Arrest-Related Deaths Program Assessment: Technical Report BJS 2015
Download original document:
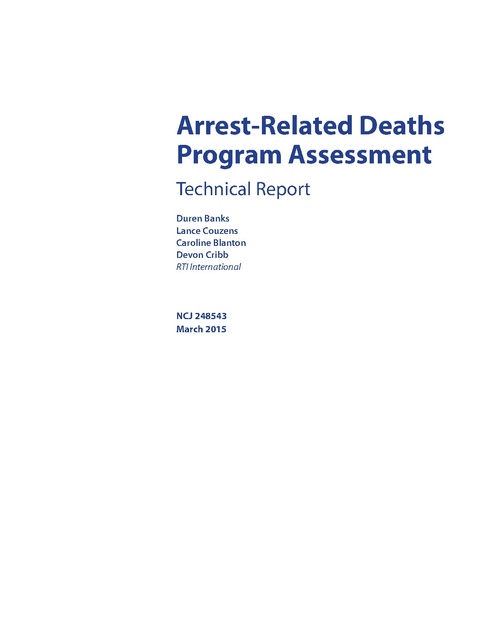
Document text
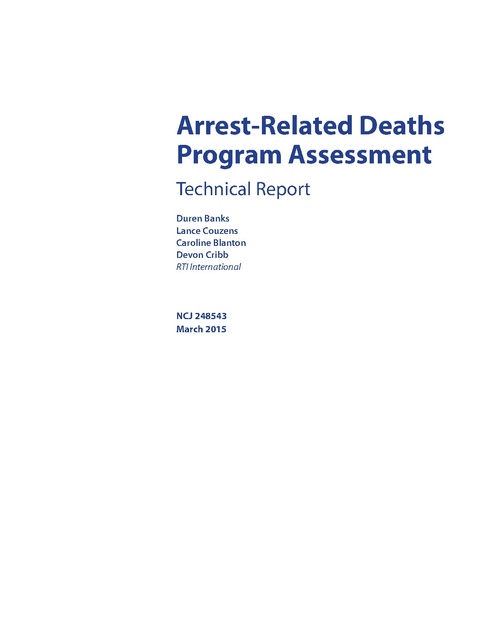
Document text
This text is machine-read, and may contain errors. Check the original document to verify accuracy.
Arrest-Related Deaths Program Assessment Technical Report Duren Banks Lance Couzens Caroline Blanton Devon Cribb RTI International NCJ 248543 March 2015 Contents Executive summary .....................................................................................................................1 1 Background ..............................................................................................................................3 2 Methodology ............................................................................................................................4 2.1 Probabilistic case-level linking ......................................................................................4 2.1.1 Calculation of similarity scores .........................................................................5 2.1.2 Thresholds for matching ....................................................................................6 2.1.3 Linking algorithm ..............................................................................................6 2.1.4 Monte carlo replication ......................................................................................8 2.2 Capture-recapture analytic approach .............................................................................8 2.2.1 Simple poststratified Lincoln-Petersen approach ..............................................9 2.2.1.1 Assumptions........................................................................................9 2.2.1.2 Strengths and limitations...................................................................10 2.2.2 Poststratified Lincoln-Petersen approach with constant ratio adjustment for unobserved agencies .............................................................10 2.2.2.1 Assumptions......................................................................................11 2.2.2.2 Strengths and limitations...................................................................11 2.2.3 Data assumptions and coverage estimate implications ....................................12 3 Findings ..................................................................................................................................13 3.1 Arrest-related death coverage ......................................................................................13 3.2 Variability in ARD methodology across states ............................................................23 3.2.1 ARD law enforcement homicide reporting methodology................................27 3.2.2 Location of SRC ..............................................................................................29 3.3 Number of years at least one death was reported to ARD and SHR ...........................31 4 Conclusions and recommendations ........................................................................................32 4.1 Relative strengths and weaknesses of the ARD program methodology ......................32 4.1.1 Types of cases that were covered well by the ARD program ..........................32 4.1.2 Identification approaches that are associated with better coverage .................32 4.2 Recommendations ........................................................................................................33 4.3 Study limitations ..........................................................................................................34 References .................................................................................................................................37 Appendix A: Similarity scoring for ARD/SHR matching.........................................................38 Introduction ........................................................................................................................38 iii Continuous variables ..........................................................................................................38 Categorical variables ..........................................................................................................39 Calculating the overall score ..............................................................................................40 Appendix B: Linking algorithm ................................................................................................41 Estimate the agency population size and agency undercoverage adjustment factor .........41 Classify cases as matchable or unmatchable .....................................................................41 Probabilistic linking between ARD and SHR ....................................................................42 Recombine cases ................................................................................................................43 Stratify by individual-level characteristics ........................................................................43 Estimate population size and variance by stratum .............................................................43 Estimate population size ....................................................................................................44 Adjust population size for agency undercoverage .............................................................44 Estimate ARD coverage .....................................................................................................44 Replication .........................................................................................................................44 Appendix C: Race/Hispanic origin coding ................................................................................46 Hispanic origin in the SHR ................................................................................................46 List of tables Table 1: ARD and SHR law enforcement homicides, by match outcome, 2003–09 and 2011 ................................................................................................................ 16 Table 2: ARD law enforcement homicides, by match outcome, 2003–09 and 2011 .............. 16 Table 3: ARD and SHR law enforcement homicides, by matching variable and match outcome, 2003–09 and 2011 ................................................................................. 19 Table 4: ARD law enforcement homicides, by matching variable and match outcome, 2003–09 and 2011 ................................................................................................. 20 Table 5: ARD law enforcement homicide decedent characteristics, by source, 2003– 09 and 2011 ........................................................................................................... 22 Table 6: ARD coverage estimates and confidence intervals, by estimation approach, 2003–09 and 2011 ................................................................................................. 23 Table 7: ARD law enforcement homicides, by region, state, and source, 2011 ..................... 25 Table 7: Primary methodology for identifying arrest-related deaths, by state, 2011 .............. 27 Table 8: SRC agency location, 2011 ....................................................................................... 29 Table 9: Number of years at least one death was reported to ARD and SHR......................... 31 iv List of figures Figure 1: ARD and SHR coverage and overlap of the universe of law enforcement homicides in the United States, with no agency adjustment, 2003–09 and 2011....................................................................................................................... 14 Figure 2: Estimated proportion of law enforcement homicide universe covered by each source, with no agency adjustment, 2003–09 and 2011 ............................... 15 Figure 3: Percent of observed ARD law enforcement homicides, by match score, 2003–09 and 2011 ................................................................................................. 17 Figure 4: Percent of law enforcement homicide universe covered by ARD, by estimation method, 2003–09 and 2011 ................................................................. 24 Figure 5: Law enforcement homicides, by source and primary reporting method, 2011....................................................................................................................... 28 Figure 6: Law enforcement homicides, by source and SRC location, 2011 ........................... 30 Figure 7: ACS 5-year Hispanic origin, by county ................................................................... 48 v Executive summary The Bureau of Justice Statistics (BJS) designed the Arrest-Related Deaths (ARD) program to be a census of all deaths that occur during the process of arrest in the United States. The manner in which these data were collected varied from state to state, and often depended on the data systems available to the state reporting coordinators (SRCs) responsible for data collection throughout the state, the involvement of local law enforcement agencies or medical examiner’s/coroner’s offices, and other support that the SRC may have had to conduct the data collection. This variability in approach has led to questions about whether these data collection methods were capable of capturing the universe of arrest-related deaths and law enforcement homicides in particular. BJS requested RTI International to conduct an assessment of the ARD program to evaluate (1) the coverage of the program in comparison to Supplementary Homicide Reports (SHRs) maintained by the Federal Bureau of Investigation (FBI) and (2) various aspects of the current program methodology. The coverage assessment matched law enforcement homicides captured by the ARD program to those found in the SHR justifiable homicide file, followed by a capture-recapture analysis to provide information on the scope and characteristics of cases eligible for inclusion in the ARD program that are captured in one or both of these data systems. The ARD law enforcement homicides and SHR justifiable homicide files are similar; however, some law enforcement homicides that were not classified as justifiable are not identified in the SHR. RTI calculated the size of the law enforcement homicide population in the United States and the ARD program coverage using two methods to estimate the lower and upper bounds of ARD coverage. We found that over the study period from 2003 through 2009 and 2011, the ARD program captured, at best, 49% of all law enforcement homicides in the United States. The lower bound of ARD program coverage was estimated to be 36%. These findings indicate that the current ARD program methodology does not allow a census of all law enforcement homicides in the United States. The ARD program captured approximately 49% of law enforcement homicides, while the SHR captured 46%. An estimated 28% of the law enforcement homicides in the United States are not captured by either system. However, the methodology for identifying ARD cases has changed over the observation period. In 2011, the ARD program was estimated to cover between 59% and 69% of all law enforcement homicides in the United States, depending on the estimation method used. While this coverage estimate still does not result in a census, it does suggest