Does Being Left Behind in Childhood Lead to Criminality in Adulthood - Evidence From Data on Rural-Urban Migrants and Prison Inmates in China
Download original document:
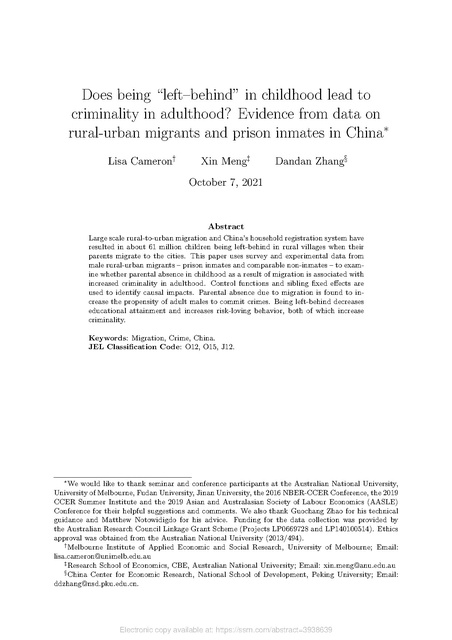
Document text
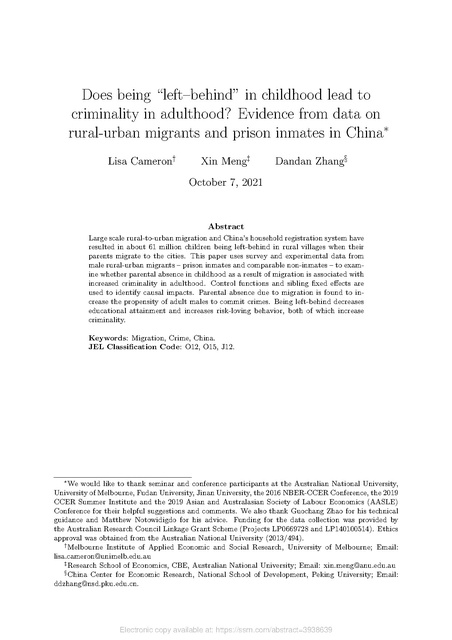
Document text
This text is machine-read, and may contain errors. Check the original document to verify accuracy.
Does being “left–behind” in childhood lead to criminality in adulthood? Evidence from data on rural-urban migrants and prison inmates in China∗ Lisa Cameron† Xin Meng‡ Dandan Zhang§ October 7, 2021 Abstract Large scale rural-to-urban migration and China’s household registration system have resulted in about 61 million children being left-behind in rural villages when their parents migrate to the cities. This paper uses survey and experimental data from male rural-urban migrants – prison inmates and comparable non-inmates – to examine whether parental absence in childhood as a result of migration is associated with increased criminality in adulthood. Control functions and sibling fixed effects are used to identify causal impacts. Parental absence due to migration is found to increase the propensity of adult males to commit crimes. Being left-behind decreases educational attainment and increases risk-loving behavior, both of which increase criminality. Keywords: Migration, Crime, China. JEL Classification Code: O12, O15, J12. ∗ We would like to thank seminar and conference participants at the Australian National University, University of Melbourne, Fudan University, Jinan University, the 2016 NBER-CCER Conference, the 2019 CCER Summer Institute and the 2019 Asian and Australasian Society of Labour Economics (AASLE) Conference for their helpful suggestions and comments. We also thank Guochang Zhao for his technical guidance and Matthew Notowidigdo for his advice. Funding for the data collection was provided by the Australian Research Council Linkage Grant Scheme (Projects LP0669728 and LP140100514). Ethics approval was obtained from the Australian National University (2013/494). † Melbourne Institute of Applied Economic and Social Research, University of Melbourne; Email: lisa.cameron@unimelb.edu.au ‡ Research School of Economics, CBE, Australian National University; Email: xin.meng@anu.edu.au § China Center for Economic Research, National School of Development, Peking University; Email: ddzhang@nsd.pku.edu.cn. Electronic copy available at: https://ssrn.com/abstract=3938639 1 Introduction The unprecedented economic growth in China in the past few decades has led to large scale rural-urban migration. Between 1997 and 2019 the number of rural workers working in Chinese cities increased from 38 to 174 million (World Bank, 2009; NBS, 2020). Institutional restrictions on rural workers’ access to social services and social welfare in cities - China’s household registration system (hukou) – has resulted in a large proportion of migrants leaving their families, especially their children, behind in rural areas and coming to cities alone to work, Meng and Yamauchi (2017). This has induced dramatic changes in family structure in rural China. A large cohort of migrant children have been leftbehind in rural villages where they are often looked after by one parent, grandparents, or other relatives, with a small proportion live in boarding schools. According to the 2010 Population Census, there were 61 million left-behind children, accounting for 38% of all rural children and 22% of all children nationwide. There are yet another 9 million children left–behind in one city by parents working in another. This makes 70 million in total, almost equal to the total number of children in the United States (All-Women Federation in China, 2013; Economist, 2015). This phenomenon of “Left-behind Children” in China has given rise to concerns about the potential consequences for society as parents play an important role in guiding their children’s development and modelling socially-acceptable behavior, (Maccoby and Martin, 1983; Zinn et al., 2016; Wright and Wright, 1993; Bornstein, 2002; McLanahan and Percheski, 2008). Family structure is thought to affect the propensity for adolescent delinquency (which is associated with criminality in adulthood) via its effects on parental control, supervision and the emotional attachment of child to parent, (Nye, 1958; Van Voorhis et al., 1988)). Nye (1958)’s social control theory maintains that children’s delinquency is influenced through direct control of behavior through parental supervision, restrictions and punishment; internalized control through the influence on the child’s conscience; and indirect control through the affectionate attachment between the child and parent. Hirschi (1969)’s social bond theory argues that the bond of attachment (Nye’s indirect and internalized controls) is likely the most important family factor in determining delinquency, with empirical support, (for example, see Demuth and Brown, 2004). All of these forms of parental influence and control are potentially disrupted for China’s “left-behind children” as parental migration results in the parent being physically absent, which not only limits his or her ability to supervise, monitor and punish, but also may lead to emotional absence and weakened attachment. Parental absence could also lead to the child feeling rejected which has been shown to be one of the most significant predictors of delinquency, Wright and Wright (1993). Empirical research on the impacts of family dissolution, mostly in developed countries, has found parental divorce or separation to be associated with negative outcomes in terms 1 Electronic copy available at: https://ssrn.com/abstract=3938639 of children’s education and health, behavioral problems, delinquency, and crime (See, for example, Geismar and Wood, 1986; Brady et al., 1986; Wright and Wright, 1993; McLanahan and Sandefur, 1994; Amato and Keith, 1991; Demuth and Brown, 2004). These associations have been corroborated by studies that seek to identify causal impacts. Manski et al. (1992) generate a range of estimates of the effect of family structure on high school graduation obtained under differing assumptions about the process generating these variables. Their results strengthen the evidence that living in an intact family increases the probability of high school graduation. Gruber (2004) exploits variation across states and over time in changes in divorce regulations in the US and 40 years of census data to show that adults who were exposed to unilateral divorce laws as children experienced higher rates of parental divorce, were less well educated, had lower family incomes, married earlier but separated more often, and were at higher risk of suicide. Grogger and Ronan (1995) and Sandefur et al. (1997) use sibling fixed effects estimation which identifies the impact of fatherlessness off a comparison of siblings with different exposure to it due to their different ages. They find that each additional year of fatherlessness in the US reduces children’s educational attainment and entry wages when they grow up.1 Almost all of the literature on the impact of parents leaving children behind in China has focused on the impacts in childhood. Zhang et al. (2014) finds that parental absence, especially of both parents, reduces children’s cognitive achievements. Meng and Yamauchi (2017) found a sizeable adverse impact of exposure to being left-behind due to parental migration on children’s health and education outcomes. Shi et al. (2016) and Zhao et al. (2017) found that parental out-migration has a significant negative impact on the mental health and psychosocial well-being of “left-behind” children, as they tended to exhibit higher levels of anxiety and lower levels of self-esteem, emotional difficulties and difficulties in conduct, peer relationships and pro-social behaviors. Wang (2019) reveals that being left-behind reduces children’s probability of being enrolled at school, while Hong and Fuller (2019) identified adverse impacts on children’s educational aspirations. A few empirical attempts have also been made to link parents’ out-migration to children’s wellbeing indicated by education and health attainments in other developing countries. For example, Yang (2008), in the context of the Philippines, and Hanson and Woodruff (2003), in the context of Mexico, found that out-migration could generate a “positive income effect” which might mitigate the “negative impact” arising from the lack of parental care. A recent Lancet study combines data from many developing countries to conduct a meta-analysis of the impact of parental migration on left-behind children’s 1 Lang and Zagorsky (2001), however, find that the impact of the number of years lived with one’s biological mother and father before age 18 is less strongly associated with some outcomes after controlling for a suite of family background variables. Using parental death as a “natural experiment” they found little evidence that parental absence during childhood affects economic wellbeing in adulthood. Finlay and Neumark (2010) used an instrumental variables approach to quantify the effect of never-married motherhood (i.e., paternal absence) on high school dropout rates of black and Hispanic children and found no negative effect. 2 Electronic copy available at: https://ssrn.com/abstract=3938639 health outcomes. The study concludes that left-behind children and adolescents have substantial unmet mental health and nutritional needs and as labour migration increases globally, many children are at risk (Fellmeth et al., 2018).2 Few studies, if any, have addressed long-term effects of being left-behind during childhood by parents who migrated. It is, however, well-established that childhood experiences often have life-long impacts. Even “relatively mild shocks in early life can have substantial negative impacts”, Almond et al. (2018). The large numbers of “left-behind” children in China who were born in the 1990s and early 2000s have now grown up and have started migrating to cities themselves. They now account for a sizeable share of the Chinese urban labor force.3 The parental absence they experienced during their childhoods not only potentially affects their own wellbeing and human capital accumulation, but also the Chinese economy and society. Policy makers have been particularly concerned as to whether, and how, the experience of being “left-behind” in childhood affects adult social and economic outcomes. In the same period that many left-behind children are reaching adulthood, crime rates in China are increasing dramatically - from 7.4 per 10,000 in 1982 to 47.8 per 10,000 in 2014 (Zhang et al. (2011b) and Law Yearbook of China 2010-2015). Previous studies have highlighted several factors that could contribute to increased crime rates, including large scale rural-urban migration with a high proportion of migrants being crime-prone young males (Zhang et al., 2014), significant increases in the sex ratio (ratio of males to females) due to the introduction of the One Child Policy which generated fierce marriage market competition and the need for financial advancement (Edlund et al., 2013; Cameron et al., 2019) and significant increases in income inequality (Zhang et al., 2011a). In this paper we investigate another potential contributing factor to the increase in crime: being “left-behind” in childhood and its effects on subsequent adult criminal behavior. Despite media attention on a possible link between being left-behind and increases in crime rates, we are aware of no studies which have investigated such a link. This is largely due to a lack of data. In 2013 we implemented a survey and conducted economic experiments with inmates in a prison for male offenders in Shenzhen, China. Over 85% of the inmates in the prison were rural-to-urban migrants. In the same year we conducted the same experiments and survey with a randomly selected sample of non-inmate rural-urban migrants in Shenzhen. Using these unique survey and experimental data on rural-urban migrants this paper examines whether parental absence in childhood (prior to age 16) due to parents migrating from rural areas to urban areas for jobs is associated with increased criminality in adulthood. We examine the extent to which educational at2 The study combines data from 111 studies, 91 of which were studies of left-behind children in China. The remainder include studies of Thailand, the Philippines, Indonesia, India, Mexico, Guatemala, Peru, Ethiopia, Kenya, Malawi, Trinidad, Tobago, Jamaica, Romania, and Moldova. 3 The 2014 Rural-Urban Migration in China (RUMiC) survey shows that 18% of those in the labor market (who were born since 1990) were left-behind in childhood due to parental migration. 3 Electronic copy available at: https://ssrn.com/abstract=3938639 tainment, behavioral preferences and personality traits are affected by being left-behind and drive increases in crime. The behavioral preferences we focus on are risk-attitudes and time preferences as these are known to be associated with criminality (Wood et al., 1993). Risk-taking may be affected by parental absence as the presence of parents has been observed to buffer adolescents from the risk-taking influence of their peers, see van Hoorn et al. (2018). Parental presence has similarly been associated with more future-oriented time preferences, Lersch and Baxter (2021). Unlike much of the previous research which compares left-behind children in rural areas with rural children whose parents did not migrate, e.g. Zhang et al. (2014),Meng and Yamauchi (2017) and Shi et al. (2016), we compare the outcomes for left-behind individuals with an additional comparison group - children who migrated to cities with their parents. Our main focus is on this latter comparison as our aim is to inform government policy which currently actively discourages parents from migrating with their children. To do so we need to compare the outcomes of children who did and did not migrate with their parents. The potential endogeneity of being left-behind and the migration decision are addressed using a control function approach. This involves two-stage estimation. The first stage models the endogenous variables. The (generalized) residuals from the first stage (the control functions) capture the endogenous component of these variables and are included in the second stage regressions which renders the endogenous variables appropriately exogenous, Wooldridge (2015). We use control functions rather than the more oft-used instrumental variables (IV) estimation as IV estimation produces biased estimates in models such as ours with non-linear endogenous explanatory variables (the binary variables reflecting whether one’s parents migrated and whether one was left-behind). We also estimate sibling fixed effects models. Our results show that being left behind in childhood by one or both rural-urban migrant parents increases the propensity of males to commit crimes in adulthood. Being ‘left-behind’ results in lower educational attainment and also in more risk-loving preferences. Lower educational attainment and risk-loving behavior are both significant determinants of being in prison in adulthood. Together these factors account for over half of the total impact of being left-behind on criminality, with education accounting for the lion’s share. The paper is organised as follows. Our data sources are discussed in Section 2. Empirical methods are presented in Section 3, which is followed by the discussion of the main results and an investigation of the channels through which parental absence affects left-behind children’s outcomes in Section 4. Concluding remarks and policy implications are presented in Section 5. 