7151) 9.7525** 3.8169** (1.7936) (.8288) 11.3812** 4.9544** (1.9796) (.9357) 11.5140** 5.7868** (1.8625) (.9379) 9.6155** 6.1693** (1.3890) (.8919) 5.1504** 5.9572** (1.2548) (1.2246) ⫺2.4167 5.0057* (2.9291) (2.3023) 8.8982** (2.7212) 4.6809** (1.8030) 2.1918 (1.4138) 1.1249 (1.2969) 1.1741 (1.2434) 2.0331⫹ (1.2119) 3.3960** (1.2393) 4.9566** (1.3329) 6.4088** (1.4343) 7.4465** (1.4599) 7.7635** (1.3599) 7.0537** (1.2357) 5.0110** (1.5719) 1.3294 (2.7680) Hispanic White 1.1444** (.3919) .6431** (.2128) .3025⫹ (.1667) .1001 (.1794) .0134 (.1799) .0199 (.1609) .0971 (.1392) .2225 (.1373) .3736* (.1568) .5278** (.1756) .6626** (.1739) .7556** (.1510) .7842** (.1728) .7259* (.3355) Black Hispanic White .4848 (1.4103) .1427 (.8469) ⫺.0456 (.5150) ⫺.0994 (.3849) ⫺.0381 (.3569) .1190 (.3384) .3525 (.3128) .6429* (.2994) .9710** (.3086) 1.3173** (.3214) 1.6624** (.3133) 1.9871** (.3048) 2.2719** (.4293) 2.4974** (.7933) Black 1.1705 (.8809) 1.3282* (.5513) 1.4462** (.5290) 1.5308** (.5653) 1.5885** (.5477) 1.6255** (.4846) 1.6482** (.4274) 1.6630** (.4311) 1.6763** (.4914) 1.6943** (.5522) 1.7235** (.5687) 1.7702** (.5506) 1.8407** (.6378) 1.9415⫹ (1.0408) .4689** (.1685) .2957** (.0828) .1682** (.0593) .0809 (.0672) .0281 (.0683) .0044 (.0595) .0043 (.0496) .0222 (.0504) .0525 (.0610) .0897 (.0693) .1284⫹ (.0664) .1629** (.0553) .1877* (.0785) .1973 (.1673) White ⫺.1687 (.5212) ⫺.0674 (.2993) .0263 (.1779) .1124 (.1411) .1911 (.1354) .2622* (.1255) .3258** (.1134) .3820** (.1135) .4306** (.1285) .4718** (.1431) .5056** (.1420) .5320** (.1275) .5509** (.1583) .5624⫹ (.3052) Black .0594 (.3547) .2582 (.1878) .4034** (.1543) .5032** (.1726) .5659** (.1747) .5999** (.1567) .6132** (.1361) .6143** (.1358) .6114** (.1586) .6127** (.1854) .6266** (.2024) .6613** (.2183) .7250** (.2795) .8261⫹ (.4410) Hispanic Heterosexually Contracted AIDSrsat Women Hispanic AIDSrsat from Any Source 5.9793 ⫺.4340 4.3079** (4.1330) (1.0168) (1.1540) .7346 ⫺.9441 1.6735** (2.9731) (.7027) (.6227) ⫺1.8863 ⫺.9555 .1752 (2.4181) (.5858) (.6085) ⫺2.3962 ⫺.5854 ⫺.3986 (2.0747) (.5221) (.6540) ⫺1.3084 .0491 ⫺.2596 (1.7218) (.4309) (.5762) .8642 .8309** .3805 (1.3667) (.3074) (.4048) 3.6085** 1.6429** 1.3100** (1.1887) (.2047) (.2854) 6.4114** 2.3679** 2.3174** (1.3291) (.2279) (.4150) 8.7598** 2.8889** 3.1909** (1.6142) (.3277) (.6232) 10.1408** 3.0888** 3.7189** (1.7898) (.3939) (.7519) 10.0412** 2.8505** 3.6897** (1.6869) (.3729) (.7238) 7.9481** 2.0568** 2.8916** (1.2607) (.2460) (.5057) 3.3482** .5906* 1.1131* (1.1640) (.2578) (.4382) ⫺4.2713 ⫺1.6651* ⫺1.8577 (2.7126) (.7515) (1.2473) Homosexually Contracted AIDSrsat Men Note. Constrained 13-year distributed lag models are estimated using a third-order polynomial to represent the lag weights. The models include the same series of lagged female incarceration rates as shown for men and controls for education; coefficient estimates on these variables are suppressed. All regressions are weighted by cell frequency. All models include sex market fixed effects (race # state # age group) and race-specific year effects (race # state # year). Robust standard errors (clustered on race # state # age) are in parentheses. N p 5,265. ⫹ Significant at the 10% level. * Significant at the 5% level. ** Significant at the 1% level. Male incarceration ratersat⫺13 Male incarceration ratersat⫺12 Male incarceration ratersat⫺11 Male incarceration ratersat⫺10 Male incarceration ratersat⫺9 Male incarceration ratersat⫺8 Male incarceration ratersat⫺7 Male incarceration ratersat⫺6 Male incarceration ratersat⫺5 Male incarceration ratersat⫺4 Male incarceration ratersat⫺3 Male incarceration ratersat⫺2 Male incarceration rate rsat⫺1 Male incarceration ratersat AIDSrsat from Any Source Table 7 Effect of Male Incarceration Rates on AIDS Infection Rates by Race/Ethnicity and Gender, 1982–96 282 The Journal of LAW & ECONOMICS incidence, given the substantial degree of racial endogamy. As expected, we find that only black male incarceration rates are significantly positively related to black women’s subsequent AIDS infection rates and that the inclusion of these additional covariates does not impact our estimates. In particular, the lagged effects of the proportion of black males incarcerated parallels the distribution of the incubation time between HIV infection and the onset of full-blown AIDS, with small effects for early lags and relatively large effects for later lags. In contrast, white male incarceration rates do not exhibit this significant pattern on AIDS rates among black women. 