improvements over time in the overall approach to identifying law enforcement homicides and reporting them to the ARD program. We found considerable variability between states in the proportion of law enforcement homicides that are reported to the ARD program only, the SHR only, or to both sources in 2011. Twelve states reported only to the ARD program in 2011, while no states reported cases only to the SHR. Additional analyses to explore the effect of case identification methodology and SRC affiliation failed to identify a specific ARD methodology that was associated with better program coverage in 2011. Page 1 The current analyses only compared ARD program coverage to the SHR. Other sources may also provide additional information about the extent of law enforcement homicides in the United States or in selected jurisdictions, and coverage of the various data collection systems. These sources include the Fatal Injury Reports that are part of the National Vital Statistical System maintained by the Centers for Disease Control and Prevention and direct reports from local law enforcement agencies. In addition, the ARD program assessment examined only arrested-related deaths that are the result of law enforcement homicides. Arrest-related deaths due to illness, overdose, accidents, and other manners of death are likely even more difficult to identify and, if included, could have a significant downward impact on our coverage estimates. However, no other national data collection exists that examines arrest-related deaths due to a manner other than law enforcement homicide. If BJS pursues a collection to measure law enforcement homicides or all manners of arrest-related deaths in the United States, changes must be made to the data collection methodology to support more complete coverage. Page 2 1 Background By definition, the Arrest-Related Deaths (ARD) program was to be a national census of law enforcement homicides that occurred during the process of arrest or during an attempt to obtain custody by a state or local law enforcement agency in the United States. The program was implemented by the Bureau of Justice Statistics (BJS) in 2003 as part of its Deaths in Custody Reporting Program (DCRP) in response to the Deaths in Custody Reporting Act of 2000 (DICRA). In addition to the ARD program, the DCRP includes collections that measure deaths occurring in jails and state prisons. The ARD program methodology relied on state reporting coordinators (SRCs) in each of the 50 states and the District of Columbia. SRCs were responsible for understanding the scope and definition of the ARD program, identifying eligible cases in their state, and working within available resources to collect and report information about those cases. The manner in which these data were collected varied from state to state, and often depended on the data systems available to the SRC, the involvement of local law enforcement agencies or medical examiner’s/coroner’s offices, and other support that the SRC may have had to conduct the data collection. This variability in approach led to questions about whether the existing data collection methods were capable of capturing the universe of arrest-related deaths and law enforcement homicides in particular. The Supplementary Homicide Reports (SHRs) represent another national-level data source that provides information on law enforcement justifiable homicides in the United States. 1 The SHR is part of the Uniform Crime Reporting (UCR) Program conducted by the Federal Bureau of Investigation (FBI). While the UCR summary program provides aggregate annual counts of the number of homicides occurring in the United States, the SHR data provide additional details about each homicide incident, including the jurisdiction, month, year, victim and offender demographic characteristics, weapon, the circumstances surrounding the incident (e.g., argument, robbery, gangrelated), and the relationship between the victim and offender, if known. To determine whether the ARD program met the criteria for a valid and reliable statistical collection, BJS tasked RTI to undertake a program assessment to better understand the utility of the ARD data collection approach and to effectively assess alternative approaches. RTI conducted the ARD program assessment to evaluate (1) the homicide coverage of the program in comparison to the Supplementary Homicide Reports (SHRs) and (2) the current program methodology. The coverage assessment matched ARD cases to the SHR. A capture-recapture analysis provided information on the scope and characteristics of cases eligible for inclusion in the ARD program that are captured in one or more of these data systems. The program methodology assessment further compared ARD cases identified by various approaches currently employed by the state reporting coordinators (SRCs) and the ARD staff, including media searches, access to state reporting systems, and direct reporting from law enforcement agencies. The program assessment process and findings are documented below. 1 The SHR homicide also includes a small number of law enforcement-related homicides that are not classified as justifiable, but those cases cannot be identified. Page 3 2 Methodology The Arrest-Related Deaths (ARD) program relied on a census collection from agencies that experienced one or more deaths to estimate the number of arrest-related deaths in a particular calendar year. Because the census was (1) obtained through self-reporting by agencies, as opposed to targeted collection of a probability sample derived from a robust agency frame, and (2) included no agencies that did not experience a death, it is difficult to make inferences regarding any undercoverage using information from the ARD program alone. In light of these constraints, a coverage assessment that utilizes comparable data from an auxiliary source is appropriate. The U.S. Department of Justice, through the Federal Bureau of Investigation (FBI), releases annual law enforcement-reported homicide data in the form of Supplementary Homicide Reports (SHRs). Because the SHR data provide incident-level information on homicides, they can be used to help assess the coverage of the ARD data collection. Whereas the ARD collection documents all manners of death that occur during the arrest process or in the presence of law enforcement, the SHR is limited to homicides and therefore does not include suicide, accidents, illness or natural causes, drug intoxications, and other manners of death not considered to be homicides. Therefore, to make comparisons between these data collections, each must be restricted to a similar population of cases. For the analyses presented below, we restricted the SHR to only the subset of homicides in which an offender is killed by the police (justifiable homicides) and subset the ARD to those incidents defined as homicides committed by law enforcement. Both of these subsets are limited to incidents involving state or local law enforcement agencies. The SHR and ARD data sets include all law enforcement homicides reported in the years that the ARD program produced annual datasets: 2003 through 2009 and 2011. Some states did not report any SHR data in some years. With the exception of Florida, which did not report any SHR data for the entire period, no additional information was added to the FBI’s SHR file for other states. Homicide cases for Florida were collected independently from the FBI collection and added to the SHR file for this analysis. By linking ARD decedent records to those captured in the SHR, it is possible to make inferences about the total size of the law-enforcement homicide population and, thereby, about the population coverage achieved in the ARD program. This analytic technique, known as capturerecapture, is typified by studies of wild animal populations, though it is widely used in the study of human populations as well, particularly in coverage evaluation for censuses (Wolter, 1986). Though the ARD coverage assessment is based on traditional capture-recapture methods, unique characteristics of ARD data necessitate special techniques, including probabilistic record linkage between data sources and adjustments for unobserved agencies. 2.1 Probabilistic case-level linking Capture-recapture analysis depends on the ability to link records across data sources. In wildlife applications, this requirement is easily met as captured animals are identified through tagging. In the context of a coverage assessment utilizing an auxiliary data source not collected as part of the coverage study, this requirement becomes more difficult. Because decedents are not uniquely identified across data sources (by name or Social Security number, for example), record linkage must be based on comparable decedent demographic and incident characteristics in an attempt to Page 4 create a unique link. However, linkages made without unique identifiers are inherently uncertain, decedents are matched between ARD and SHR sources probabilistically based on measured similarity between records, within a monte carlo replication framework. This means that although a given ARD decedent will be linked to either no SHR decedents or a single SHR decedent in a particular replicate, the nature of the link for that ARD decedent (whether it is linked and, if so, to which SHR decedent) is allowed to vary across replicates according to the probabilities associated with particular record pairings, based on measured similarity between cases. Probabilistic linking is random in nature and incorporates additional uncertainty into the process of estimating population coverage; monte carlo replication allows for this additional uncertainty to be captured in variance estimates. 2.1.1 Calculation of similarity scores Records cannot be uniquely identified across sources so linking decedent records is done probabilistically, based on the measured similarity between two records. The first step in determining link probabilities for a given ARD decedent and the decedent’s potential matches in the SHR is to calculate a similarity score. Similarity is based on the following variables that are common to both ARD and SHR: Originating Agency Identifier (ORI) Sex Year Date reported/date of death Age Race/Hispanic origin Decedent records are considered 0% similar if they do not match on at least ORI, sex, and year and are not eligible for linking. For records that have common values for these three variables across sources, scores are calculated based on the values of the date, age, and race/ Hispanic origin variables. These measures include a mix of continuous and categorical variables; therefore, the method for calculating the similarity score must account for both types. The portion of the similarity score determined by the continuous variables (date and age) is calculated as a function of the standard Euclidean distance for two variables, such that two records having identical values across sources have a similarity of 1. The portion of the similarity score determined by the continuous variables approaches 0 as dates and ages become more dissimilar. The portion of the similarity score determined by the race/Hispanic origin values is based on a penalized scoring approach described in Boriah et al. (2008). This approach assigns a similarity of 0 if the variables disagree across sources and a value of less than 1 when the variables agree. The amount that matching records’ similarity score is discounted is determined by the probability that the race/Hispanic origin value