4 Electronic copy available at: https://ssrn.com/abstract=3938639 2 Data In 2013 we conducted two sets of surveys and experiments - one with a random sample of the male rural-urban migrant population in Shenzhen, and one with inmates in a male prison in the same city. Shenzhen is at the heart of China’s manufacturing boom and has been a magnet for migrant workers since the early 1990s. In 2013 50% of Shenzhen’s population were rural-urban migrants, while in the city’s male prison, 85% of its inmates were rural-urban migrants. The data set used in this study is obtained by combining these two sources of information. We surveyed a random sample of 959 rural-urban migrants in the prison. Of these, 735 prisoners were randomly chosen to participate in experimental sessions.4 Our non-inmate sample consists of 299 male rural-urban migrant workers in Shenzhen. We oversampled inmates as they constitute only a very small percentage of the population and account for this in our estimation strategy, as is discussed in detail below.5 The non-inmate sample was constructed using the sampling frame of a representative sample of rural-urban migrants - the 2012 wave of the Rural-Urban Migration in China (RUMiC) survey. The prison takes inmates arrested in both Shenzhen and the neighboring city of Dongguan so our migrant sample is selected to be representative (in terms of age, education and industry of employment) of the male migrant population in these two cities, weighted by the relative size of the migrant populations in Shenzhen and Dongguan. From this combined sample of 1034 individuals, we exclude observations with missing values for key variables (28 observations) and, in order to focus on the phenomenon of being left-behind due to parental migration, 38 individuals who experienced parental absence due to other reasons (e.g. divorce, death of a parent). Our final analysis sample size is 968, comprised of 678 inmate migrants and 290 non-inmate migrants. 2.1 The Experiments Both the inmate and non-inmate samples participated in a series of economics experiments. The sessions were conducted by one of the authors of this paper (Zhang) who worked with a team of 20 student research assistants from Peking University. The experiments were all conducted in Mandarin with pen and paper. Each participant received a printed copy of the experimental instructions which were also read to the group as a whole. There were opportunities to ask questions and test questions were embedded to 4 Fewer prisoners were selected to participate in the experiment for budgetary reasons and because of the limited time we were given to access the inmate subjects. The experiments were conducted in September 2013. We first randomly selected 1200 prisoners to be survey respondents. From these we then randomly selected 1000 to participate in the experimental sessions. We then drop inmates in our sample who are not rural-urban migrants. 5 The ratio of inmate to non-inmate observations also reflects survey budget constraints and the greater cost associated with implementing the non-inmate sample. 5 Electronic copy available at: https://ssrn.com/abstract=3938639 enable us to discern whether participants understood the instructions.6 The participant information sheet and experimental instructions are included in Appendix A.1. The experimental protocols were kept as similar as possible for the inmates and non-inmates. This includes using the same script, same procedures, same researcher conducting the session, spacing between desks and prohibition on communication across participants during the session. In the prison the experiments were conducted a week before the survey. For non-inmate participants the experiments and surveys were conducted in the same session, with the experiments being conducted first. In the prison, the randomly selected inmates were told that they had been selected to participate in an experiment in which there would be the chance to earn some money. The experiments were conducted during the Thursday afternoon and Saturday free time periods in a large conference room. At the conclusion of the games participants received a deposit receipt for the money which was paid into their savings accounts.7 Migrant participants were told that there was an opportunity to take part in some activities in which they could earn some money. If they agree to participate, they were invited to go to a meeting room in a hotel in downtown Shenzhen, close to public transport, where the experiments and exit survey were conducted.8 A show-up fee of RMB100 (USD14) was paid to offset transport and opportunity costs and so to ensure that we were able to attract a representative sample of migrants.9 A total of 10 sessions were conducted with each session lasting between 2.5 and 3 hours. At the end of each session, and after the completion of the survey, the participants were paid in cash according to their experimental choices and outcomes, plus the show-up fee. The risk experiments implemented were standard multiple price list format experiments which involve a series of eleven choices between lotteries, similar to that used in Murnighan et al. (1988). Each decision involves choosing between receiving an amount with certainty or a lottery with a 50% chance of receiving a larger amount and a 50% chance of receiving nothing. The specific choices faced by the inmate participants are shown in Appendix A.2.10 The “winning” amount increases as one works one’s way 6 Participants were also read statements assuring data confidentiality and lack of identifiability and were invited to leave if they no longer felt like participating, before commencing or at any point during the sessions. They were informed that if they decided not to participate at any point, any data they provided to that point would be destroyed. Verbal consent was sought prior to commencing the experiments. 7 Each prisoner has a prison savings account in which they receive payment for any work they do while in prison, for example, working in the prison factory. These funds can be used to buy items in prison, can be transferred to people outside the prison and can be withdrawn when the inmate leaves prison. 8 The migrant sample appears to be relatively representative. For example, the distribution of place of birth in our migrant sample is very similar to the distribution in the representative sample of migrants in the RUMiC survey data. The rate of unemployment in our migrant sample (9.3%) is also very similar to that amongst rural migrants in Shenzhen and Dongguan (8.9%) in the RUMiC data. 9 We paid a show-up fee and used higher stakes for the migrant sample to reflect their higher opportunity cost of time. This is the protocol typically used when conducting games across different cultural settings so as to keep the real value of the stakes as close as possible to constant across groups, Cameron et al. (2009). 10 The choice of stakes was complicated by the fact that prisoners’ earnings in jail are very low (less than 6 Electronic copy available at: https://ssrn.com/abstract=3938639 through the choices. Relatively risk-loving individuals will prefer the lottery even if they stand to gain only a small amount, while more risk-averse individuals will switch to choosing the lottery only when the “prize” becomes sufficiently large. Participants were allowed to switch from choosing the certain sum to choosing the lottery only once. Once all participants had made their choices a participant was asked to draw a ball from a bag in which there were eleven balls numbered from 1 to 11. The chosen ball determined which choices the participants were to be paid for. Then a different participant was asked to roll a dice which determined the outcome for those who had selected a lottery.11 2.2 Survey and Administrative Data The survey collected a wide array of information including demographic information, place of birth, migration history, labour market history, criminal history, and conducted personality tests.12 In addition, a simple Raven’s test was implemented to elicit a measure of individuals’ cognitive ability. A series of unincentivized time preference questions were also asked during the survey which are used to construct our measures of patience. The respondent is asked to choose between getting a certain amount of money in a month’s time (1000 RMB or approximately USD160) and a series of higher amounts of money in seven months’ time. More patient people will choose to defer payment even for a relatively small increase in the amount received, whereas less patient people will only choose to defer payment if the 10% of that of the non-prison migrant sample) and not representative of their earnings outside prison. For this reason we chose stakes for prisoners which were lower in absolute value than those for migrants but a significantly greater percentage of their current daily earnings. The lottery choices for the inmate (non-inmate) sample involved a choice between receiving RMB45 (RMB67) with certainty and a lottery with a 50% chance of receiving nothing and a 50% chance of receiving a sum which ranged from RMB60 to RMB 210 (RMB 90 to RMB 315) The average experimental earnings received by non-inmates was RMB100 (excluding the show-up fee), while for prisoners it was RMB64. Non-inmates received on average 175% of their daily income. Prisoners received approximately 18 times their average daily earnings but only 6% of the average amount held in their prison savings account. Importantly, we have since conducted the same risk games, using the same protocols, with a sample of female prisoners in China. In these games we varied the stakes used. We find that increasing or decreasing the stakes by 50% does not affect their behavioral choices (p=0.63). Results are available on request. 11 The actual payoff method was slightly more complicated than this as each participant played four games in the following order: 1) ultimatum game; 2) risk game; 3) trust game; 4) half of the sample played a dishonesty game based on Mazar et al. (2008) and Friesen and Gangadharan (2013) and half played a compliance game based on (Friesen, 2012). Participants were aware that payments would be made for only one of these games, which was selected randomly by drawing balls with number 1 to 4 from a bag. The method described above was followed if the risk game was randomly selected for payment. We focus on risk attitudes and time preferences in this paper because, as discussed above, risk-taking and short time horizons are known to be related to criminality. Further, being left-behind and parental migration are not strong determinants of behavior in the trust and ultimatum games (see Appendix Table C4). The other games are quite different in nature and were included for another paper. 12 The same survey and experiments were conducted across the two groups, with some small changes necessitated by the different contexts. For questions about job status and living conditions we asked migrants about their current situation whereas we asked the prisoners about their situation prior to being imprisoned. These particular questions are not used in this paper. 7 Electronic copy available at: https://ssrn.com/abstract=3938639 amount received increases by a substantial amount. The choices in these time preference questions are presented in the Appendix A.3. The survey also collects information about the respondents’ parents and their siblings (birth year, gender, education, current employment, migration history, and whether he/she ever committed a crime). The prison also granted us permission to access the prisoners’ administrative records and we merge these onto the survey and experimental data. The administrative data include demographic information such as age and ethnicity and hukou (residency status). These allow us to confirm information provided directly by the inmate respondents. 2.3 Other Data We also make use of a characteristics of migrants’ sending prefectures/counties as control variables and instrumental variables in the control function estimation. At the prefecture level these include GDP per capita, Gini coefficients of individual income, the proportion of the population who come from an ethnic minority group, and the sex ratio. At the county level we have the teacher-student ratio, out-migration rate, and the proportion of children aged 0-15 who were left-behind. The latter two variables are used as instrumental variables. The majority of these variables were calculated using China’s 2000 Population Census, with the exception of per capita GDP which is from the China City Statistical Yearbooks (various years) and the county level teacher-student ratio which is from the Ministry of Education’s National Statistical Report on Education Finance: 1993-2013. 2.4 Descriptive statistics Table 1 shows what percentage of our samples were left–behind and whether they were left-behind by their mother, father or both parents. Table 2 presents summary statistics by left-behind and inmate status. Note that as our sample over-represents the prisoner population and under-represents the migrant population, summary statistics presented in both tables are weighted by the population weight.13 Table 1 shows that on average, about 16 per cent of prisoners were left-behind by one or both parents during their childhood (before the age of 16). This is about twice the rate experienced by non-inmate migrants (8.3 per cent). The predominant form of parental absence in our sample, for both prison inmates and non-inmates, is both parents having been absent. Very few respondents (0.7%) were left behind by their mother to live with their father, with only slightly more (1.75%) being left behind by their father to live with their mother.14 13 14 The data and assumptions used to generate the weight are detailed in Appendix B. These small sample sizes preclude us from separately examining the impact of being left-behind by both parents and the impact of being left behind by one parent. The point estimates obtained if one tries to do this suggest slightly smaller effects of one parent being absent than both parents being absent. 8 Electronic copy available at: https://ssrn.com/abstract=3938639 The average age of men in our sample is 29 years. Forty-three percent are married. On average they have 11 years of education. Most of our sample was born after the introduction of the One Child Policy but as they were born in rural areas where couples were allowed to have two children if the first born was a girl and where the policy was also often not strictly enforced, ninety per cent have siblings, with the majority having more than one sibling. Those who were left-behind because of parental migration are five years younger on average (reflecting the increase in migration over time), less likely to be married, more likely to be an only child and have slightly better-educated mothers and fathers. The raw data show that those who were left-behind also have higher cognitive ability, are more risk-loving and less patient, and are more likely to be in jail at the time of the survey. They are more likely to have been born in counties where a greater proportion of children were left-behind. The inmates in our sample are a similar age to their non-inmate counterparts, but are less likely to be married, have lower cognitive scores, less educated parents, less education themselves and are less likely to be ethnically Han Chinese (the predominant ethnic group in China). They are also more likely to make the most risky and least patient choices in the experimental tasks. 