6.6. Testing the Stationarity of AIDS and Prison Population Data We use annual state-level data in incarceration rates from the BJS and statelevel AIDS incidence to test for unit roots in both the AIDS incidence and incarceration-rate time series. Ordinary least squares regressions performed on nonstationary data series can yield spurious results unless the trend is removed by direct subtraction or by differencing. The unit root tests, which include statespecific time trends, show that these series appear to be stationary or I(0) processes. We also used the state-level BJS data to reestimate the model in firstdifference form. The first-difference form of the model estimates the annual change in the state’s AIDS incidence rate on 13-year distributed lags of annual changes in the state’s incarceration rate—in other words, the model estimates the effects of an increase in incarceration on the acceleration in the growth of AIDS cases distributed over the subsequent 13-year period. The first-difference results again show significant lagged effects: increases in incarceration rates accelerate the growth rate of AIDS infections, with peak acceleration in years 7 and 8 following the incarceration rate increase (these results are available from the authors on request). 6.7. Altering Sample Periods, Lag Lengths, and Order of the Almon Lag We examined the sensitivity of the results to modest changes in the choice of analysis period and lag length and allowed higher order polynomials of the lag structure for the Almon lag. The results from these checks were not fundamentally altered from the qualitative patterns of results reported in this paper. 6.8. Allowing for Time-Varying Effects of Incarceration Another potential threat to uncovering unbiased estimates of the effects of incarceration dynamics on AIDS infection rates stems from the fact that the strength of any underlying relationship between the probability of acquiring HIV and the proportion that has ever served time in prison may change as the AIDS epidemic progresses. In particular, we expect any relationship between HIV incidence and prison population size to grow stronger over time as the prevalence of HIV increases in the population. This resulting lack of stability in the strength of the relationship could lead to biased estimates of the dynamic structure linking This content downloaded from 169.229.139.238 on Wed, 25 Jun 2014 18:03:07 PM All use subject to JSTOR Terms and Conditions Incarceration and AIDS Rates 283 incarceration rates and AIDS, since the later lagged incarceration coefficients are identified disproportionately from the most recent observation years on AIDS incidence. We examine this issue directly by extending our primary models to allow incarceration effects to vary linearly over the course of the epidemic. We find some evidence of positive interaction effects, which suggests that these effect sizes have increased over the course of the epidemic. 6.9. Exploring Whether Male Incarceration Has a Measurable Effect on Female AIDS Transmission via Intravenous Drug Use As a final robustness check, we explore whether male incarceration rates have any measurable effect on HIV infections among women occurring via intravenous (IV) drug use. The causal factors that we have discussed that link male incarceration to female AIDS infections are primarily sexual. While one can argue that prison-induced AIDS infections among men may lead to higher prevalence among noninstitutionalized IV drug users, this path of infection is less direct. Thus, one might expect to see smaller effects of changes in male incarceration on changes in female AIDS infection through IV drug use relative to infections occurring through heterosexual sex. We explore this possibility by estimating separate models for female AIDS infections contracted through heterosexual sex and through IV drug use. We find once again a lag structure of male incarceration effects that mirrors the incubation period distribution with an additional 2–3-year lag for heterosexually contracted AIDS infection rates, but interestingly, we do not observe any significant lagged effects of incarceration on black women’s infection rates of AIDS contracted via IV drug use. 7. Extending the Model to Incorporate Prison Turnover and Crack Cocaine Usage Our estimation results thus far have modeled AIDS incidence among specific demographic groups as a function of the fraction of the males in the group’s cohort who are currently incarcerated or who have been incarcerated in the past. While we have demonstrated a robust relationship between these sets of variables, one can easily think of some potentially important extensions of this basic empirical model. First, our specification does not allow for an independent effect of prison turnover on AIDS infections, a potentially important complication. Prison populations can expand in two ways: (1) a larger proportion of convicted offenders may be sent to prison, or (2) the sentences given may increase in length. The effects of each of these sources of increases in prison population size on a community’s HIV incidence or risk need not be of the same magnitude or even have the same sign or direction. For example, increasing the time served of the current stock of inmates will, all else equal, increase the size of the prison population. Such a change should reduce AIDS infections among women as current high-risk inmates are kept out of society for a marginally longer time. This content downloaded