A Deeper Dive into Racial Disparities in Policing in Vermont, Stephanie Seguino and Nancy Brooks, 2018
Download original document:
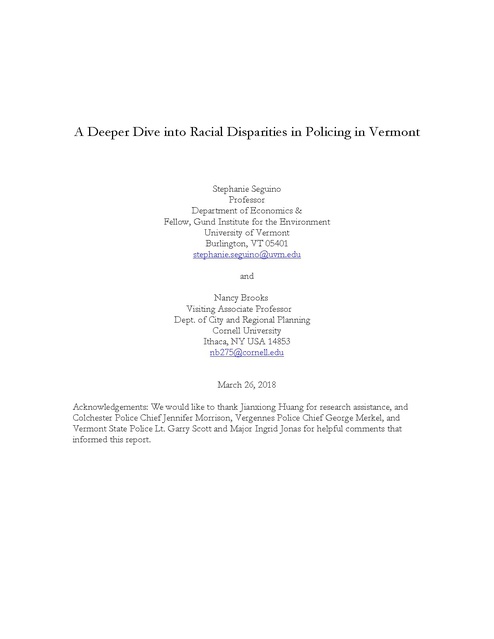
Document text
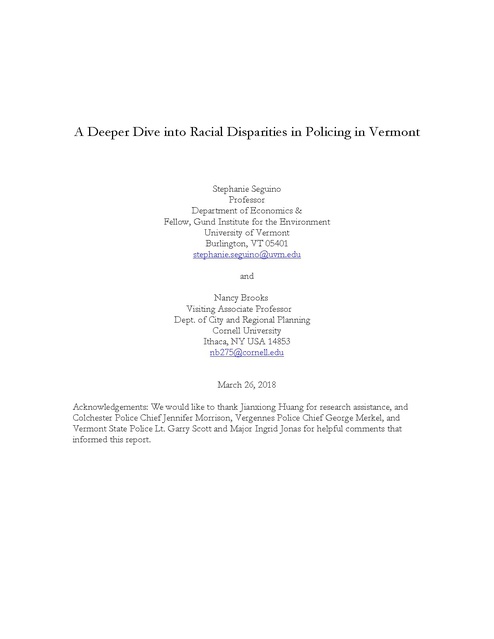
Document text
This text is machine-read, and may contain errors. Check the original document to verify accuracy.
A Deeper Dive into Racial Disparities in Policing in Vermont Stephanie Seguino Professor Department of Economics & Fellow, Gund Institute for the Environment University of Vermont Burlington, VT 05401 stephanie.seguino@uvm.edu and Nancy Brooks Visiting Associate Professor Dept. of City and Regional Planning Cornell University Ithaca, NY USA 14853 nb275@cornell.edu March 26, 2018 Acknowledgements: We would like to thank Jianxiong Huang for research assistance, and Colchester Police Chief Jennifer Morrison, Vergennes Police Chief George Merkel, and Vermont State Police Lt. Garry Scott and Major Ingrid Jonas for helpful comments that informed this report. A Deeper Dive into Racial Disparities in Policing in Vermont EXECUTIVE SUMMARY In 2014, the Vermont legislature passed a bill requiring all Vermont law enforcement agencies to collect traffic stop data so as to make it possible to identify and track any racial disparities in policing. The first round of data became available in 2016, and “Driving While Black and Brown in Vermont” (Seguino and Brooks 2017), an analysis of that data was released in January 2017. The report has generated wide-ranging conversations about the role of race in policing. Several Vermont law enforcement agencies have responded by taking the initiative to invest in training to address potential implicit bias in policing and to improve the quality of their data. At the same time, there have been questions raised by some observers about the quality of the data and methodology used in the 2017 study, and a concern that the study does not account for the context of traffic stops that may justify the racial disparities found in the original report. In this brief, we address these questions and report results from a logistic regression analysis that accounts for other factors beyond race that may influence the probability of being searched and of contraband being found. We also present new results of an analysis of race and the types of contraband found in 2016 Vermont State Police vehicle searches. A brief summary of our findings is as follows: • Data quality. A concern has been raised that the data are of poor quality and therefore should not be used to make inferences. Control for data quality lies with law enforcement agencies. There is room for improvement to ensure complete data and uniform methods of coding. These efforts appear to be underway. That said, we did not find evidence of serious miscoding or overt efforts to manipulate the data in the dataset law enforcement agencies provided. • Home state of driver and vehicle registration, and passenger data. There is a view that racial disparities in stops and post-stop outcomes would be smaller if the data analysis controlled for out-of-state drivers, vehicles, and passengers. That is possible, but law enforcement agencies have not provided that data to the public—nor does the legislation require it. Once that is made available, those variables can be included in the analysis. • The benchmarking issue. A perennial question in race data analysis in policing across the country is what is the appropriate denominator to use in calculating stop rates by race? The best practice nationally is to use data on the race of not-at-fault drivers in accidents, which we have done in our 2017 study. Our analysis also includes indicators based on post-stop outcomes, where the race of the driver is known (or, more precisely, at which time the officer has formed a perception of the race of the driver). By basing conclusions from analysis of multiple indicators instead of only one (such as the stop rates), we avoided the problem of “cherry-picking” indicators. This approach provides a more accurate assessment of the data in the event there are mismeasurement problems in any one of the indicators. Our assessment of racial disparities in policing then relies not on any one indicator, but the patterns across all 1 • • indicators. Insofar as those patterns are consistent across indicators, more robust conclusions can be drawn about the degree of racial disparities. The role of context in explaining racial disparities. In this report, we conducted logistic regression analysis to account for all the contextual factors that are available in the data that might influence the probability of being searched and of contraband being found. Controlling for these factors, we find that race continues to be a statistically significant factor in an officer’s decision to search a vehicle and in the probability of finding contraband. Wide racial disparities persist. Specifically, Black and Hispanic drivers continue to be roughly 2.5 to 4.0 times more likely to be searched that White drivers, and 30 to 50 percent less likely to be found with contraband subsequent to a search than White drivers. These findings indicate probable oversearching of Black and Hispanic drivers compared to White drivers. The analysis of Vermont State Police contraband data, resulting from 440 searches in 2016, provides an initial detailed racial analysis of the types of contraband found in searches based on probable cause or reasonable suspicion. Two striking features of these data are: 1) over 70 % of all contraband found is marijuana with 13% comprised on heroin, cocaine, or opioids, and 2) only White drivers were found with heroin, cocaine, and/or opioids. No drivers of any other race with found with this contraband. This suggests that, at least for this dataset for 2016, and for these types of searches, assumptions held by the public and law enforcement about the race of drivers carrying this type of contraband should be revisited. 2 A Deeper Dive into Racial Disparities in Policing in Vermont I. INTRODUCTION In early 2017, a report on racial disparities in Vermont policing was issued by the authors (“Driving While Black and Brown in Vermont”). Our goal was to assess the extent of racial differences in the experience of drivers on Vermont roads. The study included data from 29 law enforcement agencies, policing roughly 78% of the Vermont population.1 We utilized a methodology that is easily replicable so as to provide a format for data analysis that community groups, law enforcement, and policymakers could all use to monitor the evolution of racial disparities in policing. We reported on seven indicators that measure racial disparities: arrest and search rates by race, “hit” rates2 by race, racial shares of traffic stops by agency and officer as compared to racial shares of the driving population, racial differences in ticket rates, male shares of stops by race, and officer stop rates by race. In our analysis, we explored the data for a pattern across several indicators. The state-wide data showed that there were racial disparities across a number of indicators (disparities differed by agency, however), with Black and Hispanic drivers more likely to be stopped and arrested than White or Asian drivers. Of particular note, they were also significantly more likely to be searched but less likely to be found with contraband than White or Asian drivers. For reference, Table 1 reports several post-stop outcomes from the 2017 report for all Vermont law enforcement agencies combined. Panel A shows results for all years for which we had data and Panel B is for 2015 only. By basing conclusions drawn from the data analysis on multiple indicators instead of only one (such as the stop rates), researchers avoid the problem of “cherry-picking” those indicators that are consistent with their prior assumptions. This methodological approach, which is widely accepted in social science research, also provides a more accurate assessment of the data in the event there are mismeasurement problems in any one of the indicators. Mismeasurement may be due to miscoding, misunderstanding of the concept of discretionary stops (and other variables), missing data, or simple mistakes in recording. 1 Numerous agencies did not submit their data by September 2016 and so could not be included in our original analysis. 2 A “hit” is defined as a search of a vehicle that results in contraband being found. The hit rate then is the percentage of searches that are “productive,” that is, the percentage of searches in which contraband is found. 