3 Estimation Strategy To examine whether being left-behind before age 16 because of parental migration increases individuals’ probability of committing crime and affects other adult outcomes, we estimate the following equation: Yi = α0 + α1 LBi + α2 M igiP + α3 Xi + α4 WiP + α5 SiS + δp + i , (1) where Yi is a vector of outcome variables. Our main interest is in examining the effect of being left-behind due to parental migration on the probability of being in prison, P risoneri , a dummy variable indicating whether individual i is in prison or not. In addition, we examine whether being left-behind in childhood affects behavioral preferences, personality traits, and education outcomes. LBi is a variable indicating whether the individual was left-behind when under the age of 16 due to one or both parents having migrated (in some specifications we alternatively use the number of years the child was left-behind); M igiP is a dummy variable indicating whether one or both parents migrated to a city; Xi is a vector of individual level control variables including age, cognitive test score, whether the individual is married or not, whether the individual is a single child, the number of siblings he has, and Han ethnicity; WiP is a vector of parental controls which includes mothers’ and fathers’ years of schooling and whether either parent has ever committed a crime; and SiS is a vector of sending region (s) controls, including prefecture 9 Electronic copy available at: https://ssrn.com/abstract=3938639 level log per capita GDP, Gini coefficients of individual income, the share of minority groups in the prefecture population, and the prefecture’s sex ratio. We include the sex ratio because previous studies have found that sex ratio imbalance and the consequent increase in marriage market competition is an important driving force for increases in the crime rate (see, for example, Edlund et al., 2013; Cameron et al., 2019).15 A county level control variable, the teacher-student ratio, is included to absorb differences across sending counties in investment in public education. δp are sending province fixed effects.16 Our sample is comprised of three groups of individuals: 1) those who were left-behind due to parental migration when they were under 16 years of age; 2) those whose parents migrated but were not left-behind, and 3) those whose parents did not migrate. α1 captures differences in outcomes between groups 1 and 2 and is our key parameter of interest in Equation 1. It measures the effect of being left-behind, relative to someone whose parents migrated and who was not left-behind, i.e. who migrated to the city with their parents.17 α1 + α2 captures differences between groups 1 and 3 and is also of interest as it measures the sum of the effects of being left-behind and parental migration, and so captures the impact of being left-behind relative to someone whose parents stayed living with them in the village through childhood. We focus primarily on α1 as it captures the effect of being left-behind, abstracting from the migration decision, and it is the policy lever over which the government has some control. That is, given the level of rural-urban migration – which is widely viewed as being essential to economic development (Lewis, 1954) and so is not something a government would normally wish to inhibit – α1 identifies what would be gained by making it easier for parents to bring their children to the cities. 15 We calculate the sex ratio for those aged 18 to 27 at the time of our survey (2013) as this captures the crime-prone years for men. The variable is calculated using data on those aged 5 to 14 in the 2000 population census. 16 We have elected to examine the impact of being left-behind on educational attainment, rather than including it as a control variable in the vector of individual characteristics Xi when examining the causes of criminality. We make this decision acknowledging that education is a known determinant of criminality (Lochner and Moreffi, 2004; Machin et al., 2011) but as the existing literature has identified a causal link between being left-behind and child school performance (see, for example Zhang et al., 2014; Meng and Yamauchi, 2017) take the view that it is important to treat education as a channel through which being left-behind can lead to crime. One could argue that whether a child is left-behind is potentially a function of the child’s abilities as reflected in educational status. For example, parents may be more likely to leave a more able child behind or vice-versa. If so, the omission of education could give rise to omitted variable bias. To mitigate against this we directly control for individuals’ Ravens test scores as a non-verbal measure of cognitive ability. Marital status could similarly be modelled as a mediating variable, however, it is unclear why being leftbehind would affect marriageability. Further, once one controls for education and age, any difference in marital status between those who were left-behind and those who were not is statistically insignificant. 17 The majority of the individuals in group 2 accompanied their parents to the cities, with a small number not being “left-behind” because their parents either migrated before their birth, or after they turned 16. We are unable to define this group to be exclusively those who migrated with their parents as the date of parental migration is missing for some observations. For observations which are missing the date of parental migration we only know that they report not being left-behind before the age of 16 and that their parents migrated. However, for those who reported a date of migration and were not left-behind, 72% had a parent who migrated when they were aged between 0 and 15. 10 Electronic copy available at: https://ssrn.com/abstract=3938639 In addition to capturing the effect of being left-behind, α1 also captures the impact of growing up in the city as opposed to a rural area. We are unable to separately identify these impacts. From a policy perspective, however, the inability to separately identify these two effects is unimportant as a policy that supports parents to migrate with their children will lead to both impacts being experienced. It is important to understand, however, that migration in China, as a result of the hukou system which does not grant urban residency rights to those who were born in rural areas, is largely a temporary phenomenon, and is commonly characterized by “movements back and forth from rural to urban areas”, Dustmann et al. (2021). Hence those parents (and children) who migrate to cities normally return to their home village from where they may embark on another migration, often to another city. Only 37% of our sample of rural-urban migrants report having lived in only one city. Hence, those children who accompanied their parents who moved to the city for work are unlikely to have stayed permanently.18 There are two main issues we need to address when estimating equation 1. The first is that we have a choice-based sample. When estimating equation 1 (and all subsequent specifications with Prisoner i as the dependent variable) we need to account for the way in which our sampling frame was constructed. Incarceration is a rare event. To understand factors related to incarceration we cannot use a random sample of the population as that would provide a very small sample of prison inmates. To overcome this problem we oversampled prison inmates. We then use a case-control approach to account for this choicebased sampling. Case-control approaches have been widely used to study criminality and other relatively rare events such as maternal mortality and infant mortality (Ganatra et al., 1998; Blair et al., 1996; Dobrin, 2001). In this paper when modelling the probability of being incarcerated we present results from weighted probit estimation where the weights reflect the share of the sampled groups in the population.19 The second issue needing to be addressed is the potential endogeneity of both LBi and M igiP . Not everybody’s parents choose to migrate. The migration decision may depend 18 An additional possible benefit accrues to those who migrate with their parents and stay in the city – that of having lived in the city for a longer time and so having more extensive networks and a greater familiarity with the city systems. It is also possible that as our sample consists of people who are ruralurban migrants in the Shenzhen region, some of the “left-behind” individuals in our sample may have rejoined their parents when they migrated to the cities (if their parents’ migration was to Shenzhen and they remained there). However, due to the circular nature of rural-urban migration in China, these are very rare events as explained above. To the extent this happened and there were benefits to migrants reuniting with their parents in the city, our estimates will be underestimates of the impact of being left-behind. 19 An alternative approach is to estimate logistic regressions with a correction for the oversampling of the rare event. This approach relies on the assumption that the errors follow a logistic distribution, King and Zeng (2001) which can be tested by comparing the results with those obtained from weighted logit estimation, Xie and Manski (2010). If the errors follow a logistic distribution the results of the weighted regressions should be very similar to the results obtained by the corrected logistic regression. Table C1 in the appendix conducts this comparison and as the results differ substantially, we proceed using weighting to address the sample design. See Appendix B for a detailed discussion of how the weights are constructed. 11 Electronic copy available at: https://ssrn.com/abstract=3938639 on unobservable characteristics of the parents and their children, which in turn could also be related to whether the child later commits a crime or not. Also, once parents decide to migrate to a city, whether they take the child with them potentially reflects the household’s and child’s unobservable characteristics. These two potential selection biases could result in the estimates of α1 and α2 in Equation (1) being inconsistent. We employ two methods to handle the potential endogeneity: a control function approach and sibling fixed effects estimation. 3.1 Control Functions We are unable to use standard instrumental variables estimation to deal with the endogeneity because it produces biased estimates in a non-linear setting such as ours. Instead we use a control function approach as our main estimation method. This is closely related but parsimoniously handles fairly complicated models that are non-linear in endogenous explanatory variables, Wooldridge (2015).20 This approach involves adding variables (the control functions) to the regression to control for the endogenous components of LBi and M igiP and which then renders these variables appropriately exogenous. The control functions utilized are the generalized residuals from first stage regressions of the endogenous independent variables on all exogenous regressors in the system of equations. That is, we estimate probit models with the endogenous variables from the structural model as the dependent variables and the exogenous variables as the explanatory variables. So as to not be relying on functional form for identification we include instruments (zi ) which affect the dependent variable in the second stage only through their effect on LBi and M igiP . We estimate: LBi = γ1 Zi + 1i (2) M igiP = η1 Zi + 2i (3) where Zi = (Xi , WiP , Sis , zi , δp ). The exogenous variation induced by the instruments provides separate variation in the residuals obtained from the reduced form, and these residuals act as the control function. The instruments we use are: 1) the proportion of children aged 0-15 in five year age groups who were left-behind in one’s own sending county (calculated from the 2000 Population Census data);21 and 2) the share of individuals aged 20-44 who had migrated out of the 20 The non-linear functional form involves an assumption that the model is correctly specified in the first stage, which is not necessary in standard instrumental variables. 21 For individuals who were under the age of 16 in 2000 we set this instrument equal to the proportion of children of the same gender and age (five year age group) in their sending county who were left-behind in 2000. For those who were aged 16 and above in 2000 we set the instrument to zero as we assume they were not left behind which is reasonable because migration in those years (prior to the late 1990s) 12 Electronic copy available at: https://ssrn.com/abstract=3938639 county at the time of the 2000 Population Census. The share of people migrating and the share of children being left behind in the county-birth cohort will mainly be driven by the county’s geographic, economic, and cultural environment and so are likely to be independent of individuals’ family and personal situations. To further mitigate against the possibility that these variables are directly related to the probability of an individual committing a crime we also include the vector of prefecture and county level controls, SiS , and sending province fixed effects, δp , in all estimations. We then calculate the generalized residuals from each equation: r1 (LBi , δ1 Zi ) = LBi λ(δ1 Z) − (1 − LBi )λ(−δ1 Z) (4) r2 (M igiP , η1 Zi ) = M igiP λ(η1 Z) − (1 − M igip )λ(−η1 Z) (5) where λ is the inverse Mills ratio, φ(.)/Φ(.), and include them (and higher powers of these when they are statistically significant) in the second stage regression: P risoneri = β0 + β1 LBi + β2 M igiP + β3 xi + β4 r1i + β5 r2i + 3i 3.2 (6) Sibling Fixed Effects An advantage of our survey is that it provides information not only on the respondents’ characteristics and how long their parents were away due to migration before they turned 16 years of age, but also information on their siblings’ characteristics, how long the siblings were left behind by one or more parents, and whether their siblings ever committed a crime. Equipped with these data we are able to estimate a model with family fixed effects as follows: P risonerij = θ + γ1 LBij + γ2 Xij + Fj + εij , (7) where LBij is the number of years either parent was away from individual i in family j before he turned age 16; Xij is a vector of individual characteristics, including years of schooling, age, gender, and birth order;22 Fj are family fixed effects; and εij is the random error term. Equation (7) identifies the effect of being left behind on crime from within family variation in the length of time children were left behind. It examines whether variation in the length of time siblings were left-behind during childhood is associated with different 22 was a rare event. Survey respondents were directly asked the question as to how many years they were left-behind, whereas for their siblings we calculate this number from their birth year and the years of parental migration. To control for this discrepancy and potential measurement errors in other sibling variables due to recall errors, we also include a dummy variable indicating whether the observation is for a survey respondent or a sibling of a respondent. The results are also robust to including an interaction of this dummy variable with the parental absence variables. 13 Electronic copy available at: https://ssrn.com/abstract=3938639 probabilities of the siblings committing crimes when they become adults. The fixed effects model thus removes any effect of unobserved parental and household characteristics, e.g. variations in parental preferences and upbringing style, on the probability of children committing a crime later in life. It is an alternative to the control function approach. Although it brings us closer to identifying a true causal impact than estimates from equation 1 it does not completely solve the issue as child-specific unobservable characteristics may affect the probability of an individual child in the household being left-behind for a longer period than his or her sibling. For example, parents may elect to take unruly children with them which would bias the results. We judge that children’s characteristics are likely to play a lesser role in determining parent’s decisions than parental and household characteristics in the decision whether to leave a child behind. Regardless, a comparison of these results with estimates from equation 1 (which does not account for the potential endogeneity of the parental migration decision and the decision whether to leave a child behind) and the estimates from equation 6 (control function estimates) is instructive as an examination of the extent and direction of endogeneity bias in the original estimates. 