Distortion of Justice How the Inability to Pay Bail Affects Case Outcomes, Stevenson, 2016
Download original document:
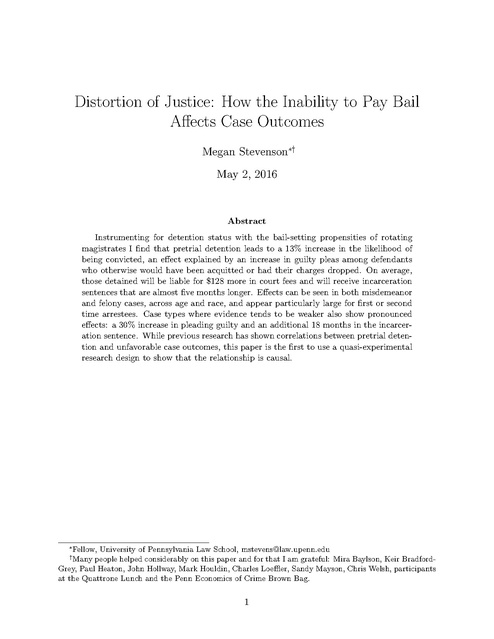
Document text
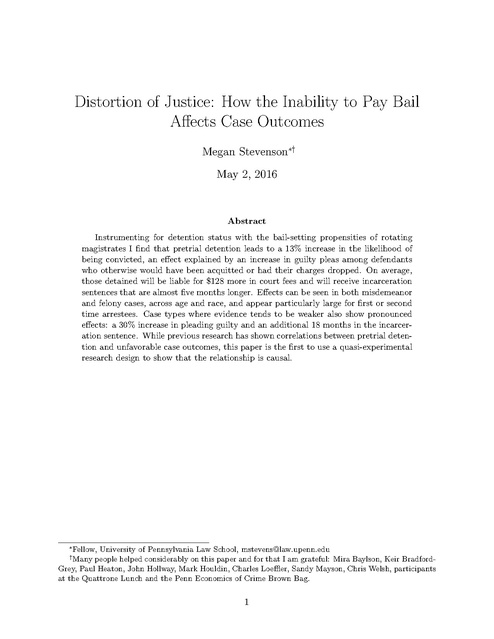
Document text
This text is machine-read, and may contain errors. Check the original document to verify accuracy.
Distortion of Justice: How the Inability to Pay Bail Affects Case Outcomes Megan Stevenson∗† May 2, 2016 Abstract Instrumenting for detention status with the bail-setting propensities of rotating magistrates I find that pretrial detention leads to a 13% increase in the likelihood of being convicted, an effect explained by an increase in guilty pleas among defendants who otherwise would have been acquitted or had their charges dropped. On average, those detained will be liable for $128 more in court fees and will receive incarceration sentences that are almost five months longer. Effects can be seen in both misdemeanor and felony cases, across age and race, and appear particularly large for first or second time arrestees. Case types where evidence tends to be weaker also show pronounced effects: a 30% increase in pleading guilty and an additional 18 months in the incarceration sentence. While previous research has shown correlations between pretrial detention and unfavorable case outcomes, this paper is the first to use a quasi-experimental research design to show that the relationship is causal. ∗ Fellow, University of Pennsylvania Law School, mstevens@law.upenn.edu Many people helped considerably on this paper and for that I am grateful: Mira Baylson, Keir BradfordGrey, Paul Heaton, John Hollway, Mark Houldin, Charles Loeffler, Sandy Mayson, Chris Welsh, participants at the Quattrone Lunch and the Penn Economics of Crime Brown Bag. † 1 I have had the ‘you can wait it out or take the deal and get out’ conversation with way too many clients. -a public defender, Philadelphia 1 Introduction The use of money bail has long been contentious, as it implies that freedom is re- served for those who can afford to pay for it. According to Bureau of Justice Statistics, five out of six people detained before trial are there because they could not afford their bail (Cohen and Reaves, 2007). For some, bail was intentionally set at an unaffordable level to keep them behind bars. But many have bail set at amounts that would be affordable for the middle or upper-middle class but are simply beyond the reach of the poor. In Philadelphia, the site of this study, only 51% of those who were assigned a bail amount less than or equal to $500 were able to pay the $50 deposit required for release within the first three days, and 25% remained in jail at the time of disposition. While the loss of freedom during the pretrial period is a serious concern, there are indirect consequences of pretrial detention which are potentially just as serious. If detention increases the likelihood that the defendant will plead guilty although innocent, accept an excessively-punitive plea deal, or lose at trial because detention impaired her ability to mount a successful defense, then the socio-economic disparities induced by a money bail system extend beyond the pretrial period. Right now there is a wave of momentum in bail reform that dwarfs any seen in decades. Media attention to the recent deaths of Kalief Browder and Sandra Bland and budget pressures imposed by the 11.4 million yearly admissions to local jails have spurred jurisdictions all over the country to reconsider long-standing practices.12 Yet despite all this activity, research on the pretrial period is limited. The work that does exist is almost entirely qualitative or correlational; the few experimental or quasiexperimental studies that have been conducted either did not examine case outcomes or did not report the results in a way that allowed for causal inference (Ares et al., 1963; Goldkamp, 1980; Abrams and Rohlfs, 2011). In this paper I present the first quasi-experimental evidence that pretrial detention increases the likelihood of being convicted, pleading guilty, being sentenced to incarceration, and being required to pay hundreds or thousands of dollars in court fees. In Philadelphia, the bail decision is made by one of six Arraignment Court Magistrates 1 In the last several years, pretrial reform has been committed to or implemented in New Jersey, Kentucky, Colorado, Maryland, New Mexico, Connecticut, Chicago, New York City. 26 cities are implementing new pretrial risk assessment regimes in partnership with the Laura and John Arnold Foundation and 20 cities are developing pretrial reform proposals with a $75 million fund from the MacArthur Foundation. 2 Many of the 11.4 million admissions will spend only short periods in jail. The point-in-time count of jail inmates is 750,000, two thirds of whom are awaiting trial. BJS estimates that 95% of jail growth since the year 2000 has come from pretrial detainees (Minton and Zeng, 2015). 2 who have broad discretion in setting bail. These magistrates work on a rotating schedule which ensures that over time, the sample of defendants seen by each magistrate will be statistically near identical. By determining the bail amount, the magistrate has influence over whether a defendant is detained pretrial, but is unlikely to affect case outcomes through any other channel. All of the other activities that take place in the bail hearing are pro-forma and the schedules of the magistrates do not correlate with the schedules of the judges or attorneys that are influential later in the criminal proceedings. Thus the bail preferences of the magistrate who presides over the bail hearing provide an exogenous shock to the likelihood of being detained pretrial: a suitable instrument with which to identify the causal impact of pretrial detention on case outcomes. The data used in this analysis covers all criminal cases originated in Philadelphia between September 2006 and February 2013. The sample size is 331,615 criminal cases with eight total bail magistrates. I use a jackknife instrumental variables technique which, in my preferred specification, allows the preferences of the magistrates to vary over time and according to offense, criminal history, race, and gender of the defendant (Mueller-Smith, 2015). This allows me to exploit considerable within-magistrate variance in detention rates. For example, a defendant with a shoplifting charge is 30 percentage points more likely to be detained if she is seen by the magistrate who is most strict on shoplifting charges instead of the one who’s most lenient. However the magistrate that is most lenient on shoplifting charges is most strict on robbery charges, the magistrate that is most lenient on robbery is strict on drug possession, and so forth. Using variation in the propensity to detain both within and across the magistrates, I estimate that pretrial detention leads to a 6.6 percentage point increase in the likelihood of being convicted on at least one charge, over a mean 50% conviction rate. The effect on conviction (being found guilty either through plea or at trial) is almost entirely explained by a 5.3 percentage point increase in the likelihood of pleading guilty among those who would otherwise have been acquitted, diverted, or had their charges dropped. Those detained will be liable for $128 more in non-bail court fees and will be sentenced to an additional 140 days of incarceration.3 Effects appear to be present in both misdemeanors and felonies, although the effects are much more precisely estimated in the former. Those detained on a misdemeanor charge will be 8 percentage points more likely to be convicted and 8 percentage points more likely to receive a sentence of incarceration. Effects are similar in magnitude for white and black defendants, don’t vary substantially according to defendant’s age, but appear largest for first or second time arrestees. For those with very limited experience 3 Average court fees for the convicted are $775 and those who receive a prison sentence will serve almost two years on average before being eligible for parole. 3 in the criminal justice system, pretrial detention leads to a 17 percentage point increase in the likelihood of being convicted. I divide the sample according to the strength of the evidence that tends to be available in different crime types. My hypothesis is that extra-legal factors such as detention will be more influential among cases where the facts are in dispute than among cases where the evidence is difficult to contest. I find that effects among ‘strongevidence’ crimes (DUI, drugs, illegal firearms) are generally small and not statistically significant in the IV regressions, but effects among ‘weak-evidence’ crimes (assault, vandalism, burglary) are more pronounced. Those detained on a weak-evidence charge are 7 percentage points more likely to plead guilty and will receive an additional 18 months of incarceration. Since weak-evidence crimes tend to have higher rates of wrongful convictions, these findings are consistent with the claim that pretrial detention increases the likelihood that innocent people will plead guilty.4 Previous studies using multivariate regression techniques usually show that pretrial detention is correlated with unfavorable case outcomes even after controlling for a number of defendant and case characteristics (Ares et al., 1963; Rankin, 1964; Goldkamp, 1980; Williams, 2003; Free, 2004; Phillips, 2007, 2008; Tartaro and Sedelmaier, 2009; Sacks and Ackerman, 2012; Oleson et al., 2014; Heaton et al., 2016). Many have found very large correlations. An often-cited study by the Arnold Foundation concludes that low risk defendants who are detained throughout the pretrial period are 5.41 times more likely to be convicted and sentenced to jail than those who are released (Lowenkamp et al., 2013). They control for offense within eight broad categories as well as basic demographics and criminal history measures, but variations in charge severity, strength of the evidence, quality of the representation, wealth of the defendant, and a variety of other unobservable differences may have biased the results. The IV estimates I present here are considerably lower than most previous estimates. This may be due to omitted variable bias in previous research, differences in effect sizes across jurisdictions, or the fact that the IV estimates capture the effect of pretrial detention only for those on the margins of being detained. I also conduct OLS regressions of case outcomes on pretrial detention, controlling for narrowly defined offense categories as well as many other defendant and case characteristics. The OLS results do not differ substantially from the IV results, suggesting that researchers who are able to control for narrowly defined offense categories as well as a variety of criminal history and demographic variables may be able to produce reasonable estimates of the effect of pretrial detention on case outcomes even in the absence of a natural 4 A forthcoming study by Charles Loeffler and Jordan Hyatt examines wrongful convictions through the use of anonymous surveys of prison inmates. Their preliminary findings suggest that the rate of wrongful convictions is higher among assaultive crimes and lower among DUIs and some drug crimes. 