3 Table 1. Post-Stop Outcomes, All Agencies Panel A. All Years White Black Asian Hispanic Arrest rate 1.1% 2.0% 0.8% 1.5% Search rate 1.0% 3.9%* 0.6% 3.4%* Hit rate (includes all outcomes) 76.3% 62.6%* 77.8% 64.3%* Hit rate (outcome = arrest/ticket) 60.0% 49.9%* 79.4% 46.6%* White Black Asian Hispanic Arrest rate 1.2% 2.1%* 1.1% 1.3% Search rate 0.9% 3.6%* 0.5% 2.6%* 79.4% 72.8% 88.9% 75.0% Hit rate (% of searches with contraband) Panel B. 2015 only Hit rate (% of searches with contraband) Hit rate (includes all outcomes) 56.1%* Hit rate (outcome = arrest/ticket) 67.0% 88.9% 60.7% Note: Total stops for all years is 425,388 and for 2015, there were 107,497 stops. These data exclude externally generated stops and searches based on a warrant. Asterisks on arrest, search, and hit rates indicate statistically significant differences of a racial group as compared to White drivers at the 5 percent level. Our report has since generated numerous conversations and responses. On the one hand, some law enforcement agencies, communities, and legislators have used the results of our paper and methodology to further conversations about racial disparities and potential racial bias in policing. Some agencies (Vermont State Police and Burlington Police Department, in particular) have invested heavily in training to address potential implicit bias in policing and to improve the quality of their data. Other agencies have raised questions about the results of our study, concerned it does not account for the context of traffic stops that may justify the racial disparities founds in our original report. Worries have also been voiced about the quality of the data on which our study was based. This report addresses those concerns. We discuss the issue of data quality first. We then present additional analysis of the data, using logistic regression analysis to further explore the role of race by controlling for contextual factors that may be correlated with the race of the driver. Logistic regression analysis is a method for measuring the odds a particular outcome will occur, such as the search of a vehicle subsequent to a stop. This type of analysis allows us to identify how various factors (such as reason for the stop, and age and gender of the driver) contribute to those odds. In this way, we can control for the context of the event, and avoid misattributing to race the reason for a search (or other outcome). Finally, we take this opportunity to discuss results of a preliminary analysis of 2016 Vermont State Police data on types of contraband found in searches that sheds light on racial outcomes by type of contraband. 4 II. DATA ISSUES Several concerns have been raised about the quality of the data our analysis was based on. Questions about how the data were analyzed have also been indicated. We take this opportunity to respond to those questions. 1. Data quality and missing data: Police leaders have expressed concern that the data are of poor quality in the sense that some data is incomplete, and in some categories, there is not a uniform agreement across agencies (or officers) about what actual policing situations the data captured. (For example, one agency may record searches of passengers while another may only record searches of drivers). Police leadership sees the lack of uniform agreement across departments about the circumstances to be captured as an important issue, undermining their confidence in any analysis of those data. In addition to quality of the data, a major problem is that the law requiring traffic data collection by race has not been followed. The law (20 V.S.A. § 2366) requires that by or before September 1, 2014, every state, county, and municipal law enforcement agency is required to collect roadside stop data consisting of the following: 1) age, gender, and race of driver, 2) reason for the stop, 3) type of search conducted, if any, 4) evidence located, if any, and 5) outcome of the stop. All roadside stop data, as well as reports and analysis of roadside stop data, are to be made public. The law further required agencies by September 1, 2016 and annually thereafter, to provide the data collected to the vendor chosen by the Criminal Justice Training Council. A number of agencies did not report their data by the September 2016 due date, although some agencies subsequently submitted their data after that time. Table A1 in Appendix A lists agencies for which data was available and those whose data had not been submitted in time for this analysis. For those that did submit data, a number of required categories of data were missing from some agencies. A table of missing data by agency is provided in Table A2 in Appendix A. The question of whether racial disparities would disappear if quality were improved is an empirical one. It will be incumbent on agencies to provide accurate data and only then will we know the effect on racial disparities. Further, it should be noted that all data sets have some problems of quality, whether it is educational data, unemployment data, measures of GDP, or many others. This problem is not unique to the Vermont traffic policing dataset. (That said, of course, the negative impact of inaccurate data on perceptions of police are of great concern). Absent evidence of serious miscoding or overt efforts to manipulate the data, social science researchers use existing data to answer questions, and then typically offer advice on how to improve data quality. Were researchers to wait for perfect datasets, no analysis would ever be conducted. It is our professional opinion with over 50 years of combined experience in statistical analysis, that the size of the racial disparities found in our dataset should lead to deeper reflection about the role of race in policing in Vermont. A number of Vermont law enforcement agencies are 5 now actively engaged in improving their data quality. Over time, as quality improves, the task will be to analyze that data and to compare to earlier years to observe trends over time. An instructive example is the Vermont State Police, which has invested significant resources into improving data quality. Their analysis of their 2016 data reflects this improvement, and also shows a substantial decline in missing data.3 It is instructive that in 2016, racial disparities in stop, arrest, and search rates (notably, between Black and White drivers) continue to be large, although search rate disparities have narrowed from 2015. Another important issue is the inability of a number of Vermont law enforcement agencies to produce all the data required by law. Data reporting is new for a number of agencies that find themselves under-resourced and without the skilled staff to perform this work. Moreover, the legislation (cited above) requires law enforcement agencies to work with the Criminal Justice Training Council with the goals of collecting uniform data, adopting uniform storage methods and periods, and ensuring that data can be analyzed. That work appears to be underway, although funding challenges to address this problem exist. As a result, analyzing traffic stop data has until now been an arduous process because coding is not uniform and due to numerous instances of missing data. We are hopeful some of the quality issues are in the process of being resolved. 2. Duplicate records: A question was raised about whether our dataset, obtained from law enforcement agencies, included duplicate incidents. More specifically, the concern is that in cases of more than one outcome of a stop (for example, a driver may have been issued more than one ticket), the incident is recorded multiple times, one for each outcome. This could skew the results and erroneously contribute to data suggesting wider racial disparities if drivers of color were more likely to have more than one outcome per stop. We addressed that issue in our original report. Specifically, we “de-duplicated” our data through a complex programming procedure, relying on incident numbers, and matching of age, gender, date of birth, and time of day to identify duplicates.4 3. Home state of driver and vehicle registration: There is a view that racial disparities in stops and post-stop outcomes would be smaller if the data analysis controlled for out-ofstate drivers and vehicles. That is possible, but law enforcement agencies have not provided that data to the public—nor does the legislation require it. Once that is made available, that variable can be included in the analysis. 4. Search and contraband data may be inaccurate because data does not differentiate between passenger and driver. Some agencies have indicated that there is confusion about whether data on passengers should be recorded or only on the driver. This may affect results if the 3 Vermont State Police traffic policing data can be found on their website: http://vsp.vermont.gov/communityaffairs/trafficstops 4 Some agencies did not provide incident numbers, making the de-duplication process more complex. In the future, such data should be reported with incident numbers so that agencies can more easily monitor their own traffic stop data for racial disparities. 