4 4.1 The Empirical Results Parental Absence and Its Impact on Migrant Criminality We first examine whether parental absence in childhood due to migration, LBi , is associated with a higher probability of migrants committing crimes (and hence being incarcerated).23 Panel A in Table 3 presents marginal effects from weighted probits with the dependent variable defined to equal 1 if the individual is in prison and 0 otherwise (without the inclusion of generalized residuals as control functions). We start by regressing this variable, P risoneri , on LBi , a dummy variable indicating whether the individual was left-behind between age 0 and 15 due to parental migration, M igiP , whether one or both parents ever migrated to an urban area, and a set of provincial fixed effects. Column 1 of Table 3 shows that migration of parents is significantly associated with a reduced likelihood of incarceration in adulthood (at the time of the survey) – a 0.7 percentage point decrease – but that being left behind in the rural area by migrant parents significantly increases the probability of incarceration by 2.5 percentage points relative to children who were not left behind (significant at the 1% level). Left-behind children are thus 2.8 percentage points more likely (α1 + α2 ) to be incarcerated in adulthood than children whose parents never migrated and 2.5 percentage points more likely than children who 23 Note that our dependent variable reflects incarceration, and not criminality per se. It thus reflects the probability of committing a crime and the probability of being caught and jailed. The widely cited crime statistics that show crime increasing in China similarly reflect criminal convictions, rather than the number of crimes actually committed. As not everyone who commits a crime is imprisoned, our estimates are likely to be an underestimate of the effect on criminality. 14 Electronic copy available at: https://ssrn.com/abstract=3938639 were either older than 15 when parents migrated or taken to the cities with their parents. Column 2 presents results when household, individual and regional (prefecture and county) controls are added. The addition of the controls leaves the size of the association of being left-behind with incarceration largely unchanged such that being left-behind is associated with a 2.5 percentage point increase in the probability of incarceration relative to children whose parents didn’t migrate (and to children whose parents migrated when they were older than 15 or were took them to cities with them, as the coefficient on parents migrating is now insignificant and close to zero). 8.5 percent of the weighted sample were left-behind, so the predicted increase in the incarceration rate is 0.025 x 0.085 = 0.21 percentage points. This is a large effect - a 8.5 % increase - as the incarceration rate for males in Shenzhen is 2.5%. Column 3 shows results when we control for the length of parental absence, instead of just whether the child was left-behind or not.24 Every additional year of parental absence is associated with a 0.2 percentage point increase in the probability of being incarcerated as an adult. (The average years of absence in our sample is 8 years.) Being married reduces the probability of being in prison. As does having a better educated mother and higher cognitive ability. Coming from a prefecture with a higher GDP/capita is associated with an increased incarceration probability, possibly at odds with expectations. Higher inequality is positively associated with a higher probability of being in prison, as is a higher sex-ratio (more males to females), consistent with Cameron et al. (2019) and Edlund et al. (2013). Being Han Chinese significantly decreases the probability of being in prison and the proportion of the prefecture population who are non-Han Chinese, i.e., from ethnic minority groups, is strongly associated with increased likelihood of incarceration. A higher teacher-student ratio reduces the probability of being incarcerated as an adult. As discussed above, the estimates presented in Panel A of Table 3 are potentially contaminated by selection bias as the decisions whether to migrate and whether to leave the child behind are likely to be a function of unobservable household and/or child characteristics. Panel B of Table 3 presents the control function results which correct for this potential endogeneity. The instruments are strongly statistically significant determinants of being left-behind, and marginally significant in the parental migration first stage (Columns 1 to 3 of Table C2).25 The test of joint significance of the generalized 24 The length of absence is calculated as the total number of years the individual was left-behind between age 0 and 15 by one or both parents. If the mother and father were absent for different number of years we use the maximum of these two figures. 25 Jack-knifed standard errors are reported for these specifications. The control function approach involves including a control for the endogenous component of the potentially endogenous variables. The endogenous component falls in to the (generalized) residuals from the first stage and for identification (other than that achieved off nonlinearities) at least one instrument with a non-zero coefficient is needed, Wooldridge (2015). Our first stages for the left-behind variables are strong. The first stages for parental migration are less strong but have one variable either statistically significant or close to it. (The county left-behind rate is not statistically significant in the parental migration regression. 15 Electronic copy available at: https://ssrn.com/abstract=3938639 residuals in the second stage indicates that they are statistically significant in the specification using the left-behind dummy variable (Column 2 of Table 3) and when we focus on the length of time left-behind (Column 3 of Table 3), p=0.018 and p=0.066 respectively. This suggests that being left-behind and parental migration are likely to be endogenous to criminality. Being left-behind remains statistically significant in the second stages. The magnitude of the effect is now larger. Being left-behind by either/both parent increases the probability of being incarcerated as an adult by 12.2 percentage points relative to otherwise similar children who were not left–behind (statistically significant at the 5% level). The coefficient on parental migration is now also larger but remains statistically insignificant. Column 3 shows that an additional year of absence is associated with a 0.5 percentage point increase of the probability of incarceration (significant at the 10% level). The parental migration dummy in this specification is also positive and statistically significant, adding to the disadvantage of left-behind children compared to children whose parents never migrated. Having one or both parents migrate is associated with a 10 percentage point increase in the probability of being incarcerated. The results of our second method of accounting for the potential endogeneity of the decision whether to leave a child behind – estimation with sibling fixed effects - are shown in Table 4. Column 1 of Table 4 presents results analogous to those in Table 3 and examines the effect of the length of parental absence (defined as the absence of the father or the mother, whichever is longer). Column 2 examines paternal absences, column 3 maternal absences, and column 4 includes the length of both maternal and paternal absences. We control for years of schooling, age, gender (although our sample comprises only male individuals, many have female siblings) and birth order. The fixed effects results are largely consistent with the control function results in that every additional year of absence of either parent when the individuals were less than 16 years of age increases the probability of being incarcerated as an adult. The estimated magnitude of the effect is however larger (1.4 percentage points per year of absence versus 0.5 percentage points, and now significant at the 1% level). How the length of parental absence is measured does not seem to matter, there is a slightly larger effect detected for mothers’ absences than fathers’ absences.26 This is anticipated as it was only intended to predict the county left-behind rate.) Nevertheless, to examine the sensitivity of the results to the ability to instrument for parental migration, we estimated models where we exclude observations where parents did not migrate and so only estimate one first stage equation - for being left-behind. The point estimates are of a similar magnitude. Due to the significantly smaller sample (412 observations) the estimates are imprecisely estimated. These results are presented in Appendix C3. 26 The sibling fixed effects results could be larger than the control function results for several reasons. The control function approach attempts to correct for the potential endogeneity of being left-behind that is a result of unobserved family and child characteristics. The sibling fixed effects model controls for unobservable family factors which affect how long each child is left-behind, but not for unobservable child characteristics. The larger effect in the sibling fixed effects estimation could reflect that children who are left behind are those who behaviorally are more likely to end up in prison. The differences between the sets of estimates however also reflect the different sample in the sibling fixed effects estimation as 16 Electronic copy available at: https://ssrn.com/abstract=3938639 The within family estimations also show that less-educated individuals are more likely to be incarcerated and younger siblings are less likely to be in prison. Males are much more likely to commit crime. These results are intuitive and consistent with the criminality literature. 4.2 Identifying the channels via which parental absence affects criminality The previous discussion provides empirical evidence that parental absence due to migration increases the probability of an individual committing crime. However, it does not tell us how being left-behind results in greater criminality. Sociologists have long discussed the importance of complete family structure as a form of ‘social capital’ in inducing children’s aspiration to achieve educational and other social advantages (see, for example, Bourdieu, 1977; Coleman, 1988). And, as discussed above, psychologists and economists have shown that family structure can affect children’s behavior. Thus, possible mechanisms via which being left-behind may affect the probability of committing a crime in adulthood include lower educational attainment (leading to disadvantage and fewer viable income-earning options), and impacts on children’s behavioral traits such as risk and time preferences, and personality traits. We examine the relative importance of each of the above channels through which parental absence may affect criminality below. We start by examining educational attainment and then go on to examine the role of behavioral preferences and personality traits. 4.2.1 Education Studies of educational outcomes of left-behind children in China are abundant. Most find that being left-behind has a negative correlation with schooling outcomes whether relative to children whose parents did not migrate or children who migrated with parents to urban cities (see, for example, Zhang et al., 2014; Meng and Yamauchi, 2017; Wang, 2019; Chen, 2018). The existing studies examine left-behind children’s school performance when they were still at school, while here we examine their final educational outcome as measured by years of schooling in adulthood. The results for education are presented in Table 5. Columns 1 and 2 present results of ordinary least squares estimations which show that being left behind in childhood due to parental migration is associated with lower educational attainment, controlling for individual’s innate cognitive ability. Individuals who were left-behind due to parental there is one observation for each sibling pair (with many of those with siblings have more than one) and those without siblings are excluded. Table 2 shows that only children are less likely to be in prison. It would be interesting to examine whether parental absence is particularly problematic in some age ranges. Our sample is however not large enough to allow such an examination. 17 Electronic copy available at: https://ssrn.com/abstract=3938639 migration attain on average about 0.8 years less education and each additional year they are left-behind reduces education by around 0.07 of a year. Columns 3 and 4 present the control function results. The coefficient on being left-behind becomes slightly larger and on the length of being left-behind remains about the same size but both are not precisely estimated. The coefficients on parental migration, however, now become large and statistically significant. Thus, left-behind children obtain less education relative to children whose parents migrated but were not left-behind, and even less again compared to children of parents who never migrated. The tests of joint significance of the generalized residuals are not significant which suggests that the specifications in Columns 1 and 2 may be preferred.27 4.2.2 Behavioral Preferences and Personality Traits Table 6 presents the results of regressing measures of risk-aversion and time preferences (patience) on the indicator of being left-behind, LBi , the indicator of parental migration and the same individual and household control variables included previously. We examine two measures of both risk and time preferences. The first set of measures of risk and time preference measures are continuous variables. Risk-aversion takes on greater values the later the individual switches from the certain payment to the risky gamble, i.e., when the amount to be won in the gamble is higher. Patience reflects the number of times the individual chose to wait longer in order to receive the larger sum of money. The higher the value of this variable, the more patient is the individual. The second set of measures indicate that the respondent selected the riskiest or least patient choice. Riskiest equals 1 if the respondent always chose the gamble instead of the certain payment in the risk game, and zero otherwise. The time preferences variable, Least Patient is defined analogously and reflects whether the respondent always chose to get the money in the time preferences task in one month’s time rather than after seven months. We examine the effect of being left-behind on these extreme behaviors as extreme risk-taking and lack of patience are likely to be more strongly associated with criminality. Columns 1 to 4 of Table 6 present results of tobit estimation for the continuous variables (which are censored as they lie on a twelve point scale) and probit estimation (for the riskiest and least patient indicators). They show that being left-behind has a negative, but insignificant, effect on risk-aversion. It however is strongly associated with a greater probability of making the riskiest decisions (it increases the probability of doing so by 8.7 percentage points). The coefficients on being left-behind are insignificant in both time preference equations. It is possible that parents take into account the behavioral traits of their children 27 When using sibling fixed effects we also observe a reduction in education with each year of being leftbehind, around 0.06 of a year of schooling, but due to small within family variation in education, this result is not statistically significant. Results available on request. 