from 169.229.139.238 on Wed, 25 Jun 2014 18:03:07 PM All use subject to JSTOR Terms and Conditions 284 The Journal of LAW & ECONOMICS Conversely, reducing incarceration rates via early releases may increase AIDS infections among the noninstitutionalized via the reverse effect.20 On the other hand, expanding incarceration rates along the extensive margin may have a short-term incapacitation effect on the disease but may elevate transmission rates in the long term. The long-term effect would occur through the exposure of a greater proportion of men to a high-risk prison environment, a factor that should elevate transmission rates among men and possibly from men to women postrelease. We expect the consequences for community HIV infection risk of releasing one inmate early to differ from that of contracting the scope of incarceration to exclude one additional inmate. In particular, the early release may be of an inmate who has already experienced an elevated risk of acquiring HIV due to imprisonment, whereas reductions in the scope of incarceration may expose fewer men to transmission risk behind bars. On the other hand, the “new” inmate is likely to have faced a higher probability (relative to the general population) of acquiring and transmitting HIV as a result of risky behaviors prior to imprisonment. Whether the stock of prisoners matters more than the flow rates in and out of prison for HIV risk in the short-run and longer run is an empirical question. An additional factor for which we have yet to control and that some have argued helped propagate the AIDS epidemic throughout the black community is the introduction of crack cocaine. Emergency room admission statistics suggest that the use of crack cocaine in American cities began in earnest between 1984 and 1987 (Grogger and Willis 2000), the precise time when HIV infections were on the rise in African American communities. In his ethnography of the AIDS epidemic in the black community, Levenson (2004) intimates that promiscuity and unprotected sex are integrally related to the crack cocaine trade, through users trading sex for crack or sex for money to buy crack and through a psychopharmacological effect of the drug itself. Moreover, the introduction of crack cocaine has been linked to a number of negative outcomes, including homicide rates and infant mortality (see Blumstein 1995; Fryer et al. 2005). Here we make use of incarceration rates and AIDS infection rates measured at the state-year level to extend the model specification in these directions. Analysis of state-level data permits inclusion of a measure of crack cocaine prevalence as tabulated by Fryer et al. (2005) as well as inclusion of annual prison admissions and prison release flow rates in addition to overall incarceration rates (all three variables from the BJS). In addition to expanding the model specification to incorporate these additional control variables, we free up the specification to allow the incarceration effects to vary over the course of the epidemic. Finally, the state-level data do not require linear interpolation of in20 The effect on male infections is theoretically ambiguous and depends on the shape of the infection/time served hazard function. If inmates are most at risk when first entering prison, extending sentences may have little effect on infection. On the other hand, if this hazard function increases with time served, longer sentences may result in more male-to-male transmission of the disease. This content downloaded from 169.229.139.238 on Wed, 25 Jun 2014 18:03:07 PM All use subject to JSTOR Terms and Conditions Incarceration and AIDS Rates 285 Table 8 Incarceration and AIDS Infection Rates, 1982–96: State-Year Level Panel Explanatory Variable Contemporaneous year (t) Lag year t⫺1 Lag year t⫺2 Lag year t⫺3 Lag year t⫺4 Lag year t⫺5 Lag year t⫺6 Lag year t⫺7 Lag year t⫺8 Lag year t⫺9 Lag year t⫺10 Lag year t⫺11 Lag year t⫺12 Lag year t⫺13 F-test (p-value) for joint significance of lagged variables Prison Population Size Annual Prison Admissions Annual Prison Releases Crack Cocaine Index ⫺24.1524 (19.1980) ⫺9.7688 (8.4641) 2.4583 (10.1042) 12.6802 (12.7866) 20.8434 (13.3183) 27.0436* (12.8741) 31.2615* (13.2449) 33.5380* (14.9591) 33.9159* (16.8596) 32.3682⫹ (17.3950) 29.0753⫹ (15.5189) 23.8832⫹ (12.7773) 17.0909 (18.4934) 8.4094 (37.5058) ⫺.0550⫹ (.0299) ⫺.0094 (.0234) .0158 (.0270) .0232 (.0291) .0157 (.0291) ⫺.0040 (.0295) ⫺.0330 (.0330) ⫺.0684⫹ (.0395) ⫺.1075* (.0469) ⫺.1473** (.0535) ⫺.1852** (.0589) ⫺.2183** (.0652) ⫺.2439** (.0784) ⫺.2590* (.1051) .0508 (.0314) .0026 (.0258) ⫺.0208 (.0301) ⫺.0235 (.0317) ⫺.0092 (.0299) .0182 (.0280) .0547⫹ (.0300) .0964** (.0369) .1394** (.0459) .1796** (.0544) .2132** (.0616) .2364** (.0692) .2452** (.0822) .2360* (.1078) 2.3093** (.5209) 1.8291** (.2241) 1.4767** (.2832) 1.2160** (.3400) 1.0108** (.3153) .8248** (.2459) .6219** (.2053) .3659 (.2513) .0205 (.3311) ⫺.4504 (.3988) ⫺1.0831* (.4860) ⫺1.9137** (.7073) ⫺2.9785* (1.1679) ⫺4.3136* (1.9090) 29.75 (.0082) 22.61 (.0669) 129.34 (.0000) 30.16 (.0072) Note. The dependent variable is AIDSst from any source. The coefficients of the linear trend in the effects of the prison variables are suppressed. The regression is weighted by population size and includes state and year fixed effects. Bootstrapped standard errors are in parentheses unless otherwise indicated. N p 765. ⫹ Significant at the 10% level. * Significant at the 5% level. ** Significant at the 1% level. carceration rates for noncensus years and permit inclusion of all AIDS cases in the dependent variable (that is, we do not lose the roughly 15 percent of cases because of confidentiality restrictions).21 Table 8 presents the results for this model. For each of the key explanatory 21 The AIDS PIDS provides total counts by state for overall AIDS cases, by gender, and by race. The data do not include counts by race interacted with gender. This content downloaded from 169.229.139.238 on Wed, 25 Jun 2014 18:03:07 PM All use subject to JSTOR Terms and Conditions 286 The Journal of LAW & ECONOMICS variables, the table includes contemporaneous measures and 13 lags. Each lag function is constrained by a third-order polynomial, and the three sets of prison variables are all interacted with a linear time trend. The coefficients for the variables pertain to the lag function as of 1996. Beginning with the results for the prison incarceration rates, we find a pattern very similar to that from our results using the sex-market-based panel. There is no contemporaneous effect of incarceration and significant effects after lag five that remain significant through lag 11 and peak at 8 years. The results for prison admissions and releases suggest that, holding the scope of incarceration constant, releasing an additional offender results in more AIDS infections 6–13 years later, while admitting an additional offender reduces AIDS infections 7–13 years later. In other words, while expanding the scope of incarceration increases AIDS infections, we also find evidence that incarceration incapacitates, holding the incarceration rate constant. We do find several significant coefficients for crack cocaine usage. However, the pattern of the lag function is not consistent with the incubation distribution of the disease. There is a positive and significant contemporaneous effect of the crack index and significant positive effects for the first through sixth lags. However, we also find negative and significant effects of the 10th through 13th lag. This pattern casts some doubt on the hypothesis that crack cocaine explains the rise of AIDS infections among minorities in the United States. Nonetheless, our principal results remain qualitatively unchanged with or without the controls for crack cocaine prevalence. The effect sizes and dynamic structure of the impact of prison releases, prison admissions, and overall incarceration rates on AIDS infection rates is robust to the inclusion or exclusion of the indices of crack cocaine prevalence. 8. Conclusion The findings of this study are several. We demonstrate a strong positive correlation between increases in incarceration rates occurring among men within narrowly defined demographic groups and corresponding increases in the incidence of new AIDS infections among both men and women. This relationship survives detailed controls for sexual relationship market fixed effects; overall national time trends; time trends that are specific to age, racial, and state groups; and controls for education. The estimated dynamic relationship between male incarceration and AIDS infections resembles estimates of the probability distribution of the incubation period between seroconversion and the onset of symptomatic AIDS. Moreover, given the sizable racial differentials in incarceration rates at the beginning of the AIDS epidemic and the increases in these differentials thereafter, our model estimates suggest that the lion’s share of the racial differentials in AIDS infections rates for both men and women are attributable to racial differences in incarceration trends. While we have focused explicitly on the transmission of HIV/AIDS, both the theoretical story being told here and the empirical analysis can easily be extended This content downloaded from 169.229.139.238 on Wed, 25 Jun 2014 18:03:07 PM All use subject to JSTOR Terms and Conditions Incarceration and AIDS Rates 287 to other communicable diseases that have high prevalence among prisoners. For example, we have cited existing evidence of higher than usual interpersonal transmission of the hepatitis B and hepatitis C viruses as well as tuberculosis among inmates. Given the large numbers of individuals cycling through corrections systems in the United States, the more general issue of how prison is impacting the transmission of communicable diseases broadly defined is clearly an issue in need of further research and attention from policy makers. What do these results imply for national- and state-level policy debates regarding the optimal