4 experiment.5 The impact that pretrial detention has on case outcomes could come through a variety of channels. A common claim is that people plead guilty just to get out of jail (Bibas, 2004). While this is a reasonable mechanism for misdemeanor cases, effects are also seen in much more serious charges, where it is unlikely that a defendant would be able to avoid a prison sentence. It may be that since some of the disruptions of incarceration have already occurred – loss of job/housing, the initial adjustment to life behind bars – the incentives to fight the charges are lower. Jail may affect optimism about the likelihood of winning the case, or may affect risk preferences in such a way that the certainty of a plea deal seems preferable to the gamble of a trial.6 Detention also impairs the ability to gather exculpatory evidence, makes confidential communication with attorneys more difficult, and limits opportunities to impress the judge with gestures of remorse or improvement (taking an anger management course, entering rehab, etc.) (Goldkamp, 1980). Detained defendants are likely to attend pretrial court appearances in handcuffs and/or prison garb, creating superficial impressions of criminality. Furthermore, if a defendant must await trial behind bars he may be reluctant to employ legal strategies that involve delay. Whereas a released defendant may file continuances in the hopes that the prosecution’s witnesses will fail to appear, memories will blur, or charges eventually get dropped, a detained defendant pays a much steeper price for such a strategy. More nefariously, those detained have less opportunity to coerce witnesses, destroy evidence or otherwise impede the investigation (Allen and Laudan, 2008). While pretrial detention may increase wrongful conviction, pretrial release may decrease the likelihood of successfully prosecuting the truly guilty. While this paper does not argue for or against the use of pretrial detention, it shows that detention has significant downstream consequences. Being found guilty in the court of law comes with hefty court fees, periods of incarceration or probation, potential disenfranchisement, a criminal record, and challenges securing future employment, school loans, or housing (Uggen et al., 2012; SHRM, 2012; Thacher, 2008; ONDC, n.d.). If wealth or race influence the likelihood of being detained pretrial – and both previous research as well as evidence presented in this paper suggest that they do – then pretrial detention exacerbates socio-economic inequalities in the criminal justice system (Schlesinger, 2005; Wooldredge, 2012). In Section 2 I give a brief overview of the pretrial process, in Section 3 I describe 5 In forthcoming work with Paul Heaton and Sandra Mayson (Heaton et al., 2016) we use OLS methods with extensive controls to estimate the impact pretrial detention has on case outcomes in Harris County, Texas. In general, results are similar to those found in Philadelphia; effect sizes are slightly larger but this may be explained by institutional differences across the different jurisdictions. 6 Detention may change the reference point so that freedom is seen as a gain instead of incarceration being seen as a loss. Prospect theory would thus predict a shift towards more risk averse decisions (Daniel Kahneman, 1979). 5 the natural experiment, and in Section 4 I discuss the data and provide descriptive statistics and graphs. Section 5 tests the identifying assumption and Section 6 discusses the empirical strategy and shows a visual representation of the first and second stage. Section 7 presents the results for the full sample and Section 8 shows results for various subgroups. Section 9 provides robustness tests and shows how results vary with the number of days detained. Section 10 concludes. 2 Bail, pretrial detention, and an overview of criminal proceedings Pretrial detention is the act of keeping a defendant confined during the period between arrest and disposition for the purposes of ensuring their appearance in court and/or preventing them from committing another crime. The vast majority of jurisdictions use a money bail system to govern whether or not a defendant is detained. In such a system a judge or a magistrate determines the amount of the bail required for release and the defendant is only released if she pays that amount and agrees to certain behavioral conditions. In some cases the defendant will be released without having to pay anything, in others (usually only the most serious cases) she will be denied bail and must remain detained. While the defendant is liable for the full amount of the bail bond if she fails to appear at court or commits another crime during the pretrial period, she usually does not need to pay the full amount in order to secure release. In many jurisdictions she will borrow this sum from a bail bondsman, who charges a fee and holds cash or valuables as collateral. In some jurisdictions, Philadelphia included, the courts act as a bail bondsman and will release the defendant after the payment of a deposit. Bail hearings are generally quite brief – in Philadelphia most last only a minute or two – and often do not have any lawyers present. After the bail hearing there are a series of pretrial court appearances that defendants must attend. Although the exact procedure varies across jurisdictions these usually include at least an arraignment (where formal charges are filed) and some sort of preliminary hearing or pretrial conference (where the case is discussed and plea deals can be negotiated). Plea bargaining usually begins around the time of arraignment and can continue throughout the criminal proceedings. In some jurisdictions, like New York City, the arraignment happens simultaneous to the bail hearing and it is not uncommon to strike a plea deal at this first appearance. In other jurisdictions, such as New Orleans, arraignments often do not happen until six months after the bail hearing and a defendant who is unable to make bail must wait until then to file a plea. In Philadelphia, arraignments usually happen within a month of the bail hearing. 6 Plea negotiation is a process in which the defendant receives reduced charges or shorter sentences in return for pleading guilty and waiving her right to a trial. Since defendants often face severe sentences if found guilty at trial, the incentives to plead are strong. It’s estimated that 90-95% of felony convictions are reached through a plea deal (Devers, 2011). Philadelphia differs from many other jurisdictions in its wide use of bench trials on felony cases. Since sentencing tends to be more lenient in bench trials than jury trials, this reduces the incentive to plead guilty.7 As such, only about 75% of felony convictions are reached through plea in Philadelphia. Trial by jury is not constitutionally required if the maximum incarceration sentence is less than six months, and the use of bench trials for misdemeanors, as is the custom in Philadelphia, is more common across jurisdictions. This paper shows that pretrial detention increases the likelihood that a defendant will plead guilty and will plead to less favorable terms. While there is little reason to believe this result is unique to Philadelphia, the magnitude of the effect is likely to differ across jurisdictions due to variations in the criminal justice proceedings. 3 The natural experiment Immediately after arrest, arrestees are brought to one of seven police stations around the city. There, the arrestee will be interviewed via videoconference by Pretrial Services. Pretrial Services collects information about various risk factors as well as financial information to determine eligibility for public defense. Using risk factors and the current charge, Pretrial Services will determine the arrestee’s place in a 4 by 10 grid of bail recommendations. These bail guidelines suggest a range of appropriate bail, but are only followed about 50% of the time (Shubik-Richards and Stemen, 2010). Once Pretrial Services has entered the bail recommendation and the financial information into the arrest report the arrestee is ready for her bail hearing. Once every four hours the magistrate will hold bail hearings for all arrestees on the ‘list’ (those who are ready to be seen). The bail hearing will be conducted over videoconference by the magistrate, with a representative from the district attorney’s office, a representative from the Philadelphia Defender Association (the local public defender), and a clerk also present. In general, none are attorneys. The magistrate makes the bail determination on the basis of information in the arrest report, the pretrial interview, criminal history, bail guidelines, and advocacy from the district attorney and public defender representatives. 7 In Philadelphia, a bench trial is the default for all but the most serious felonies. The right to a jury trial can be asserted upon request, but this is uncommon. While there is no formal mechanism that ensures that a bench trial will lead to better outcomes for the defendant than a jury trial, all defense attorneys interviewed assured me that this was the case. 7 There are four things that happen during the bail hearing: the magistrate will read the charges to the arrestee, inform her of her next court appearance, determine whether the arrestee will be granted a court-appointed defense attorney, and set the bail amount. The first two activities are formalities that ensure the defendant is aware of what she is being charged with and where her next court date is. Eligibility for public defense is determined by income. If the defendant is deemed eligible, she will be assigned either to the Defender Association, or to a private attorney who has been approved to accept court appointments by the City of Philadelphia. The default is to appoint the Defender Association; if procedural rules require the court to appoint an attorney outside of the Defender Association the magistrate’s clerk will appoint the attorney at the top of a rotating list of eligible attorneys known as a ‘wheel’.8 A typical bail hearing lasts only a minute or two and the magistrate has broad authority to set bail as she sees fit.9 Bail decisions fall into three categories: release with no payment required, cash bail or no bail.10 Those with cash bail will be required to pay a 10% deposit on the total bail amount in order to be released. After disposition, and assuming that the behavioral conditions of the pretrial period were met, 70% of this deposit will be returned. The City of Philadelphia retains 30% of the deposit, even if charges get dropped or the defendant is acquitted on all charges. Those who do not have the 10% deposit in cash can borrow this amount from a commercial bail bondsman, who will accept cars, houses, jewelry and other forms of collateral for their loan. If the defendant’s arrest occurred while she is already on parole, her parole officer may choose to file a detainer. If a detainer is filed she may not bail out until a judge removes the detainer.11 The research design uses variation in the propensity of the magistrates to assign affordable bail as an instrument for detention status. The validity of the instrument rests on several factors, including that the magistrate received is essentially random and that the instrument not affect outcomes through a channel other than pretrial detention. The following details help ease concerns along these lines. 8 If there are multiple codefendants, such that representing all of them would pose a conflict of interest, one defendant will be randomly selected to be served by the Defender Association and the others will receive a court-appointed attorney. For opaque historical reasons, four out of five defendants charged with murder will be represented by court-appointed attorneys and the fifth will be represented by the homicide division of the Defender Association (Anderson and Heaton, 2012). This decision is made by the order in which defendants are entered into the data system and the court-appointed attorney is chosen by a Municipal Court judge, not a magistrate. 9 If either the defense or the prosecution is unhappy with the decision they can make an appeal to a judge immediately after the bail hearing. However the bar is high for overturning the original bail decision so this is not very common. 10 Holding a defendant without bail is uncommon, although bail is sometimes set at prohibitively high rates. 11 The detainer hearing usually happens within a week of arrest. Detainer cases are evenly distributed across magistrates and should not bias the results. 