18 Electronic copy available at: https://ssrn.com/abstract=3938639 when deciding whether to leave them behind when they migrate. To remove the potential for endogeneity bias, Columns 5 to 8 present the analogous results when we include the control function to correct for endogeneity. Being left-behind now has a larger and more strongly significant effect on both the probability of making the riskiest choice and the continuous measure of risk-aversion. Being left-behind is associated with a 0.35 decrease in the switching point on a 12 point scale and increases the probability of making the riskiest choice by 64 percentage points. This is a very large effect. (Only 19% of those who weren’t left-behind chose this option.) The 95% confidence interval for this variable lies between 0.16 and 1.11 so the true impact, while large, may not be of this magnitude. The result for time preferences is again negative and insignificant, although those who were left-behind are significantly (p=0.08) more likely to make the least patient choices than those whose parents stayed with them in the rural areas. The test of joint significance of the generalized residuals strongly suggests endogeneity for the risk measures and for making the least patient choices. The difference between the control function results and the results in Columns 1 to 4 suggests that parents are less likely to leave risk-loving and impatient children behind. This could reflect a fear that risk-loving, impatient children might be more likely to get up to mischief in their parents’ absence. Table 7 presents a similar analysis except in this case for personality traits. There are no robust findings in relation to personality traits. The OLS results suggest a significant negative relationship between being left-behind and extroversion, which becomes insignificant in the control function results. The control function results suggest that being left-behind may result in lower levels of conscientiousness. However, the tests of the joint significance of the generalized residuals do not find strong evidence of the endogeneity of being left-behind with respect to these traits, except in relation to neuroticism. 4.3 Do lower educational attainment and risk-loving preferences explain the left-behind effect? In this sub–section we examine whether the potential mechanisms we have identified explain the effect of being left-behind on criminality. We do this by including these variables as additional explanatory variables in the criminality regression, alongside being left-behind.28 Tables 8 present the results (estimated using the control function). Column 1 presents the original results from Panel B of Table 3 which show that being left-behind is strongly associated with an increased probability of being incarcerated.29 28 The first stage estimation results are shown in Appendix Table C2. Columns 4 and 5 of Table C2 are the first stages for Column 2 of Table 8 and Columns 6 and 7, and 8 and 9 of Table C2 are first stages results for Columns 3 and 4 of Table 8. In all cases the instruments have predictive power over being left-behind but not parental migration. 29 A possible concern with these results is that being in prison may affect preferences and so we may be picking up the effect of being incarcerated on preferences rather than the effect of preferences on criminality. This is less of a concern for the Big-5 personality traits which are thought to be relatively 19 Electronic copy available at: https://ssrn.com/abstract=3938639 We start by adding controls for years of schooling in Column 2. This reduces the magnitude of the effect of being left-behind by more than half (relative to those who went to the city with their parents) from 0.122 to 0.052 and the effect becomes insignificant. Those who were left-behind in rural areas however still remain more likely to be in prison than those whose parents did not migrate (by 12.3 percentage points, p=0.07). Next we add behavioral preferences (risk-aversion and patience), in addition to years of schooling. We include a square of risk aversion as the data suggest the relationship is non-linear.30 The coefficient on risk aversion is negative and significant at the 5% level and the squared term is small, positive and significant at the 1% level. A reduction in risk aversion associated with being left-behind is associated with a small increase in the probability of being incarcerated.31 Adding our measures of risk aversion and patience further reduces the coefficient on being left-behind from 0.052 to 0.046. The difference between those who were left-behind and those who lived with their parents in rural areas now also becomes statistically insignificant (p=0.104). Finally we add the Big-5 personality traits (Column 4 of Table 8). Agreeableness and extroversion are significantly negatively associated with criminality. The coefficient on being left-behind remains statistically insignificant as does the difference between those in rural areas who were left-behind and those who were not. To quantify the relative importance of the estimates in Table 8 we conduct a mediation analysis (following, for example, Heckman et al. (2013) and Heckman and Pinto (2015)). The mediation analysis identifies the share of the impact of being left-behind (and parental migration) on criminality that is attributable to the direct effect of being left-behind and the share attributable to indirect effects operating through the mediating factors, i.e., education and behavioral preferences. We also decompose the indirect effect into components attributable to each of the individual mediating variables. Section D in the appendix explains the methodology in more detail.32 stable over time but is a greater concern for the questions on time preferences and risk attitudes. If incarceration does affect behaviour then one would expect that the length of time spent in prison would be associated with behavioural differences. In another paper which uses the same data, Cameron et al. (2019), we test this by adding the time spent in prison and sentence length as additional control variables. Doing so shows that they are not significant determinants of these behavioural preferences. 30 We also ran a specification which included a square of patience but it was insignificant. 31 Calculated from the mean level of risk-aversion of those who were not left-behind and using the estimate of the effect of being left-behind in Column 6 in Table 6. 32 The decomposition method makes assumptions similar to many mechanical decompositions e.g. Oaxaca decomposition. It assumes away the impacts of unobserved mediators that are correlated with the observed mediators and control variables (so we can attribute the component calculated to accrue to the observed mediators and the control variables to these variables, and not unobserved mediators that are correlated with them) and that treatment (being left-behind) does not affect the impact of the mediator variables or the control variables on the outcome variable (the probability of being incarcerated). That is, the model is correctly specified without interactions between treatment and these variables (as is suggested when tested in the OLS model and which cannot be tested in the control function model owing to a lack of suitably strong instruments for the interaction terms). As we do not find a systematic effect of being left-behind on big-5 personality traits, we do not treat them as mediating variables. Doing so, however, does not substantively affect the results. 20 Electronic copy available at: https://ssrn.com/abstract=3938639 The mediation analysis finds that 64% of the effect of being left-behind on criminality operates via a direct effect, with the remaining 36% being due to the effect of being leftbehind on mediating variables. Education is by far the most important mediating factor accounting for 94% of the indirect effect, or 34% of the total impact of being left-behind on criminality. Risk-preferences account for 6% of the indirect effect (2.1% of the total impact). Time preferences have no impact. To summarize, our analysis suggests that being left-behind in childhood due to parental migration has adverse impacts on several human capital related attributes. We examined the impact on educational attainment, personality traits, and risk and time preference. We did not find any robust relationship with personality traits. However, being leftbehind makes people more risk-loving and less likely to stay in school. These attributes partially explain why being left-behind increases the probability of committing crimes in adulthood. The most important channel is through being left-behind reducing educational attainment. 5 Conclusions Industrialization, and its accompanying large scale rural-to-urban migration, coupled with the hukou residency system has induced dramatic changes in Chinese family structure over the past three decades. A large cohort of children of rural-urban migrants have been leftbehind in rural villages. The lack of parental care during childhood has been shown to have important social consequences in the short run. This has aroused concern among public policy makers and society in general in relation to the possible long-term consequences for anti-social behavior. Although there are many studies that have examined the impact in childhood of being “left-behind” on education and health (including mental health), little is known about the impacts of parental absence on behavior in adulthood. This paper uses unique survey and experimental data on rural-urban migrants – prison inmates and comparable non-inmates – in China to examine whether parental absence in childhood is associated with increased criminality in adulthood. We find that parental absence in childhood due to migration increases the propensity for men to commit crimes. Being left-behind reduces educational attainment and increases risk-loving behaviors, both of which are associated with increased criminality in adulthood. However, the decrease in educational attainment plays the more important role in increasing criminality. These findings provide useful insights for policy-making aimed at alleviating the social costs arising from rural-to-urban migration. While the generalizability of our results may be limited by our focus on people who were rural-urban migrants at the time of our surveys, our findings suggest that policies that support migrants to migrate with their families and, failing that, policies that support children who are left-behind to stay in school, are 21 Electronic copy available at: https://ssrn.com/abstract=3938639 likely to provide significant benefits to Chinese society. 22 Electronic copy available at: https://ssrn.com/abstract=3938639 References All-Women Federation in China (2013). Research report on the rural left-behind children and the rural-to-urban migrating children (in chinese). China Womens Movement (zhongguofuyun) 6, 3034. Almond, D., J. Currie, and V. Duque (2018, December). Childhood circumstances and adult outcomes: Act ii. Journal of Economic Literature 56 (4), 1360–1446. Amato, P. R. and B. Keith (1991). Parental divorce and adult well-being: A meta-analysis. Journal of Marriage and the Family, 43–58. Blair, P. S., P. J. Fleming, D. Bensley, I. Smith, C. Bacon, E. Taylor, J. Berry, J. Golding, and J. Tripp (1996). Smoking and the sudden infant death syndrome: results from 1993-5 case-control study for confidential inquiry into stillbirths and deaths in infancy. Bmj 313 (7051), 195–198. Bornstein, M. H. (Ed.) (2002). Handbook of Parenting. New York: Routledge. Bourdieu, P. (1977). Cultural reproduction and social reproduction. Brady, C. P., J. H. Bray, and L. Zeeb (1986). Behavior problems of clinic children: Relation to parental marital status, age and sex of child. American Journal of Orthopsychiatry 56, 399–412. Cameron, L., A. Chaudhuri, N. Erkal, and L. Gangadharan (2009). Propensities to engage in and punish corrupt behavior: Experimental evidence from australia, india, indonesia and singapore. Journal of Public Economics 93 (7-8), 843–851. Cameron, L., X. Meng, and D. Zhang (2019). China’s sex ratio and crime: Behavioural change or financial necessity? The Economic Journal 129, 790–820. Chen, Y. (2018). School performance of chinese iternal migrants’ children. Discussion Paper, Center for Research in Economic Analysis, University of Luxembourg. Coleman, J. S. (1988). Social capital in the creation of human capital. The American Journal of Sociology 94, S94–S120. Demuth, S. and S. L. Brown (2004). Family structure, family processes, and adolescent delinquency: The significance of parental absence versus parental gender. Journal of research in crime and delinquency 41 (1), 58–81. Dobrin, A. (2001). The risk of offending on homicide victimization: A case control study. Journal of Research in Crime and Delinquency 38 (2), 154–173. Dustmann, C., F. Fasani, X. Meng, and L. Minales (2021). Risk attitudes and household migration decisions. Journal of Human Resources. Economist, T. (2015, October). Chinas left-behind generation: Pity the children. The Economist. Edlund, L., H. Li, J. Yi, and J. Zhang (2013). Sex ratios and crime: Evidence from china. Review of Economics and Statistics 95 (5), 1520–1534. 23 Electronic copy available at: https://ssrn.com/abstract=3938639 Fellmeth, G., K. Rose-Clarke, C. Zhao, L. K. Busert, Y. Zheng, A. Massazza, H. Sonmez, B. Eder, A. Blewitt, W. Lertgrai, M. Orcutt, K. Ricci, O. Mohamed-Ahmed, R. Burns, D. Knipe, S. Hargreaves, T. Hesketh, C. Opondo, and D. Devakumar (2018). Health impacts of parental migration on left-behind children and adolescents: a systematic review and meta-analysis. The Lancet 392, 2567–2582. Finlay, K. and D. Neumark (2010). Is marriage always good for children? evidence from families affected by incarceration. Journal of Human Resources 45 (4), 1046–1088. Friesen, L. (2012, Oct). Certainty of punishment versus severity of punishment: An experimental investigation. Southern Economic Journal 79 (2), 399–421. Friesen, L. and L. Gangadharan (2013, Oct). Designing self-reporting regimes to encourage truth telling: An experimental study. Journal of Economic Behavior and Organisation 94, 90–102. Ganatra, B., K. Coyaji, and V. Rao (1998). Too far, too little, too late: a communitybased case-control study of maternal mortality in rural west maharashtra, india. Bulletin of the World Health Organization 76 (6), 591. Geismar, L. L. and K. M. Wood (1986). Family and Delinquency: Resocializing the Young Offender. New York: Human Science Press. Grogger, J. and N. Ronan (1995). The intergenerational effects of fatherlessness on educational attainment and entry-level wages. National Longitudinal Surveys Discussion Paper . Gruber, J. (2004). Is making divorce easier bad for children? The long-run implications of unilateral divorce. Journal of Labor Economics 22 (4), 799–833. Hanson, G. H. and C. Woodruff (2003). Emigration and educational attainment in mexico. Technical Report 1, Mimeo., University of California at San Diego. Heckman, J. and R. Pinto (2015). Econometric mediation analyses: Identifying the sources of treatment effects from experimentally estimated production technologies with unmeasured and mismeasured inputs. Econometric Reviews 34 (1-2), 6–31. Heckman, J., R. Pinto, and P. Savelyev (2013). Understanding the mechanisms through which an influential early childhood program boosted adult outcomes. American Economic Review 103 (6), 2052–2086. Hirschi, T. (1969). Causes of Delinquency. Berkeley: University of California Press. Hong, Y. and C. Fuller (2019). Alone and left-behind: A case study of left-behind children in rural china. Cogent Education 6 (1), 1–16. King, G. and L. Zeng (2001). logistic regression in rare events data. Political Analysis 9 (2), 13763. Lang, K. and J. L. Zagorsky (2001). Does growing up with a parent absent really hurt? Journal of Human Resources, 253–273. Lersch, P. and J. Baxter (2021). Parental separation during childhood and adult children’s wealth. Social Forces 99, 1176–1208. 