level of incarceration? Existing research clearly documents the benefits of prison in terms of crime reduction that extend beyond society’s desire to punish those who transgress the law. However, imprisonment is costly, and some of the costs come in the form of unintended consequences. To assess whether we are at, below, or beyond the optimal level of incarceration, one would need to put a monetary value on the benefits to society in terms of the crime reduction of incarcerating the last offender and compare these benefits with the costs. Donohue (2005) estimates that we are currently incarcerating people at a rate beyond the point at which the benefits exceed the costs. On the basis of an annual per-inmate cost of $46,000 per year, Donohue argues that the optimal incarceration level is roughly 300,000 persons less than the current level. The findings of our study suggest that there are additional costs to society of imprisonment that extend beyond the per-inmate per-year costs of incarceration. These additional costs include the additional medical expenditure for postrelease treatment of offenders and the treatment of others who are infected as a result of incarceration as well as the loss of health and happiness among those affected. While it is difficult to place a monetary value on these factors, they certainly add to the overall costs of incarceration, and their incorporation into cost-benefit accounting would certainly lower the optimal incarceration point even further than that estimated by Donohue. Our results suggest that there are large and important unintended health consequences for former offenders and for nonincarcerated members of the communities that disproportionately send young men into the state and federal prison systems. A comprehensive assessment of criminal justice policy in the United States should clearly be taking these considerations into account. Appendix Data To estimate the model, we construct a panel data set covering the period 1982–96 that measures the rate of late-stage HIV infection22 for subpopulations of the United States as well as a host of own-gender and cross-gender incarceration rates. The dimensions of the panel are defined by the interactions among the year of diagnosis, the state of residence at the time of diagnosis, age group, 22 Late-stage HIV is commonly referred to as full-blown AIDS. This content downloaded from 169.229.139.238 on Wed, 25 Jun 2014 18:03:07 PM All use subject to JSTOR Terms and Conditions 288 The Journal of LAW & ECONOMICS racial/ethnic group, and gender. We calculate AIDS infection rates using data from the 2001 CDC AIDS PIDS as well as the 1980, 1990, and 2000 5-Percent PUMS from the U.S. Census of Population and Housing. We calculate incarceration rates using the census data. In this section, we discuss the construction of these variables and the details of our panel data set. A1. Calculating the AIDS Infection Rate The AIDS PIDS provides case-level information on all known AIDS cases measured by the national AIDS surveillance system. Since 1985, all states require that health service providers report diagnosed AIDS cases to state and local health departments. In turn, these departments voluntarily report the details of such cases to the CDC.23 Since the onset of the AIDS epidemic, the definition of a case has changed several times. Prior to the ability to identify the HIV antibody, AIDS cases were defined by the presence of a disease indicative of a suppressed immune system, such as pneumocystis carinii pneumonia, Kaposi’s sarcoma, and other opportunistic infections. The definition was changed in 1985, reflecting the discovery of HIV as a causative agent of AIDS. The 1985 change added additional medical conditions and the restriction to those with HIV infections. The number of admissible conditions for an AIDS diagnosis was expanded again in 1987. Finally, the definition of AIDS was expanded once again in 1993 to reflect more generally those with HIV infections and measurably suppressed immune systems. The redefinition also expanded the number of medical conditions that would lead to an AIDS diagnosis for an HIV-positive individual. The three redefinitions of an AIDS case increased the likelihood of an AIDS diagnosis independent of actual prevalence. The CDC reports that the 1985 redefinition added 3–4 percent to the total annual new diagnoses, while the 1987 change augmented cases by nearly 25 percent. Similarly, the expanded definition based on a gauge of a suppressed immune system caused a discrete change in reported cases. Moreover, there is evidence that the redefinitions had larger effects on reporting for racial and ethnic minorities and on AIDS cases that were not contracted through men having sex with men. To control for the effects of these case-reporting redefinitions and any other common temporal changes, we include complete controls for year of diagnosis as well as complete sets of race-specific, age-specific, and region-specific time effects. Using the AIDS