6 driver and passenger are of different races. We concur and have proposed that agencies begin to collect passenger data as well. 5. Small sample sizes. A concern has been raised about small sample sizes for some agencies, inhibiting the ability to make sound statistical inferences. The sample size in our 2017 study for our full dataset was over 400,000. For some analyses, we restricted our sample to data for 2015, yielding a sample size of over 107,000. These sample sizes are more than adequate to make reliable statistical inferences. We made no inferences on data from agencies if sample sizes were too small. We also conducted tests of statistical significance of all of our results. 6. Lack of controls for context. Concerns have been raised that were we to have controlled for driver age, gender, and reason for the stop, racial disparities would be smaller if not non-existent. For example, younger drivers are typically more likely to exhibit poorer quality driving than older drivers. If stopped Black or Hispanic drivers on average are younger than White or Asian drivers, failure to control for age may result in overstating the role of race in traffic policing. We address this concern in the next section where we show that even when these variables are controlled for, our original results stand: Black and Hispanic drivers are searched at a higher rate than White drivers, but are less likely to be found with contraband. 7. The benchmarking issue. A perennial concern with traffic data analysis is that researchers do not have an accurate measure of the driving population. In the absence of precise data (which would be very costly to obtain), many studies use the Census population as an estimate of the driving population. The best practice for measuring the driving population in calculating stop rates by race, however, is to use racial shares of not-atfault drivers in accident reports as the denominator in the stop rate calculation (Alpert, et al., 2004; McLean and Rojeck 2016). This is because not-at-fault drivers in accidents are considered to be a representative sample of the driving population.5 In our 2017 study, we use both the Census data and not-at-fault driver data by race from the Department of Motor Vehicles. The accident data capture the fact that the driving population in towns and on highways is at least partly comprised of transients (that is, non-residents traveling through a city or town). Using both measures, racial disparities exist to varying degrees in many of agencies in our sample. There is a good deal of variation in stop rates by race across agencies, and this in itself is notable. 8. Racial disparities and racial bias. There is a concern that evidence of racial disparities may be interpreted by the public as racial bias. The two concepts have different meanings. Racial disparities are the statistically significant differences in racial outcomes of policing. They may be due to a variety of factors, including contextual factors as well as implicit or unconscious officer bias. Therefore, evidence of racial disparities should signal to law enforcement agencies the importance of digging 5 Another method used to identify racial shares of the driving population is an observational traffic study, where surveyors stand on street corners, recording the perceived race of drivers. This approach is rarely used, in part because it is very expensive and also because it too has problems of accuracy due to changing driving populations over time. 7 deeper into police practices to understand the source of those disparities. The existence of racial disparities therefore does not in and of itself provide evidence of racial bias (defined as the case where the race of the driver influences officer decisions about whom to stop, ticket, arrest, or search). Evidence of disparities, therefore, should be interpreted with caution. That said, the “gold standard” used in the race and traffic policing literature for identifying racial bias is racial differences is hit rates (the percentage of searches that yield contraband) [Persico and Todd 2008]. Evidence that the Black or Hispanic hit rate is significantly lower than that of White drivers is interpreted as an indication of inefficient policing at best, and is consistent with a claim of biased policing. We found such evidence using the statewide data and for several agencies. In sum, higher quality data are always welcome in the world of social science research. But in the real world, data are imperfect. This does not preclude such data being used unless it is found to be so flawed as to be misleading, which is not our assessment of the the data we analyzed. In our 2017 report, we highlighted our concerns with quality of data and urged agencies and the state to take steps to address any lacuna or inconsistencies. A few agencies are taking steps to address this issue, with the most significant efforts being made by the Vermont State Police followed by Burlington Police Department. III. DO RACIAL DISPARITIES IN SEARCH RATES AND CONTRABAND FOUND DISAPPEAR WHEN WE CONTROL FOR CONTEXT? A. Background One of the apprehensions about the results of our 2017 report is that it does not take into account the context of the stop and of post-stop outcomes. That is, it is argued that if we controlled for time of day, gender, age, reason for stop, and other relevant variables, racial disparities would likely disappear. Our original report did not include such an analysis because our goal was to produce a data analysis methodology that is easily replicable and thus a useful tool for law enforcement agencies and for community groups who wish to monitor racial disparities in policing. It is worthwhile to note that our methodology reflects guidelines by a number of national experts on race data analysis (Fridell 2004). It is, however, reasonable to question whether context can explain racial disparities in traffic stop policing in Vermont. Some driving behaviors and circumstances may co-vary with race, and may be the dominant reason behind a search rather than the race of the driver. For example, perhaps people of color are more likely to be younger drivers, a group that typically exhibits poorer quality driving than older drivers. Age of the driver may contribute to the likelihood of a search for drivers of any race. If drivers of color are more likely to be young, compared to White drivers, then they would experience higher search rates on average. Failing to control for such factors risks misattributing search rate differences to race rather than the explicit behavior of the driver. If, even after controlling for factors like gender, age, reason for stop, and time of day, we still find that race is a statistically significant predictor of a search and a predictor of a lower likelihood that contraband is found, then that provides evidence that the race of the driver, independent of other factors, influences traffic policing in Vermont. 8 The way that researchers address the possibility that other factors may influence the probability of a driver being searched is to conduct multivariate logistic regression analysis. The goal in this methodology is to determine whether there are statistically significant differences in the probability of drivers being searched by race.6 Such analyses have been done for other states and for Vermont agencies (Seguino, Brooks, and Mitofsky 2012; Baumgartner et al., 2016; Pierson, et al., 2017), and have found that once context is controlled for, Black drivers (and in some cases, Hispanic drivers) are still more likely to be searched than White drivers. In this section, we report the results of the logistic regression analysis on our Vermont-wide data to respond to this concern. In our analysis, we use the data produced by the law enforcement agencies themselves upon which our 2017 study was based.7 8 B. Probability of Search Methodology Multivariate logistic regression analysis assesses the differential probability of being searched for Black, Hispanic and Asian drivers as compared to White drivers. The full model takes this general form: Probability of Search = β0 + βb*Black + βa*Asian βna*Native American + + βh*Hispanic + βi*Day of Weeki + βj*Reason for Stopj + βm*Male + βage*Age + βk*Time of Dayk + βl*Agencyl + Residual. Dummy variables for each racial group are included, with White the excluded category. In our model, the coefficients for each of the driver race variables can be interpreted as the odds of a search for a driver of that race as compared to the odds for White drivers Researchers cannot conduct this type of analysis on the probability of being stopped because we do not have data on who is not stopped. Although this analysis could theoretically be conducted on the probability of being arrested, we lack sufficient information about arrest reasons to make this analysis meaningful. Hence, studies that use this method to assess racial disparities in policing typically analyze the probability of being searched. We add to this an analysis of the probability of contraband being found, subsequent to a search. The reason for this is that the “gold standard” for identifying racial disparities and potential bias in traffic policing is a comparison of search and “hit” rates (Persico and Todd 2008). In particular, we would expect that the probability of finding contraband is equal across all racial groups, regardless of differences in search rates. The reason for this is that law enforcement agencies presumably have an interest in efficient use of their resources. If agencies are over searching a group such that it has a lower “hit” rate than other racial groups, we would expect that officers recognize the higher percentage of unproductive searches of that racial group and they would adjust their assessment of the criteria on which they base a search. 