24 Electronic copy available at: https://ssrn.com/abstract=3938639 Lewis, W. A. (1954, May). Economic development with unlimited supplies of labour. The Manchester School 22 (2), 253–273. Lochner, L. and E. Moreffi (2004). The effect of education on crime: Evidence from prison inmates, arrests, and self-reports. American Economic Review 94 (552), 155–189. Maccoby, E. E. and J. Martin (1983). Specialization in the context of the family: Parentchild interaction. Machin, S., O. Marie, and S. Vujic (2011). The crime reducing effect of education. Economic Journal 121 (552), 463–484. Manski, C., G. Sandefur, S. McLanahan, and D. Powers (1992). Alternative estimates of the effect of family structure during adolescence on high school graduation. Journal of the American Statistical Association 87 (417), 25–37. Mazar, N., O. Amir, and D. Ariely (2008). The dishonesty of honest people: a theory of self-concept maintenance. Journal of Marketing Research 45, 633–644. McLanahan, S. and C. Percheski (2008). Family structure and the reproduction of inequalities. Annual Review of Sociology. McLanahan, S. and G. Sandefur (1994). Growing Up with a Single Parent: What Hurts, What Helps, Volume 34. Cambridge, Mass: Harvard University Press. Meng, X. and C. Yamauchi (2017). Children of migrants: The impact of parental migration on their childrens education and health outcomes. Demography 54 (5), 1677–1714. Murnighan, J. K., A. E. Roth, and F. Schoumaker (1988). Risk-aversion in bargaining: An experimental study. Journal of Risk and Uncertainty 1, 101–124. NBS (2020). 2019 Monitoring Report on Rural Migrant Workers. Beijing: China Statistics Press. http://www.stats.gov.cn/tjsj/zxfb/202004/t20200430_1742724.html. Nye, I. F. (1958). Family Relationships and Delinquent Behavior. New York: John Wiley and Sons. Sandefur, G. D., T. Wells, et al. (1997). Using siblings to investigate the effects of family structure on educational attainment. Institute for Research on Poverty Working Papers. Shi, Y., Y. Bai, Y. Shen, K. Kenny, and S. Rozelle (2016). Effects of parental migration on mental health of left-behind children: Evidence from northwestern china. China & World Economy 24 (3), 105–122. van Hoorn, J., E. McCormick, C. Rogers, S. Ivory, and E. Telzer (2018). Differential effects of parent and peer presence on neural correlatses of risk taking in adolescence. Social Cognitive and Affective Neuroscience, 945–955. Van Voorhis, P., F. Cullen, R. Mathers, and C. Chenoweth Garner (1988). The impact of family structure and quality on delinquency: Acomparative assessment of structural and functional factors. Criminology 26, 235–261. 25 Electronic copy available at: https://ssrn.com/abstract=3938639 Wang, S. X. (2019). Timing and duration of parental migration and the educational attainment of left-behind children: Evidence from rural china. Review of Development Economics 23 (2), 727–744. Wood, P., B. Pfefferbaum, and B. Arneklev (1993). Risk-taking and self-control: Social psychological correlates of delinquency. Journal of Crime and Justice 16, 111–130. Wooldridge, J. (2015, Spring). Control function methods in applied econometrics. Journal of Human Resources 50 (2), 420–445. World Bank (2009). From Poor Areas to Poor People: China’s Evolving Poverty Reduction Agenda : an Assessment of Poverty and Inequality in China. World Bank. Wright, K. N. and K. E. Wright (1993). Family Life and Delinquency and Crime: A Policymaker’s Guide to the Literature. U.S Department of Justice. Xie, Y. and C. F. Manski (2010). The logit model, the probit model, and response-based samples. University of Wisconsin-Madison, Center for Demography and Ecology 88-4. Yang, D. (2008). International migration, remittances and household investment: Evidence from philippine migrants exchange rate shocks. The Economic Journal 118 (528), 591–630. Zhang, H., J. R. Behrman, C. S. Fan, X. Wei, and J. Zhang (2014). Does parental absence reduce cognitive achievements? evidence from rural china. Journal of Development Economics 111, 181–195. Zhang, Y., S. Liu, and L. Liu (2011a). Can we attribute increasing criminal rate to enlarging urban-rural inequality in china? (in chinese). Economic Research Journal (jingjiyanjiu 2, 5972. Zhang, Y., S. Liu, and L. Liu (2011b). Ruralurban income difference, unemployment of migrants, and increase in chinas crime rate (in chinese). Economic Research Journal (jingjiyanjiu 46 (2), 5772. Zhao, C., F. Wang, L. Li, X. Zhou, and T. Hesketh (2017). Long-term impacts of parental migration on chinese children’s psychosocial well-being: mitigating and exacerbating factors. Social Psychiatry and Psychiatric Epidemiology 52, 669–677. Zinn, M. B., D. S. Eitzen, and B. Wells (2016). Diversity in Families (Tenth Edition. Pearson. 26 Electronic copy available at: https://ssrn.com/abstract=3938639 Table 1: Left–Behind Status Full Sample 8.46 At least one parent absent (%) of which: Both parents absent Only father absent Only mother absent Neither parent absent N Prison Non-inmate Inmates Migrants 15.93 8.28 6.01 1.75 0.71 11.80 2.65 1.47 5.86 1.72 0.69 91.54 84.07 91.72 968 678 290 Note: We present weighted means of variables. 27 Electronic copy available at: https://ssrn.com/abstract=3938639 Table 2: Descriptive Statistics All (1) By Left-behind Status LeftNot leftbehind behind (2) (3) By Prisoner Status NonPrisoners prisoners (4) (5) Individual Characteristics: Age Married Siblings Only Child Paternal education Maternal education Cognitive ability score Han Ethnicity Parents had been in jail 29.4 0.43 1.74 0.10 8.01 6.70 6.92 0.97 0.01 24.5 0.20 1.35 0.24 8.65 8.02 7.79 1.00 0.00 29.7 0.44 1.77 0.08 7.96 6.60 6.85 0.96 0.01 29.8 0.32 2.00 0.06 6.92 4.94 5.93 0.86 0.02 29.3 0.43 1.74 0.10 8.04 6.74 6.94 0.97 0.01 Outcome Variables: Prisoner inmate Years of education Risk aversion Riskiest Patience Least Patient Conscientious Extroverted Agreeable Neurotic Openness 0.024 11.12 5.97 0.13 5.51 0.22 3.63 3.33 3.77 2.66 3.37 0.045 12.09 4.95 0.20 3.62 0.44 3.59 3.37 3.71 2.75 3.39 0.023 11.04 6.05 0.13 5.66 0.20 3.64 3.33 3.77 2.66 3.37 7.95 6.82 0.23 5.26 0.32 3.42 3.02 3.54 2.89 3.16 11.19 5.95 0.13 5.52 0.22 3.64 3.34 3.78 2.66 3.38 Home Prefecture Variables: ln(GDP/capita) Gini coefficient Ethnic minority share Sex-ratio 9.46 0.35 0.05 1.12 9.52 0.35 0.02 1.13 9.46 0.35 0.05 1.12 9.38 0.35 0.13 1.15 9.47 0.35 0.04 1.12 0.05 0.05 0.05 0.05 0.05 0.05 0.12 968 0.05 0.24 109 0.05 0.11 859 0.07 0.12 678 0.05 0.12 290 Home County Variables: County teacher/student ratio Instruments: County outmigration rate County left-behind rate No. of obs. Note: 1. Columns (1) to (3) present weighted means. 28 Electronic copy available at: https://ssrn.com/abstract=3938639 Table 3: Marginal Effects from Weighted Probit Estimation Dependent Variable: Prisoner (0/1) A. Not allowing for Endogeneity Left-behind (1) (2) 0.025∗∗∗ (0.007) 0.025∗∗∗ (0.008) Length of parental absence -0.007∗ (0.004) Parent(s) migrated Age Married Cognitive ability score Siblings Only child Paternal education Maternal education Han ethnicity Parent had been in jail Regional control variables: Ln(Prefecture GDP/capita) Prefecture gini coefficient Ethnic minority share Prefecture sex ratio County teacher-student ratio Test of α1 + α2 = 0 (p-value): B. Control Function Results Left-behind 0.007 0.003 (0.004) 0.001 (0.000) -0.018∗∗∗ (0.005) -0.004∗∗∗ (0.001) 0.000 (0.002) -0.002 (0.008) -0.001 (0.001) -0.003∗∗∗ (0.001) -0.021∗ (0.012) 0.014 (0.019) 0.013∗∗ (0.005) 0.093∗ (0.054) 0.079∗∗∗ (0.022) 0.141∗∗∗ (0.042) -1.250∗∗∗ (0.330) 0.0003 (3) 0.002∗∗∗ (0.001) 0.004 (0.004) 0.001 (0.000) -0.019∗∗∗ (0.006) -0.005∗∗∗ (0.001) 0.000 (0.002) -0.003 (0.008) -0.001 (0.001) -0.003∗∗∗ (0.001) -0.021∗ (0.012) 0.014 (0.019) 0.013∗∗ (0.005) 0.102∗ (0.054) 0.078∗∗∗ (0.022) 0.150∗∗∗ (0.042) -1.217∗∗∗ (0.330) 0.122∗∗ (0.056) Length of parental absence Parent(s) migrated Test of α1 + α2 = 0 (p-value): Test of jt sig. of the generalized residuals (p-value): N 0.066 (0.056) 0.009 0.018 968 0.005∗ (0.003) 0.101∗ (0.053) 0.066 968 Note: 1. We present marginal effects from weighted probits where we weight by the ratio of the population size (of migrants/inmates) to the sample size (of migrants/inmates). All specifications also include province fixed effects. The specifications in Panel B also include the full set of control variables shown in Panel A. 2. Robust standard errors are shown in parentheses. Panel B reports jackknifed standard errors. 3. Column 1 in Panel B uses generalized residuals calculated from the specifications in Columns 1 and 3 in Table C2 and Column 2 in Panel B above uses generalized residuals calculated from the specifications in Columns 2 and 3 in Table C2. 29 Electronic copy available at: https://ssrn.com/abstract=3938639 Table 4: Sibling Fixed Effects Results Dependent Variable: Prisoner (0/1) Length of parental absence (1) 0.014∗∗∗ (0.004) (2) Male Birth order Constant Observations Within R2 0.008 (0.006) 0.010 (0.006) -0.012∗∗∗ (0.002) -0.004∗ (0.002) 0.048∗∗∗ (0.012) -0.023∗∗∗ (0.009) 0.256∗∗∗ (0.092) 3061 0.666 -0.012∗∗∗ (0.002) -0.004 (0.002) 0.048∗∗∗ (0.012) -0.023∗∗∗ (0.009) 0.250∗∗∗ (0.091) -0.012∗∗∗ (0.002) -0.004∗ (0.002) 0.048∗∗∗ (0.012) -0.023∗∗ (0.009) 0.253∗∗∗ (0.091) 0.015∗∗∗ (0.004) -0.012∗∗∗ (0.002) -0.004∗ (0.002) 0.048∗∗∗ (0.012) -0.023∗∗∗ (0.009) 0.263∗∗∗ (0.093) 3061 0.665 3061 0.665 3061 0.666 Years mother absent Age (4) 0.014∗∗∗ (0.004) Years father absent Years of education (3) Note: 1. Results presented here are from fixed effects estimation. We also control for whether the observation is an individual in our survey sample, or a sibling of such a person. 2. Standard errors are clustered at the household level and shown in parentheses. 3. *, **, and *** denote statistical significance at the 10%, 5%, and 1% levels, respectively. 30 Electronic copy available at: https://ssrn.com/abstract=3938639 Table 5: Effect of Being Left-Behind on Educational Attainment OLS Left-behind (1) -0.796∗∗∗ (0.294) Length of parental absence Parent(s) migrated Age Married Cognitive ability score Siblings Only child Paternal education Maternal education Han ethnicity Parent had been in jail -0.033 (0.200) -0.023 (0.015) 0.529∗∗ (0.206) 0.363∗∗∗ (0.037) -0.132 (0.084) -0.374 (0.369) 0.065∗∗ (0.029) 0.159∗∗∗ (0.027) 0.428 (0.368) -0.750 (0.473) Regional control variables: Ln(GDP/capita) Control Function (2) -0.069∗∗ (0.029) -0.071 (0.197) -0.023 (0.015) 0.540∗∗∗ (0.206) 0.363∗∗∗ (0.037) -0.129 (0.084) -0.373 (0.370) 0.066∗∗ (0.029) 0.158∗∗∗ (0.027) 0.428 (0.369) -0.834∗ (0.453) 0.187 0.199 (0.198) (0.198) Gini coefficient -0.454 -0.613 (2.310) (2.300) Ethnic minority share -0.883 -0.853 (0.564) (0.565) Sex ratio -2.598∗ -2.721∗ (1.540) (1.543) Teacher-Student Ratio 1.198 0.716 (13.240) (13.255) Test of joint significance of the generalized residuals (p-value): Test of α1 + α2 = 0 (p-value): 0.005 Observations 968 968 (3) -0.949 (1.432) -3.082∗ (1.735) -0.087∗∗ (0.037) 0.420∗ (0.222) 0.390∗∗∗ (0.041) -0.222∗∗ (0.099) -0.231 (0.405) 0.088∗∗∗ (0.032) 0.187∗∗∗ (0.031) 0.426 (0.379) -0.022 (0.757) 0.104 (0.203) 2.138 (2.765) -1.598∗∗ (0.688) -2.557 (1.559) -0.463 (13.669) 0.37 0.038 968 (4) -0.063 (0.061) -3.198∗∗ (1.569) -0.087∗∗ (0.036) 0.424∗ (0.219) 0.391∗∗∗ (0.040) -0.222∗∗ (0.099) -0.227 (0.393) 0.088∗∗∗ (0.031) 0.188∗∗∗ (0.032) 0.432 (0.378) -0.117 (0.644) 0.113 (0.203) 2.035 (2.703) -1.564∗∗ (0.689) -2.657∗ (1.557) -1.036 (13.712) 0.35 968 Note: We present coefficients from ordinary least squares estimation. Robust standard errors for OLS and Jackknifed standard errors for control function estimations are shown in parentheses. The specifications reported in Column 2 also includes generalized residuals calculated from the specifications in Columns 1 and 3 in Table C2. The specifications reported in Column 4 also includes generalized residuals calculated from the specifications in Columns 4 and 5 in Table C2. All specifications also include province fixed effects. 31 Electronic copy available at: https://ssrn.com/abstract=3938639 Table 6: Effect of Being Left-Behind on Behavioral Preferences (1) (2) (3) (4) Assuming exogeneity of being left-behind probit tobit probit tobit Estimation type: Dependent variable: Riskiest Left-behind Parent(s) migrated Age Married Cognitive ability score Siblings Only child Paternal education Maternal education Han ethnicity Parent had been in jail Regional control variables: Ln(GDP/capita) Gini coefficient Ethnic minority share Sex ratio Teacher-Student Ratio (5) (6) (7) Control Function Results probit tobit probit Least patient 0.069 (0.051) -0.033 (0.034) -0.004 (0.003) -0.012 (0.035) -0.001 (0.006) -0.002 (0.014) -0.096 (0.065) -0.005 (0.005) 0.002 (0.005) 0.150∗∗ (0.069) -0.243∗ (0.139) Patience Riskiest 0.087∗∗ (0.043) 0.022 (0.030) -0.004 (0.002) 0.042 (0.032) -0.010∗∗ (0.005) 0.015 (0.011) -0.009 (0.056) -0.005 (0.004) -0.000 (0.004) 0.038 (0.060) -0.069 (0.105) Risk aversion -0.032 (0.031) -0.015 (0.019) 0.004∗∗ (0.002) -0.029 (0.022) -0.003 (0.004) 0.003 (0.009) 0.031 (0.036) 0.001 (0.003) -0.002 (0.003) -0.037 (0.044) -0.001 (0.060) -0.021 (0.042) 0.006 (0.026) 0.003 (0.002) 0.018 (0.028) -0.011∗∗ (0.005) -0.009 (0.011) 0.049 (0.050) -0.002 (0.004) 0.002 (0.004) -0.137∗∗ (0.059) 0.092 (0.071) 0.048 (0.030) 0.224 (0.321) 0.159∗ (0.091) 0.545∗∗ (0.240) -0.316 (1.828) -0.015 (0.020) -0.024 (0.221) -0.045 (0.073) -0.425∗∗ (0.185) -0.250 (1.314) 0.018 (0.034) 0.269 (0.360) 0.384∗∗∗ (0.108) 0.836∗∗∗ (0.287) 4.463∗∗ (2.087) 0.47 968 Test of joint significance of the generalized residuals (p-value): Test of α1 + α2 = 0 (p-value): 0.01 0.13 Observations 968 968 (8) tobit Least patient 0.208 (0.384) 0.542 (0.380) 0.010 (0.007) 0.019 (0.038) -0.007 (0.007) 0.017 (0.016) -0.145∗∗ (0.073) -0.009∗ (0.005) -0.004 (0.006) 0.144∗ (0.075) -0.461∗ (0.256) Patience 0.638∗∗∗ (0.242) -0.108 (0.256) -0.001 (0.005) 0.065∗ (0.035) -0.010∗ (0.006) 0.017 (0.014) -0.072 (0.065) -0.006 (0.004) -0.001 (0.005) 0.016 (0.063) -0.304∗∗ (0.149) Risk aversion -0.349∗∗ (0.176) 0.164 (0.185) 0.004 (0.004) -0.039∗ (0.023) -0.004 (0.004) 0.005 (0.010) 0.060 (0.039) 0.000 (0.003) -0.003 (0.003) -0.024 (0.045) 0.100 (0.088) -0.019 (0.027) -0.406 (0.291) -0.279∗∗∗ (0.101) -0.623∗∗ (0.243) -4.604∗∗∗ (1.694) 0.064∗∗ (0.032) -0.169 (0.384) 0.197∗ (0.107) 0.424∗ (0.255) -0.431 (1.929) -0.022 (0.022) 0.108 (0.270) -0.042 (0.086) -0.346∗ (0.192) -0.057 (1.350) 0.041 (0.036) -0.386 (0.428) 0.540∗∗∗ (0.131) 0.768∗∗ (0.299) 4.623∗∗ (2.169) -0.032 (0.028) -0.044 (0.351) -0.374∗∗∗ (0.118) -0.596∗∗ (0.253) -4.772∗∗∗ (1.741) 0.73 968 0.007 0.06 968 0.038 0.37 968 0.038 0.08 968 0.338 0.13 968 -0.074 (0.251) -0.363 (0.246) -0.005 (0.005) 0.001 (0.030) -0.008 (0.005) -0.021 (0.013) 0.074 (0.055) 0.001 (0.004) 0.006 (0.004) -0.136∗∗ (0.062) 0.206∗ (0.112) Note: 1. We present marginal effects from probits and tobit estimation. 2. Robust standard errors are shown in parentheses. Columns 5 to 8 report jackknifed standard errors. 3. Columns 5 to 8 also include generalized residuals calculated from the specifications in Columns 1 and 3 in Table C2. All specifications also include province fixed effects. 32 Electronic copy available at: https://ssrn.com/abstract=3938639 Table 7: Effect of Being Left-Behind on Big Five Personality Traits (1) Extroverted Left-behind Parent(s) migrated Age Married Cognitive ability score Siblings Only child Paternal education Maternal education Han ethnicity Parent had been in jail Regional control variables: Ln(GDP/capita) Gini coefficient Ethnic minority share Sex ratio Teacher-Student Ratio Test of α1 + α2 = 0 (p-value): Left-behind Parent(s) migrated Test of joint sig. of gen’d residuals (p-value): Test of α1 + α2 = 0 (p-value): N -0.096∗ (0.050) 0.057∗ (0.032) -0.000 (0.002) 0.093∗∗∗ (0.032) 0.013∗∗ (0.005) -0.014 (0.012) 0.064 (0.060) -0.005 (0.005) 0.009∗∗ (0.004) 0.090 (0.058) -0.085 (0.110) -0.008 (0.030) -0.090 (0.349) -0.113 (0.088) -0.075 (0.243) -0.626 (1.942) 0.44 -0.186 (0.262) -0.304 (0.255) (2) (3) (4) (5) Open Neurotic Agreeable Conscientious A. No correction for endogenity -0.045 0.069 -0.060 -0.054 (0.050) (0.061) (0.046) (0.048) 0.017 -0.037 0.043 0.006 (0.030) (0.037) (0.031) (0.033) 0.003 0.003 0.001 0.003 (0.002) (0.003) (0.003) (0.003) 0.069∗∗ -0.085∗∗ 0.052 0.127∗∗∗ (0.031) (0.037) (0.032) (0.032) 0.021∗∗∗ -0.016∗∗∗ 0.021∗∗∗ 0.022∗∗∗ (0.005) (0.006) (0.005) (0.006) 0.023∗ -0.007 0.012 -0.011 (0.012) (0.014) (0.012) (0.013) 0.082 -0.054 0.036 -0.038 (0.059) (0.072) (0.051) (0.059) 0.008∗∗ -0.007 0.006 -0.003 (0.004) (0.005) (0.004) (0.005) 0.010∗∗∗ 0.004 0.001 0.005 (0.004) (0.005) (0.004) (0.004) 0.000 -0.063 0.044 0.120∗ (0.061) (0.060) (0.065) (0.063) -0.082 0.234 -0.084 -0.149 (0.074) (0.145) (0.067) (0.091) -0.048∗ 0.093∗∗∗ -0.060∗∗ (0.028) (0.036) (0.030) 0.187 0.061 0.282 (0.323) (0.379) (0.325) -0.114 0.102 -0.158 (0.093) (0.096) (0.106) -0.048 0.194 -0.057 (0.217) (0.317) (0.243) 2.815 -0.908 0.548 (1.882) (2.363) (1.881) 0.58 0.59 0.71 B. Control Function Results -0.010 -0.158 -0.073 (0.263) (0.305) (0.243) -0.272 -0.142 0.821∗∗∗ (0.290) (0.287) (0.307) 0.45 0.10 968 0.98 0.63 968 0.0002 0.04 968 0.63 0.29 968 -0.107∗∗∗ (0.032) 0.568∗ (0.337) -0.141 (0.097) 0.046 (0.280) 4.099∗∗ (1.940) 0.32 -0.499∗∗ (0.245) -0.088 (0.334) 0.11 0.11 968 Note: We present coefficients from ordinary least squares estimation. Robust standard errors for OLS and jackknifed standard errors for control function estimations are shown in parentheses. The specifications reported in Panel B also include the full set of controls shown in Panel A and generalized residuals calculated from the specifications in Columns 1 and 3 in Table C2. All specifications also include province fixed effects. 