would be in agreement by random chance alone. This approach is based on the premise that matches that have a high probability of occurring by random chance should not count as much as those that would be observed very rarely by chance Page 5 alone. The final similarity score is calculated as a weighted mean of the components based on continuous variables (weight = 2/3) and race/Hispanic origin (weight = 1/3). Once similarity scores are calculated for each potential pairing of a given ARD decedent and SHR decedents from the same ORI, sex, and year, these values are used to create an additional score. This score represents the combined dissimilarity between the ARD case and all of its potential matches. Following calculation of this dissimilarity score, all scores are scaled such that their sum is 1. These scaled scores are treated as relative probabilities and determine how cases are linked. A detailed description of similarity score calculation, including all formulas, may be found in appendix A. 2.1.2 Thresholds for matching Potential record pairings fall into one of two categories: those that are within a predetermined threshold of similarity and those that are not. If two records each have the same value of ORI, sex, year, and race/Hispanic origin, there is a difference in the dates of report and death less than or equal to 30 days, and there is a difference in recorded age of less than or equal to 5 years 2, the pairing is thought to be within an acceptable threshold to consider the records as representing the same decedent. In these instances, the scaled similarity scores (match probabilities) for all pairings for the same ARD decedent that are not within threshold are set to 0. This includes the scaled dissimilarity score, which represents the joint probability across potential pairings that no SHR record represents the same decedent. This means that when an ARD decedent has one or more pairings that are within threshold, the probability that the record will not be linked at all is 0. In some cases, a single ARD case may have more than one pairing that is within threshold. In these instances, a link will take place according to probabilities resulting from rescaling the similarity scores among only the within-threshold pairings such that they sum to 1. ARD decedent records that have a single potential pairing that is within threshold undergo the same rescaling process, resulting in a single potential pairing with a link probability of 1; these pairings are thereby referred to as certainty pairings. For ARD decedent records with no potential within-threshold pairings, linking occurs according to the probabilities associated with all potential pairings and the calculated probability that no pairing is correct. Thus, these decedent records may remain unlinked, and in the event that all potential pairings are very dissimilar, this will be the most probable outcome. 2.1.3 Linking algorithm The first step in the linking process is separating ARD and SHR decedent records into two groups: those that have ORI, sex, and year values common across data sources and those that do not. The latter group is considered unlinkable; those records with common values of ORI, sex, and year are linked probabilistically according to the following steps (a more technical description is provided in appendix B): 2 Age values in the SHR are considered imprecise and have been observed to clump around 5-year marks. Additionally, the SHR date is the month and year the homicide was reported. These factors necessitate a certain level of leeway in the criteria for linking and are what drive the linking threshold definitions. Page 6 1. Randomly sort the ARD cases within ORI, sex, and year. The first step in linking ARD decedent records to the SHR is a random sort. Because the ARD decedents are processed sequentially and a linked SHR decedent is unavailable for linking to other ARD records processed after the link is made, it is important to ensure that that the order in which the ARD cases are processed is not fixed. This is necessary for monte carlo replication as described in 2.1.4. 2. For each ARD case, calculate a similarity score corresponding to each SHR case with the same ORI, sex, and year. In this step, similarity scores are calculated as described in 2.1.1, above. Note that for any ARD decedent record that is not the first to be processed in its ORI, sex, and year classification, there may be SHR decedents ineligible for linking, as they are linked to other ARD records processed previously. The similarity scores for such ineligible pairs are set to 0. 3. Treating the scores from (2) above as probabilities, calculate the dissimilarity score (the probability that the ARD case is not represented by any SHR decedent in the same ORI, sex, and year). Given the small number of variables that overlap between the ARD and SHR and the inability to perfectly match across sources, it is important to assign a nonzero probability to the possibility that the ARD decedent is not represented by any of the SHR decedent records in the same ORI, sex, and year. 4. Determine the number of cases that are within threshold. Each potential pairing for a given ARD decedent is checked against the threshold criteria described in 2.1.2. If one or more pairings are within threshold, all other scores (including the one described in (3), above) are set to 0. 5. Scale scores. Though the scores described above may be individually interpreted as probabilities, their sum may be greater than 1, complicating both their joint interpretation and the process of linking. In this step, each of the scores is divided by their sum, maintaining their relative proportions. Note that as a result of (2) and (4) above, several outcomes are possible: i. No potential SHR decedent records have been previously linked to other ARD records, and there are no within-threshold potential pairings—in this case there will be a nonzero probability that no linkage will be made. ii. No potential SHR decedent records have been previously linked to other ARD records and there is one potential within-threshold pairing—in this case the within-threshold pairing is a certainty. iii. No potential SHR decedent records have been previously linked to other ARD records and there are multiple potential within-threshold pairings—in this case, a linkage will be made only according to the probabilities among within-threshold pairings, as all other probabilities have been set to 0 prior to scaling. iv. One or more potential SHR decedent records have been previously linked to other ARD records and there are one or more potential SHR records available for linking —these cases are then treated identically to i. through iii., above. v. One or more potential SHR decedent records have been previously linked to other ARD records and there are no potential SHR records remaining for linking—in Page 7 this case, the probability that the ARD decedent record is not represented in the SHR is 1 and the record is not linked. 2.1.4 Monte carlo replication The inability to precisely match decedents between the ARD and the SHR necessitates a probabilistic linking approach, but this technique is inherently random in nature, achieving the most probable outcomes only in expectation. By repeating the linking algorithm many times, the variability resulting from that randomness can be incorporated into final estimates. The monte carlo framework leverages two points at which randomness enters the linking process: the use of random numbers for sorting ARD decedent records and the use of random numbers to select from potential pairings. This means that uncertainty in record linking resulting from the lack of overlapping variables, and/or errors in variables that do overlap, will be incorporated in final estimates. Generation of random number streams depends on selecting ‘seed’ values—numbers that are fed into the algorithm as a starting point for generating the stream. By setting random number seeds in a predictable way (for repeating the results) that is a function of both the replicate number and the ORI, sex, and year grouping, variation inherent to the probabilistic linking can be correctly accounted for. Given the resource-intensive nature of probabilistic linking in general, a replicate count of 1,000 was selected to balance resource usage and precision. 2.2 Capture-recapture analytic approach Once decedent records have been linked across the ARD and SHR, capture-recapture methods can be used to estimate the total population of law enforcement homicide and, thereby, the proportion of that population covered by the ARD program. This analysis makes use of the commonly applied Lincoln-Petersen (LP) estimator, with modifications for bias amelioration necessitated by the characteristics of the ARD program. Use of the basic LP estimator implies acceptance of several assumptions, including the following: a. The population of interest is closed geographically and in time and is of fixed size; b. Correct matches may be made between sources; c. Each data source is free of spurious cases, such as those that are out of scope or that are duplicates; d. The probability of inclusion is independent across lists; e. The probability of inclusion is constant within lists; and f. The probability of inclusion is constant across lists. For the purposes of evaluating ARD coverage, some of the above assumptions may be accepted as true, while others must be accepted with qualification and, in some cases, in conjunction with specific analytic techniques designed to minimize the biases incurred as a result of their likely violation. Given the goal of the analysis—to determine if ARD coverage is high enough to Page 8 justify continuing the program in its current form—violation of some assumptions is not as problematic as would be the case in other applications because the direction of incurred bias is known. This allows for reasonable assertions of upper limits for ARD coverage, which provides sufficient information given the circumstances. In addition to the assumptions presented as a.–f. listed above, this analysis must account for an additional, and seemingly unique, challenge: estimating the impact that unobserved law enforcement agencies with law enforcement homicides have on decedent population coverage. Because agencies with law enforcement homicides report inconsistently to the ARD and SHR, proper evaluation of coverage must account for whether or not agencies that are not observed in either source experienced law enforcement homicides, and, if so, how many. Sections 2.2.1 and 2.2.2, below, address two methods of conducting the capture-recapture analysis, including assumptions made and the benefits of each technique. A detailed, technical description of the techniques employed is provided in appendix B. 2.2.1 Simple poststratified Lincoln-Petersen approach This approach is the simpler of the two described here, as it does not incorporate any correction for unobserved agencies. In other words, under this approach there is an implied assumption that any agency not observed in either the ARD or the SHR has not experienced a law enforcement homicide, or, put another way, this method assumes that every agency with homicides is observed in at least one source. Though this assumption is strong (and very likely violated), the results of this method are still quite useful given the context in which they’re being used. Specifically, assuming that all unobserved agencies are so-called ‘true zeroes’ (they have not experienced any cases of law enforcement homicide), results in a coverage estimate that represents the best-case scenario. Thus, the simple, poststratified LP estimate may be interpreted as an upper bound for ARD coverage, with the true coverage rate falling somewhere below. From a decision-making perspective, knowing the best-case estimate may be sufficient, especially if the estimated coverage is considered unacceptable. 