PIDS database, we first tabulate the total number of newly diagnosed AIDS cases by the state of residence, race, age, gender, and year of diagnosis for individuals with advanced-stage HIV. We then use data from the 1980, 1990, and 2000 U.S. Census PUMS to estimate the national population corresponding to each state # race # age # gender # year cell. For census 23 Evaluation studies have estimated the reporting of AIDS cases to be more than 85 percent complete, with the level of reporting completeness varying by geographic area. For a complete discussion, see Rosenblum et al. (1992). This content downloaded from 169.229.139.238 on Wed, 25 Jun 2014 18:03:07 PM All use subject to JSTOR Terms and Conditions Incarceration and AIDS Rates 289 years, we directly calculate the population with the sample data by summing the provided sample weights within cells. For intercensus years, we linearly interpolate the population using the population estimates for the 2 census years bracketing the year in question. We then tabulate an AIDS diagnosis rate expressed per 100,000 individuals. This variable is the principal dependent variable of our analysis. An individual’s race/ethnicity is defined by four mutually exclusive categories: non-Hispanic white, non-Hispanic black, non-Hispanic Asian, and Hispanic. We use nine of the 10 age groupings to characterize new diagnoses in the AIDS PIDS data, effectively limiting the analysis to AIDS cases among individuals between 20 and 65 years of age. The introduction and widespread use of medical therapies, particularly medical advances introduced since 1996, have slowed the HIV progression to AIDS and therefore may have altered or elongated the lagged structure of the relationship between incarceration and AIDS incidence. In light of this fact, our analysis focuses on the period from 1982 to 1996. One problem with the AIDS PIDS data concerns the ability to identify the state of residence at the time of diagnosis. Because of confidentiality restrictions due to small cell size within some dimensions of our panel, roughly 15 percent of AIDS cases observed over this period lack state identifiers. For the levels of disaggregation of AIDS cases required by our analysis, PIDS identified the metropolitan area of residence for those individuals residing in large metropolitan areas. This accounts for 85 percent of documented AIDS cases and includes AIDS cases for 38 states plus Washington, D.C. For the remaining 15 percent of documented AIDS cases, the only geographic identifier is the region of residence (defined as West, South, Midwest, and Northeast). Thus, the infection rates in our panel data set are estimated using only 85 percent of the total number of AIDS cases recorded in the United States. To make use of all cases, we also estimated the models using the four-category region of residence to define geographic locations rather than state of residence. The results are qualitatively and numerically similar to what we present below and are available from the authors on request. Given that the panel spans 15 years (1982–96) and covers 38 states plus Washington, D.C., the dimensions of the panel define 21,060 individual demographic groups for each gender. A2. Calculating Incarceration Rates from the Public Use Microdata Samples Estimating equation (1) requires data on current and lagged incarceration rates for both men and women. Here we first describe how we estimate incarceration rates with data from the U.S. Census. We then describe the lagged structure of our panel data set and the manner in which we calculated the lagged incarceration rates. To estimate the proportion incarcerated for each subgroup of our panel, we make use of the group-quarters identifier included in the PUMS data. The This content downloaded from 169.229.139.238 on Wed, 25 Jun 2014 18:03:07 PM All use subject to JSTOR Terms and Conditions 290 The Journal of LAW & ECONOMICS decennial census enumerates both the institutionalized and the noninstitutionalized population. The PUMS data for each census include a flag for the institutionalized as well as microlevel information on age, education, race, and all other information available for other noninstitutionalized long-form respondents. The group-quarters variable allows us to identify those individuals residing in nonmilitary institutions, a category that includes inmates of federal and state prisons, local jail inmates, residents of inpatient mental hospitals, and residents of other nonaged institutions. We use this variable as our principal indicator of incarceration.24 Raphael (2005) presents a comparison of incarceration estimates from the census with those tabulated by the BJS using alternative data sources and shows that the institutionalized in the decennial census provide a good proxy for the incarcerated population. For the census years 1980, 1990, and 2000, we measure the contemporary incarceration rate for each demographic group defined by state of residence, age group, race/ethnic group, and gender