33 Electronic copy available at: https://ssrn.com/abstract=3938639 Table 8: Criminality, Education and Behavioral Preferences - Control Function Results Dependent variable: Prisoner Left-behind Parent(s) migrated (1) (2) (3) (4) 0.122∗∗ (0.056) 0.066 (0.056) 0.052 (0.050) 0.071 (0.055) -0.016∗∗∗ (0.002) 0.046 (0.050) 0.068 (0.055) -0.015∗∗∗ (0.002) -0.007∗∗ (0.003) 0.0007∗∗∗ (0.000) 0.000 (0.001) 0.049 (0.052) 0.057 (0.057) -0.012∗∗∗ (0.002) -0.007∗∗ (0.003) 0.0006∗∗ (0.000) -0.000 (0.001) 0.006 (0.008) -0.010 (0.008) -0.025∗∗ (0.010) -0.024∗∗ (0.010) 0.006 (0.008) 0.183 0.104 968 0.298 0.154 968 Years of education Risk-aversion Risk-aversion squared Patience Neurotic Open Agreeable Extroverted Conscientious Test of joint significance of the generalized residuals: 0.027 0.154 Test of α1 + α2 = 0 (p-value): 0.009 0.072 Observations 968 968 Notes: 1. We present marginal effects from weighted probit estimation where we weight by the ratio of the population size (of migrants/inmates) to the sample size (of migrants/inmates). 2. Jackknifed standard errors are shown in parentheses. 3. All specifications also include the full set of controls shown in Table 3 and generalized residuals. Column 1 uses generalized residuals calculated from the specification in Columns 1 and 3 in Table C2. Column 2 uses generalized residuals calculated from Columns 4 and 5 in Table C2. Column 3 uses generalized residuals calculated from Columns 6 and 7 in Table C2. Column 4 uses generalized residuals calculated from Columns 8 and 9 in Table C2. All specifications also include province fixed effects. 34 Electronic copy available at: https://ssrn.com/abstract=3938639 A A.1 Appendix: Experiments Information Sheet Participant Information Sheet Researcher: My name is Dandan Zhang and I am a lecturer at Peking University. I work with Prof. Xin Meng from the Australian National University and Prof. Lisa Cameron at Monash University on this project. Project Title: Migration and Crime General Outline of the Project: The current project is an extension of the Rural-Urban Migration in China and Indonesia project. During our previous five years of research in this area, one important issue stands out: many migrant workers are arrested for criminal activities. This sub-project will collect behavioral information to help us to understand the reasons for this. Participant Involvement: The project is voluntary and you can at any point, without any penalty, decline to take part or withdraw from the activities. In this study we will conduct interviews and economic experiments. Each participant will spend approximately one and half hours in a meeting room environment and participate in a number of experiments in the form of group games. The tasks you are required to perform are simple and undemanding activities. In addition, the participants will fill in a survey questionnaire, which will take around one hour, and an IQ test, which will last for 15 minutes. All participants will receive a payment for participating in the economic experiments. Precisely how much you will receive depends on the decisions you made, the decisions your partner made as well as some random elements. In particular, you will be askedto perform some activities, and one of the activities will be paid. Exactly which one will be determined by rolling a dice. The amount you received from the game will be directly transferred into individuals’ personal accounts. If any individual decides to withdraw from the project, any information provided prior to the time of withdrawal will be destroyed. Confidentiality: The information you provide will be kept confidential as far as the law allows. The research outcome will be reported in the form of group averages and no individual information will be identified or reported. The names of the participants will only appear on the first page of your answer sheet and they will later be removed and replaced with an assigned ID number. Data Storage: Any identifying information will be stored separately from the questionnaires and kept in a locked file cabinet at the Research School of Economics at the Australian National University, to which only the principal investigators have access. The data sets used for analysis will contain only the ID numbers and no identifying information. The data will be stored for 5 or more years from the date of the research publication. Queries and Concerns: Dr. Dandan Zhang China Center for Economic Research National School of Development Peking University Beijing, 100871, China Email: ddzhang@nsd.edu.cn Office: (+86)10-6275-9779 Ethics Committee Clearance: The ethical aspects of this research have been approved by the ANU Human Research Ethics Committee.If you have any concerns or complaints about how this research has been conducted, please contact: Ethics Manager, The ANU Human Research Ethics Committee, ,The Australian National University Telephone: +612 6125 3427; Email: Human.Ethics.Officer@anu.edu.au 35 Electronic copy available at: https://ssrn.com/abstract=3938639 A.2 Instruction for Experiment General Explanations for Participants Thank you for taking part in this study. As part of today’s experiment, we will be performing some tasks. The funding for this research has been provided by the Peking University and the Australian National University and any money that you end up with will be yours to keep. You will be paid for one of the tasks. The different parts are independent in the sense that the decisions you make in one will have no impact on your outcome in the other. At the end of all the tasks I will throw a dice in front of you to determine which task you will be paid for. We will give you a receipt for this money and it will be paid into your savings account. We are about to begin the first task. Please listen carefully. It is important that you understand the rules of the task properly. If you do not understand, you will not be able to participate effectively. A clear understanding of the instructions will help you make better decisions and increase your earnings. We will explain the task and go through some examples together. There is to be no talking or discussion of the task amongst you. There will be opportunities to ask questions to be sure that you understand how to perform each task. At any time whilst you are waiting during this experiment, please wait at your seat and do not do anything unless instructed by the experimenter. Also do not look at others responses at any time during this experiment. If at any time you decide that you are not happy with the task you have been invited to perform, you can decide not to participate. After we have completed all the tasks, I would like you to answer some questions about yourself. Please take your time and answer honestly and as accurately as possible. You will not be identified and your survey answers will only be used for this experiment and will only be used by the researcher(s) involved in this project. Finally, stapled behind this page is a slip of paper with your ID# on it. Please keep this page with the stapled ID# with you at all times. Do not show this ID# to anyone or allow it to be visible to anyone during or after this experiment. You will need to present this page with the stapled ID# to the cashier at the end of the experiment in order to receive your payment information. If you are ready, then we will proceed. Please turn the page and follow along with the experimenter. All decisions that you make today are recorded only by an anonymous subject number and will only be used for research purposes. Your decisions will remain completely anonymous. 36 Electronic copy available at: https://ssrn.com/abstract=3938639 TASK #1 Instructions This task is played by pairs of individuals. Each pair is made up of a Player 1 and a Player 2. Each of you will play this task with someone. However, none of you will know exactly with whom you are playing. You will never find this out. Each Player 1 has 100 yuan. No money will be given at this point. All actual payments will be decided at the end of the experiment if this task is chosen as the one that you will be paid for. Player 1 must decide how to divide this money between himself and Player 2. Player 1 must allocate between 0 yuan and the total 100 yuan to Player 2. Player 2 is then informed about Player 1’s decision and gets to decide whether to accept or reject the offer. If Player 2 accepts the offer, Player 2 gets whatever Player 1 allocates to him, and Player 1 takes home whatever he does not allocate to Player 2. If Player 2 rejects Player 1’s offer, then both players get $0. Let’s go through an example: Imagine that Player 1 chooses to allocate 50 yuan to Player 2. If Player 2 accepts the offer, then, Player 2 will get 50 yuan. Player 1 will get 50 yuan (100 yuan minus 50 yuan equals 50 yuan). If, however, Player 2 rejects the offer, both Player 2 and Player 1 will get 0 yuan. Note that this is an example only. The actual decision is up to you. Each of you will play as both Player 1 and Player 2 in this task. Each of you will be paired with two different people. In one pair you will be Player 1 and in the other pair you will be Player 2. So you will play this task once as Player 1 and once as Player 2. The important thing to remember is that you will NOT be paired with the same person twice and you will always remain anonymous to each other. No-one will be told who they are paired with. We will hand out all forms that you will record your decisions on throughout this experiment in envelopes to ensure this. If this task is chosen for payment, I will then toss a coin to determine which pairing you will be paid for. So for any given toss of the coin, half of you will go home with what you kept as Player 1, half of you will go home with what the Player 1s have given you. Are there any questions? If you are ready, we will proceed. You will write your decision on the form provided. Please turn over the page and look at the form that you will record your decision on. There is an example question and a table for you to record your decision. Please complete the example question first and then fill in Boxes A and B of the table. Once done, please place your form back into the envelope, raise your hand and we will collect the form from you. Electronic copy available at: https://ssrn.com/abstract=3938639 For experimenter use only Player ID #: ____________________ Paired Player ID #: _______________ Form for Recording Decisions for Task #1 Before you fill out this form, please complete the example below: 1. Say you are Player 1 and you have 100 yuan. You choose to give 60 yuan to Player 2. If Player 2 accepts the offer how much will you have and how much will Player 2 have? Player 1(yourself):_______________ Player 2:________________ If Player 2 rejects the offer how much will you have and how much will Player 2 have? Player 1(yourself):_______________ Player 2:________________ 2. When you have completed the example above, please enter the amount, in dollars, that you wish to keep and the amount that you wish to give to Player 2 in the table below. Total amount A Amount I wish to keep B Amount I wish to send to anonymous Player 2 100 yuan Electronic copy available at: https://ssrn.com/abstract=3938639 TASK #2 Instructions We are about to begin the 3rd task. How much you will be paid in this task depends on your own decision and your luck. No money will be given at this point. All actual payments will be decided at the end of the experiment as to whether this task will be chosen as the one that you will be paid for. Please listen carefully to the instructions. In this task, you need to answer 11 questions. For each question, you are given two choices, Choice A and Choice B. You can choose one of them. Let’s have a look at these questions. Choice A 1 45 Yuan for sure Choice B 60 if you roll 1,2,3 0 if you roll 4,5,6 I 2 45 Yuan for sure 75 if you roll 1,2,3 0 if you roll 4,5,6 3 45 Yuan for sure 90 if you roll 1,2,3 0 if you roll 4,5,6 4 45 Yuan for sure 105 if you roll 1,2,3 0 if you roll 4,5,6 5 45 Yuan for sure 120 if you roll 1,2,3 0 if you roll 4,5,6 6 45 Yuan for sure 135 if you roll 1,2,3 0 if you roll 4,5,6 7 45 Yuan for sure 150 if you roll 1,2,3 0 if you roll 4,5,6 8 45 Yuan for sure 165 if you roll 1,2,3 0 if you roll 4,5,6 9 45 Yuan for sure 180 if you roll 1,2,3 0 if you roll 4,5,6 10 45 Yuan for sure 195 if you roll 1,2,3 0 if you roll 4,5,6 11 45 Yuan for sure 210 if you roll 1,2,3 0 if you roll 4,5,6 Electronic copy available at: https://ssrn.com/abstract=3938639 There are two important rules in your choice you need to take notice of: First, you cannot choose Choice B first and then switch in subsequent questions to choice A. Second, you cannot switch twice from Choice A to Choice B and then back to Choice A. You can choose all A, or all B, or switching from A to B once. When you finish answering all 11 questions, we will choose one person from the group to come up and pick one ball from this bag, which has 11 balls, all the same size but each has a different number on it. The number on the ball which is picked by that person will be the question for which we will pay you according to the answer you are given to that question. For example, if number 10 were chosen, we will ask another person from the group to roll a dice to see if the choice is 1, 2, or 3; or 4, 5, or 6. Once we made these decisions, we can decide how much you will get paid for this task if this task is chosen at the end of the games. For example, if your answer to question number 10 was A, you will be paid 45 yuan. Otherwise, if your answer was B, then we will pay you 195 yuan if the number on the dice was 1, 2, or 3 and 0 yuan if the number on the dice was 4, 5, or 6. Do you have any questions? If you are ready, we will proceed. Please answer the 11 questions on the form in front of you. I will then collect the forms. Then, we will choose somebody to select the question number and another person to roll the dice. Electronic copy available at: https://ssrn.com/abstract=3938639 Player ID #: __________________________ Task 2 Answer Sheet Choice A 1 45 Yuan for sure Choice B 60 if you roll 1,2,3 0 if you roll 4,5,6 I 2 45 Yuan for sure 75 if you roll 1,2,3 0 if you roll 4,5,6 3 45 Yuan for sure 90 if you roll 1,2,3 0 if you roll 4,5,6 4 45 Yuan for sure 105 if you roll 1,2,3 0 if you roll 4,5,6 5 45 Yuan for sure 120 if you roll 1,2,3 0 if you roll 4,5,6 6 45 Yuan for sure 135 if you roll 1,2,3 0 if you roll 4,5,6 7 45 Yuan for sure 150 if you roll 1,2,3 0 if you roll 4,5,6 8 45 Yuan for sure 165 if you roll 1,2,3 0 if you roll 4,5,6 9 45 Yuan for sure 180 if you roll 1,2,3 0 if you roll 4,5,6 10 45 Yuan for sure 195 if you roll 1,2,3 0 if you roll 4,5,6 11 45 Yuan for sure 210 if you roll 1,2,3 0 if you roll 4,5,6 Electronic copy available at: https://ssrn.com/abstract=3938639 TASK #3 Instructions We are about to begin the 2nd task. Please listen carefully to the instructions. This task is performed by pairs of individuals. Each pair is made up of a Player A and a Player B. Each Player A has 50 yuan. No money will be given at this point. All actual payments will be decided at the end of the experiment if this task is chosen as the one that you will be paid for. Each Player A will have the opportunity to keep all of 50 yuan to himself or allocate some or all of it to a Player B. However, each yuan that Player A sends to Player B will be tripled by the experimenter and given to player B. Player B will then have an opportunity to keep all of the money sent to him from Player A or to send some or all of it back to Player A. This time the money will not be tripled again. The experiment ends at this point. Player B takes home whatever money that he/she does not give back to Player A. Player A takes home whatever he/she did not give to Player B and whatever money Player B gave back to him. Here are 2 examples of what could happen: 1) Say Player A gives Player B 25 yuan. This will be tripled and it will be 75 yuan when it reaches Player B. Then Player B sends back to Player A, say, 35 yuan. Then Player A will have 60 yuan (50 yuan minus the 25 yuan sent to Player B plus the 35 yuan sent back by Player B). Player B will have 40 yuan (75 yuan minus the 35 yuan sent back to Player A). 