8 Philadelphia employs six Arraignment Court Magistrates at a time, and one of the six will be on duty 24 hours a day, 7 days a week, including holidays. Each day is composed of three work shifts: graveyard (11:30 pm-7:30 am), morning (7:30 am-3:30 pm) and evening (3:30 pm-11:30 pm). Each magistrate will work for five days on a particular shift, take five days off, then do five days on the next shift, five days off, and so forth. For example, a magistrate may work the graveyard shift from January 1st to January 5th, have January 6th-10th off, then work the morning shift from January 11th-15th, have the 16th-20th off, do the evening shift from January 21st-25th, take the next five days off, and then start the cycle all over again. This rotation relieves concerns that certain magistrates set higher bail because they work during shifts which see higher-risk defendants. Over time, each magistrate will be scheduled to work a balanced number of weekends, graveyard shifts, and so forth. However the magistrates do not always work their appointed shifts; in fact, about 20% of the time there is a substitute (usually one of the other magistrates). To avoid potential confounds I instrument with the magistrate who was scheduled to work instead of the magistrate that actually worked. Furthermore, arrestees do not have latitude to strategically postpone their bail hearing to receive a more lenient magistrate. The process from arrest to bail hearing has been described as a conveyor belt: on average the time from arrest to the bail hearing is 17 hours and defendants are seen as soon as Pretrial Services notifies the Arraignment Court that they are ready (Clark et al., 2011). Thus the cases seen by each scheduled magistrate should be statistically very close to identical. I confirm this empirically in Section 6. Since the duties of the bail magistrate are so limited, there are few channels outside of the setting of bail through which the magistrate could affect outcomes. One concern would be a correlation between the schedules of the magistrates and the likelihood of receiving a particular judge, prosecutor or defense attorney later on in the criminal proceedings. However the peculiar schedule of the magistrates does not align with the schedule of any other actors in the criminal justice system. For one, this is because the other courts are not open on weekends. This is also because Philadelphia predominantly operates on a horizontal system, meaning that a different prosecutor handles each different stage of the criminal proceedings. Likewise, if the defendant is represented by the Defender Association (∼60% of the sample), she will have a different defense attorney at each stage.12 While attorneys often rotate duties, their rotations are based on a Monday-Friday work week and not the ‘five days on, five days off’ schedule of the magistrates. While eligibility for public defense is another potential channel through which the 12 The most serious cases are not handled horizontally, however the choice of attorney to handle these cases has nothing to do with the magistrate. 9 magistrate could affect outcomes, this is supposed to be a pro-forma action based on the income of the defendant. I am not able to see whether the defendant is deemed eligible, I only know the attorney type at the time of disposition. This is likely to be affected by detention status, as the decision to hire a private attorney is based both on having the money to pay one and expectations over case outcomes. While I cannot test directly whether magistrates vary in the rates at which they grant eligibility, I conduct robustness checks in which I control for the attorney type at the time of disposition. This has only a trivial impact on the results. 4 Data and descriptive statistics The data for this analysis comes from the court records of the Pennsylvania Unified Judicial System; PDF files of case dockets and criminal histories are publicly available online. The data covers all criminal cases which had a bail hearing between September 13, 2006 and February 18, 2013.13 Before September 13, 2006, Philadelphia used a different data management system with much lower data quality. I do not look at cases which began after February 18, 2013 both because I wanted to leave ample time for all cases to resolve and because one of the magistrates was replaced by a new one on that date. Figure 1a shows a histogram of the number of days defendants are detained before disposition, conditional on being detained more than three days and less than 600 days.14 The left tail of the distribution is omitted since the primary definition of ‘detainees’ used in this paper is being unable to make bail within three days; the long right hand tail of the distribution is omitted for visual simplicity. The median number of days detained for those who are unable to make bail within three days is 78, the mean is 146. Summary statistics for the released group, the detained group, and the whole sample are shown in Table 1. Defendants are predominantly male, and, although race is missing for 11% of the sample, largely African-American. Those detained tend to have longer criminal histories and are facing more serious charges than those released. It should be noted, however, that 25% of the detained sample are only facing misdemeanor charges.15 Almost half the sample have their charges dropped, dismissed, or are placed in 13 Case outcome or bail data was missing for 0.44% of the sample. These observations were dropped. Although the sixth amendment guarantees the right to a speedy trial this can be waived if the defendant finds it beneficial to extend. 15 The offense information used in this paper is taken from the charge at the time of the bail hearing. Many of those who were originally charged with felonies subsequently had the felony charge downgraded to a misdemeanor. 14 10 some sort of diversion program.16 Almost everyone else was convicted, through plea or at trial, on at least one charge. 90% of cases resolved at trial result in convictions, suggesting that prosecutors will not bring a case to trial if they don’t believe they have a strong chance of winning. If a detained defendant pleads quickly to avoid more time waiting in jail, she may be pleading guilty on a case that otherwise would not have proceeded to court. One third of the sample is released without being required to pay bail and an additional 26% are able to pay their way out within three days of the bail hearing. Figure 1b shows the number of people detained and released at a variety of bail ranges. The median amount of bail for the detained group is $10,000. Almost 40% of those who were given bail amounts less than or equal to $2000 are detained for longer than three days. Among this low-bail sample – 77% of whom are charged only with misdemeanors – the average number of days detained pretrial is 28. This group would need to pay a deposit $200 or less to secure their freedom. Table 2 shows the most common lead charges. The table is organized in descending order, where the top of the list represents crime types where evidence tends to be strongest. The horizontal line separates those classified as ‘strong-evidence’ crimes from those classified as ‘weak-evidence’ crimes. The ranking of charges by strength of evidence was done in two ways. First, I surveyed a variety of Philadelphia lawyers and professors who specialize in criminal law. I asked them to rank each crime on a scale of one to five, where a one meant that the evidence in that crime type tended to be ambiguous and subject to multiple interpretations and a five meant that the evidence in that crime type tended to be clear and difficult to dispute. Second, I calculated the average conviction rate per crime type under the assumption that the conviction rates would be higher among crime types where the evidence was stronger. I ranked each crime according to both measures of evidence strength; the order in which they are presented here is the average of the two rankings. While there were differences across the two methods in the exact ordering, the general placement was quite similar.17 Figures 1c shows the likelihood of pleading guilty at various levels of ‘sentence exposure’. Sentence exposure is a measure of how serious the case is: the average incarceration sentence that similarly situated defendants receive if they are found guilty at trial. This is estimated by taking the fitted values from a regression of sentence length on offense, criminal history, demographics, and time controls, using the sample of cases in which the defendant was found guilty at trial. A log transformation is 16 Diversion programs are designed for those with low level misdemeanor charges; if the defendant agrees to requirements such as paying restitution to victims, entering rehab, or performing community service, they are generally able to avoid a formal adjudication of guilt and a criminal record. 17 The ranking based on the survey would have placed car theft in the ‘strong-evidence’ category and other types of theft in the ‘weak-evidence’ category. 11 applied to the fitted values to compress the long right tail. Figure 1c shows that while the likelihood of pleading guilty is relatively flat at low levels of sentence exposure, it rises rapidly and then falls off at higher levels of sentence exposure. While no definitive conclusions should be inferred from this descriptive graph, it is consistent with a story in which defendants plead guilty out of fear of a sentence exposure at trial. While the plea rate drops off at the highest levels of sentence exposure, this may be because the guilty pleas themselves come with increasingly long prison sentences. A present-biased defendant may underweight the difference between the 10 year sentence she is offered via plea or the 25 year sentence she is at risk of receiving at trial. Figure 1d shows conviction rates at various levels of sentence exposure. It shows that throughout most of the support of the distribution, the likelihood of being convicted decreases with sentence exposure. Figure 1e shows the likelihood of detention at various levels of sentence exposure. We see a monotonically increasing relationship between sentence exposure and the likelihood of being detained. This is not surprising, as those who face longer sentences will face increased incentive to flee, or may pose greater public safety risks to the community.18 In Table 3 I test to see if there are socio-economic disparities in the likelihood of being detained pretrial. I regress the log bail amount and a dummy for pretrial detention on race and the log of average income in the defendant’s zip code, controlling for offense, criminal history, age and gender.19 I limit the sample to those for whom both zip code and race is available.20 I find that those coming from wealthier neighborhoods have bail set lower and are less likely to be detained pretrial even after controlling for a wide range of characteristics. A 10% increase in zip code wealth is correlated with a .4% decrease in bail and a .2 percentage point decrease in the likelihood of being detained pretrial. Race does not correlate with the bail amount in a statistically significant manner, however African-Americans are three percentage points more likely to be detained pretrial than Caucasian defendants with similar offense profile, age, gender, and criminal history. 18 These three graphs, taken together, suggest that OLS regressions of case outcomes on detention status may in fact be biased downwards. Since the seriousness of the case is not fully visible in the data (offense can be controlled for, but severity can vary within charge categories) this omitted variable will increase the likelihood of detention but may actually decrease the likelihood of conviction. Other likely omitted variables, such as wealth, lawyer quality, and community ties are more consistent with an upward bias of OLS estimates. 