2.2.1.1 Assumptions Assumptions a. and c. from 2.2 are accepted as valid in this approach. With both sources limited to cases from 2003–09 and 2011, and all arising from U.S. agencies, assumption a. is reasonable. Because each source has been subset to the common subpopulation of justifiable law enforcement homicides, and BJS has verified that no duplicates are included in the files provided, assumption c. is also reasonable. 3 As stated previously, given the inability to uniquely identify decedents across data sources, assumption b. cannot be reasonably made. Conducting the analyses within a monte carlo 3 As part of the data quality checks, the ARD files were sorted several ways (i.e., state, year, name, date of death, and date of birth) to identify duplicate records. This method was successful in identifying duplicate entries even when the decedent had different names listed (e.g., first and last name inverted or middle name used as last name). In addition, every ARD case was cross-referenced with the DCRP Jails file to identify duplicates across DCRP collections. BJS used a similar method with the SHR file and removed any duplicates identified. Page 9 replication framework allows for incorporation into final estimates of error incurred through violation of this assumption, and for this reason, no single replicate can be evaluated for linking correctness. Individual linkages may be made in a single replicate with low probability, though across replicates they will occur rarely. Taken as a whole, across all replicates, average linking outcomes represent the best matches given the available agency and individual information. Assumption d., commonly referenced in the context of behavioral response in capture-recapture literature, cannot be validated and potential biases incurred through violation cannot be mitigated or quantified given the information available. It is worth noting, however, that the nature of this bias may not be particularly important for this analysis. In the context of this study, a behavioral response would mean that those responsible for collecting and reporting data to the ARD and SHR programs would be more or less likely to report to one program if they had already reported to the other. Because (1) it is reasonable to assume that such an effect could work in both directions (i.e., a record may be more or less likely to be captured in one source if it has been previously captured in the other) and (2) there is no reason to believe that one source consistently captures decedent records before the other, any bias resulting from behavioral responses is assumed to be diffused and to contribute little, if any, directional bias to final coverage estimates. Homogeneity of capture probabilities implied by assumptions e. and f. cannot be guaranteed within or across data sources, so the LP estimator of population size may contain downward bias, leading to an overestimation of coverage (Wolter, 1986). The common method for dealing with this bias—and the one employed in this analysis—is poststratification. Once decedent records are linked, they are grouped into strata corresponding to the cross-classification of available demographic characteristics. The simple LP estimator is then used within strata and the results are aggregated over the complete population. This approach is based on the idea that, once grouped, capture probabilities become homogeneous within strata. Poststratification on these variables may not account for all bias because the number of reliable characteristics with enough samples per category common to both sources is low (race/Hispanic origin and age only). Again, in the context of this analysis, remaining bias may have little to no effect on the analytic utility of the results, as the direction of the bias (if not its magnitude) is known. Heterogeneity in capture probabilities yield population size estimates that are deflated and coverage rates that are artificially high. Thus, final coverage estimates can be interpreted as the upper bounds on the actual coverage rate. 2.2.1.2 Strengths and limitations This simple LP estimator method has the notable benefit of being the simpler of the two methods implemented, as it does not require estimation of the number of unobserved agencies with law enforcement homicides. Additionally, this method yields an estimate that represents the best-case scenario for ARD coverage. From the perspective of a user wanting to know the most accurate coverage estimate, rather than its upper boundary, this method is not ideal. 2.2.2 Poststratified Lincoln-Petersen approach with constant ratio adjustment for unobserved agencies Unlike the approach described in 2.2.1, the poststratified LP method rejects the assumption that any agency not observed in either the ARD or the SHR has not experienced a law enforcement Page 10 homicide. It therefore must account for two additional unknown factors: the number of unobserved agencies with law enforcement homicides and the number of homicides experienced among those agencies. Under this approach, the simple LP estimator is used to estimate the total number of agencies that experienced law enforcement homicides in the reference period. By treating the ratio of this estimate over the number of agencies actually observed across both sources as an agency undercoverage adjustment factor, decedent population estimates corresponding to each poststratum (as described in 2.2.1) may be extrapolated to represent the decedent population among unobserved agencies. Because the decedent population is inflated by a constant factor, this approach implies a strong assumption that missing agencies with homicides are missing at random (i.e., the distribution of missing agencies in terms of decedent counts is identical to that of the observed agencies). 4 Notably, agencies that are captured in both the ARD and SHR have, on average, more homicides than those observed in only one source (5.2 vs. 1.5), and it may be reasonable to infer that unobserved agencies are more like the latter group. In other words, assuming that missing agencies are just like those that have been observed likely results in an inflationary bias in the decedent population size estimate and, therefore, a deflationary bias in the corresponding ARD coverage estimate. This implies that the coverage estimate derived using a constant ratio adjustment for unobserved agencies represents a worst-case scenario, or lower bound, for ARD coverage. Presenting the coverage estimates derived using this method along with the one described in 2.2.1 yields a bounded range representing the most extreme assumptions regarding the nature of unobserved agencies. Given the requirements of the analysis, this is likely sufficient and benefits from making as few assumptions as possible. 2.2.2.1 Assumptions Beyond the assumptions inherent to the agency undercoverage adjustment factor noted above, the assumptions for this model are identical to those described in 2.2.1.1 and are not repeated here. 2.2.2.2 Strengths and limitations The postratified LP methodology provides additional benefit over that described in 2.2.1 in that it rejects the (likely false) assumption that all unobserved agencies are so-called true zeroes. The notable downsides of this approach are the necessity of making additional assumptions regarding the nature of unobserved agencies—each additional assumption introduces new sources of potential error. Additionally, as this method treats unobserved agencies as being like those that are observed, in terms of the volume of law enforcement homicides, it potentially yields a deflated coverage estimate. 4 Use of a simple, unstratified LP estimator likely results in deflationary bias in the law enforcement homicide agency population estimate; its use is necessitated by resource constraints that precluded collection and inclusion of agency-level characteristics for poststratification. Page 11 2.2.3 Data assumptions and coverage estimate implications The sections discussed above deal with theoretical assumptions underpinning the LP estimator. As noted, these assumptions have been addressed where possible and, in all cases, their potential effects on coverage estimates have been discussed. Given the context in which these estimates are being used and the careful statements regarding their interpretation, the analysis is robust to violations of these assumptions. Fundamental assumptions about the data, however, are largely unverifiable. For certain data items, specifically date and race/Hispanic origin variables that are used in linking and that are measured differently across sources, comparability is maximized through recoding (as noted in appendixes A and C) and the impact of remaining inconsistencies can be inferred based on the amount of variability in final estimates across replicates, as the linking procedure results in cases being linked probabilistically even when dates and race/Hispanic origin codings are not in agreement across data sources. Because the range of final ARD coverage estimates across replicates is less than 2 percentage points, it is reasonable to infer that inconsistencies in these variables across sources do not have a large impact. Furthermore, the small range of replicate estimates indicates that final estimates are not being driven by the probabilistic linking. Across replicates, 68% of decedent records remain unmatched after linking. Of these unmatched records, 53% come from ARD and an overwhelming 82% of those are not even eligible to enter the probabilistic linking procedure because they have no match in the SHR on ORI, year, and sex. Given the relative rarity of female law enforcement homicides and the low estimated probability of decedent records appearing in the incorrect year, ORI can be viewed as the linking variable with by far the largest impact on final estimates. Data quality issues relating to ORI codes may therefore affect estimated coverage estimates, though, unfortunately, errors resulting from problems with ORI codes are of an unknown magnitude and direction. Page 12 3 Findings 3.1 Arrest-related death coverage As requested by BJS, RTI estimated the size of the law enforcement homicide population in the United States and the Arrest-Related Death (ARD) program coverage using two methods. The simple, poststratified Lincoln-Petersen method assumes that all nonreporting law enforcement agencies did not have any law enforcement homicides during the observation period. This approach provides the highest possible ARD coverage estimate and the lowest estimate of the total population size (also referred to as the “right bookend” approach). Following this approach, the ARD coverage of all law enforcement homicides is estimated to be 48.73%, with a 95% confidence interval ranging from 47.28% to 50.18%. In other words, the ARD program captured about half of all law enforcement homicides in the United States during the observation period. The second coverage estimate assumes that all nonreporting agencies have the same number of law enforcement homicides as agencies that did report to either the