7 Numerous agencies did not submit their data by the September 2106 deadline. Some have subsequently submitted their data, but it is not included in this analysis because we want to assess the extent to which racial disparities in our 2017 report can be explained by other contextual factors. 8 Additional variables might have been useful to include in our analysis (discussed above), but Vermont law enforcement agencies are not required to report to the public data on: 1) the state in which the stopped vehicle is registered, 2) the year the vehicle was made (which might offer information on potential “poverty profiling”), 3) officer information (the age, race, gender, and job tenure of officers, for example), or 4) the specific type of contraband found during a search. Based on other studies done in the US that control for these variables, it is unlikely that our results would differ substantially, even if these data were available. Nevertheless, it would be useful for agencies to also report these data so as to better assess the role of race in policing. 6 9 with the same other characteristics. This is called the odds ratio, because it is the ratio of the odds of a non-White driver being searched over the odds that a White driver is searched.9 An odds ratio of 1 indicates equal probabilities of being searched. A ratio that is greater than one indicates a group is more likely to be searched than the omitted (or benchmark) group. Finally, an odds ratio that is less than 1 is indicative of a lower probability of a group being searched relative to the omitted group. We add driver age, measured in years, as an explanatory variable. The coefficient on Male indicates the odds a male driver will be searched as compared to the odds a female driver will be searched. The excluded category for the Reason for Stop set of variables is motor vehicle violation (such as speeding). The coefficients on the Reason for Stop variables indicate the odds of being searched for each reason for stop as compared to odds of being searched due to motor vehicle violations where the reason is one of the following: investigatory stop, suspicion of driving while under the influence (DWI), vehicle equipment, and for reasons unknown (that is, the reason was not stipulated in the incident report). This control can help to eliminate misattribution of race to search disparities, if for example, people of color are more likely to drive older vehicles with equipment problems, or are more likely to be DWI. Externally generated stops are excluded from this analysis because we want to focus on searches based on officer discretion.10 We also control for the day of the week and the time of day, with the excluded categories, respectively, Friday and afternoon. And finally, we control for law enforcement agencies. This helps to address the potential problem of unequal sample sizes by agency as well as the fact that agencies are located in different parts of the state with varying conditions that might influence search rates. Controlling for all of these factors allows us to interpret the race variable, net of the impact of the other control variables.11 We report results on four variations of our basic model. The need to run the regression with more than one specification is due to the missing data problem. Because some agencies did not report data on control variables such as gender or age, we lose those observations when we include those independent variables in our regression. In Model 1 in Table 2, we start with a basic model, in which race of the driver is our only explanatory variable (this specification is analogous to our crosstab analysis in our 2017 paper). The results show that, compared to White drivers, Black drivers are 3.994 times more likely to be searched than White drivers. (This represents the ratio of the odds of a Black driver being search compared to the odds of a White driver being searched). In contrast, Asian drivers are a little more than half has likely to be searched as White drivers. Native Americans are 2.83 times more likely to be searched, and Hispanic drivers 3.49 times more likely. 9 Appendix 2 provides a brief explanation of how to interpret odds ratios. 10 For this reason, we also exclude searches based on a warrant. 11 Omitted variable bias is still possible if race is correlated with variables that we are not able to include in the model. 10 In Model 2, adding controls for reason for stop and agency, we find that investigatory stops are more than 6.5 times as likely to lead to a search as a stop initiated due to a moving violation.12 Stops based on suspicion of DWI are almost 10 times more likely to lead to a search compared to a moving violation. Stops based on vehicle equipment are about 60% more likely to lead to a search than stops due to a moving violation. These results are statistically significant and not surprising. It is notable that the odds ratio by racial group changes very little with the addition of controls for reason for stop and agency. In particular, for example, the Black/White odds ratio changes just a small amount from 3.994 to 3.796. Asian, Native American, and Hispanic odds ratios are similarly stable when we control for reason for stop and agency. Coefficients on all racial variables continue to be statistically significant at the one percent level. That is, we can reject the null hypothesis that there is no difference in search rates by race with a high degree of certainty. In Model 3, we incorporate all available controls, adding gender and age of driver, time of day, and day of week to the independent variables in Model 2. Since not all agencies report these variables, the number of observations has decreased from roughly 409,000 to about 367,000. (If all of the agencies had reported gender, age, etc., we would not have bothered running the second specification). The odds ratio on age is below 1.0, meaning that the older the driver, the lower the probability of being searched. The odds ratio on the gender of the driver indicates that male drivers are more than twice as likely to be searched as female drivers. The time of day results show that the probability of being searched is lower in the morning than in the afternoon and about 28% greater at night than in the afternoon. The addition of these controls slightly reduces the odds ratio of Black drivers being searched compared to White drives to 3.2. For Hispanic drivers, the odds ratio also falls marginally to 3.022. 12 Law enforcement agencies that have reviewed their data entry practices, such as VSP, have indicated that some searches may not have been recorded. This may have happened if, for example, a driver was arrested for DWI, careless and negligent driving, or excessive speed. Subsequent to those arrests, a search may have been conducted. There may therefore be an undercounting of searches in the data up to this point, although there are efforts underway to rectify this issue. Because those were not discretionary searches, their omission may not have had a significant impact on the data, however. 