19 I add one to all bail amounts to avoid taking the log of zero. 20 Average gross income per zip code in 2010 was downloaded from IRS.gov. 12 5 Identification test Given the rotation of the work schedules, there may be slightly more imbalance across the magistrates than there would be if the magistrate was randomly and independently drawn for each bail hearing. Since most parametric tests of randomness assume an independent random draw, I conduct a permutation test to verify that the sample of defendants seen by each magistrate are no more different from one another than would be expected by chance, given a rotating work schedule such as the one in use. I generate a variety of “false” work schedules, keeping the basic parameters of the work rotation fixed: five days on, five days off, rotating shifts, three eight hour shifts per day, etc. However I vary both the day at which the five day rotation begins, the hour at which the shifts begin, and the direction of rotation. (The actual magistrates move from graveyard to morning to evening shift, a reverse rotation would move from graveyard to evening to morning.) Since the schedule is five days long there are four potential “false” start dates to choose from. Since the shift is eight hours long there are three potential “false” start times, spaced two hours apart. I generate three false rotations: one in which both groups work a reverse rotation, and two in which one of the groups work a forward rotation while the other group works a reverse rotation. Given five start dates (four false and one real), four start times (three false and one real) and the three false rotations (I do not use the real rotation to minimize correlations with the actual work schedule) I build 60 false work schedules. Covi = α + β ∗ M agistratei + ψ ∗ T imei + ei (1) With each of these work schedules I run the regression specified in Equation 1, where Covi is one of 66 different offense, criminal history and demographic covariates, M agistratei is the dummy for the magistrate who was scheduled during the bail hearing for case i under the false schedule, and T imei are the full set of controls for the time and date of the bail hearing as described in Section 4.21 For each covariate and each false 21 Unless otherwise specified, all regressions shown in this paper control for the variables listed as follows. Demographic variables include age, age squared, age cubed, gender, and dummies for being black or white. Criminal history variables include the number of prior cases, prior felony cases, prior cases involving a serious violent crime (robbery, assault, burglary, murder, rape), prior cases where the defendant was found guilty of at least one charge, and dummies for having at least one prior case, having at least three prior cases, awaiting trial on another charge, having a detainer placed on them, and having a prior arrest within five years of the bail hearing. Offense variables include dummies for having a charge in the following category: murder, rape, robbery, aggravated assault, burglary, theft, shoplifting, simple assault, drug possession, drug selling, drug buying, possession of marijuana, F2 firearm, F3 firearm, possession of stolen property, vandalism, a nonfirearm weapon charge, prostitution, first offense DUI, second offense DUI, resisting arrest, stalking, motor vehicle theft, indecent assault, arson, solicitation of prostitutes, disorderly conduct, pedophilia, intimidation of witnesses, accident due to negligence, false reports to a police officer, fleeing an officer, and reckless 13 work schedule I collect the F statistic for the joint magistrate dummies. This creates an empirical distribution of the F statistic under false schedules. I then compare the F statistic from the Equation 1 regression in which the magistrate dummies are those who were actually scheduled to the distribution of F statistics under false schedules. For each covariate I build an ‘empirical p value’ which is the fraction of false-schedule F statistics that are larger than the true-schedule F statistic. I also perform this technique on three summary variables which are the fitted values from a regression of a dummy for pretrial detention, a dummy for pleading guilty to at least one charge, and a dummy for being convicted on at least one charge on all 66 of the offense, criminal history, demographic and time controls. These predicted values are a weighted average of the characteristics that correlate most strongly with the main dependent variables and the endogenous independent variable. I show the F statistic and the empirical p value for these three summary statistics in Table 4. The F statistics are 1.84, 2.59 and 1.91 respectively; as expected the covariates are somewhat less balanced across the magistrates than they would be if the bail magistrate was independently and randomly assigned to each bail hearing. However, at 0.96, 0.56 and 0.58 respectively, the empirical p values show that any imbalance in the predicted likelihood of detention, guilt, or guilty pleas across the magistrates is no more than would be expected by chance. Figure 1f shows a histogram of the 69 empirical p values from the 66 covariates and three summary statistics. As can be seen, the empirical p values are evenly spread between zero and one. The mean p value is 0.56, the median is 0.58, and three p values are less than or equal to 0.05. The mean F statistic is 2.21 and the median F statistic is 1.84. The ‘false’ F statistics comprising the empirical distribution will be correlated, since there is overlap in the false schedules. This reduces the power on any single test (any endangerment. Additional offense controls include dummies for being charged with a first, second or third degree felony, an unclassified felony, a first, second or third degree misdemeanor, an unclassified misdemeanor, or a summary offense. I also control for the total number of charges, the total number of felony charges, the total number of misdemeanor charges, and the total ‘offense gravity score’ of the charges (the offense gravity score is used by Philadelphia to measure the seriousness of a charge on a scale of 1-8). Time controls include dummies for the day of the week that the bail hearing occurs, a dummy for graveyard, morning, and evening shift, a cubic in day of the year (1-365), the bail date, and year dummies. Since magistrates tend to leave and be replaced in the third week of February, I define the year dummies to align with the start and end of a magistrate’s work period. The following dates serve as dividers: February 21, 2008, February 23, 2009, February 23, 2010, February 23, 2011, February 23, 2012, and February 18, 2013. I interact some of the covariates with three time periods, as divided by February 23, 2009 and February 23, 2011. The covariates that I allow to have differing impacts over these three time periods are the same as the ones that I interact with the magistrate fixed effects in various specifications: murder, robbery, aggravated assault, burglary, theft, shoplifting, simple assault, drug possession, drug sale, drug purchase, marijuana possession, F2 firearm, F3 firearm, vandalism, prostitution, first offense DUI, motor vehicle theft, gender, a dummy for being African-American, the number of prior cases, the number of prior violent crimes, a dummy for having at least one prior and a dummy for having a detainer. 14 single covariate) since the 60 different false F statistics are not independent from one another. However among the 69 different tests there is no evidence to suggest strategic behavior that would undermine the credibility of the research design. 6 Empirical strategy and visual representations of the research design Instrumenting for sentencing outcomes using varying propensities of randomly assigned or rotating judges is a popular method of identifying the impact that the criminal justice system has on defendants (Kling, 2006; Aizer and Doyle, 2009; Loeffler, 2013; Tella and Schargrodsky, 2013; Mueller-Smith, 2015). Two concerns with this methodology are that the judges may affect outcomes through channels other than the primary sentencing characteristics the researcher is interested in, and that the monotonicity assumption may be violated. Concern about alternate channels of influence are minimized in this setting due to the limited nature of the bail magistrates’ responsibilities and the fact that their work schedule does not align with the other actors in the criminal justice system. However the assumption that the bail-setting propensities for each magistrate do not vary according to defendant characteristics would be harder to defend. I follow Mueller-Smith (2015) in allowing the magistrates’ bail habits to vary across time and according to defendant and case characteristics. Allowing the preferences of the magistrates to update every two years relaxes the assumption that all magistrates respond in the same way to changes in the criminal justice system: a new district attorney, an update to the bail guidelines, a change in capacity constraints at the local jail. Allowing the preferences of the magistrates to vary across case and defendant characteristics relaxes the assumption that a strict magistrate must be equally strict on all crimes and all defendants. In addition to minimizing non-monotonicity bias, exploiting within-magistrate variation in bail-setting propensities increases power. Empirically, allowing the magistrates’ bail preferences to vary is accomplished by interacting the magistrate fixed effects with time period fixed effects and a subset of the covariates in the first stage regression, as shown in Equation 2. Detentioni is a dummy which is equal to one if the defendant is detained for more than three days in case i, M agistratei is a dummy for the magistrate who was scheduled to work during the bail hearing for case i, and Covi1 are the subset of the covariates across which I allow the magistrate’s preferences to vary: the 16 most common crime types, gender, race, and criminal history. Covi2 are the remainder of the offense, demographic and criminal history controls, as listed in Footnote 21. Ti3 is a dummy for the time period of the bail hearing for case i (there are three time periods as divided by February 23, 15 2009 and February 23, 2011) and T imei are the full set of controls for the time and date of the bail hearing (which include Ti3 as a subset). I use a jackknife instrumental variables technique to avoid the bias induced by many instruments (Angrist et al., 1999). Detentioni = α2 + M agistratei ∗ Ti3 ∗ ω2 + M agistratei ∗ Covi1 ∗ φ2 + Covi1 ∗ Ti3 ∗ δ2 + Covi2 ∗ γ2 + T imei ∗ ψ2 + ei (2) The second stage is shown in Equation 3 where Case Outcomei represents a variety of case outcomes, Detentioni is the fitted value from the jackknifed first stage, and Covi1 , Covi2 , Ti3 and T imei are as described above. I present results from a linear regression in both stages; logit regressions yield similar results. Case Outcomei = α3 + Detentioni ∗β3 +Covi1 ∗Ti3 ∗δ3 +Covi2 ∗γ3 +T imei ∗ψ3 + i (3) Figures 2 and 3 show visual representations of the first and second stage of the IV regression. Figure 2 shows how pretrial detention rates vary by magistrate for different crime types. The y axes show residuals from a regression of pretrial detention on controls for the time and date of the bail hearing. The eight bars represent the average detention residuals for the eight magistrates, ordered so that Magistrate 1 has the lowest overall rate of pretrial detention, Magistrate 2 has the second lowest, and so forth. The order of the magistrates remains the same in all subplots. Figure 2a shows the magistrate means across the entire sample, Figures 2b-f show the means for different crime types. In each offense category, n refers to the number of cases charged with that offense and F refers to the joint significance of the magistrates in a regression of detention status on magistrate dummies and time controls on that subsample. The figures demonstrate that while magistrates vary in their overall detention rates, there is quite a bit of variance across the different crime types. For example, although the overall detention rate of Magistrates 2 is quite low, this magistrate has the highest detention rates for prostitution and drug possession. This within-magistrate variation in detention rates suggest that the monotonicity assumption will be violated if detention status is instrumented for with the overall detention rate of the magistrates. Figure 3 shows that defendants whose bail hearing is presided over by high-bailsetting magistrates are more likely to be convicted or to plead guilty. In Figure 3a the y and x axes show residuals from a regression of conviction and detention dummies respectively on the set of time controls described by T ime. Figure 3b is similar except the dummies are residualized over Cov 1 ∗ T 3 , Cov 2 and T ime. Each circle represents the average detention and conviction residuals of the eight magistrates; the size of the circle is proportional to the total number of cases seen by each magistrate. As 16 can be seen there is a clear positive correlation between the conviction and detention residuals which changes very little once the effect of covariates have been removed. This provides visual evidence that the slight differences in the groups of defendants seen by each magistrate are not the cause of the positive relationship between detention and conviction. Figures 3c and d show the relationship between pretrial detention and pleading guilty for those who are charged with weak-evidence crimes and those charged with strong-evidence crimes respectively. In this graphic, the magistrate dummies have been interacted with T 3 , dummies for the three time periods. The x and y axes in both figures show residuals from a regression of pretrial detention and guilty pleas respectively on covariates, time controls, and time-covariate interactions. The circles show the average residuals per-magistrate-per-time period. A clear positive relationship can be seen between the likelihood of being detained and the likelihood of pleading guilty in weak-evidence cases. There is no visually discernible relationship among strong-evidence cases. 