2) Say Player A gives Player B 40 yuan. This will be tripled and it will be 120 yuan when it reaches Player B. Then Player B sends back to Player A 60 yuan. Then Player A will have 70 yuan (50 yuan minus the 40 yuan sent to Player B plus the 60 yuan sent back by Player B). Player B will have 60 yuan (120 yuan minus the 60 yuan sent back to Player A). Note that these are only examples. The actual decisions are up to you. Each of you will play as both Player A and Player B in this task. Each of you will be paired with two different people in the other prison cell. (Note that the pairings in Task 2 are different from the pairings in Task 1.) In one pair you will be Player A and in the other pair you will be Player B. So you will play this task once as Player A and once as Player B. The important thing to remember is that you will NOT be paired with the same person twice and you will always remain anonymous to each other. No-one will be told who they are paired with. If this task is chosen for payment, I will then toss a coin to determine which pairing you will be paid for. So for any given toss of the coin, half of you will go home with what you kept as Player A, half of you will go home with what the Player As have given you. Are there any questions? If you are ready, we will proceed. You will convey your decisions using the form provided. Please turn over the page and look at the form that you will record your decision on. I will read through the form first. Please do not write anything until instructed to. Electronic copy available at: https://ssrn.com/abstract=3938639 For experimenter use only Player ID #: ____________________ Paired Player ID #: Form for Recording Decisions for Task #3 Part A Before you fill out this form, please complete the example below: 1. You are Player A and you have 50 yuan. You choose to give 40 yuan to Player B. How much will Player B have? $_______ x_______ =____________ Player B decides to send 20 yuan back. How much will you have in total and how much will Player B have in total? Player A:$_____ - $_____+$_____= _____ Player B:$_____-$_____ = _____ When you have completed the example above, please fill out Boxes A, B and C of the table below. When you have made your decision as Player A, your task as Player A is done at this point. Once you have completed Boxes A, B and C, raise your hand and I will collect the form from you. You will be informed of how much the Player B gave back to you at the end of the experiment when you collect your payment. 2. Your decision as Player A Starting amount A Amount I wish to keep as Player A B Amount I wish to send to anonymous Player B C Amount that Player B will receive (Box B x 3) 50 yuan When you have completed Part A, please read the instructions for Part B over page. Electronic copy available at: https://ssrn.com/abstract=3938639 Form for Recording Decisions for Task #3 Part B Recall that you will also be a Player B in another pairing. I will record how much Player A in this pairing has sent to you in Box D when I collect your forms after you fill in Boxes A, B and C. The amount in Box D will already be tripled. I will then return the form to you and you will then decide how much money to keep and how much to send back to Player A. You will need to fill in Boxes E and F. When you have read the above paragraph, place your form for Task #2 into the envelope, raise your hand and I will collect your form from you. 3. Your decision as Player B D Amount received from Player A (already tripled) E Amount I wish to keep F Amount I wish to send back to Player A Once you have completed boxes E and F, your task is done. Please place this form into your envelope, raise your hand, and I will collect the form from you. Two additional tasks (which are not used in this paper and which we do not explain here) were then conducted for use in another paper. The experimental session was then concluded, payments were determined and participants paid. Electronic copy available at: https://ssrn.com/abstract=3938639 A.3 Time Preference Choices G. Time preferences Suppose that you can get some money in two ways. Choice A gets you 1000 Yuan after one month, and Choice B gets you more money but after seven months. Mark your choice for the 11 situations listed below. 1 A To get 1000 Yuan after one month B To get 1025 Yuan after seven months 2 To get 1000 Yuan after one month To get 1075 Yuan after seven months 3 To get 1000 Yuan after one month To get 1125 Yuan after seven months 4 To get 1000 Yuan after one month To get 1175 Yuan after seven months 5 To get 1000 Yuan after one month To get 1225 Yuan after seven months 6 To get 1000 Yuan after one month To get 1275 Yuan after seven months 7 To get 1000 Yuan after one month To get 1325 Yuan after seven months 8 To get 1000 Yuan after one month To get 1375 Yuan after seven months 9 To get 1000 Yuan after one month To get 1425 Yuan after seven months 10 To get 1000 Yuan after one month To get 1475 Yuan after seven months 11 To get 1000 Yuan after one month To get 1525 Yuan after seven months 45 Electronic copy available at: https://ssrn.com/abstract=3938639 B Appendix: Weight Generation In 2012 there were 767,130,000 migrant residents of Shenzhen (with non-local hukou), Social and Economic Development Statistical Report. Assuming that about 90% of these are rural-urban migrants and about half are male, we estimate there are 3.45 million rural-urban male migrants in Shenzhen. The same data source tells us that there were 50,315 arrests made in Shenzhen in 2012/2013. Using the prison administration data we calculate a prison inflow ratio (equal to the inflow in 2012/2013 divided by the total number of prisoners in this period) of 0.55. Dividing the total number of arrests in Shenzhen in 2012/2013 by this inflow rate we obtain an estimate of the rural-urban migrant prison population in the city of 50,315/0.55=91,481. In 2012 94.17% of prisoners were male, China Statistical Yearbook (2004-2013). So our estimate of the male prison population is 86,148. These numbers are used to calculate the poulation weights used in this study. 46 Electronic copy available at: https://ssrn.com/abstract=3938639 C Appendix: Tables Table C1: Marginal Effects from Weighted Logit and Unweighted (Corrected) Logit Dependent Variable: Prisoner Left-behind Parent(s) migrated Age Married Cognitive ability score Siblings Only child Paternal education Maternal education Han ethnicity Parent had been in jail Weighted Logit Results (1) (2) 0.026∗∗∗ 0.025∗∗∗ (0.007) (0.008) -0.007∗ 0.004 (0.004) (0.005) 0.001 (0.000) -0.020∗∗∗ (0.006) -0.005∗∗∗ (0.001) -0.000 (0.002) -0.000 (0.008) -0.001 (0.001) -0.003∗∗∗ (0.001) -0.025** (0.012) 0.017 (0.017) Regional Variables: ln(GDP capita) 0.017∗∗∗ (0.006) 0.118∗∗ (0.058) 0.079∗∗∗ (0.021) 0.167∗∗∗ (0.046) -1.503∗∗∗ (0.358) 968 Gini coefficient Ethnic minority share Sex ratio Teacher/student ratio N 968 Unweighted (Corrected) Logit Results (3) (4) 0.004∗∗∗ 0.007∗∗∗ (0.001) (0.002) -0.001∗ 0.000 (0.001) (0.001) 0.000 (0.000) -0.005∗∗∗ (0.001) -0.001∗∗∗ (0.000) 0.000 (0.001) -0.001 (0.002) -0.000 (0.000) -0.001∗∗∗ (0.000) -0.005* (0.003) 0.005 (0.007) 968 0.002 (0.001) 0.017 (0.013) 0.017∗∗∗ (0.005) 0.042∗∗∗ (0.013) -0.205∗∗ (0.083) 968 Note: Columns 1 and 2 present marginal effects from weighted probit estimation where we weight by the ratio of the population size (of migrants/inmates) to the sample size (of migrants/inmates). Columns 3 and 4 present marginal effects from logistic regressions where we correct for choice-based sampling following King and Zeng (2001). All specifications include province fixed effects. Standard errors are shown in parentheses. 47 Electronic copy available at: https://ssrn.com/abstract=3938639 48 Electronic copy available at: https://ssrn.com/abstract=3938639 968 0.004 968 0.001 (2) Length of absence 20.182∗∗ (10.253) 44.511∗∗ (21.505) 0.181 968 (3) Parent(s) migrated -0.197 (0.552) 2.603∗ (1.409) 0.004 968 (4) Leftbehind 1.841∗∗ (0.839) 3.337∗ (1.756) -0.070∗∗∗ (0.026) 0.181 968 (5) Parent(s) migrated -0.197 (0.552) 2.603∗ (1.409) For specifications also including education 0.012 968 (6) Leftbehind 1.760∗∗ (0.849) 2.885 (1.835) -0.069∗∗∗ (0.026) -0.112∗ (0.063) 0.008 (0.005) -0.009 (0.013) 0.275 968 (7) Parent(s) migrated -0.208 (0.553) 2.299 (1.432) -0.016 (0.018) -0.031 (0.049) 0.001 (0.004) -0.001 (0.010) For specifications also including education and behavioral preferences 0.010 (8) Leftbehind 1.838∗∗ (0.838) 2.797 (1.852) -0.065∗∗ (0.026) -0.114∗ (0.063) 0.008∗ (0.005) -0.009 (0.013) 0.088 (0.151) -0.015 (0.177) 0.006 (0.182) -0.101 (0.183) -0.022 (0.177) 968 0.228 (9) Parent(s) migrated -0.260 (0.563) 2.489∗ (1.450) -0.020 (0.018) -0.034 (0.050) 0.002 (0.004) -0.001 (0.011) -0.035 (0.110) -0.029 (0.136) 0.165 (0.126) 0.163 (0.130) -0.118 (0.127) 968 For specifications also including education, behavioral preferences and personality traits Note: All columns except for Column 2 report coefficients and standard errors from probit estimation. Column 2 reports coefficients and standard errors from interval regression. The county left-behind rate and county out-migration rate are the instrumental variables. All standard errors are clustered by county of birth. All specifications also included all of the control variables used in Table 3 and province fixed effects. Standard errors are shown in parentheses. Observations Test of joint significance of instruments (p-value): Conscientious Extroverted Agreeable Openness Neurotic Patience Risk aversion squared Risk aversion Years of education County out-migration rate County left-behind rate (1) Leftbehind 1.733∗∗ (0.830) 4.102∗∗ (1.738) For the base specification Table C2: First Stage Regressions for Control Function Estimation Table C3: Selected Marginal Effects for the Sample of Individuals whose Parents Ever Migrated Dependent Variable: Prisoner (0/1) (1) A. Not allowing for Endogeneity Left-behind (2) 0.025∗∗∗ (0.008) 0.003∗∗∗ (0.001) Length of Parental Absence B. Control Function Results Left-behind 0.128∗ (0.070) Length of Parental Absence Test of jt sig. of generalized residuals (p-value) F-Test of weak IVs: Observations 0.035 12.75 412 0.004 (0.004) 0.50 11.41 412 Note: 1. The results presented here are from the estimation of Equation 1 for a sample of individuals whose parents ever migrated. The full results are available upon request from the authors. 2. We present marginal effects from weighted probits where we weight by the ratio of the population size (of migrants/inmates) to the sample size (of migrants/inmates). All specifications also include the control variables and province fixed effects. 3. Robust standard errors are shown in parentheses. Panel B reports jackknifed standard errors. 4. The results used to calculate generalized residuals for Panel B are available upon request from the authors. 49 Electronic copy available at: https://ssrn.com/abstract=3938639 Table C4: Effect of Being Left-Behind on Additional Behavioral Preferences (1) Dependent variables: Estimation type: Left-behind Parent(s) migrated Age Married Cognitive ability score Siblings Only child Paternal education Maternal education Han ethnicity Parent had been in jail Regional Variables: Ln(GDP/capita) Gini coefficient Ethnic minority share Sex ratio Teacher-Student Ratio Test of α1 + α2 = 0 (p-value): Observations (2) Trust Game Trust Trust-worthiness (% sent) ( % returned) (3) (4) Ultimatum Game Altruism Punishment (% sent) (0/1) tobit 0.006 (0.005) 0.000 (0.003) 0.000 (0.000) -0.006∗ (0.003) 0.001∗∗ (0.001) 0.000 (0.001) 0.004 (0.006) -0.000 (0.000) -0.000 (0.000) -0.005 (0.005) 0.005 (0.009) tobit 0.010∗ (0.006) -0.005 (0.003) 0.001∗∗∗ (0.000) -0.005∗ (0.003) -0.001∗∗∗ (0.001) 0.002 (0.001) 0.005 (0.006) 0.000 (0.000) -0.000 (0.000) -0.014∗ (0.007) -0.005 (0.006) tobit 0.001 (0.000) 0.000 (0.000) 0.000 (0.000) -0.000 (0.000) -0.000 (0.000) 0.000 (0.000) -0.000 (0.000) -0.000 (0.000) -0.000 (0.000) -0.000 (0.001) -0.000 (0.001) probit 0.008 (0.039) 0.023 (0.025) -0.006∗∗∗ (0.002) 0.076∗∗∗ (0.027) 0.011∗∗∗ (0.004) 0.002 (0.009) -0.003 (0.046) 0.001 (0.003) 0.002 (0.003) -0.065 (0.050) 0.096 (0.088) -0.000 (0.003) -0.046 (0.031) -0.001 (0.008) 0.011 (0.022) -0.208 (0.173) 0.218 964 -0.002 (0.003) -0.002 (0.033) -0.005 (0.011) 0.049∗ (0.028) -0.312 (0.193) 0.293 951 0.000 (0.000) -0.001 (0.003) 0.001 (0.001) 0.002 (0.002) -0.009 (0.014) 0.108 968 -0.002 (0.026) -0.132 (0.275) -0.088 (0.080) 0.024 (0.205) 0.567 (1.543) 0.423 968 Note: 1. We present marginal effects from probits and tobit estimation. 2. Robust standard errors are shown in parentheses. 3. All specifications also include province fixed effects. 50 Electronic copy available at: https://ssrn.com/abstract=3938639 D Appendix: Mediation Analysis The median analysis closely follows that of Heckman et al. (2013) and Heckman and Pinto (2015). Details of the model underlying the mediation analysis can be found in these references. Here we explain the steps underlying the analysis. In the notation below for simplicity we suppress the individual index, i, and ignore the existence of control variables in the regressions. Our analysis uses estimates from the model estimated in Column 3 of Table 8 where P risoneri is regressed on the treatment variables (LB and M ig P ) and the mediating variables (education, riskiest and patience); the regressions of each of the mediating variables on the treatment variables reported in Column 3 of Table 5 for education, Column 4 of Table 6 for riskiest and Column 6 of Table 6 for patience. If we notate these models as follows: P risoner = τ0 + τ1 LB + τ2 M ig P + αE Education + αR Riskiest + αP P atience + , (8) E E P E Education = µE 0 + µ1 LB + µ2 M ig + , (9) R R P R Riskiest = µR 0 + µ1 LB + µ2 M ig + , (10) P atience = µP0 + µP1 LB + µP2 M ig P + P , (11) then, as demonstrated in the above references, and assuming an underlying linear model between the estimated marginal effects, the total effect of being left-behind (and parental migration) can be expressed as: E[P risoner1 − P risoner0 ] = X X τk + X k∈{1,2} j∈{E,R,P } m∈{1,2} | {z } | direct effect µjm αj , {z indirect effect (12) } where P risoner1 is the probability of being incarcerated if left-behind and P risoner0 is the probability of being incarcerated if not left-behind; τk capture the direct effects of the treatment variables (left-behind and parental migration) on the probability of being incarcerated; µjm are the effect of the treatment variables on the mediating variables; and αj are the effects of the mediating variables on the probability of being incarcerated. The first element in equation 12 thus is the total direct effect of being left-behind (and parental migration) and the second expression is the sum of the indirect effects of being left-behind which operate through the mediating variables. 51 Electronic copy available at: https://ssrn.com/abstract=3938639