Supplementary Homicide Reports (SHRs) or the ARD program. The poststratified Lincoln-Petersen approach with a constant ratio adjustment for unobserved agencies provides the “left bookend” or lower estimate of ARD coverage. Under this approach, the ARD program is estimated to capture 36.43% of all law enforcement homicides in the United States, with a 95% confidence interval ranging from 34.50% to 38.35%. Figure 1 is a Venn diagram of the ARD and SHR coverage following the simple, poststratified Lincoln-Petersen method (the first coverage estimate described above), which assumes that all nonreporting law enforcement agencies did not have any law enforcement homicides during the observation period. Under these assumptions, the universe of law enforcement homicides in the United States across all years examined (2003–09 and 2011) is 7,427, or an average of 928 law enforcement homicides per year. The ARD program captures approximately 49% of these homicides, while the SHR captures 46%. About 28% of the law enforcement homicides in the United States are not captured by either system (see figure 2). Page 13 Figure 1: ARD and SHR coverage and overlap of the universe of law enforcement homicides in the United States, with no agency adjustment, 2003–09 and 2011 Page 14 Figure 2: Estimated proportion of law enforcement homicide universe covered by each source, with no agency adjustment, 2003–09 and 2011 Unobserved 28% SHR only 23% ARD only 26% ARD and SHR 23% RTI implemented a monte carlo replication approach to match SHR and ARD cases (see table 1). There were an estimated 5,324 law enforcement homicides reported to either system in the observed years. More than two-thirds of those homicides were not able to be matched across data sources. The level of unmatched cases was greatest in 2005 (75.6%) and lowest in 2011 (60.4%). Certainty matches across sources are created when cases match on the originating agency identifier (ORI) number, year, and sex, within 1 month of date of death (or date of report for SHR) and within 5 years for age. Across all observed years, approximately 21% of all law enforcement homicides reported to either source were certainty matches, ranging from 15% in 2008 to 26% in 2009 and 2011. These certainty matches represent 94.5% of ARD cases with one or more SHR pairings satisfying the certainty criteria noted above (not shown). The remaining 5.5% have more than one match that satisfies the certainty criteria (4.9% have two matches, and the remaining 0.6% have between three and six matches). Page 15 Table 1: ARD and SHR law enforcement homicides, by match outcome, 2003–09 and 2011 Probability match score No match ARD program data collection year All LE homicides AVG N 0.01 – 0.25 % AVG N 0.26 – 0.50 % AVG N % 0.51 – 0.75 AVG N % 0.76 – 0.99 AVG N % Certainty match Total AVG N % AVG N % 3,643.9 68.4 221.0 4.2 190.1 3.6 150.8 2.8 6.8 0.1 1,111.9 20.9 5,324.5 100 2003 424.6 71.3 23.6 4.0 19.3 3.2 15.2 2.5 0.1 0.0 112.6 18.9 595.3 100 2004 416.7 70.6 18.9 3.2 19.4 3.3 11.8 2.0 0.6 0.1 123.1 20.8 590.4 100 2005 456.7 75.6 19.8 3.3 16.8 2.8 14.8 2.5 1.8 0.3 94.4 15.6 604.3 100 2006 469.8 70.9 22.7 3.4 27.0 4.1 24.4 3.7 0.1 0.0 118.4 17.9 662.4 100 2007 450.1 66.2 25.2 3.7 23.2 3.4 21.1 3.1 1.5 0.2 158.9 23.4 680.0 100 2008 482.6 73.4 37.1 5.6 21.1 3.2 18.5 2.8 0.0 0.0 98.0 14.9 657.3 100 2009 443.4 62.7 30.1 4.3 27.7 3.9 17.2 2.4 1.2 0.2 187.1 26.5 706.7 100 2011 500.1 60.4 43.6 5.3 35.7 4.3 27.8 3.4 1.5 0.2 219.4 26.5 828.0 100 Note: The 'AVG N' represents the mean count across all replicates. Table 2: ARD law enforcement homicides, by match outcome, 2003–09 and 2011 Probability match score ARD program data collection year 0.01 – 0.25 No match 0.26 – 0.50 AVG N 1,939.5 53.6 221.0 6.1 190.1 5.3 150.8 4.2 6.8 0.2 2003 206.3 54.7 23.6 6.3 19.3 5.1 15.2 4.0 0.1 2004 201.4 53.7 18.9 5.0 19.4 5.2 11.8 3.1 2005 229.3 60.8 19.8 5.3 16.8 4.5 14.8 3.9 2006 254.4 56.9 22.7 5.1 27.0 6.0 24.4 2007 225.0 49.5 25.2 5.5 23.2 5.1 All LE homicides % % AVG N 0.76 – 0.99 % AVG N AVG N 0.51 – 0.75 % AVG N % Certainty match AVG N Total % AVG N % 1,111.9 30.7 3,620.0 100 0.0 112.6 29.9 377.0 100 0.6 0.2 123.1 32.8 375.0 100 1.8 0.5 94.4 25.0 377.0 100 5.5 0.1 0.0 118.4 26.5 447.0 100 21.1 4.6 1.5 0.3 158.9 34.9 455.0 100 2008 229.3 56.8 37.1 9.2 21.1 5.2 18.5 4.6 0.0 0.0 98.0 24.3 404.0 100 2009 232.7 46.9 30.1 6.1 27.7 5.6 17.2 3.5 1.2 0.2 187.1 37.7 496.0 100 2011 361.0 52.4 43.6 6.3 35.7 5.2 27.8 4.0 1.5 0.2 219.4 31.8 689.0 100 Note: The 'AVG N' represents the mean count across all replicates. Page 16 There were 3,620 cases reported to ARD in the years observed (see table 2). Across all years, 54% of those were not able to be matched to an SHR case, and 31% were a certainty match. Probability matches included 11.4% of ARD cases with a low probable match (probability score of 0.50 or less), and 4.4% with a probability match score between 0.51 and 0.99. Among all matched ARD cases, 47.6% were linked to a single SHR case across replicates (not shown), and 74.7% were matched to five or fewer distinct SHR cases. The maximum number of distinct SHR pairings for a single ARD case across replicates was 24. Across years, the lowest overlap with the SHR occurred in 2005 when 61% of all ARD cases were not able to be matched to an SHR case. In 2009, however, 38% of ARD cases were a certainty match to SHR, 15% were a probable match, and 47% were a no match. Figure 3 further shows the distribution of match scores for cases reported to ARD across all years observed. Figure 3: Percent of observed ARD law enforcement homicides, by match score, 2003–09 and 2011 No match 0.01 - 0.25 0.26 - 0.50 0.51 - 0.75 0.76 - 0.99 Certainty match 100.0 90.0 80.0 70.0 30.7 29.9 25.0 24.3 26.5 32.8 34.9 37.7 31.8 60.0 50.0 40.0 30.0 20.0 10.0 0.0 53.6 54.7 53.7 60.8 56.9 49.5 56.8 46.9 52.4 All years 2003 2004 2005 2006 2007 2008 2009 2011 Table 3 describes characteristics of law enforcement homicides reported to either the SHR or the ARD program by match score. As expected, law enforcement homicide victims with an unknown age are more likely to have no match. Law enforcement homicide victims that are identified as white, non-Hispanic (75%) are most likely to have a no match compared to other race/Hispanic origin groups, while victims of an “other” race are most likely to have a certainty match (26%) compared to unknown or other unspecified race/Hispanic origin groups. Page 17 The characteristics of cases reported to the ARD program by probability match score are described in table 4. Cases with victims of any unknown age are more likely to have no match compared to any known age group. Similarly, cases with victims of an unknown race/Hispanic origin are more likely to have a low probability match score compared to any known race/Hispanic origin groups. ARD cases with a victim identified as Hispanic (28%) are least likely to result in a certainty match compared to other known race/Hispanic origin groups. As described in appendix C, the race/Hispanic origin variable is measured differently by each data source, and there is a high amount of missing data regarding Hispanic origin in the SHR. This measurement issue may account for some of the differences in probability match scores by race/Hispanic origin group. A subgroup analysis on states with larger proportions of Hispanic populations was conducted to provide further insight into whether the difference in probability match scores across race/Hispanic origin groups is meaningful. RTI calculated coverage estimates among five states that report Hispanic origin to SHR greater than 75% (Arizona, California, North Carolina, Pennsylvania, and Texas). Following the simple, poststratified Lincoln-Peterson approach (upper bound), the ARD coverage in these five states is 71.91% (95% confidence interval range from 69.47% to 74.34%). With agency adjustment (providing the lower bound), the estimated ARD coverage is 54.90% in these five states (95% confidence interval range from 50.52% to 59.29%). Given the wide range of these results, it is likely that they are confounded with other, unmeasured agency characteristics, such as agency size, reporting requirements, and the prevalence of law enforcement homicides. That is, the estimates with these five states were intended to act as sensitivity analyses for measuring the Hispanic origin of victims of arrest-related homicides; however, they suggest that other variables may be driving ARD program coverage estimates. Page 18 Table 3: ARD and SHR law enforcement homicides, by matching variable and match outcome, 2003–09 and 2011 Probability match score No match 0.01 – 0.25 AVG N % AVG N % 0.76 – 0.99 AVG N % Certainty match AVG N % Total % 3,643.9 68.4 221.0 4.2 190.1 3.6 150.8 2.8 6.8 0.1 1,111.9 20.9 5,324.5 100 Male 3,500.4 68.3 220.4 4.3 189.7 3.7 143.9 2.8 6.0 0.1 1,063.4 20.8 5,123.7 100 Female 140.6 71.1 0.6 0.3 0.4 0.2 6.9 3.5 0.8 0.4 48.5 24.5 197.8 100 17 or younger 115.4 70.0 10.7 6.5 5.4 3.3 2.8 1.7 0.0 0.0 30.5 18.5 164.9 100 18–24 850.9 67.8 52.4 4.2 52.4 4.2 39.9 3.2 1.1 0.1 258.5 20.6 1,255.2 100 25–34 1,087.1 67.4 69.4 4.3 64.7 4.0 46.0 2.9 0.9 0.1 344.1 21.3 1,612.0 100 35–44 786.3 68.0 41.6 3.6 35.5 3.1 37.3 3.2 2.2 0.2 252.7 21.9 1,155.6 100 45–54 509.5 70.5 30.0 4.2 21.1 2.9 14.6 2.0 0.8 0.1 147.1 20.3 723.3 100 55 or older 246.2 69.9 12.3 3.5 8.0 2.3 6.7 1.9 0.0 0.0 79.0 22.4 352.2 100 Unknown 48.5 79.1 4.5 7.4 3.0 5.0 3.5 5.7 1.7 2.8 0.0 0.0 61.3 100 White, nonHispanic 1,764.9 75.0 27.9 1.2 47.4 2.0 36.5 1.5 3.2 0.1 472.5 20.1 2,352.3 100 Black, nonHispanic 1,093.6 64.9 77.0 4.6 70.5 4.2 36.2 2.1 2.2 0.1 404.2 24.0 1,683.7 100 Hispanic, any race 581.7 59.2 89.1 9.1 56.1 5.7 54.9 5.6 1.4 0.1 199.7 20.3 982.7 100 All LE homicides % 0.51 – 0.75 AVG N Matching variable AVG N 0.26 – 0.50 AVG N % Sex Age Race/ Hispanic origin Other 86.0 63.2 4.8 3.5 4.9 3.6 4.9 3.6 0.0 0.0 35.5 26.1 136.2 100 Unknown 117.8 69.5 22.2 13.1 11.2 6.6 18.3 10.8 0.0 0.0 0.0 0.0 169.6 100 Note: The 'AVG N' represents the mean count across all replicates. Page 19 Table 4: ARD law enforcement homicides, by matching variable and match outcome, 2003–09 and 2011 Probability match No match Matching variable 0.01 – 0.25 % AVG N % 0.51 – 0.75 AVG N % 0.76 – 0.99 AVG N % Certainty match AVG N Total AVG N % 1,939.5 53.6 221.0 6.1 190.1 5.3 150.8 4.2 6.8 0.2 Male 1,865.7 53.5 220.4 6.3 189.7 5.4 143.9 4.1 6.0 Female 73.8 56.3 0.6 0.5 0.4 0.3 6.9 5.3 0.8 17 or younger 57.5 53.8 10.7 10.0 5.4 5.0 2.8 2.7 0.0 0.0 30.5 28.5 107.0 100 18–24 427.7 51.4 52.4 6.3 52.4 6.3 39.9 4.8 1.1 0.1 258.5 31.1 832.0 100 25–34 555.0 51.4 69.4 6.4 64.7 6.0 46.0 4.3 0.9 0.1 344.1 31.9 1,080.0 100 35–44 426.7 53.6 41.6 5.2 35.5 4.5 37.3 4.7 2.2 0.3 252.7 31.7 796.0 100 45–54 297.3 58.2 30.0 5.9 21.1 4.1 14.6 2.9 0.8 0.2 147.1 28.8 511.0 100 55 or older 143.1 57.5 12.3 4.9 8.0 3.2 6.7 2.7 0.0 0.0 79.0 31.7 249.0 100 Unknown 32.2 71.5 4.5 10.1 3.0 6.7 3.5 7.8 1.7 3.8 0.0 0.0 45.0 100 White, nonHispanic 926.6 61.2 27.9 1.8 47.4 3.1 36.5 2.4 3.2 0.2 472.5 31.2 1,514.0 100 Black, nonHispanic 550.8 48.3 77.0 6.8 70.5 6.2 36.2 3.2 2.2 0.2 404.2 35.4 1,141.0 100 Hispanic, any race 322.9 44.6 89.1 12.3 56.1 7.7 54.9 7.6 1.4 0.2 199.7 27.6 724.0 100 All LE homicides AVG N 0.26 – 0.50 % AVG N % 1,111.9 30.7 3,620.0 100 0.2 1,063.4 30.5 3,489.0 100 0.6 48.5 37.1 131.0 100 Sex Age Race/ Hispanic origin Other 45.8 47.8 4.8 5.0 4.9 5.1 4.9 5.2 0.0 0.0 35.5 37.0 96.0 100 Unknown 93.2 64.3 22.2 15.3 11.2 7.7 18.3 12.6 0.0 0.0 0.0 0.0 145.0 100 Note: The 'AVG N' represents the mean count across all