7 Full sample results In Table 5 I show results from a variety of jackknife instrumental variables spec- ifications where the outcome variable is a dummy which equals one if the defendant is convicted on at least one charge in Panel A, and a dummy variable which equals one if the defendant pled guilty to at least one charge in Panel B. In Column 1 the only instruments are the eight magistrate dummies and in Column 2 the magistrate dummies are interacted with T 3 , the three time period dummies. The only controls in the first two columns are T ime. The standard errors decrease between the first and second column by about 10%, suggesting that allowing the magistrates to respond differently to the various changes that occur during the period of my analysis increases the power of the instrument. Covariates are added in Column 3 and the effect sizes either increase (Panel A) or remain constant (Panel B). Columns 4-6 allow the bail setting habits of the magistrates to vary according to offense, criminal history and demographics of the defendant. In Column 4, the magistrate dummies are interacted with dummies for the five most common lead charges: drug possession, first offense DUI, robbery, selling drugs, and aggravated assault. In Column 5 I add interactions between magistrate dummies and the number of prior cases/prior violent crimes, dummies for having at least one prior case, having a detainer, and being African-American or female. In Column 6 I add interactions between the magistrate dummies and the other most common crime types as listed in Table 2. The number of instruments per specification as well as the F statistic of joint significance on the first stage instruments 17 are included in the bottom panel. Both effect sizes and standard errors decrease as instruments are added. This suggests two things: that allowing the bail-setting habits of the magistrates to vary across defendant characteristics both increases the power and reduces non-monotonicity bias in the results. In particular, if treatment effects are smaller among crime types for which the monotonicity assumption is violated, then the estimates in Columns 1-3 will be biased upwards. It should be noted, however, that non-monotonicity bias will not generate spurious results if no treatment effects exist. Under the null hypothesis it would be very unlikely to see effect sizes as large as those shown in Table 5. My preferred specification is the one where magistrate’s preferences are allowed to vary across all 16 of the most common crime types, across the criminal history, race, and gender of the defendant, and over the three time periods. I estimate that pretrial detention leads to a 6.6 percentage point increase in the likelihood of being convicted and a 5.3 percentage point increase in the likelihood of pleading guilty. Compared to the means for each dependent variable, that converts into a 13% increase in the probability of conviction and a 21% increase in the likelihood of pleading guilty. Table 6 shows how pretrial detention affects conviction rates, guilty pleas, court fees, the likelihood of being incarcerated, and both the maximum and minimum incarceration sentence.22 Panel A shows results from the jackknife instrumental variables method with the most fully interacted specification and Panel B shows results from an OLS regression controlling for the full set of offense, criminal history, demographic and time controls. With the exception of court fees and the incarceration dummy, results do not vary substantially between IV and OLS. This suggests that researchers who are interested in estimating the effects of pretrial detention in other jurisdictions may be able to achieve reasonable results with standard court data even in the absence of a natural experiment. The IV estimates suggest that pretrial detention leads to an average increase of $128 in non-bail court fees owed. Conditional on being convicted, court fees average at $775, and $1250 if convicted of a felony. For the tens of thousands of people convicted as a result of pretrial detention – many of whom were unable to pay even fairly small amounts of bail – these court fees may pose a significant burden. The IV results for the likelihood of being incarcerated and the maximum incarceration sentence are positive but noisy, however the estimates on the minimum incarceration sentence are more precise. Pretrial detention leads to an expected increase of 140 days in sentence length before being eligible for parole. Conditional on receiving a sentence of incarceration, defendants spend an average of 22 months in jail before being eligible for parole. In results not shown here, I test to see if pretrial detention affects post-disposition 22 Sentence length is coded as zero for individuals who do not receive an incarceration sentence. 18 crime. The estimates are noisy but are generally negative, suggesting that some crimes may be averted as a result of increased incapacitation. Forthcoming work will provide a cost-benefit analysis of the impact pretrial detention has on pretrial crime, including novel estimates of the cost of incarceration to the incarcerated (Mayson and Stevenson, 2016). 8 Results for various subsamples In Table 7 I show results for misdemeanors and felonies using both IV and OLS techniques; the discussion below focuses on the IV results.2324 The effect sizes of the felony sample are similar in magnitude to the full sample, but are noisy. The effects among misdemeanors are more precisely measured and are slightly larger than the full sample estimates, especially in relation to the means of the dependent variables. In fact, pretrial detention among misdemeanor defendants leads to a statistically significant increase in all outcomes. The effects on punishment are particularly large: those detained will be 8 percentage points more likely to receive a sentence of incarceration over a mean 16 percentage point incarceration rate. While the expected increase in sentence length is only a month or two, this represents more than a 100% increase relative to the mean.25 Those who are given an incarceration sentence for a misdemeanor crime will spend an average of 9 months in jail before being eligible for parole. In Table 8 I compare effect sizes across weak-evidence crimes and strong-evidence crimes. Many of the strong-evidence crimes are possession crimes, where drugs or illegal firearms were found on the defendant’s body or in her home or car. Shoplifting crimes usually entail store merchandise found on the defendant’s person as they walk out of the store. In DUI’s, the defendant was found behind the wheel with an elevated blood alcohol level, and prostitutes are usually arrested after soliciting from an undercover officer. The main piece of evidence among strong-evidence crimes is the police officer’s statement and/or a lab report; these types of charges can be difficult to contest. Most weak-evidence crimes are complainant offenses (an arrest was made as a result of a complaint). Evidence in these types of crimes are much more likely to consist of victim testimony, eyewitness accounts, surveillance/bystander video, alibis, character 23 The felony sample is defined as those who were charged with at least one felony at the time of the bail hearing; many of these had their charges downgraded to misdemeanors only by the time of the arraignment. 24 The IV specifications allow the magistrate preferences to vary across time and across defendant characteristics, as shown in Column 6 of Table 5. 25 This increase in sentence length could not be explained solely by detained defendants being released with time-served. If all of the defendants who were convicted as a result of detention were given time served this would result in an average increase of only 12.24 days. (The average number of days detained for those unable to make bail within 3 days is 144, I estimated that detention leads to a 8.5 percentage point increase in conviction. 144 ∗ 0.085 = 12.24) 19 testimonials, identification from police lineups, etc. With this type of evidence it can be harder to discern the facts of the case. Assaultive crimes in particular may have multiple conflicting accounts of what occurred and why. Witness testimony can be inconsistent, videos blurry, alibis hard to verify, and police lineup identification is notoriously unreliable. While a number of theft crimes are included in this category, possession of a stolen object does not automatically imply culpability. For example, passengers in a stolen car may not be aware that the car was stolen. My hypothesis is that extra-legal factors such as detention status will have less effect on cases where the evidence is strong than they will in cases where the facts are difficult to discern. Prosecutors are unlikely to drop charges if the evidence is strong, nor will they lose if the case is brought to trial. While a detained defendant may plead guilty sooner or to more unfavorable terms, the effect on conviction should be minimal. In a weak-evidence case, however, the defendant’s willingness to wait may prove important. Cases where the evidence is weak are much more likely to be dropped, or to result in acquittal at trial. Furthermore, such cases may rely on the testimony of witnesses who are reluctant to cooperate or whose memory fades over time. In fact, if the prosecution’s key witnesses fail to appear four times in a row, the case is dropped. If detention leads defendants to plead guilty or move to trial quicker than they would otherwise this could be a significant disadvantage. Detention status may also directly affect the evidence available among weak-evidence crimes. 