11 Table 2. Probability of a Search, All Years Model 1 VARIABLES Race only Black Race Asian Native American Hispanic Day of Week Saturday 3.994*** (0.208) 0.559*** (0.094) 2.829*** (0.909) 3.498*** (0.322) 3.790*** (0.203) 0.562*** (0.095) 3.100*** (1.003) 3.326*** (0.310) 1.065 (0.056) 1.050 (0.058) 0.920 (0.051) 0.963 (0.0529) 0.947 (0.052) 1.031 (0.055) 6.564*** (0.390) 9.434*** (1.219) 3.508*** (0.391) 1.599*** (0.057) 0.010*** (0.001) 409,390 0.004*** (0.001) 408,872 Sunday Monday Tuesday Wednesday Thursday Reason for Stop Investigatory Stop Suspicion of DWI Unknown Vehicle Equipment Gender Male Age Age Time of Day Morning (4AM - Noon) Model 2 Race and partial controls Night (8PM - 4AM) Constant Observations Model 3 Race and all controls 3.210*** (0.189) 0.449*** (0.086) 3.427*** (1.123) 3.022*** (0.294) 0.979 (0.055) 0.950 (0.056) 0.947 (0.056) 0.981 (0.0579) 1.010 (0.059) 1.075 (0.060) 5.978*** (0.389) 8.637*** (1.175) 5.004*** (0.689) 1.432*** (0.054) 2.075*** (0.081) 0.942*** (0.002) 0.611*** (0.031) 1.281*** (0.046) 0.023*** (0.005) 367,045 Model 4 Race and all controls except agency 3.213*** (0.186) 0.478*** (0.091) 3.193*** (1.044) 3.387*** (0.327) 0.932 (0.052) 0.938 (0.055) 0.978 (0.057) 1.007 (0.0591) 1.038 (0.060) 1.110* (0.062) 5.735*** (0.359) 6.686*** (0.890) 4.491*** (0.564) 1.479*** (0.054) 2.088*** (0.081) 0.943*** (0.002) 0.649*** (0.032) 1.273*** (0.045) 0.034*** (0.002) 367,679 Note: Standard errors are in parentheses. * p<0.10, **p<0.05, ***p<0.01. Finally, in Model 4, the regression results include all controls except for law enforcement agency. As can be observed, this has very little effect on the odds ratio by racial group, except for Hispanics. There, we observe the odds of being searched rises to 3.387 for Hispanic drivers compared to White drivers. In Table 3, we report results from restricting our sample only to nighttime searches. The odds ratio of a Black driver being searched as compared to a White driver is 3.107, lower than for the full sample. The odds ratio for all other racial groups compared to White drivers falls as well. It is notable that adding the remainder of our controls (Models 2-4) has virtually no effect on the odds ratios by race. 12 Table 3. Probability of Search, Nighttime Only Model 1 VARIABLES Race Race Black Asian Native American Hispanic Saturday Sunday Monday Tuesday Wednesday Thursday Reason for Stop Model 2 Race and partial controls Investigatory Stop Suspicion of DWI Unknown Vehicle Equipment Age Age Gender Male Constant Observations 3.011*** (0.247) 0.403*** (0.113) 2.559* (1.329) 2.890*** (0.449) 0.941 (0.077) 1.174* (0.099) 1.236** (0.111) 1.049 (0.010) 1.076 (0.010) 1.237** (0.106) 5.734*** (0.569) 6.488*** (0.971) 2.817*** (0.542) 1.297*** (0.071) 0.947*** (0.002) 3.122*** (0.238) 0.441*** (0.108) 2.223 (1.144) 2.491*** (0.370) 0.0657*** (0.006) 101,748 0.0173*** (0.003) 112,247 Model 3 Race and all controls 7.333*** (0.680) 6.455*** (0.959) 2.159*** (0.334) 1.241*** (0.066) 3.011*** (0.260) 0.385*** (0.113) 2.635* (1.373) 2.551*** (0.406) 0.962 (0.081) 1.165* (0.101) 1.174* (0.109) 1.006 (0.098) 1.07 (0.101) 1.223** (0.107) 6.034*** (0.638) 7.976*** (1.233) 2.981*** (0.667) 1.274*** (0.073) 0.942*** (0.003) 1.780*** (0.106 0.0267*** (0.005) 99,156 Model 4 Race and all controls except agency 2.911*** (0.246) 0.389*** (0.114) 2.585* (1.345) 2.758*** (0.434) 0.946 (0.079) 1.171* (0.101) 1.215** (0.112) 1.025 (0.010) 1.093 (0.102) 1.259*** (0.110) 5.701*** (0.585) 6.594*** (0.991) 2.872*** (0.595) 1.331*** (0.074) 0.945*** (0.002) 1.793*** (0.106 0.0249*** (0.004) 99,350 Note: Standard errors are in parentheses. * p<0.10, **p<0.05, ***p<0.01. For purposes of comparison with our 2017 study, we repeat the logistic regression analysis, limiting our sample to data for 2015 only, the year for which we have full data from all agencies. Those results are shown in Table 4. In Model 1, where we control only for race, Black drivers are 3.871 times more likely to be searched than White drivers. The odds of being searched for Asian relative to White drivers are slightly more than one half (they are half as likely to be searched, that is). And Hispanic drivers are 2.95 times more likely to be searched, compared to White drivers. Models 2-4 show that the odds ratio of a Black driver being searched falls slightly but is still high, ranging from 2.6 times to 3.5 times, depending on the model’s controls. The odds of Asian drivers being searched compared to White drivers falls even more with the added controls, while the odds ratio of a Hispanic compared to White driver ranges from 3.0 to 3.5 times more likely, depending on the model used. (The number of Native American drivers stopped and searched in 2015 is too small to be able to make reliable inferences). The odds ratios reported here for each racial group can be compared to our 2017 results in Table 1. 13 Table 4. Probability of Search, 2015 Model 1 VARIABLES Race Model 3 Race and all controls Model 4 Race and all controls except agency 3.871*** (0.385) 0.529* (0.177) 2.950*** (0.555) 3.455*** (0.357) 0.531* (0.179) 2.607*** (0.498) 1.272** (0.133) 1.226* (0.138) 1.007 (0.116) 1.148 (0.127) 0.935 (0.107) 1.034 (0.114) 7.618*** (0.831) 8.087*** (2.107) 5.672*** (0.940) 1.253*** (0.0964) 0.010*** (0.0003) 104,596 0.003*** (0.001) 100,916 2.651*** (0.327) 0.310*** (0.140) 2.493*** (0.510) 1.178 (0.138) 1.149 (0.144) 1.075 (0.139) 1.106 (0.142) 1.019 (0.131) 1.136 (0.139) 6.472*** (0.795) 7.178*** (2.042) 6.294*** (1.247) 1.074 (0.0947) 1.721*** (0.136) 0.944*** (0.00313) 0.436*** (0.0530) 1.298*** (0.0980) 0.018*** (0.007) 81,772 2.755*** (0.333) 0.352** (0.159) 3.025*** (0.612) 1.126 (0.131) 1.141 (0.141) 1.089 (0.139) 1.130 (0.143) 1.038 (0.132) 1.177 (0.142) 6.318*** (0.729) 6.442*** (1.794) 7.828*** (1.394) 1.153* (0.0969) 1.734*** (0.136) 0.945*** (0.00309) 0.454*** (0.0539) 1.325*** (0.0961) 0.034*** (0.005) 85,440 Race only Black Asian Hispanic Day of Week Model 2 Race and partial controls Saturday Sunday Monday Tuesday Wednesday Thursday Reason for Stop Investigatory Stop Suspicion of DWI Unknown Vehicle Equipment Gender Male Age Age Time of Day Morning (4AM - Noon) Night (8PM - 4AM) Constant Observations Note: Standard errors are in parentheses. * p<0.10, **p<0.05, ***p<0.01. Figure 1 visually represents the relative likelihood of a search occurring by racial group as compared to White drivers. Panel A shows results from Table 2 for all years and Panel B shows results for 2015 only (from Table 4). 14 Figure 1. Relative Odds of Search by Race Panel A. All Years 4.5 4.0 3.8 4.0 3.5 3.3 3.5 3.2 3.4 3.2 3.0 3.0 2.5 2.0 1.5 1.0 0.6 0.6 0.4 0.5 Race only Race, some controls Race, all controls Race, all controls except agency 0.5 0.0 Black Asian Hispanic Same odds as White drivers Panel B. 2015 Only 4.5 4.0 3.9 3.5 3.5 3.0 3.0 3.0 2.6 2.8 2.7 2.5 2.5 2.0 1.5 1.0 0.5 0.5 0.5 0.0 Race only Black Race, some controls Asian 0.3 0.4 Race, all controls Race, all controls except agency Hispanics Same odds as White drivers Note: See Tables 2 and 4 for exact odds-ratios. The horizontal (purple) line indicates the situation of a non-White racial group having the same odds of being searched as Whites. Columns that exceed that bar indicate higher odds for a racial group being searched compared to White drivers, and below the bar indicates a lower probability of a search occurring for that racial group compared to White drivers. 15 Taken together, this evidence suggests that racial disparities in search rates are extremely robust at the state level, regardless of the contextual factors controlled for. (As we noted earlier, the ability to make inferences about search rates disparities at the agency level depends on the sample size. Only four agencies have sufficient data on which to make statistical inferences about search rates disparities—Burlington, Rutland, South Burlington, and VSP). Moreover, the levels of disparity indicated by the logistic regressions are very similar to those suggested by the search rate ratios in our original 2017 study (see Table 1). The use of more rigorous statistical techniques does not in any meaningful way change the nature of our 2017 findings. They simply reinforce the stark racial differences we reported in our earlier study. C. The Probability of Finding Contraband We conduct logistic regression analysis to assess the role of race in the probability of finding contraband, subsequent to a search. In our 2017 study, we found that Black and Hispanic drivers were less likely to be found with contraband than White or Asian drivers. As in the analysis of search rates, we control for other factors that may influence the probability of contraband being found to avoid erroneously attributing to race the effect of other factors. Again, we exclude externally generated stops and searches based on a warrant. The equation we estimate is as follows: Probability of Finding Contraband = β0 + βb*Black + βa*Asian βna*Native American + βh*Hispanic + βi*Day of Weeki + βj*Reason for Stopj + βm*Male + βage*Age + βk*Time of Dayk + βl*Agencyl + Residual. Table 5 reports the results of the probability of contraband found for searches for any outcome of the stop and search (that is, in which the result was a warning, a citation, or an arrest) for all years for which we have data.13 The results shown for Model 1, where the only explanatory variable is race of the driver, indicate that Black drivers are less than half as likely to be found with contraband, subsequent to a search, as White drivers. There is no statistically significant difference in the probability of Asian drivers being found with contraband compared to White drivers, while Hispanic drivers’ likelihood of being found with contraband is roughly 50% less than White drivers’. The addition of controls in Models 2-4 does not in any meaningful way alter the odds ratios of finding contraband in searches of Black, Asian, and Hispanic as compared to White drivers. 