replicates. Page 20 Table 5 describes the characteristics of law enforcement homicides that were reported to the ARD program, the SHR, or both. No differences emerged in cases reported to either system or to both in terms of age and race/ Hispanic origin of the victim. The vast majority of all law enforcement homicides resulted from a firearm, with no difference in the proportion of homicides resulting from other means (e.g., other weapon) across data sources. Across ARD program years, there does not appear to be a difference in the characteristics of cases captured by the ARD Program, the SHR, or both. Page 21 Table 5: ARD law enforcement homicide decedent characteristics, by source, 2003–09 and 2011 Percent of all law enforcement homicide victims 2003–06 All years Decedent characteristics ARD only SHR only ARD and SHR 3.4 % 2.9 % ARD only 2007–09 SHR only ARD and SHR 3.2 % 3.0 % ARD only SHR only 2011 ARD and SHR ARD only SHR only ARD and SHR Age 17 or younger 3.0 % 3.3 % 3.3 % 4.1 % 3.5 % 1.5 % 1.1 % 1.7 % 18–24 22.1 24.8 24.1 22.2 24.9 25.1 22.0 25.3 23.8 21.9 22.2 22.5 25–34 28.6 31.2 31.2 29.3 31.4 31.4 29.0 30.6 31.5 26.3 33.6 30.2 35–44 22.0 21.1 22.0 23.4 23.3 23.6 21.1 18.9 20.8 20.3 18.0 21.0 45–54 15.3 12.5 12.7 14.2 11.2 10.5 14.5 13.3 13.4 19.9 16.2 16.0 55 or older 7.4 6.1 6.3 5.7 5.3 5.3 8.2 6.6 6.2 10.0 8.4 8.5 Unknown 1.7 1.0 0.8 2.0 0.7 1.2 2.0 1.4 0.7 0.2 0.5 0.1 Race/ Hispanic origin White, non-Hispanic 47.8 % 49.2 % 35.0 % 49.8 % 49.9 % 35.2 % 43.4 % 47.0 % 35.3 % 51.0 % 55.2 % 33.8 % Black, non-Hispanic 28.4 31.8 35.1 26.5 30.1 33.2 33.3 34.4 35.7 23.9 29.8 38.0 Hispanic, any race 16.7 15.2 23.9 17.1 15.9 25.5 16.2 14.6 22.5 16.3 13.3 23.2 Other 2.4 2.4 3.0 3.1 2.9 4.4 1.7 2.0 2.4 1.9 0.5 1.2 Unknown 4.8 1.4 3.1 3.5 1.1 1.8 5.4 1.9 4.1 6.9 1.2 3.7 Manner of death Firearm 96.3 % 96.0 % 97.0 % 99.4 % 97.0 % 99.9 % 90.6 % 94.9 % 92.5 % 99.4 % 94.8 % 100 % Other 3.7 4.0 3.0 0.6 3.0 0.1 9.4 5.1 7.5 0.6 5.2 0.0 Missing 0.0 0.0 0.0 0.1 0.0 0.0 0.0 0.0 0.0 0.0 0.0 0.0 Page 22 3.2 Variability in ARD methodology across states As described in Section 3.1, RTI calculated the size of the law enforcement homicide population in the United States and the ARD program coverage using two methods. The first, a simple, poststratified Lincoln-Petersen method, assumes that all nonreporting law enforcement agencies did not have any law enforcement homicides during the observation period and therefore has no agency adjustment. The second coverage estimate assumes that all nonreporting agencies have the same number of law enforcement homicides on average as agencies that did report to either the SHR or the ARD program. This poststratified Lincoln-Petersen assumes that the population of interest is closed geographically and in time and is of fixed size, with a constant ratio adjustment for unobserved agencies. Table 6 and figure 4 show coverage estimates using each method by the year observed. The highest coverage rate with no agency adjustment was found in the most recent collection year of 2011 (69%). Table 6: ARD coverage estimates and confidence intervals, by estimation approach, 2003– 09 and 2011 No agency adjustment Year 95% Lower bound Coverage estimate Agency adjustment 95% Upper bound 95% Lower bound Coverage estimate 95% Upper bound All study years 47.28 % 48.70 % 50.18 % 34.50 % 36.43 % 38.35 % 2003 36.87 41.84 46.82 21.96 26.61 31.27 2004 37.30 42.89 48.49 24.41 29.46 34.52 2005 33.67 38.55 43.43 19.90 24.25 28.59 2006 42.50 46.99 51.48 28.73 33.37 38.02 2007 46.45 50.51 54.58 35.18 39.61 44.04 2008 36.50 40.81 45.12 22.57 26.82 31.07 2009 51.37 54.98 58.59 35.00 39.64 44.29 2011 65.12 69.01 72.90 53.85 58.58 63.30 Page 23 Figure 4: Percent of law enforcement homicide universe covered by ARD, by estimation method, 2003–09 and 2011 Percent of estimated law enforcement homicides 100% 80% 69% No agency adjustment 60% 42% 43% 40% 55% 51% 47% 59% 41% 39% 40% 40% 20% 27% 29% 33% 27% 24% Agency adjustment 0% 2003 2004 2005 2006 2007 2008 2009 2011 Year The methodology for identifying ARD cases has changed over the observation period, and figure 4 suggests that the methods used in more recent years are associated with better coverage. To better understand the methodologies used to identify arrest-related deaths in 2011, RTI conducted interviews with state reporting coordinators (SRCs). These interviews explored the primary method each SRC used for identifying cases, whether direct reporting from law enforcement agencies was involved, and whether the SRCs conducted any sort of follow-up or verification of cases reported to them. In addition, RTI tracked information about reporting methods and SRC location throughout the 2011 data collection period. This information was used to further examine the utility of various methodologies for identifying arrest-related deaths, as implemented by the SRCs during the 2011 data collection. The remaining analyses examine differences in case matching by state and reporting method. RTI grouped states by the primary data collection methodology and the location of the SRCs. Then, RTI conducted analyses to compare the number of cases reported only to ARD, only to SHR, or to both programs. Because the methodology for reporting was determined largely through the SRC interviews and information gained during the 2011 data collection period, these analyses are limited to 2011 data only. Table 7 describes state-level variation in reporting. In 2011, 83% of law enforcement homicides reported to either system were estimated to be captured in the ARD program. Approximately 139 of the estimated 828 law enforcement homicides captured by either system were reported only to the SHR. Page 24 There is considerable variability among states in the proportion of law enforcement homicides that are reported only to the ARD program, only to SHR, or to both sources. Three states reported to both systems but had no estimated overlap between the two (Iowa, Minnesota, and Arkansas). In other words, no law enforcement homicides reported to ARD were matched to homicides found in the SHR, and the SHR deaths were not matched to any of the ARD deaths in these three states. Twelve states reported all law enforcement homicides captured by either system to ARD: Connecticut, Massachusetts, New Hampshire, New York, Kansas, North Dakota, Alabama, Mississippi, West Virginia, Alaska, Idaho, and Montana. No states reported law enforcement homicides only to the SHR. Reporting to ARD is estimated to capture at least 75% of cases reported to either system for 38 states, 11 of which reported at least 20 law enforcement homicides to either system in 2011. Missouri, Maryland, Hawaii, and Nevada were each estimated to report more cases to the SHR than the ARD program. However, ARD still captured 82% of the estimated cases in Maryland and 84% of the cases in Nevada, and Hawaii had very few arrest-related deaths reported to either system. In Missouri, half of the cases reported to either system were found in the ARD, but 87% of the cases reported to either system were found in the SHR. Rhode Island, Vermont, Delaware, and Wyoming did not report any law enforcement homicides to either system in 2011. Table 7: ARD law enforcement homicides, by region, state, and source, 2011 ARD only Region and state SHR only ARD and SHR AVG N Row % Col % AVG N Row % Col % AVG N Row % Col % 361.3 43.6 100 139.3 16.8 100 327.7 39.6 100 50.0 55.6 13.8 9.0 10.0 6.5 31.0 34.4 9.5 Connecticut 2.0 100 0.6 0.0 0.0 0.0 0.0 0.0 0.0 Maine 2.6 30.6 0.7 2.6 30.6 1.9 3.4 38.9 1.0 Massachusetts 5.0 100 1.4 0.0 0.0 0.0 0.0 0.0 0.0 New Hampshire 4.0 80.0 1.1 0.0 0.0 0.0 1.0 20.0 0.3 New Jersey 4.0 25.0 1.1 4.0 25.0 2.9 8.0 50.0 2.4 26.0 100 7.2 0.0 0.0 0.0 0.0 0.0 0.0 Pennsylvania 6.4 23.3 1.8 2.4 8.7 1.7 18.6 68.0 5.7 Rhode Island 0.0 0.0 0.0 0.0 0.0 0.0 0.0 0.0 0.0 Vermont 0.0 0.0 0.0 0.0 0.0 0.0 0.0 0.0 0.0 78.7 50.6 21.8 31.7 20.4 22.8 45.3 29.1 13.8 Illinois 9.1 28.4 2.5 1.1 3.4 0.8 21.9 68.2 6.7 Indiana 8.7 46.6 2.4 5.7 30.6 4.1 4.3 22.8 1.3 Iowa 4.0 80.0 1.1 1.0 20.0 0.7 0.0 0.0 0.0 Kansas 6.0 85.7 1.7 0.0 0.0 0.0 1.0 14.3 0.3 Michigan 8.0 44.4 2.2 5.0 27.8 3.6 5.0 27.8 1.5 Minnesota 2.0 50.0 0.6 2.0 50.0 1.4 0.0 0.0 0.0 Missouri 2.4 13.2 0.7 9.4 51.2 6.8 6.6 35.6 2.0 Nebraska 1.0 33.3 0.3 1.0 33.3 0.7 1.0 33.3 0.3 North Dakota 1.0 100 0.3 0.0 0.0 0.0 0.0 0.0 0.0 Total Northeast New York Midwest Page 25 ARD only Region and state SHR only ARD and SHR AVG N Row % Col % AVG N Row % Col % AVG N Row % Col % 29.5 88.0 8.2 0.5 1.4 0.3 3.5 10.6 1.1 South Dakota 3.0 42.9 0.8 3.0 42.9 2.2 1.0 14.3 0.3 Wisconsin 4.0 50.0 1.1 3.0 37.5 2.2 1.0 12.5 0.3 125.8 41.0 34.8 52.8 17.2 37.9 128.2 41.8 39.1 Alabama 7.0 100 1.9 0.0 0.0 0.0 0.0 0.0 0.0 Arkansas 3.0 75.0 0.8 1.0 25.0 0.7 0.0 0.0 0.0 Delaware 0.0 0.0 0.0 0.0 0.0 0.0 0.0 0.0 0.0 District of Columbia 0.4 6.6 0.1 0.4 6.6 0.3 4.6 86.8 1.4 Florida 20.8 24.2 5.8 19.8 23.1 14.2 45.2 52.7 13.8 Georgia 12.9 43.1 3.6 3.9 13.0 2.8 13.1 43.8 4.0 Kentucky 8.0 61.5 2.2 3.0 23.1 2.2 2.0 15.4 0.6 Louisiana 3.7 42.8 1.0 1.7 20.0 1.3 3.3 37.2 1.0 Maryland 2.8 13.4 0.8 3.8 18.2 2.7 14.2 68.3 4.3 Mississippi 7.0 87.5 1.9 0.0 0.0 0.0 1.0 12.5 0.3 12.0 80.0 3.3 1.0 6.7 0.7 2.0 13.3 0.6 Oklahoma 5.1 31.5 1.4 3.1 19.1 2.2 7.9 49.4 2.4 South Carolina 5.4 52.1 1.5 3.4 32.9 2.5 1.6 15.0 0.5 Tennessee 2.1 15.8 0.6 2.1 15.8 1.5 8.9 68.5 2.7 Texas 23.1 47.1 6.4 7.1 14.5 5.1 18.9 38.4 5.8 Virginia 10.5 56.8 2.9 2.5 13.6 1.8 5.5 29.6 1.7 2.0 100 0.6 0.0 0.0 0.0 0.0 0.0 0.0 106.7 38.7 29.5 45.7 16.6 32.8 123.3 44.7 37.6 Alaska 1.0 50.0 0.3 0.0 0.0 0.0 1.0 50.0 0.3 Arizona 5.0 21.7 1.4 3.0 13.0 2.1 15.0 65.3 4.6 California 52.7 34.7 14.6 27.7 18.3 19.9 71.3 47.0 21.8 Colorado 10.1 59.1 2.8 5.1 29.8 3.7 1.9 11.1 0.6 Hawaii 0.1 4.4 0.0 2.1 68.1 1.5 0.9 27.4 0.3 Idaho 3.0 100 0.8 0.0 0.0 0.0 0.0 0.0 0.0 Montana 2.0 100 0.6 0.0 0.0 0.0 0.0 0.0 0.0 Nevada 1.6 9.4 0.4 2.6 15.4 1.8 12.4 75.2 3.8 New Mexico 5.9 49.4 1.6 1.9 15.6 1.3 4.1 35.0 1.3 Oregon 3.2 61.7 0.9 0.2 4.3 0.2 1.8 33.9 0.5 Utah 2.6 39.4 0.7 1.6 24.3 1.1 2.4 36.3 0.7 19.6 58.3 5.4 1.6 4.7 1.1 12.4 37.0 3.8 0.0 0.0 0.0 0.0 0.0 0.0 0.0 0.0 0.0 Ohio South North Carolina West Virginia West Washington Wyoming Note: These are the mean counts across all replicates. Page 26 3.2.1 ARD law enforcement homicide reporting methodology Primary data collection methodology was determined through interviews with the SRCs in 2012 and from information gathered during the 2011 data collection period (see table 7). Most states (N = 26) relied solely on media to identify ARD-eligible cases. ARD program staff identified cases in four states, also relying primarily on media sources. Eight states used direct reporting from local law enforcement agencies, and seven states relied on direct reporting from medical examiner’s/coroner’s offices. Four states accessed the National Violent Death Reporting System (NVDRS). Finally, two states had a legislative mandate to report arrest-related deaths. Table 7: Primary methodology for identifying arrest-related deaths, by state, 2011 Legislative mandate Direct law enforcement agency report Direct medical examiner’s/coroner’s report National Violent Death Reporting System KY Media only CA AL CO TX DC ME KS MA MO NM UT NH NC FL ND NV SC HI OH TN WA ID PA IL RI IN SD IA VA LA WV MD MS MI MT VT ArrestRelated Death program staff AK MN AR OK AZ NE GA OR CT NY WI DE NJ WY Note: States are listed using standard U.S. postal abbreviations. Figure 5 shows that there was not much variability in the proportion of observed law enforcement homicides that