75% of the sample is represented by a public or court appointed attorney; the high case volumes handled by these attorneys suggest that they may not have the time to do as much investigative work as is necessary. A released defendant can contact eyewitnesses, secure surveillance video, take photos of the crime scene, and otherwise collect exculpatory evidence. A released defendant can also pressure witnesses, destroy evidence, or otherwise impede the investigation. While the standard errors in the IV estimates are large enough that definitive conclusions can’t be drawn, the results generally suggest that effect sizes are larger among weak-evidence crimes. The difference is particularly striking for sentence length: those detained on a weak-evidence crime can expect to be sentenced to an additional 18 months in prison before being eligible for parole. With the exception of court fees, the IV effects for strong-evidence crimes are close to zero and are not statistically significant. The OLS results also support the hypothesis that effect sizes are larger among weakevidence crimes. The OLS estimates of the effect pretrial detention has on conviction are 0.098 among weak-evidence crimes and 0.007 among strong-evidence crimes. Figure 4 shows OLS effects by crime category (the IV results are too noisy for such small samples). Strong-evidence crimes are at the top and weak-evidence crimes are at the 20 bottom. The coefficient plot, which shows the estimated effect that pretrial detention has on guilty pleas, again suggests that effect sizes are larger among weak-evidence crimes. Table 9 shows IV results for blacks, whites, young defendants, older defendants, those with one or no prior arrests, and those with more extensive criminal history. Overall, there is nothing to suggest that effect sizes differ across race. The point estimates are generally quite similar, although the subsample is small enough that many are not statistically significant. Results are also similar among younger and older defendants. The point estimates for sentence outcomes are greater among the younger defendants, but the standard errors are large as well. We do, however, see suggestive evidence that effect sizes are larger for those with limited prior interactions with the criminal justice system. Among first or second time arrestees, pretrial detention leads to a 12 percentage point increase the likelihood of pleading guilty and a 17 percentage point increase in the likelihood of being convicted.26 9 Robustness checks and effect sizes for varying definitions of pretrial detention In Table 10 I present several robustness checks for the full sample results. Panel A is identical to Panel A of Table 6 except that magistrate dummies are also included as controls in the second stage regression. Controlling for the eight magistrate dummies implies that the impact pretrial detention has on case outcomes is being identified solely off of within-magistrate variations in detention rates. In particular, these controls will absorb any other fixed aspects of the magistrates that may affect the results. For example, the most lenient magistrate may also be particularly encouraging or supportive during the bail hearing. If this affects the defendant’s expectations of success at trial – and thus their willingness to accept a guilty plea – this would undermine the exogeneity assumption. If the effect sizes change greatly as a result of including magistrate fixed effects as controls this would raise concerns that the magistrates are affecting case outcomes through channels other than pretrial detention. Panel A shows that although the inclusion of magistrate fixed effects increases the standard errors, the effect sizes are not changed dramatically. Panel B is identical to Panel A of Table 6 except that controls for attorney type are added. While attorney type is likely to be endogenous to both the bail amount and detention status, a large change in effect size as a result of these controls would raise concerns that the effects are being driven by variations in the magistrate’s willingness 26 The sample of first time arrestees is so small that the IV results are hard to interpret. 21 to grant public defense. Alternatively, it could suggest that the effects seen are not as a result of pretrial detention per se, but rather the wealth affects of bail and the ability to hire a quality lawyer. However the inclusion of controls for having a public defender, a court-appointed attorney, or a private attorney have only trivial results on the estimates. In Figure 5 I look at how effect sizes differ if pretrial detention is defined as spending at least one night in jail after the bail hearing, more than two weeks, more than thirty days, or still being in jail at the time of disposition. The figures show regression estimates from the IV specification where magistrate’s preferences are allowed to vary over time and according to defendant characteristics. Figures 5a and b show results for the full sample, Figure 5c shows the weak-evidence sample and Figure 5d shows cases for first or second time arrestees. The outcome in Figure 5b is conviction and the outcome for all other figures is guilty pleas. In each subplot, the effect size increases as the number of days detained increases. The standard errors increase as well. This is because the initial bail amount set by the magistrate becomes less relevant to detention status as time goes on (future judges may revise bail downward).27 However despite the noisy estimates, the lower bound on the 95% confidence interval is far from zero in some of these specifications. Among weak-evidence crimes, the lower bound of the effect of being detained throughout the entire pretrial period on the likelihood of pleading guilty is almost 10 percentage points. 10 Conclusion Using a natural experiment in Philadelphia where the likelihood of being detained pretrial is exogenously affected by the magistrate who presides over the bail hearing, I find that pretrial detention leads to an increase in the likelihood of being convicted, mostly by increasing the likelihood that defendants, who otherwise would have been acquitted or had their charges dropped, plead guilty. The effects are larger among first or second time arrestees and among crime types where evidence tends to be weak. In Philadelphia, almost 80 percent of arrestees have bail set at $10,000 or less. These arrestees would only need to pay up to a $1000 deposit to secure their release, an amount that is likely to be had in savings or available to borrow by most middle and upper middle class Americans. Yet 60% of arrestees with a $10,000 bail are unable to pay this amount within three days and 34% remain in jail at the time of disposition. Some argue that money bail is unconstitutional since it is so difficult to ensure that the price of bail is set proportional to one’s means in a way that precludes detention 27 I chose ‘detained more than three days’ as my preferred measure since it seemed a reasonable balance between strength of instrument and size of the effect. 22 based on wealth. In fact, many jurisdictions do not take ability to pay into account at all when setting bail, a practice that is clearly a violation of the Equal Protection Clause.28 A nonprofit legal organization called Equal Justice Under Law has been filing a series of lawsuits to ensure that the defendant’s ability to pay is taken into account in the setting of bail. Yet this is not the only important legal/policy question which hinges, at least partly, on the full costs of pretrial detention to the defendant. Another as-of-yetunanswered question is whether defendants have a right to counsel at the bail hearing. The Supreme Court has ruled that the state must pay for indigent defendants to have an attorney at all ‘critical stages’ of the criminal proceedings, but what exactly constitutes a critical stage is not completely clear. Many jurisdictions, including Philadelphia, do not provide counsel to indigent clients at the bail hearing. Another outstanding question is whether detention places undue coercive pressure on defendants to plead guilty. Guilty pleas are required to be voluntary by the Due Process Clauses of the Fifth and Fourteenth Amendments and if the ‘punishment’ of waiting in jail until trial is worse than the penalties involved with pleading guilty then a plea may might not be considered voluntary. Finally, the Eighth Amendment prohibits ‘excessive bail’. Since the full costs of bail include its effect on case outcomes, bail amounts that might have been considered reasonable when only weighing the costs of a pretrial loss of liberty against the benefits of averted crime may seem excessive when including all the downstream effects of pretrial detention.29 The findings presented in this paper, taken in context of these four highly policyrelevant questions, suggest several things. First they suggest that jurisdictions should move away from a means-based method of determining who is detained pretrial, as the socio-economic disparities of such a system ripple far beyond the pretrial period. Second, they suggest that the bail hearing is indeed a critical stage, and indigent defendants should have the right to counsel. Third, they suggest that low risk defendants – for whom the ‘punishment’ of pretrial detention is worse than the expected punishment for the crime – should not be detained as detention may result in coerced guilty pleas. Finally, while determinations of ‘excessiveness’ are beyond the scope of this paper, the evidence suggests that the costs of detention are high and, if justified, the benefits must be high as well. 28 The Department of Justice issued a Statement of Interest in Varden v. City of Clanton (February 2015) declaring that fixed bail bond schedules that do not take indigence into account are a violation of the Fourteenth Amendment. 29 For a more detailed discussion of the constitutional questions which hinge at least partly on how pretrial detention affects case outcomes see (Heaton et al., 2016). 23 References Abrams, David S. and Chris Rohlfs, “Optimal Bail and the Value of Freedom: Evidence from the Philadelphia Bail Experiment,” Economic Inquiry, 2011, 49 (3), 750–770. Aizer, Anna and Joseph J. Jr. Doyle, “Juvenile Incarceration, Human Caoital and Future Crime: Evidence from Randomly-Assigned Judges,” National Bureau of Economic Research Working Paper, 2009. Allen, Ronald J. and Larry Laudan, “Deadly Dilemmas II: Bail and Crime,” Northwestern Public Law Research Paper, December 2008, (08-44). Anderson, James M. and Paul Heaton, “How Much Difference Does the Lawyer Make? The Effect of Defense Counsel on Murder Case Outcomes,” Yale Law Journal, 2012, 122 (1). Angrist, Joshua D., Guido W. Imbens, and Alan B. Krueger, “Jackknife Instrumental Variables Estimation,” Journal of Applied Econometrics, 1999, 14 (1), 57–67. Ares, Charles E., Anne Rankin, and Herbert Sturtz, “The Manhattan Bail Experiment: An Interim Report on the Use of Pretrial Parole,” New York University Law Review, 1963, 38. Bibas, Stephanos, “Plea Bargaining Outside the Shadow of the Trial,” Harvard Law Review, June 2004, 117 (8). Clark, John, Daniel Peterca, and Stuart Cameron, “Assessment of Pretrial Services in Philadelphia,” Technical Report, Pretrial Justice Institute February 2011. Cohen, Thomas H. and Brian A. Reaves, “Pre-Trial Release of Felony Defendants in State Court,” Technical Report, Bureau of Justice Statistics Special Report November 2007. Devers, Lindsey, “Plea and Charge Bargaining: Research Summary,” BJA Report January 2011. Free, Marvin D. Jr., “Bail and Pretrial Release Decisions,” Journal of Ethnicity in Criminal Justice, 2004, 2 (4), 23–44. Goldkamp, John S., “The Effects of Detention on Judicial Decisions: A Closer Look,” The Justice System Journal, 1980, 5 (3), 234–257. 24 Heaton, Paul, Sandra Mayson, and Megan Stevenson, “The Downstream Criminal Justice Consequences of Pretrial Detention,” Working Paper 2016. Kahneman, Amos Tversky Daniel, “Prospect Theory: An Analysis of Decision under Risk,” Econometrica, 1979, 47 (2), 263–291. Kling, Jeffrey R., “Incarceration Length, Employment, and Earnings,” American Economic Review, June 2006, 96 (3), 863–876. Loeffler, Charles E., “Does Imprisonment Alter the Life Course? Evidence on Crime and Employment From a Natural Experiment,” Criminology, 2013, 51 (1), 137–166. Lowenkamp, Christopher T., Marie VanNostrand, and Alexander Holsinger, “Investigating the Impact of Pretrial Detention on Sentencing Outcomes,” Technical Report, Laura and John Arnold Foundation 2013. Mayson, Sandra and Megan Stevenson, “Cost of Crime Versus the Cost of Doing Time: Is Pretrial Detention ‘Cost-Justified’ When the Loss To Legally Innocent Detainees is Included?,” Working Paper 2016. Minton, Todd D. and Zhen Zeng, “Jail Inmates at Midyear 2014,” Technical Report, Bureau of Justice Statistics Bulletin June 2015. Mueller-Smith, Michael, “The Criminal and Labor Market Impacts of Incarceration: Identifying Mechanisms and Estimating Household Spillovers,” Working Paper August 2015. Oleson, J.C., Christopher T. Lowenkamp, Timothy P. Cadigan, Marie VanNostrand, and John Wooldredge, “The Effect of Pretrial Detention on Sentencing in Two Federal Districts,” Justice Quarterly, 2014. ONDC, “”FAFSA Facts”,” Technical Report, Office of National Drug Control Policy U.S. Department of Education. Phillips, Mary T., “Pretrial Detention and Case Outcomes, Part 1: Nonfelony Cases,” Final Report, New York Criminal Justice Agency, Inc. November 2007. , “Bail, Detention and Felony Case Outcomes,” Research Brief, New York Criminal Justice Agency, Inc. September 2008. Rankin, Anne, “The Effect of Pretrial Detention,” New York University Law Review, 1964, 39. 