as the proportion of the members of the demographic cell that is institutionalized. For noncensus years, we linearly interpolate the incarceration rate using the estimated rates for the 2 years bracketing the year in question. Our model requires that we estimate lagged incarceration rates for each demographic group defined by our panel data set. We assume that the AIDs epidemic begins in 1980 and allow for up to 13 lags of the incarceration rate.25 We calculate the lagged incarceration rates in the following manner. First, we redefine the age groupings of our panel to reflect the effect of a time lag. For example, for black women 30–34 in New Jersey who are infected in 1990, the 1-year lagged incarceration rate should correspond to New Jersey black women who are 29–33 in 1989, the 2-year lagged incarceration rate should correspond to New Jersey black women who are 28–32 in 1988, and so on. Given that the maximum number of lags in our panel is 13 years, we must adjust the age structure 13 times. Next, for each of these 13 additional age structures interacted with the other dimensions of our panel, we estimate the contemporary incarceration rate for each year from 1980 to 2000 using PUMS. This essentially creates 13 ancillary panel data sets using 13 alternative age groupings. Finally, we match observations from our original panel to the corresponding observations from each of the 13 ancillary panels that gauge the appropriate time lags. For example, using the ancillary panel in which the age structure is lagged 1 year, the 1995 incarceration rates provide the 1-year lag for 1996, the 1994 incarceration rate provides the 1-year lag for 1995, and so on. Using the ancillary panel in which the age structure is lagged 2 years, the 1994 observations 24 See Butcher and Piehl (1998) for an analysis of incarceration among immigrant men that also uses the group-quarter variable to identify the incarcerated. 25 Recall from our methodological discussion above that, for any year where lags 1–13 occur prior to 1980, we constrain the coefficient on that lag for that year to zero. This content downloaded from 169.229.139.238 on Wed, 25 Jun 2014 18:03:07 PM All use subject to JSTOR Terms and Conditions Incarceration and AIDS Rates 291 provide the 2-year lag for 1996, the 1993 observation provides the 2-year lag for 1995, and so on. Each observation in our final data set is matched to 13 lags of the own-gender incarceration rate, for which observations with infection years between 1982 and 1992 will have missing values for lags that date prior to 1980. In addition, each observation is also matched by year of infection, state of residence, race/ethnicity, and age to the contemporary and lagged incarceration rates for individuals of the opposite gender. References Adimora, Adaora A., and Victor J. Schoenback. 2005. Social Context, Sexual Networks, and Racial Disparities in Rates of Sexually Transmitted Infections. Journal of Infectious Diseases 191:S115–S122. Andrews, Donald W.K., and Ray C. Fair. 1992. Estimation of Distributed Lags and Leads with End Point Constraints. Journal of Econometrics 53:123–39. Bacchetti, Peter. 1990. Estimating the Incubation Period of AIDS by Comparing Population Infection and Diagnosis Patterns. Journal of the American Statistical Association 85:1002–8. Beck, Allen J., and Timothy A. Hughes. 2005. Sexual Violence Reported by Correctional Authorities, 2004. Bureau of Justice Statistics Special Report No. NCJ 210333. Washington, D.C.: U.S. Department of Justice. http://www.ojp.usdoj.gov/bjs/pub/pdf/ svrca04.pdf. Blumstein, Alfred. 1995. Youth Violence, Guns, and the Illicit Drug Industry. Journal of Criminal Law and Criminology 86:10–36. Brewer, T. Fordham, David Vlahov, Ellen Taylor, Drusilla Hall, Alavaro Munoz, and B. Frank Polk. 1988. Transmission of HIV-1 within a Statewide Prison System. AIDS 2: 363–67. Brookmeyer, Ron. 1991. Reconstruction and Future Trends of the AIDS Epidemic in the United States. Science, July 5, pp. 37–42. Butcher, Kristin F., and Anne M. Piehl. 1998. Recent Immigrants: Unexpected Implications for Crime and Incarceration. Industrial and Labor Relations Review 51:654–79. Castro, K. G., L. J. Conley, R. Shansky, V. Scardino, T. Green, J. Narkunas, et al. 1994. Report of a Study of HIV Infection in Selected Illinois Correctional Facilities. Unpublished manuscript. Abt Associates, Cambridge, Mass. Charles, Kerwin K., and Ming Ching Luoh. 2005. Male Incarceration, the Marriage Market and Female Outcomes. Working paper. University of Michigan, Department of Economics, Ann Arbor. Donohue, John J. 2005. Fighting Crime: An Economist’s View. Milken Institute Review (1st quarter):46–58. Francis, Andrew M. 2006. The Economics of Sexuality: The Effects of HIV/AIDS on Homosexual Behavior, Desire, and Identity in the United States. Unpublished manuscript. University of Chicago, Department of Economics. Fryer, Roland G., Paul S. Heaton, Steven D. Levitt, and Kevin M. Murphy. 