were found in the ARD program based on the primary identification method. The proportion of observed cases found in ARD ranged from a low of 80% for states that relied primarily on direct law enforcement agency reports to a high of 85% for states that relied on reports from medical examiner’s or coroner’s offices. The lack of association between the primary identification method and the proportion of cases found in ARD could be due to imprecise methods for categorizing states. For example, there may be additional variability within primary methodologies. Some states with direct law enforcement agency reports may have received these from only a few agencies in the state, and without a backup form of identification, were missing many law enforcement homicides. The majority of states relied on media reports, but there was likely to be considerable variability in the number of media sources reviewed, whether such review was proactive or reactive, and whether media-identified reports were then verified. Furthermore, the information RTI received about primary identification methodology may not have reflected the true methods that the SRC uses in that state, due to incomplete or outdated information collected during the SRC interviews or during the 2011 data collection period. Page 27 Figure 5: Law enforcement homicides, by source and primary reporting method, 2011 ARD only 90% SHR only Total cases (avg across replicates) 900 828 17% 16% 17% 15% 20% 80% 19% 800 19% 700 70% 600 60% 500 50% 371 400 40% 300 30% 201 200 20% 102 10% 72 42 41 ARD program staff identification only Access to and use of NVDRS 0% 100 - All states Media report only Legislative mandate Direct medical examiner/ coroner's office report Direct law enforcement agency report Primary identification methodology Page 28 Total number of law enforcement homicides observed Proportion of observed cases captured in each source 100% ARD and SHR 3.2.2 Location of SRC States were also categorized by the location of the SRC. The greatest number of SRCs (25) were located in a planning, commission, or statistics agency, such as a statistical analysis center, a research or data agency within the state’s department of criminal justice, or a planning organization (see table 8). SRCs in 13 states were situated in a state-level law enforcement or public safety department. There were three SRCs in a state prosecutor’s office, three in a medical examiner/coroner’s office or health agency, and three in a state corrections agency. Figure 6 describes the proportion of observed law enforcement homicides that were found in ARD by SRC location. SRCs located in state prosecutors’ offices and statistical analysis or other research centers, and those located in “other” locations, each had approximately 87% of the observed cases found in ARD, and only 13% of the observed cases found only in SHR. SRCs located in state law enforcement agencies and state corrections agencies had the lowest proportion of observed cases found in ARD (76% and 75%, respectively). State law enforcement agencies may not have collected data regularly from local law enforcement agencies to report to ARD, or they may have captured data only from a subset of agencies in the state. Data reported to the SHR, on the other hand, came directly from local law enforcement agencies. SRCs located in state corrections agencies may not have had the direct access or necessary relationships to regularly collect information on law enforcement homicides that occurred during the process of arrest. Further analysis may be warranted to determine how staff in state law enforcement agencies work with local agencies to identify and collect information about law enforcement homicides. Table 8: SRC agency location, 2011 State law enforcement agency or public safety dept State prosecutors office Medical examiner/coroner’s office or health agency CO NH ME FL ND NC IN HI TX OR LA MA MI MN IL UT State corrections agency ID Planning, commission, or statistics agency AK NM AR AZ NY GA CA OH WI CT OK WY DE PA MO IA VT RI KS VA SC KY WV SD MD AL TN NV MS WA NE MT DC NJ Note: States are listed using standard U.S. postal abbreviations. Page 29 ArrestRelated Death program staff Figure 6: Law enforcement homicides, by source and SRC location, 2011 ARD only 90% SHR only Total cases (avg across replicates) 900 828 17% 13% 13% 13% 19% 80% 24% 25% 800 700 70% 600 60% 500 451 50% 400 40% 30% 300 221 200 20% 55 10% 42 30 29 0% 100 - All states Statistical analysis or other research center State law enforcement agency State prosecutors ARD program staff State corrections office agency SRC location Page 30 Other Total number of observed law enforcement homicides Proportion of observed homicides found in each source 100% ARD and SHR 3.3 Number of years at least one death was reported to ARD and SHR Table 9 describes the number of agencies reporting at least one death for the 8 years observed in these analyses, and the estimated number of deaths reported by those agencies. Overall, more unique agencies reported to ARD in the years observed, 2003 through 2009 and 2011 (N = 1,261), compared to SHR (N = 881). For both systems, the majority of these unique agencies reported a death in only 1 of the 8 years examined. Fifteen agencies reported at least 1 death to ARD in all 8 years, and 28 agencies reported at least 1 death to SHR in all 8 years. The number of unique agencies reporting deaths in 7 or 8 years is higher for the SHR system compared to the ARD program. This finding suggests that the SHR is more likely to include data from agencies that consistently have at least one law enforcement homicide each year, as compared to the ARD program. Agencies that do not have law enforcement homicides each year are more likely to be found in the ARD data. Table 9: Number of years at least one death was reported to ARD and SHR ARD Number of years at least one death was reported Total SHR Number of agencies Number of deaths Number of agencies Number of deaths 1,261 3,620 881 3,385 8 15 696 28 1075 7 13 348 17 368 6 23 278 20 240 5 23 262 18 149 4 42 273 40 304 3 81 358 77 295 2 189 478 167 401 1 875 927 514 553 Page 31 4 Conclusions and recommendations The capture-recapture analysis described above estimates that, at best, the Arrest-Related Deaths (ARD) program captured approximately half of all law enforcement homicides in the United States during the study period (2003–09, 2011). This estimate assumes that all unobserved law enforcement agencies had zero law enforcement homicides. If those unobserved agencies did have a similar number of law enforcement homicides as those that did report to the ARD program or the Supplementary Homicide Reports (SHRs), the ARD coverage estimate is reduced to 36%. This information alone may be sufficient for BJS to determine whether to continue the ARD data collection program. Additional considerations for BJS to explore regarding continuing data collection through the ARD program are described below. 4.1 Relative strengths and weaknesses of the ARD program methodology A primary strength of the ARD program is that it was the only data collection that attempted to enumerate all arrest-related deaths in the United States. Unlike the SHR, or any other national- or state-level database, it also included accidental and natural deaths that occurred during the process of arrest in addition to law enforcement homicides. Although the current analyses did not identify any specific program methodologies that were more likely to identify law enforcement homicides than others, we did not examine their utility for identifying other types of arrestrelated deaths. 4.1.1 Types of cases that were covered well by the ARD program Analyses of decedent characteristics did not reveal any meaningful differences in capture rates by age, sex, or race/Hispanic origin. The 2011 data collection year did exhibit some differences across decedent characteristics compared to previous years, but these differences were not confined to cases found only in the ARD program. 4.1.2 Identification approaches that are associated with better coverage The analyses described above did not identify specific methodologies that were associated with increased coverage. There was little variation in the percentage of observed cases found in ARD (as opposed to the SHR) based on the primary methodology employed by the SRCs. For example, our analyses indicated that 20% of all observed homicides were found only in the SHR for states where the SRC relied primarily on direct reports from law enforcement agencies to identify homicides. In other words, ARD captured 80% of the observed homicides in these states. Eighty-five percent of the observed law enforcement homicides in states that relied primarily on data from medical examiner’s or coroner’s offices were found in the ARD program. Among these states, 15% of the cases observed in 2011 were found only in the SHR. Examining state-level variations in reporting and matching those directly to the time put in by the SRC, the location of the SRC, and the primary identification methodology will likely shed more light on effective identification practices. BJS may also further examine the policies and practices in California and Texas. Both states have mandated reporting legislation, but our analysis suggests that approximately a fifth of the observed cases in California in 2011 were reported to the SHR only, while 14% of the observed cases in Texas in 2011 were reported to the SHR only. Page 32 4.2 Recommendations While the capture-recapture analysis estimated that approximately half of all law enforcement homicides were not captured in the ARD program during the study period, the estimated rate of coverage for ARD in 2009 and 2011 was higher than the earlier years examined. In 2003, we estimated that the ARD program captured, at best, 42% of the universe of law enforcement homicides in the United States. In 2011, that coverage estimate increased to 69%. However, the analyses described above did not uncover any significant variations in coverage for observed homicides based on the location of the SRC or the primary identification methodology used in 2011. It is possible that all of the states were using more rigorous methodologies in 2011 as compared to earlier data collection years. Even states that relied primarily on media to identify cases may have been applying a comprehensive and regular approach to identifying these cases and following up with other agencies to verify the death. In fact, most states relied on multiple approaches to identify cases, but only the primary method was analyzed in this report. In general, more of the observed law enforcement homicides in 2011 were found in the ARD program compared to the SHR. There was still considerable state-to-state variation in reporting to one or both programs, however, and our analyses failed to identify specific reasons for this variation, such as the SRC’s location or the primary identification methodology used. A combination of data from both the SHR and the ARD is estimated to capture, at best, 72% of all law enforcement homicides in the United States across all years observed. In 2011, the ARD program captured, at most, an estimated 69% of the universe. Despite demonstrated improvements in the ARD program coverage over time, there are still a large number of law enforcement homicides that go unreported, indicating that the ARD is not a census. A first step to increasing the coverage of ARD is to define a consistent data collection approach