25 Sacks, Meghan and Alissa R. Ackerman, “Pretrial detention and guilty pleas: if they cannot afford bail they must be guilty,” Criminal Justice Studies, 2012, 25 (3), 265–278. Schlesinger, Traci, “Racial and ethnic disparity in pretrial criminal processing,” Justice Quarterly, 2005, 22 (2), 170–192. SHRM, “”Society for Human Resource Management Survey Findings: Background Checking - The Use of Criminal Background Checks in Hiring Decisions”,” Technical Report, The Sentencing Project July 2012. Shubik-Richards, Claire and Don Stemen, “Philadelphia’s Crowded, Costly Jails: The Search for Safe Solutions,” Technical Report, Pew Charitable Trusts Philadelphia Research Inititiative May 2010. Tartaro, Christine and Christopher M. Sedelmaier, “A tale of two counties: the impact of pretrial release, race, and ethnicity upon sentencing decisions,” Criminal Justice Studies, 2009, 22 (2), 203–221. Tella, Rafael Di and Ernesto Schargrodsky, “Criminal Recidivism after Prison and Electronic Monitoring,” Journal of Political Economy, 2013, 121 (1), 28 – 73. Thacher, David, “The Rise of Criminal Background Screening in Rental Housing,” Law & Social Inquiry, 2008, 33 (1), 5–30. Uggen, Christopher, Sarah Shannon, and Jeff Manza, “”State-Level Estimates of Felon Disenfranchisement in the United States, 2010”,” Technical Report, The Sentencing Project July 2012. Williams, Marian R., “The Effect of Pretrial Detention on Imprisonment Decisions,” Criminal Justice Review, 2003, 28 (2), 299–316. Wooldredge, John, “Distinguishing Race Effects on Pre-Trial Release and Sentencing Decisions,” Justice Quarterly, 2012, 29 (1), 41–75. 26 Table 1: Summary statistics Age Male Caucasian African-American Missing race Number of prior cases Has felony charge Number of charges Bail Bail=$0 Detained>3 days Never released All charges dropped Case went to trial Not guilty on all charges Guilty of at least one charge Pled guilty to at least one charge Court fees charged Sentenced to incarceration Days of incarc. sentence Days before elig. for parole Observations Released 32.9 0.79 0.30 0.53 0.15 4.14 0.36 4.95 $3,345 0.54 0 0.00 0.48 0.32 0.03 0.49 0.21 $386 0.18 94 42 197,775 Detained Total 32.0 32.5 0.89 0.83 0.26 0.29 0.65 0.57 0.05 0.11 6.25 4.97 0.75 0.51 6.71 5.64 $63,336 $26,877 0.01 0.33 1 0.41 0.58 0.23 0.48 0.48 0.19 0.27 0.03 0.03 0.49 0.49 0.33 0.26 $211 $317 0.34 0.24 585 292 331 159 133,840 331,615 Note: Summary statistics are presented for those who are released within three days of the bail hearing (Column 1), those who are detained for more than three days after the bail hearing (Column 2) and the entire sample (Column 3). All offense variables refer to the charges present at the time of the bail hearing. The statistic shown is the mean and, unless otherwise indicated, variables are dummies where 1 indicates the presence of a characteristic. Age is measured in years, those marked “Number...” are count variables, and those expressed in dollar amounts are currency. The bottom two rows refer to the maximum number of days of the incarceration sentence and the minimum number of days before the defendant is eligible for parole. The sentence is coded as zero if the defendant did not receive an incarceration sentence. 27 Table 2: Main offenses DUI, 1st offense Prostitution Shoplifting Small amount marijuana Illegal firearms (F2 and F3) Drug possession Buying drugs Selling drugs Car theft Theft Robbery Burglary Murder Vandalism Simple assault Aggravated assault Observations 0.065 0.020 0.042 0.022 0.036 0.14 0.053 0.13 0.021 0.042 0.073 0.046 0.021 0.011 0.065 0.091 331615 Note: The statistic shown is the fraction of the overall sample whose lead charge is as listed. Crime types are listed according to strength of evidence; crime types at the top of the list tend to have stronger evidence than those at the top of the list. Strength of evidence is measured both by a poll of criminal justice lawyers and by average conviction rate. The horizontal line separates those placed in the ‘weakevidence’ category and those placed in the ‘strong-evidence’ category 28 Table 3: How do race and neighborhood wealth relate to bail amount and pretrial detention? African-American Log income (average per zip code) Observations R2 Demographic controls Criminal history controls Offense controls (1) Log bail amount 0.00197 (0.0125) -0.0451∗∗∗ (0.0144) 251236 0.584 Y Y Y (2) Pretrial detention 0.0278∗∗∗∗ (0.00178) -0.0217∗∗∗∗ (0.00205) 251236 0.336 Y Y Y Standard errors in parentheses Heteroskedastic-Robust Standard Errors ∗ p < 0.10, ∗∗ p < 0.05, ∗∗∗ p < 0.01, ∗∗∗∗ p < 0.001 Note: This table shows the how race and neigborhood wealth correlate with bail amount and pretrial detention status, after controlling for offense, criminal history, age, gender and time. Only the subset of defendants for whom zipcode and race information was available were included Table 4: Covariate balance across magistrates Summary Predicted Predicted Predicted statistics for defendant characteristics likelihood of pretrial detention likelihood of pleading guilty likelihood of conviction F statistic 1.84 2.59 1.91 Empirical p value 0.96 0.56 0.58 Note: The predicted likelihoods described in the left-most columns are the predicted values from a regression of pretrial detention, guilt, and conviction, respectively, on offense, criminal history, demographics and time controls. The F statistics are the F statistics in a test of joint significance of eight magistrate dummies when regressing the predicted values on the magistrate dummies and controls for the time and date of the bail hearing. The empirical p values show the likelihood of seeing an F statistic as big or bigger if the magistrate seen was due to chance; this is calculated as the fraction of ‘false’ F statistics as big or bigger in a permutation test. 29 Table 5: How does pretrial detention affect conviction rates and guilty pleas? Panel A: Full sample (IV) Pretrial detention (1) 0.166∗∗ (0.0734) Conviction (mean dep. var.= 0.49) (2) (3) (4) (5) 0.181∗∗∗ 0.252∗∗∗ 0.122∗∗∗ 0.0871∗∗ (0.0653) (0.0794) (0.0411) (0.0369) (6) 0.0665∗∗ (0.0293) (1) 0.124∗∗ (0.0617) Guilty pleas (mean dep. var.=0.25) (2) (3) (4) (5) ∗∗∗ ∗∗ ∗∗∗ 0.174 0.175 0.104 0.0582∗ (0.0561) (0.0715) (0.0364) (0.0329) (6) 0.0531∗∗ (0.0265) Panel B: Full sample (IV) Pretrial detention Magistrate X 3 time periods Magistrate X top 5 crimes Magistrate X crim. history Magistrate X demographics Magistrate X top 16 crimes Time controls Covariates Observations First stage F Number of instruments Mean indep. var Y Y Y Y Y Y Y Y Y Y 331615 34.87 8 0.41 331615 19.53 8 0.41 Y Y 331615 26.09 19 0.41 Y Y 331615 21.87 59 0.41 Y Y 331615 14.75 107 0.41 Y Y Y Y Y Y Y 331615 11.65 203 0.41 Standard errors in parentheses Heteroskedastic-Robust Standard Errors ∗ p < 0.10, ∗∗ p < 0.05, ∗∗∗ p < 0.01, ∗∗∗∗ p < 0.001 Note: The dependent variable in Panels A and B respectively is a dummy equal to one if the defendant is convicted on at least one charge and a dummy equal to one if the defendant pled guilty on at least one charge. The instruments in the first two columns are the eight magistrate dummies; in the subsequent columns the instruments include interactions between the magistrate dummies and three time period fixed effects, the five most common crime types, a variety of criminal history variables, defendant demographics, and the remainder of the 16 most common crime types as shown in Table 2. The first two columns control only for the time and date of the bail hearing, all subsequent columns include the full set of controls as described in Footnote 21. The F statistic on the exogenous first stage instruments is listed at the bottom, as are the number of instruments used in that specification and the mean of the independent variable. A linear jackknife instrumental variables regression is used. The Rˆ2 is not reported due to difficulties of interpreting this statistic in an IV regression. 30 Table 6: Full sample results - jackknife IV and OLS (1) Conviction (2) Guilty plea (3) Court Fees (4) Incarceration (5) Max days (6) Min days Panel A: Full sample (IV) Pretrial detention 0.0665∗∗ (0.0293) 0.0531∗∗ (0.0265) 128.7∗∗∗∗ (33.59) 0.0193 (0.0251) 119.3 (73.40) 140.6∗∗ (61.86) Panel B: Full sample (OLS) Pretrial detention 0.0355∗∗∗∗ (0.00197) Observations 331615 First stage F 11.65 Mean dep. var. 0.49 Mean indep. var. 0.41 0.0558∗∗∗∗ (0.00181) 331615 11.65 0.25 0.41 -103.0∗∗∗∗ (2.621) 331615 11.65 $312 0.41 0.1000∗∗∗∗ (0.00166) 331615 11.65 0.24 0.41 136.9∗∗∗∗ (3.430) 331613 11.65 292 0.41 69.88∗∗∗∗ (2.518) 331613 11.65 155 0.41 Standard errors in parentheses Heteroskedastic-Robust Standard Errors ∗ p < 0.10, ∗∗ p < 0.05, ∗∗∗ p < 0.01, ∗∗∗∗ p < 0.001 Note: This table shows how pretrial detention affects various case outcomes using both a jackknife IV regression (Panel A) and an OLS regression (Panel B). The outcome variables are dummies for being convicted/pleading guilty, total non-bail court fees in dollars, a dummy for whether or not the defendant receives an incarceration sentence, the maximum days of that incarceration sentence and the minimum days the defendant must serve before being eligible for parole. In all of the IV specifications magistrate preferences are allowed to vary across three time periods and according to offense, criminal history and demographics of defendants. The F statistic on the first stage of the jackknife IV are shown in the sub-panel, as are the means of the dependent and independent variables. All regressions include the full set of controls as described in Section 6. 31 Table 7: Comparing results for misdemeanors and felonies (1) Conviction Panel A: Misdemeanors (IV) Pretrial detention 0.0850∗∗ (0.0366) (2) Guilty plea (3) Court Fees (4) Incarceration (5) Max days (6) Min days 0.0684∗∗ (0.0300) 93.48∗∗ (37.85) 0.0851∗∗∗ (0.0279) 66.00∗∗∗ (21.64) 30.68∗∗ (12.13) -13.34∗∗∗∗ (3.081) 0.0513∗∗∗∗ (0.00213) 39.23∗∗∗∗ (2.087) 19.62∗∗∗∗ (1.401) Panel B: Misdemeanors (OLS) Pretrial detention 0.0190∗∗∗∗ 0.0515∗∗∗∗ (0.00298) (0.00249) Observations First stage F Mean dep. var. Mean indep. var. 163125 12.82 0.50 0.23 163125 12.82 0.16 0.23 163125 12.82 $351 0.23 163125 12.82 0.16 0.23 163124 12.82 48 0.23 163124 12.82 19 0.23 Panel C: Felonies (IV) Pretrial detention 0.0598 (0.0433) 0.0545 (0.0414) 136.7∗∗ (53.66) -0.0214 (0.0398) 147.5 (135.3) 179.0 (115.2) Panel D: Felonies (OLS) Pretrial detention 0.0514∗∗∗∗ (0.00266) 0.0589∗∗∗∗ (0.00259) -174.2∗∗∗∗ (4.023) 0.134∗∗∗∗ (0.00244) 194.7∗∗∗∗ (5.583) 98.10∗∗∗∗ (4.053) 168490 7.92 0.35 0.58 168490 7.92 $274 0.58 168490 7.92 0.32 0.58 168489 7.92 528 0.58 168489 7.92 294 0.58 Observations First stage F Mean dep. var. Mean indep. var. 168490 7.92 0.47 0.58 Standard errors in parentheses Heteroskedastic-Robust Standard Errors ∗ p < 0.10, ∗∗ p < 0.05, ∗∗∗ p < 0.01, ∗∗∗∗ p < 0.001 Note: This table shows effect sizes in misdemeanor crimes (Panels A and B) and felonies (Panel C and D). The outcome variables are dummies for being convicted/pleading guilty, total non-bail court fees in dollars, a dummy for whether or not the defendant receives an incarceration sentence, the maximum days of that incarceration sentence and the minimum days the defendant must serve before being eligible for parole. In all IV specifications magistrate preferences are allowed to vary across three time periods and according to offense, criminal history and demographics of defendants. The F statistic on the first stage of the jackknife IV are shown in the sub-panel, as are the means of the dependent and independent variables. All regressions include the full set of controls as described in Footnote 21. 