13 Results for 2015 from our 2017 study indicated that hit rate disparities were slightly smaller and were statistically significant only for contraband resulting in an arrest or ticket. 16 Table 5. Probability of Contraband, All Outcomes Model 1 Black Asian Native American Hispanic Day of Week Model 3 Race and all controls Model 4 Race and all controls except agency 0.484*** (0.058) 0.993 (0.449) 0.319* (0.206) 0.492*** (0.102) 0.524*** (0.066) 0.894 (0.412) 0.306* (0.201) 0.442*** (0.096) 0.667*** (0.010) 0.872 (0.141) 0.631*** (0.097) 0.579*** (0.088) 0.636*** (0.097) 0.718** (0.108) 1.195 (0.190) 0.995 (0.360) 1.098 (0.299) 1.057 (0.010) 4.700*** (0.204) 4,197 3.696*** (0.964) 4,189 0.468*** (0.064) 0.686 (0.350) 0.385 (0.264) 0.409*** (0.091) 0.668** (0.109) 0.844 (0.149) 0.623*** (0.103) 0.598*** (0.099) 0.611*** (0.099) 0.682** (0.109) 1.284 (0.232) 1.034 (0.377) 0.669 (0.250) 1.080 (0.109) 1.253** (0.133) 0.971*** (0.00408) 0.774* (0.103) 0.939 8.588*** (4.480) 3,715 0.451*** (0.059) 0.764 (0.384) 0.399 (0.273) 0.459*** (0.098) 0.745* (0.117) 0.869 (0.148) 0.689** (0.111) 0.625*** (0.100) 0.656*** (0.103) 0.737** (0.115) 1.218 (0.210) 0.854 (0.277) 0.423*** (0.137) 1.016 (0.099) 1.259** (0.129) 0.972*** (0.004) 0.687*** (0.0871) 0.869 13.71*** (2.683) 3,721 Race only VARIABLES Race Model 2 Race and partial controls Saturday Sunday Monday Tuesday Wednesday Thursday Reason for Stop Investigatory Stop Suspicion of DWI Unknown Vehicle Equipment Gender Male Age Age Time of Day Morning (4AM - Noon) Night (8PM - 4AM) Constant Observations Note: Standard errors are in parentheses. * p<0.10, **p<0.05, ***p<0.01. We re-ran the regressions, focusing on contraband that resulted in issuance of a citation or in an arrest. Minor cases of contraband, such as an open container or a juvenile in possession of cigarettes, were coded as no contraband. The results, shown in Table 6, indicate that by recoding warnings as no contraband, the probability of Black and Hispanic drivers being found with contraband is similar to when all types of contraband are included. In this regression, we found that searches following investigatory stops were more correlated with serious contraband being found compared to stops due to moving violations. Searches following stops due to vehicle equipment problems were less likely to result in contraband being found that triggers a ticket or arrest. 17 Table 6. Probability of Contraband, Citations and Arrests Only VARIABLES Race Black Asian Native American Hispanic Day of Week Saturday Model 1 Model 2 Model 3 Race only Race and partial controls Race and all controls 0.612*** (0.070) 2.077* (0.903) 0.503 (0.322) 0.498*** (0.100) 0.842 (0.101) 0.971 (0.124) 0.817 (0.103) 0.713*** (0.0891) 0.791* (0.0971) 0.726*** (0.086) 0.550*** (0.069) 1.574 (0.750) 0.563 (0.367) 0.429*** (0.090) 0.902 (0.116) 1.018 (0.140) 0.832 (0.110) 0.722** (0.0966) 0.754** (0.0975) 0.707*** (0.088) 0.508*** (0.061) 1.580 (0.745) 0.574 (0.371) 0.422*** (0.084) 0.982 (0.122) 1.071 (0.142) 0.908 (0.117) 0.737** (0.0951) 0.765** (0.0958) 0.733** (0.089) 1.781*** 1.754*** 1.651*** (0.251) 1.087 (0.341) 0.863 (0.205) 0.656*** (0.050) (0.272) 1.029 (0.325) 0.575 (0.195) 0.645*** (0.052) 1.316*** (0.115) 0.988*** (0.004') 0.839 (0.0942) 0.988 (0.0801) 3.787*** (1.823) 3,721 (0.242) 0.984 (0.275) 0.458*** (0.134) 0.626*** (0.049) 1.339*** (0.113) 0.988*** (0.004) 0.729*** (0.0777) 0.933 (0.0713) 2.921*** (0.455) 3,721 0.538*** (0.058) 2.087* (0.888) 0.541 (0.343) 0.471*** (0.089) Sunday Monday Tuesday Wednesday Thursday Reason for Stop Investigatory Stop Suspicion of DWI Unknown Gender Vehicle Equipment Male Age Age Time of Day Morning (4AM - Noon) Night (8PM - 4AM) Constant Observations 1.848*** (0.064) 4,197 0.666* (0.161) 4,195 Model 4 Race and all controls except agency Note: Standard errors are in parentheses. * p<0.10, **p<0.05, ***p<0.01. Using the data reported in Tables 5 and 6, Figure 2 visually portrays these odds ratios. Panel A gives the odds ratios of being found with contraband for Blacks, Asians, and Hispanics compared to White drivers for all outcomes of a stop and search, and Panel B reports odds ratios for those cases where the outcome was a citation or an arrest only. In both panels, it can be observed that Black and Hispanic drivers are significantly less likely to be found with contraband than White drivers. The Asian/White odds ratio differs, however, depending on the controls. 18 Figure 2. Relative Odds of Contraband Found by Race, All Years Panel A. All Contraband Found 1.2 1.0 1.0 0.9 0.8 0.6 0.8 0.7 0.5 0.5 0.5 0.4 0.5 0.4 0.5 0.5 0.4 0.2 0.0 Race only Black Race, some controls Asian Race, all controls Hispanic Race, all controls except agency Same odds as White drivers Panel B. Contraband Leading to Citation or Arrest 1.4 1.22 1.14 1.2 1.0 0.85 0.8 0.6 0.44 0.42 0.49 0.39 0.4 0.43 0.45 0.47 0.34 0.38 0.2 0.0 Race only Black Race, some controls Asian Race, all controls Hispanic Race, all controls except agency Sames odds as White drivers Note: See Tables 5 and 6 for exact odds ratios. The horizontal (purple) line indicates the situation of the racial group having the same odds of being found with contraband as Whites. Columns that exceed that bar indicate higher odds for a racial group to be found with contraband compared to White drivers, and below the bar indicates a lower probability of a contraband being found compared to White hit rates. The data in Panel A are for all outcomes of the search, while in Panel B, data are for contraband that led to a citation or an arrest. Externally generated stops are excluded as are searches on warrant and arrests on warrant. To sum up the results of the logistic regressions, adding controls for a variety of contextual factors has little effect on racial disparities in the probability of being searched and of contraband being found during a search. This is not to say that the controls were not 19 meaningful or significant. Searches and the likelihood of finding contraband are more likely to happen under some conditions as compared to others (e.g., during investigatory stops as compared to motor vehicle stops). But even controlling for these factors, race continues to be a statistically significant factor in an officer’s decision to search a vehicle. Moreover, and with regard to the question of racial bias as an explanation for such disparities, the analysis shows that Black and Hispanic drivers are less likely to be found with contraband, a finding that is consistent with oversearching of those groups. These results are consistent with those reported in our 2017 analysis. IV. 2016 VERMONT STATE POLICE CONTRABAND DATA In traffic stop reports made available to the public, Vermont law enforcement agencies identify searches that result in contraband being found, but do not indicate what that contraband is. Vermont State Police (VSP) generously agreed, however, to provide details on the type of contraband found in 2016 searches as well as the degree of the offense (civil or criminal). The dataset provided by the VSP includes the gender, race, and age of the driver, the reason for the stop, and outcome of the stop.14 These data are useful to better understand both the types of contraband found in searches and the racial composition of drivers found with various types of contraband. This is especially important since Vermont police officers frequently state that racial disparities in policing (and searches) are linked to concerns about drug trafficking, especially along the borders of Vermont with concerns about drugs entering from people of color from out-ofstate. The VSP dataset is comprised of 369 unique incidents in which contraband was found during a search.15 The total number of searches was 440. We coded the contraband data into 8 groups. Those groups are summarized in Table 7 along with the percentage of searches with each type of contraband. The data include arrests on warrant in order to provide a full spectrum of the types and relative importance of various types of contraband found in discretionary searches.16 (In total, there were six incidents with an arrest based on a warrant, all of White drivers). 14 Data on city of the stop, agency, stop date, day, and time were also provided, although we do not analyze those data here. 15 The data exclude externally generated stops and searches based on warrants. 