2005. Measuring the Impact of Crack Cocaine. Working Paper No. 11318. National Bureau of Economic Research, Cambridge, Mass. This content downloaded from 169.229.139.238 on Wed, 25 Jun 2014 18:03:07 PM All use subject to JSTOR Terms and Conditions 292 The Journal of LAW & ECONOMICS Grogger, Jeffrey, and Michael Willis. 2000. The Emergence of Crack Cocaine and the Rise in Urban Crime Rates. Review of Economics and Statistics 82:519–29. Hammett, Theodore M., Mary Patricia Harmon, and William Rhodes. 2002. The Burden of Infectious Disease among Inmates of and Releasees from US Correctional Facilities, 1997. American Journal of Public Health 92:1789–94. Horsburgh, L. Robert, Jr., Joseph Q. Jarvis, Trudy McCarthur, Terri Ignacio, and Patricia Stock. 1990. Seroconversion to Human Immunodeficiency Virus in Prison Inmates. American Journal of Public Health 80:209–10. Jaquez, John A., James S. Koopman, Carl P. Simon, and Ira M. Longini, Jr. 1994. The Role of the Primary Infection in the Epidemics of HIV Infection in Gay Cohorts. Journal of Acquired Immune Deficiency Syndromes 7:1169–84. Johnson, Rucker C., and Steven Raphael. 2006. The Effects of Male Incarceration Dynamics on AIDS Infection Rates among African-American Women and Men. Working Paper No. 06–22. University of Michgan, National Poverty Center, Ann Arbor. Kilmarx, Paul. H., Akbar A. Zaidi, James C. Thomas, Allyn K. Nakashima, Michael E. St. Louis, Melinda L. Flock, and Thomas A. Peterman. 1997. Ecological Analysis of Sociodemographic Factors and the Variation in Syphilis Rates among Counties in the United States, 1984 to 1993. American Journal of Public Health 87:1937–43. Krebs, Christopher P., and Melanie Simmons. 2002. Intraprison HIV Transmission: An Assessment of Whether It Occurs, How It Occurs, and Who Is at Risk. AIDS Education and Prevention, 14(suppl. B):53–64. Laumann, Edward O., John H. Gagnon, Robert T. Michael, and Stuart Michaels. 1994. The Social Organization of Sexuality: Sexual Practices in the United States. Chicago: University of Chicago Press. Levenson, Jacob. 2004. The Secret Epidemic: The Story of AIDS and Black America. New York: Anchor Books. Macalino, Grace R., David Vlahov, Stephanie Sanford-Colby, Sarju Patel, Keith Sabin, Kristopher Salas, and Josiah D. Rich. 2004. Prevalence and Incidence of HIV, Hepatitis B Virus, and Hepatitis C Virus Infections among Males in Rhode Island Prisons. American Journal of Public Health 94:1218–23. Morris, Martina. 1997. Sexual Networks and HIV. AIDS 11:S209–S216. Mutter, Randal C., Richard M. Grimes, and Darwin Labarthe. 1994. Evidence of Intraprison Spread of HIV Infection. Archives of Internal Medicine 154:793–95. Pakes, Ariel, and Zvi Griliches. 1984. Estimating Distributed Lags in Short Panels with an Application to the Specification of Depreciation Patterns and Capital Stock Constructs. Review of Economic Studies 51:243–62. Raphael, Steven. 2005. The Socioeconomic Status of Black Males: The Increasing Importance of Incarceration. In: Alan J. Auerbach, David Card, and John M. Quigley, eds. Poverty, the Distribution of Income, and Public Policy. New York: Russell Sage Foundation. Raphael, Steven, and Michael A. Stoll. 2004. The Effect of Prison Releases on Regional Crime Rates. Pp. 207–55 in The Brookings-Wharton Papers on Urban Economic Affairs, edited by William G. Gale and Janet Rothenberg-Pack. Vol. 5. Washington, D.C.: Brookings Institution. Rosenblum, Lisa, James W. Buehler, Meade W. Morgan, Samuel Costa, Julia Hildalgo, Richard Holmes, Loren Lieb, Anne Shields, and Bruce Whyte. 1992. The Completeness of AIDS Case Reporting, 1988: A Multisite Collaborative Surveillance Project. American Journal of Public Health 82:1495–99. This content downloaded from 169.229.139.238 on Wed, 25 Jun 2014 18:03:07 PM All use subject to JSTOR Terms and Conditions Incarceration and AIDS Rates 293 Sampson, R. J. 1995. Unemployment and Imbalanced Sex Ratios: Race-Specific Consequences for Family Structure and Crime. Pp. 229–60 in The Decline in Marriage among African-Americans: Causes, Consequences, and Policy Implications, edited by M. Belinda Tucker and Claudia Mitchell-Kern. New York: Russell Sage Foundation. Tewksbury, Richard. 1989. Measures of Sexual Behavior in an Ohio Prison. Sociology and Social Research 74:34–39. Thomas, James C., and Mary E. Gaffield. 2003. Social Structure, Race, and Gonorrhea Rates in the Southeastern United States. Ethnicity and Disease 13:362–68. Thomas, Philip A., and Martin Moerings, eds. 1994. AIDS in Prison. Brookfield, Vt.: Dartmouth. U.S. Department of Justice. 1993. Update 1992: HIV/AIDS in Correctional Facilities. Washington, D.C.: National Insitute of Justice. U.K. Register of HIV Seroconverters Steering Committee. 1998. The AIDS Incubation Period in the U.K. Estimates from a National Register of HIV Seroconverters. AIDS 12:659–67. Wooden, Wayne S., and Jay Parker. 1982. Men behind Bars: Sexual Exploitation in Prison. New York: Plenum. This content downloaded from 169.229.139.238 on Wed, 25 Jun 2014 18:03:07 PM All use subject to JSTOR Terms and Conditions