32 Table 8: Effect sizes by strength of evidence (1) Conviction (3) Court Fees (4) Incarceration (5) Max days (6) Min days Panel A: Weak-evidence crimes (IV) Pretrial detention 0.0415 0.0735∗ (0.0421) (0.0383) -42.86 (44.04) -0.00348 (0.0354) 516.3∗∗∗∗ (141.7) 541.1∗∗∗∗ (124.1) Panel B: Weak-evidence crimes (OLS) Pretrial detention 0.0983∗∗∗∗ 0.0894∗∗∗∗ (0.00316) (0.00285) -67.93∗∗∗∗ (3.797) 0.131∗∗∗∗ (0.00250) 171.3∗∗∗∗ (7.089) 83.71∗∗∗∗ (5.703) 122742 9.10 $158 0.56 122742 9.10 0.20 0.56 122741 9.10 466 0.56 122741 9.10 276 0.56 Panel C: Strong-evidence crimes (IV) Pretrial detention 0.0308 0.0277 (0.0415) (0.0369) 206.1∗∗∗∗ (49.26) 0.0132 (0.0357) 3.994 (37.54) 0.857 (17.61) Panel D: Strong-evidence crimes (OLS) Pretrial detention 0.00707∗∗ 0.0379∗∗∗∗ (0.00289) (0.00273) -111.3∗∗∗∗ (4.167) 0.0832∗∗∗∗ (0.00262) 98.36∗∗∗∗ (3.568) 47.45∗∗∗∗ (1.809) 165488 13.29 $435 0.27 165488 13.29 0.27 0.27 165488 13.29 187 0.27 165488 13.29 88 0.27 Observations First stage F Mean dep. var. Mean indep. var. Observations First stage F Mean dep. var. Mean indep. var. 122742 9.10 0.35 0.56 165488 13.29 0.60 0.27 (2) Guilty plea 122742 9.10 0.25 0.56 165488 13.29 0.27 0.27 Standard errors in parentheses Heteroskedastic-Robust Standard Errors ∗ p < 0.10, ∗∗ p < 0.05, ∗∗∗ p < 0.01, ∗∗∗∗ p < 0.001 Note: Panels A and B show effect sizes in case types where evidence tends to be relatively weak (murder, aggravated assault, simple assault, vandalism, burglary, theft, robbery, car theft) and Panels C and D show case types where evidence tends to be relatively strong (DUI, drug possession, illegal firearms, drug sale, drug purchase, prostitution, shoplifting). The outcome variables are dummies for being convicted/pleading guilty, total non-bail court fees in dollars, a dummy for whether or not the defendant receives an incarceration sentence, the maximum days of that incarceration sentence and the minimum days the defendant must serve before being eligible for parole. In all specifications magistrate preferences are allowed to vary across three time periods and according to offense, criminal history and demographics of defendants. The F statistic on the first stage of the jackknife IV are shown in the sub-panel, as are the means of the dependent and independent variables. All regressions include the full set of controls as described in Footnote 21. 33 Table 9: Comparing results across defendant characteristics (1) Conviction (2) Guilty plea (3) Court Fees (4) Incarceration (5) Max days (6) Min days Panel A: White defendants (IV) Pretrial detention 0.0700 0.0265 (0.0596) (0.0557) Observations 93937 93937 Mean dep. var. 0.55 0.29 52.43 (75.81) 93937 $361 -0.0253 (0.0541) 93937 0.27 146.7 (129.6) 93937 254 186.0∗ (102.1) 93937 124 Panel B: Black defendants (IV) Pretrial detention 0.0698∗ 0.0355 (0.0400) (0.0359) Observations 191200 191200 Mean dep. var. 0.49 0.25 136.4∗∗∗ (45.19) 191200 $296 -0.00125 (0.0342) 191200 0.25 75.48 (111.3) 191199 357 116.2 (94.53) 191199 196 Panel C: Defendants under 30 (IV) Pretrial detention 0.0487 0.0909 (0.0658) (0.0598) Observations 167392 167392 Mean dep. var. 0.47 0.27 87.38 (79.66) 167392 $304 -0.0106 (0.0573) 167392 0.24 296.2 (214.3) 167391 348 286.8 (187.3) 167391 193 Panel D: Defendants over 30 (IV) Pretrial detention 0.0748∗∗ 0.0553∗ 174.0∗∗∗∗ (0.0359) (0.0326) (40.90) Observations 164194 164194 164194 Mean dep. var. 0.51 0.25 $320 0.0254 (0.0308) 164194 0.24 18.56 (74.48) 164193 235 55.91 (60.45) 164193 117 Panel E: First or second time Pretrial detention 0.175∗∗ (0.0751) Observations 113932 Mean dep. var. 0.41 -0.0333 (0.0588) 113932 0.17 194.0 (225.8) 113930 202 300.5 (192.3) 113930 108 arrestees (IV) 0.122∗ -40.23 (0.0686) (95.65) 113932 113932 0.22 $313 Panel F: Defendants with two or more prior arrests (IV) Pretrial detention 0.0541∗ 0.0387 170.6∗∗∗∗ 0.0736∗∗∗ (0.0317) (0.0286) (36.03) (0.0282) Observations 217683 217683 217683 217683 Mean dep. var. 0.53 0.28 $312 0.27 185.8∗∗ 180.6∗∗∗ (75.66) (63.08) 217683 217683 339 180 Standard errors in parentheses Heteroskedastic-Robust Standard Errors ∗ p < 0.10, ∗∗ p < 0.05, ∗∗∗ p < 0.01, ∗∗∗∗ p < 0.001 Note: This table shows effect sizes among black defendants (Panel A), white defendants (Panel B), defendants under 30 (Panel C), defendants over 30 (Panel D), first or second time arrestees (Panel E) and defendants with two or more prior arrests (Panel F). In all specifications magistrate preferences are allowed to vary across three time periods and according to offense, criminal history and demographics of defendants. 34 Table 10: Robustness checks Panel A: Full sample, controlling for magistrate fixed effects (IV) (1) (2) (3) (4) (5) ConvGuilty Court IncarcMax iction plea Fees eration days ∗∗∗ Pretrial detention 0.0371 0.0413 106.0 0.00242 85.32 (0.0326) (0.0298) (37.28) (0.0283) (92.73) Observations 331615 331615 331615 331615 331613 (6) Min days 160.0∗∗ (79.78) 331613 Panel B: Full sample, controlling for attorney type (1) (2) (3) ConvGuilty Court iction plea Fees ∗∗ ∗∗ Pretrial detention 0.0731 0.0587 124.3∗∗∗∗ (0.0287) (0.0260) (33.56) Observations 331615 331615 331615 (6) Min days 134.9∗∗ (61.70) 331613 (IV) (4) Incarceration 0.0258 (0.0247) 331615 (5) Max days 114.4 (73.05) 331613 Standard errors in parentheses Heteroskedastic-Robust Standard Errors ∗ p < 0.10, ∗∗ p < 0.05, ∗∗∗ p < 0.01, ∗∗∗∗ p < 0.001 Note: This table presents robustness checks for the main results. Panel A includes magistrate fixed effects as controls; the effects of pretrial detention are thus identified solely off of within-magistrate variation in detention rates. Panel B includes controls for attorney type: public defender, court-appointed attorney, and private. The outcome variables are dummies for being convicted/pleading guilty, total non-bail court fees in dollars, a dummy for whether or not the defendant receives an incarceration sentence, the maximum days of that incarceration sentence and the minimum days the defendant must serve before being eligible for parole. In all specifications magistrate preferences are allowed to vary across three time periods and according to offense, criminal history and demographics of defendants. All regressions include the full set of controls as described in Footnote 21. 35 Figure 1: Descriptive graphs (a) Days detained pretrial, conditional on being detained more than three days (b) Bail amounts and detention status 3.0e+04 Median Mean $0 $1-$2000 2.0e+04 $10001-$25000 $25001-$50000 1.0e+04 People detained $2001-$5000 $5001-$10000 $50001-$100000 $100001-$500000 $500000+ 0 0 0 100 200 300 400 500 25,000 50,000 75,000 100000 125000 600 Detained Days detained Released (c) Likelihood of pleading guilty at different levels of sentence exposure (d) Likelihood of conviction at different levels of sentence exposure (e) Likelihood of being detained pretrial at different levels of sentence exposure (f) Distribution of 69 ‘empirical p values’ from permutation test Note: Figure 1a shows the number of people detained/released at various levels of bail. Figure 1b shows the number of days detained pretrial for those who are detained for more than three days. Figures 1c, d and e are a binned scatterplots showing the fraction pleading guilty, convicted, and detained pretrial at various levels of sentence exposure. ‘Sentence exposure’ is a log transform of the predicted value from a regression of days of the incarceration sentence on offense, criminal history, demographics and time controls, with the sample limited to those who were found guilty at trial. Figure 1f is a histogram of the ‘empirical p values’ of 69 different permutation tests to evaluate covariate balance across magistrates. 36 Figure 2: Average detention rates by magistrate for different offense types (a) All cases (n=331,615, F=38.56) (b) Shoplifting (n=15,775, F=31.82) (c) DUI, 1st offense (n=25,850, F=25.88) (d) Simple assault (n=85,396, F=24.93) (e) Prostitution (n=6,529, 42.14) (f) Drug possession (n=109,042, F=18.61) Note: This figure shows pretrial detention rates by magistrate over the whole sample (Figure 2a), and for different offense categories (Figure 2b-f). The numbers 1 through 8 delineate the different magistrates. The y axes show the residuals from a regression of pretrial detention on time controls. The error bars indicate 95% confidence intervals for the mean. n indicates the number of observations per category, and the F statistic refers to a joint F statistic on the eight magistrate dummies when regressing pretrial detention on the magistrate dummies and time controls. The numbering of the magistrates is consistent across all samples. 37 Figure 3: Visual IV (a) Full sample – conviction rates and pretrial detention are residualized over time controls (b) Full sample – conviction rates and pretrial detention are residualized over time controls, offense, criminal history and demographics (c) Weak-evidence crimes – guilty plea rate and pretrial detention are residualized over time controls, offense, criminal history and demographics (d) Strong-evidence crimes – guilty plea rate and pretrial detention are residualized over time controls, offense, criminal history and demographics Note: The y and x axes in Figure 3a show the residuals from a regression of a dummy for conviction and pretrial detention (respectively) on controls for the time and date of the bail hearing. Figure 3b is the same, except conviction and detention have been residualized over offense, criminal history and demographic covariates as well as time controls. The circles in Figures 3a-b show the average detention and conviction residuals for each magistrate; the size of the circle is proportional to the number of cases seen by that magistrate. The y and x axes in Figures 3c-d are residuals from a regression of pleading guilty on offense, criminal history, demographic and time controls. Figure 3c shows the weak-evidence sample and Figure 3d shows the strong-evidence sample. Here the circles represent the average detention and guilty plea residuals per-magistrate-per-time period. There are three time periods as separated by February 23, 2009 and February 23, 2011. 38 Figure 4: OLS estimates of the impact pretrial detention has on guilty pleas within different offense categories, ordered by strength of evidence Note: The above coefficient plots show the OLS estimates of the impact pretrial detention has on guilty pleas for different offense, as labeled on the left. The offenses are ordered according to the strength of evidence that tends to be present for different case types; the offenses on the top tend to have the strongest evidence. Each dot represents the estimated coefficient on pretrial detention, the line represents the 95% confidence interval. 39 Figure 5: Coefficient plots showing the impact of pretrial detention on case outcomes using various definitions of ‘pretrial detention’ (a) Full sample (b) Full sample (c) Weak-evidence crimes (d) First or second arrest Note: The above coefficient plots show jackknife IV regression results where the endogenous independent variable is defined as being detained at least one day, greater than three days, greater than 14 days, greater than 30 days, or until the time of disposition. The top two plots show results from the full sample, the bottom left plot shows results from the ‘weak-evidence’ sample and the bottom right shows results from the sample for first or second time arrestees. The instruments in all specifications are the magistrate dummies interacted with offense, criminal history, race, gender, and three time periods; the full set of controls are included. The dot shows the magnitude of the coefficient estimate as indicated on the x axis and the line indicates a 95% confidence interval. 40