16 By discretionary, we mean searches in which troopers made a decision to search, based on their assessment of the evidence. 20 Table 7. Types of Contraband Number of incidents Percentage of Total Cigarettes 1 0.3% Cash/Counterfeit money 1 0.3% Alcohol 23 6.2% Drug Paraphernalia 39 10.6% Opioids 9 2.4% Marijuana (includes + drug paraphernalia) 264 71.5% Marijuana + opioids, LSD, mushrooms, alcohol in any combination 5 1.4% Cocaine/Heroin 28 7.6% Total 369 100.0% Type of Contraband Marijuana is the most frequently identified type of contraband, comprising 71.5% of all searches in which contraband was found. This is followed by drug paraphernalia17 (10.6%), and then cocaine/heroin (7.6%). Opioid contraband comprised 2.4% of incidents. It is useful to clarify Vermont law on marijuana possession in effect in 2016. Vermont decriminalized possession of marijuana in 2013, removing criminal penalties on small amounts and replacing them with civil fines. Possession of more than an ounce of marijuana remains a criminal offense.18 It is, however, still common practice for police across Vermont to request consent to search or apply for a warrant when they smell the odor of or see evidence of marijuana use in plain view. This is because driving under the influence continues to be an offense and therefore is considered a public safety matter. Further, it remains a crime to sell marijuana, and an officer may conduct a search to determine how much marijuana is contained in a vehicle. Table 8 shows racial shares of all contraband cases and degree of offense (civil vs. criminal).19 White drivers comprised 91.3% of all drivers with contraband in 2016, and a higher percentage of all drivers with criminal quantities and types of contraband (96.9%). Black drivers were 5.9% of all drivers with contraband and 3.1% of drivers with criminal Drug paraphernalia is not automatically considered contraband. If it contains residue, it would be illegal. On the other hand, a new glass blown pipe unused is not illegal and would not be counted as contraband. 18 The law has since changed. In January 2018, Vermont legalized recreational marijuana, allowing adults over the age of 21 to possess an ounce of marijuana and to possess up to two mature plants and four immature plants in their home. The law stipulates that people convicted of possessing more than one ounce of marijuana, or more than two mature and four immature plants, can be imprisoned up to six months and fined $500 unless they participate in a court diversion program. Impaired driving remains illegal under the new law, and neither drivers nor passengers are allowed to use marijuana in a vehicle. Anyone with marijuana in a vehicle can be fined $200. 19 Criminal and civil offenses differ in terms of their punishment. Criminal cases may have jail time as punishment, whereas civil cases generally only result in monetary damages or a requirement to do or not do something. 17 21 amounts and types of contraband. No Asian or Hispanic drivers were found with contraband that led to a criminal offense. It should be noted that the sample sizes for Asians, Blacks, and Hispanics are too small to make statistical inferences. These numbers are therefore illustrative; more data would be required to identify statistically significant differences in racial shares of incidents and criminal incidents. Table 8. Racial Shares of Incidents by Degree Number Civil Criminal Share of all incidents Asian 2 2 0 0.5% Share of criminal incidents 0.0% Black 22 20 2 5.9% 3.1% Hispanic 8 8 0 2.2% 0.0% White 337 274 63 91.3% 96.9% Total 369 304 65 100.0% Note: Data do not include searches with arrests on warrant. 100.0% Table 9 provides data on racial shares of three key types of contraband: marijuana, heroin/cocaine, and opioids. The majority of marijuana incidents were civil offenses (93.8%). White drivers comprised 93.3% of the incidents in which criminal quantities of marijuana were found, and Black drivers were 6.7%. Again, it is worth noting that the small sample sizes, particularly for Blacks, indicates these data should be interpreted with caution. More data would be required to make sound statistical inferences from these data. In the case of heroin/cocaine and opioids, all incidents resulted in criminal offenses, and all were of White drivers. No Black, Asian, or Hispanic drivers were found to be in possession of heroin/cocaine or opioids in the searches conducted by Vermont State Police in 2016.20 Table 9. Racial Shares of Marijuana, Heroin/Cocaine, and Opioid Contraband Marijuana Heroin/Cocaine Opioids Civil Criminal Racial Shares of Criminal Civil Criminal Racial Shares of Criminal Civil Criminal Racial Shares of Criminal Asian 1 0 0.0% 0 0 0% 0 0 0% Black 17 1 6.7% 0 0 0% 0 0 0% Hispanic 4 0 0.0% 0 0 0% 0 0% White 228 14 93.3% 0 Note: These data do not include arrests on warrant. 31 100% 0 0 9 Race 100% In sum, the 2016 VSP contraband data are best understood as illustrative. More data would be needed (and from more agencies) to draw statistically sound inferences with regard to the 20 Of the five incidents in which marijuana was found in some combination with opioids, LSD, mushrooms, alcohol in any combination, four incidents involved White drivers and one a Black driver. 22 relationship between race and contraband. That said, the data offer a means to compare and contrast assumptions held by officers in some local law enforcement agencies (though not VSP) with regard to contraband and race. The data presented here suggest that some of those assumptions may be inaccurate, at least with regard to contraband that turns up during discretionary searches. The notable highlights of these data are as follows: • Marijuana is overwhelmingly the most frequently identified contraband in VSP searches in 2016, comprising 71.5% of contraband found subsequent to a search. Of those incidents, only 5.6% were criminal cases. The remainder were civil. • Heroin/cocaine and opioids combined were found in 10% of all searches yielding contraband. White drivers comprised 100% of those found with these types of contraband. V. CONCLUSION Vermont has embarked on a long-term project of using data to expand awareness of traffic policing and race. Because traffic stops are the most frequent interaction people have with the police, combined with the large number of traffic stops in any given year, data on stops can be a useful tool for understanding the extent of racial disparities in these interactions. They are, in other words, a way of holding up a mirror to ourselves. Though data often and usually are imperfect, that does not preclude their usefulness. In this report, we have discussed an array of concerns with traffic stop data quality, many of which have been shared with us by police. Efforts to improve data quality are important and should continue to be pursued. It is clear that there are a number of agencies pursuing that goal. In the interim, however, the data we do have are useful at gauging racial disparities in policing and give no evidence of being so systematically flawed that they are unusable. In this report, we provide details on a statistical analysis that controls for other factors that may influence the probability of being searched or of contraband being found during a search. Those results demonstrate that while other factors also contribute to the likelihood of either of those outcomes, racial disparities continue to exist when those factors are controlled for. In particular, Black and Hispanic drivers in Vermont are substantially more likely to be searched than White or Asian drivers, and are less likely to be found with contraband. The levels of disparity indicated by the logistic regressions are very similar to the search and hit rate ratios in our original 2017 study. The use of more rigorous statistical techniques therefore does not alter the nature of our 2017 findings. These disparities should be of great concern to law enforcement agencies, communities, and legislators. While the disparities in no way suggest that agencies are intentionally profiling people of color, they do indicate the necessity for law enforcement to be selfreflective about their policing practices and to interrogate the role of implicit bias in decision-making. Research shows that implicit racial bias is evident in numerous domains, not just policing. As its name suggests, it is often unconscious rather than intentional. Several agencies have planned or are planning implicit bias trainings, a positive step to work toward fair and unbiased policing in Vermont. The Vermont State 23 Police has gone beyond this to rigorously examine a wide array of practices, procedures, and policies to ensure fair and impartial policing at every level. Finally, with regard to the descriptive analysis of 2016 VSP contraband, it is instructive that for searches turning up heroin, cocaine, and opioids, drugs that are so much in the Vermont news of late, only White drivers were found with such contraband. There may be other aspects of drug trafficking in Vermont not reflected in these data. But the data tell us that in terms of discretionary searches in the course of traffic policing, the stereotype, held by society as a whole, that people of color are more likely to be drug traffickers is erroneous. 24 APPENDIX A Table A1. Agencies Represented in 2017 Study Agencies Included in 2017 Study Addison County Sheriff Agencies Not Included in 2017 Study Bellows Falls Pittsford Barre City Bennington County Sheriff Richmond Barre Town Berlin Royalton Bennington Bradford Rutland Town Brandon Brighton Shelburne Brattleboro Caledonia County Sheriff Stowe Bristol Canaan Swanton Burlington Castleton Thetford Colchester Chester VT Dept. of Fish and Wildlife Essex Chittenden County Sheriff VT Dept. Of Motor Vehicles Grand Isle County Sheriff Dover Washington County Sheriff Hinesburg Essex County Sheriff Waterbury Manchester Fair Haven Weathersfield Middlebury Fairlee Wells Constabulary Milton Franklin County Sheriff Wilmington Montpelier Hardwick Windham County Sheriff Northfield Hartford Windsor County Sheriff Randolph Killington Winhall Rutland Lamoille County Sheriff Woodstock Rutland County Sheriff Ludlow Springfield Lyndonville St. Albans Mendon Constabulary St. Johnsbury Morristown UVM Newport Vergennes Norwich VSP Orange County Sheriff Williston Orleans County Sheriff Note: Those agencies in italics provided data but it was incomplete or in a format that was unusable. 25 Table A2. Missing Values in Data Submitted by Agencies Agency Addison County Sheriff Barre City Race Reason for Stop Outcome Search Search Outcome Gender Age Time of Day 6,020 17.4% 0.3% 0.3% 0.2% 100.0% 0.2% 17.5% 0.0% 602 2.8% 4.3% 3.5% 10.8% 11.3% 0.0% 0.0% 100.0% Barre Town 2,852 0.1% 0.5% 0.0% 0.0% 0.0% 0.1% 0.1% 0.0% Bennington 5,213 3.0% 0.4% 0.4% 0.4% 2.7% 1.2% 97.5% 0.0% Brandon 2,010 10.0% 15.8% 5.7% 7.3% 7.4% 6.2% 5.8% 0.0% Brattleboro 7,945 11.5% 0.3% 1.4% 1.4% 1.6% 0.2% 0.3% 0.0% Bristol 576 8.7% 4.2% 3.0% 0.0% 0.0% 1.0% 2.6% 0.0% Burlington 24,850 4.8% 3.2% 1.1% 1.8% 1.8% 2.1% 1.7% 0.0% Colchester 7,116 3.2% 1.5% 0.4% 0.0% 0.0% 0.1% 90.4% 0.0% Essex 4,987 3.1% 2.1% 0.5% 1.9% 2.0% 2.1% 100.0% 0.0% Grand Isle 0.0% 0.0% 0.0% 0.0% 0.0% 0.0% 0.0% 4,481 0.0% Hinesburg 918 0.0% 0.0% 0.0% 0.0% 0.0% 0.0% 0.0% 0.0% Manchester 2,885 3.8% 15.7% 14.3% 19.0% 20.1% 0.5% 0.4% 0.0% Middlebury 1,465 0.8% 3.7% 0.1% 0.1% 1.6% 0.1% 0.0% 0.0% Milton 5,672 4.2% 2.2% 1.9% 2.2% 2.2% 3.7% 3.6% 0.0% Montpelier 4,147 7.6% 5.7% 1.4% 1.3% 1.8% 0.8% 0.5% 0.0% Northfield 617 0.8% 1.3% 1.5% 1.3% 1.0% 0.7% 100.0% 0.0% Randolph 292 1.4% 1.7% 0.0% 7.2% 7.2% 0.0% 0.0% 100.0% Rutland 13,523 2.6% 0.0% 0.0% 0.0% 0.0% 0.4% 4.1% 0.0% Rutland County Sheriff 4,384 3.7% 8.0% 1.5% 100.0% 100.0% 2.4% 0.5% 0.0% S. Burlington 11,644 0.5% 0.7% 0.1% 0.2% 0.2% 100.0% 100.0% 0.0% Springfield 6,356 0.0% 0.0% 0.0% 0.0% 0.0% 0.0% 0.0% 0.0% St. Albans 7,657 28.9% 6.2% 3.5% 3.9% 100.0% 3.7% 1.3% 0.0% St. Johnsbury 4,175 2.5% 1.7% 3.0% 1.7% 1.5% 0.5% 0.3% 0.0% UVM 8,851 1.3% 28.8% 1.7% 1.8% 1.9% 62.1% 62.2% 0.0% Vergennes 1,808 2.2% 0.8% 0.1% 0.1% 0.6% 0.0% 4.6% 0.0% Williston 11,912 1.0% 0.8% 1.8% 0.9% 1.0% 0.5% 4.9% 0.0% Winooski 5,283 5.4% 10.8% 8.0% 8.6% 9.1% 100.0% 3.2% 0.0% 283,285 1.7% 0.8% 0.8% 0.8% 1.0% 0.6% 0.4% 0.0% 2.9% 2.0% 1.1% 2.1% 5.4% 5.8% 8.8% 0.2% VSP Percent missing data Total Incidents 26 APPENDIX B A Primer on Interpreting Odds and Odds-ratios The first column of Table 5 with the odds ratios is copied below with an additional column showing the difference in the probability of finding contraband for each race group relative to White drivers. These are the numbers used in the example below. The Odds of an outcome occurring as a result of a police stop is defined as the ratio of the probability of that outcome occurring over the probability of that outcome not occurring. In the case of contraband being found subsequent to a search, the probability of finding contraband when the driver is White is 0.8113 and thus the probability of not finding contraband is 0.19. The odds of finding contraband when the driver is White are then 0.81/0.19 = 4.3 to 1. If the driver is Black, the probability of finding contraband is 13 percentage points lower (0.132 from table below), so the probability of finding contraband is 0.68 for a black driver and the probability of not finding contraband is 0.32. The odds of finding contraband when the driver is Black are 0.68/0.32 = 2.125 to 1. The Odds Ratios reported in the regression equations are the ratios of odds. Continuing our example, in Table 5, the first number in the first column is 0.484. This number is the odds ratio for a Black driver relative to a White driver. Or in other words, it is the odds for a Black driver divided by the odds for a White driver, thus 0.484 is equal to 2.125/ 4.32 (slight difference due to rounding). Thus, for a Black driver, the odds of a search yielding contraband is less than half the odds for a White driver. Table B1. Odds-Ratio Example with Contraband Found Variables y = Pr(ContrabandFound) (for benchmark group= White) Race only Marginal effects 0.8113 Black 0.484*** -0.132*** Asian -0.0576 -0.025 0.993 -0.001 Native American -0.449 -0.069 0.319* -0.233 -0.206 -0.157 0.492*** -0.131** -0.102 -0.044 Hispanic Note: Data are from Table 5, Model 1. Similarly, in the case of the search regressions, the baseline probability of being searched when the driver is White is a little over 1% (0.01058 in the table below). The odds of being searched are 0.01058/0.98942 = 0.01 to 1 which, in this case, is not very different from the 27 search rate itself. This will be true when the probability is very low. The search rate for a Black driver is almost 3 percentage points higher (0.029 from table below) than that White search rate of 0.01058. Consequently, the odds of a black driver being searched are (0.029+0.01058)/0.96042 = 0.04 to 1. The ratio of these odds, with a little rounding, is estimated to be 0.04/0.01 while the precise odds ratio is 3.994. A Black driver is almost 4 times as likely to be searched as a White driver in Vermont. Table B2. Odds-Ratio Example with Searches Variables y = Pr(Searched) for benchmark group = White) Black Asian Native American Hispanic Note: Data are from Table 2, Model 1. Marginal effects Race only 28 0.01058 3.994*** 0.029*** -0.208 -0.0019 0.559*** -0.005*** -0.0939 -0.001 2.829*** 0.019* -0.909 -0.009 3.498*** 0.025*** -0.322 -0.003 REFERENCES Alpert, G., M. Smith, and R. Dunham. 2004. “Toward a Better Benchmark: Assessing the Utility of Notat-Fault Traffic Crash Data in Racial Profiling Research.” Justice Research and Policy 6(1): 43-69. Baumgartner, F., L. Christiani, D. Epp, K. Roach, and K. Shoub. 2017. “Racial Disparities in Traffic Stop Outcomes.” Duke Forum for Law and Social Change 9: 21-53. Farrell, A., L. Rumminger, and J. McDevitt. 2005. New Challenges in Confronting Racial Profiling in the 21st Century: Learning from Research and Practice. Northeastern University Institute on Race and Justice. Fisher, D., M. Fargen, and V. Morris. 2017. “2016 Traffic Stops in Nebraska: A Report to the Governor and the Legislature on Data Submitted by Law Enforcement.” Nebraska Crime Commission. https://ncc.nebraska.gov/sites/ncc.nebraska.gov/files/doc/Traffic_Stops_in_Nebraska_COMPLETE_F INAL_0.pdf Fridell, L. 2004. “A Guide for A.01nalyzing Data from Traffic Stops.” Washington, DC. Police Executive Research Forum. https://cops.usdoj.gov/html/cd_rom/mayors72nd/pubs/ExecutiveSummaryBytheNumber.pdf McClean, K. and J. Rojek. 2016. “Traffic Stops, Race, and Measurement.” In B. Huebner and T. Bynum (eds), The Handbook of Measurement Issues in Criminology and Criminal Justice, pp. 452-472. Wiley and Blackwell. Missouri Attorney General. Vehicle Stops Report, 2000-2016. https://ago.mo.gov/home/vehicle-stopsreport. Persico, N. and P. Todd. 2008. “The Hit Rates Test for Racial Bias in Motor Vehicle Searches.” Justice Quarterly 25: 37-53. Pierson, E., C. Simiou, J. Overgoor, S. Corbett-Davies, V. Ramachandran, C. Phillips, and S. Goel. 2017. “A Large-scale Analysis of Racial Disparities in Police Stops Across the United States.” Stanford University. Seguino, S., N. Brooks, and K. Mitofsky. 2012. “Racial Disparities in Policing? An Assessment of 2009-10 Traffic Stop Data in Chittenden County, Vermont.” University of Vermont and Cornell University. Seguino, S. and N. Brooks. 2017. “Driving While Black and Brown in Vermont.” University of Vermont and Cornell University. https://www.uvm.edu/giee/pdfs/SeguinoBrooks_PoliceRace_2017.pdf 29