across states, as opposed to the variable SRC-driven data collection methodology employed in 2011. More guidance or even requirements for where the SRC is located, how cases are identified, and verification processes may increase the reliability and coverage of the program. However, such an approach would increase the burden of this voluntary program, and would need to be balanced with the utility of the data for data providers and stakeholders at both the national and the local level. The analyses described above only examined one alternative source for identifying law enforcement homicides. BJS may further study other potential alternatives to the SRC-focused data collection methodology to determine if any provide more cost-effective or more valid and reliable means of identifying arrest-related deaths, including primary data collection or secondary analyses of existing data sources. Primary data collection methods such as direct surveys of law enforcement agencies or medical examiner’s/coroner’s offices could identify law enforcement homicides and the circumstances surrounding those homicides in the jurisdictions surveyed. Direct surveys would involve an additional burden than the ARD program’s SRC-focused approach through the cost of fielding the study and the time required to collect the data, as well as the burden on survey respondents. If the survey has a sufficient response rate and demonstrated validity, however, it may be an accurate measure of law enforcement homicides in the United States. Page 33 Less burdensome methodologies could include data collection from open sources. Open sources are publicly available data compiled through targeted searches, such as Google Alerts and review of specific websites devoted to law enforcement homicides. Open sources also include public documents such as press releases from states’ attorney general offices, medical examiners, or state police agencies. For example, the New York City Police Department releases an annual report describing all law enforcement homicides in that jurisdiction. Finally, fee-based services such as www.webclipping.com can be used to identify information from a large number of media outlets. Reliance on open source methods, while cost-effective, has a number of limitations. For example, media sources are often limited to “newsworthy” deaths and may be biased toward higher profile cases. Furthermore, there may be considerable regional and local variation in media coverage for law enforcement homicide-related events. Google Alerts and web-clipping services are also constrained by the search terms provided, which must be balanced to include all potentially eligible law enforcement homicides while not resulting in an unmanageable number of “alerts” to review. The timeliness of open source searches presents another limitation, because information on webbased news platforms may be available for a limited time. Most importantly, however, the quality of information from open sources is unknown, and the accuracy, reliability, and type of content provided is likely to vary over time, by source, and in comparison to official records. Other alternative measures of law enforcement homicides include existing data maintained by the Federal Bureau of Investigation (FBI) and the Centers for Disease Control and Prevention (CDC). Supplementary Homicide Reports (SHRs) are collected nationally as part of the FBI’s Uniform Crime Reporting Program (UCR), where information is voluntarily provided by law enforcement agencies to their state UCR programs and then to the FBI. The SHR has the potential to identify or confirm ARD cases categorized as a “homicide by law enforcement officer(s).” Other publicly available data sources that may also measure law enforcement homicides include the FBI’s National Incident Based Reporting System (NIBRS), and CDC’s National Vital Statistics System (NVSS) and its National Violent Death Reporting System (NVDRS) Each of these data sources has known limitations. For example, data from NIBRS, NVDRS, and local law enforcement agency websites are not national in scope, and are available only for certain jurisdictions. Data from the SHR and NVSS are national in scope, but do not contain unique identifiers that would allow precise case-level matching and comparison with the cases submitted to the ARD program. Studies have further documented inconsistencies in reporting to the ARD, SHR, and NVSS both within and across states (Loftin et al., 2003; Mumola, 2007; RTI International, 2012). The ability of any of these systems to accurately capture the universe of all arrest-related deaths as defined by the ARD program, or law enforcement homicides in particular, is currently unknown. 4.3 Study limitations The findings described above have several limitations, some of which may be addressed in additional analyses as indicated by BJS. First, the study is limited by the approach to including all probable and certainty matches in the analyses reported in exhibits 8, 10, 12, and 14. BJS may choose to limit the cases identified as a match to those with a probability score cutoff of 0.50 or higher, or to limit them to certainty matches only. The analyses presented here represent the “best case scenario” for ARD; any assumption changes will lower the number and proportion of observed cases that overlap across the two systems and increase the number and proportion of observed cases found in one system only. Page 34 The most significant limitation of this study, however, is measurement discrepancies between the SHR and ARD on matching variables, and the lack of a unique identifier to positively link cases across the systems. The monte carlo replicate approach implemented here provides the most rigorous means of addressing this limitation, but the shortcomings in the matching variable measurement remain. Findings were presented here as estimates of “cases,” but all estimates are based on the replicate approach, so any conclusions about the actual number of cases matched or covered by either system are not warranted. Instead, we can only estimate the level of matching and overlap across the two systems based on the information provided. Page 35 References Boriah, S., Chandola, V., & Kumar, V. 2008. Similarity measures for categorical data: A comparative evaluation. In proceedings of the eighth SIAM International Conference on Data Mining. 243–254. Loftin, C., Wiersema, B., McDowall, D. & Dobrin, A. (2003). Underreporting of justifiable homicides committed by police officers in the United States, 1976–1998. American Journal of Public Health, 93(7), 1117–1121. Mumola, C.J. (2007). Arrest-related deaths in the United States, 2003–2005. Bureau of Justice Statistics Special Report. (NCJ 219534). Washington, DC: U.S. Department of Justice, Office of Justice Programs. Retrieved from http://bjs.ojp.usdoj.gov/index.cfm?ty=pbdetail&iid=379. RTI International. (May 2012). Arrest-related deaths program: Program assessment, alternative approaches to data collection, and recommendations for program enhancement. Draft report submitted to the Bureau of Justice Statistics. Washington, DC: U.S. Department of Justice, Office of Justice Programs. Wolter, K.M. (1986). Some coverage error models for census data. Journal of the American Statistical Association, 81 (394): 338–346. Page 37 Appendix A: Similarity scoring for ARD/SHR matching Introduction Calculation of similarity scores in the context of matching data from the Arrest-Related Deaths (ARD) program with the Supplemental Homicide Reports (SHRs) requires a method that accounts for both continuous and categorical variables. Cases will be matched between data sources and within the originating agency identifier (ORI) code and victim sex on three measures: date of incident/date of death, age, and race/Hispanic origin, with the first two measures being continuous and the latter categorical. Given this mix of variables, RTI proposes calculating scores using a mixture of methods as follows. Continuous variables For the date and age variables, we propose use of a standardized Euclidean distance, combined with a similarity transformation. Let 𝑁𝑁𝑆𝑆𝑆𝑆𝑆𝑆 = number of records in SHR (within a given year) 𝑁𝑁𝐴𝐴𝐴𝐴𝐴𝐴 = number of records in ARD (within a given year) 𝑖𝑖 𝑗𝑗 = SHR record index where 𝑖𝑖 ∈ [1, 𝑁𝑁𝑆𝑆𝑆𝑆𝑆𝑆 ] = ARD record index where 𝑗𝑗 ∈ [1, 𝑁𝑁𝐴𝐴𝐴𝐴𝐴𝐴 ] 𝑑𝑑𝑆𝑆𝑆𝑆𝑆𝑆,𝑖𝑖 = SHR date (measured in number of days since January 1, 1960 5) for record i 𝑑𝑑𝐴𝐴𝐴𝐴𝐴𝐴,𝑗𝑗 = ARD date (measured in number of days since January 1, 1960) for record j 𝑎𝑎𝑆𝑆𝑆𝑆𝑆𝑆,𝑖𝑖 = SHR age for record i 𝑎𝑎𝐴𝐴𝐴𝐴𝐴𝐴,𝑗𝑗 = ARD age for record j 𝑑𝑑 −𝑚𝑚𝑒𝑒𝑒𝑒𝑒𝑒(𝑑𝑑 ) 𝑑𝑑́𝑆𝑆𝑆𝑆𝑆𝑆,𝑖𝑖 = 𝑆𝑆𝑆𝑆𝑆𝑆,𝑖𝑖𝑠𝑠𝑠𝑠𝑠𝑠(𝑑𝑑 ) 𝐴𝐴𝐴𝐴𝐴𝐴 𝐴𝐴𝐴𝐴𝐴𝐴 𝑑𝑑 −𝑚𝑚𝑚𝑚𝑚𝑚𝑚𝑚(𝑑𝑑𝐴𝐴𝐴𝐴𝐴𝐴 ) 𝑑𝑑́𝐴𝐴𝐴𝐴𝐴𝐴,𝑗𝑗 = 𝐴𝐴𝐴𝐴𝐴𝐴,𝑗𝑗 ) 𝑠𝑠𝑠𝑠𝑠𝑠(𝑑𝑑𝐴𝐴𝐴𝐴𝐴𝐴 5 The midpoint of the reported SHR month will be used to calculate the number of days since January 1, 1960, the standard date convention used by SAS statistical software. Page 38 𝑎𝑎́ 𝑆𝑆𝑆𝑆𝑆𝑆,𝑖𝑖 = 𝑎𝑎́ 𝐴𝐴𝐴𝐴𝐴𝐴,𝑗𝑗 = 𝑎𝑎𝑆𝑆𝑆𝑆𝑆𝑆,𝑖𝑖 −𝑚𝑚𝑚𝑚𝑚𝑚𝑚𝑚(𝑎𝑎𝐴𝐴𝐴𝐴𝐴𝐴 ) 𝑠𝑠𝑠𝑠𝑠𝑠(𝑎𝑎𝐴𝐴𝐴𝐴𝐴𝐴 ) 𝑎𝑎𝐴𝐴𝐴𝐴𝐴𝐴,𝑗𝑗 −𝑚𝑚𝑚𝑚𝑚𝑚𝑚𝑚(𝑎𝑎𝐴𝐴𝐴𝐴𝐴𝐴 ) 𝑠𝑠𝑡𝑡𝑡𝑡(𝑎𝑎𝐴𝐴𝐴𝐴𝐴𝐴 ) Then the standardized Euclidean distance between records i and j on date and age is given by 2 2 𝐷𝐷𝑖𝑖,𝑗𝑗 = ��𝑑𝑑́𝑆𝑆𝑆𝑆𝑆𝑆,𝑖𝑖 − 𝑑𝑑́𝐴𝐴𝐴𝐴𝐴𝐴,𝑗𝑗 � + �𝑎𝑎́ 𝑆𝑆𝑆𝑆𝑆𝑆,𝑖𝑖 − 𝑎𝑎́ 𝐴𝐴𝐴𝐴𝐴𝐴,𝑗𝑗 � This distance is then transformed to a similarity score as 𝑆𝑆𝑐𝑐𝑐𝑐𝑐𝑐;𝑖𝑖,𝑗𝑗 = where 1 1 + 𝐷𝐷𝑖𝑖,𝑗𝑗 0 < 𝑆𝑆𝑐𝑐𝑐𝑐𝑐𝑐;𝑖𝑖,𝑗𝑗 ≤ 1 Categorical variables Rather than simply counting matches on categorical variables as 1 and mismatches as 0, we propose the use of a penalized scoring approach similar to that described in Boriah et al., 2008. 6 This approach is based on the premise that matches that have a high probability of occurring by random chance should not count as much as those that would be observed very rarely by chance alone. For each categorical variable (currently only race/Hispanic origin), the similarity between two records i and j is given by the following. Let 𝑝𝑝̂ (𝑥𝑥) = the sample probability that 𝑋𝑋 = 𝑥𝑥 Then the similarity between records i and j on variable X is given by 1 − 𝑝𝑝̂ (𝑥𝑥𝑆𝑆𝑆𝑆𝑆𝑆 )𝑝𝑝̂ (𝑥𝑥𝐴𝐴𝐴𝐴𝐴𝐴 ) if 𝑥𝑥𝑆𝑆𝑆𝑆𝑆𝑆 = 𝑥𝑥𝐴𝐴𝐴𝐴𝐴𝐴 𝑆𝑆𝑋𝑋 = � 0 otherwise 0 ≤ 𝑆𝑆𝑋𝑋 < 1 6 The proposed method is similar to the Goodall3 approach described by Boriah et al., but adapted for two data sources. Page 39 Calculating the overall score The final similarity score for records i and j is the weighted mean of the various components and is given by the following: 2 1 𝑆𝑆𝑖𝑖,𝑗𝑗 = 3 𝑆𝑆𝑐𝑐𝑐𝑐𝑐𝑐;𝑖𝑖,𝑗𝑗 + 3 𝑆𝑆𝑅𝑅𝑅𝑅𝑅𝑅𝑅𝑅/𝐸𝐸𝐸𝐸𝐸𝐸;𝑖𝑖,𝑗𝑗 Page 40 Appendix B: Linking algorithm Estimate the agency population size and agency undercoverage adjustment factor To ensure that coverage estimates apply to the population of law enforcement (LE) homicides across all agencies and not only to the population of LE homicides occurring in agencies that have been observed, we must estimate the total population size of agencies with LE homicides (reported or unreported). To achieve this, we will use the Lincoln-Petersen (LP) estimator 7 as follows: (1) � = (𝑛𝑛𝐴𝐴𝐴𝐴𝐴𝐴 +1)(𝑛𝑛𝑆𝑆𝑆𝑆𝑆𝑆 +1) − 1 𝑁𝑁 𝑛𝑛 +1 𝐴𝐴𝐴𝐴𝐴𝐴||𝑆𝑆𝑆𝑆𝑆𝑆 In (1), nARD is the number of agencies in ARD, nSHR is the number of agencies in SHR, and � is given by nARD||SHR is the number of agencies represented in both sources. The variance of N the following: �� = (2) 𝑉𝑉𝑉𝑉𝑉𝑉�𝑁𝑁 �𝑛𝑛𝐴𝐴𝐴𝐴𝐴𝐴 +1��𝑛𝑛𝑆𝑆𝑆𝑆𝑆𝑆 +1��𝑛𝑛𝐴𝐴𝐴𝐴𝐴𝐴 −𝑛𝑛𝐴𝐴𝐴𝐴𝐴𝐴||𝑆𝑆𝑆𝑆𝑆𝑆 ��𝑛𝑛𝑆𝑆𝑆𝑆𝑆𝑆 −𝑛𝑛𝐴𝐴𝐴𝐴𝐴𝐴||𝑆𝑆𝑆𝑆𝑆𝑆 � 2 �𝑛𝑛𝐴𝐴𝐴𝐴𝐴𝐴||𝑆𝑆𝑆𝑆𝑆𝑆 +2� 𝑛𝑛𝐴𝐴𝐴𝐴𝐴𝐴||𝑆𝑆𝑆𝑆𝑆𝑆 The number of agencies observed across ARD and SHR is given by 𝑛𝑛 = 𝑛𝑛𝐴𝐴𝐴𝐴𝐴𝐴 + 𝑛𝑛𝑆𝑆𝐻𝐻𝐻𝐻 − 𝑛𝑛𝐴𝐴𝐴𝐴𝐴𝐴||𝑆𝑆𝑆𝑆𝑆𝑆 and the agency undercoverage adjustment factor is given by 𝑅𝑅 = � 𝑁𝑁 𝑛𝑛 and its variance is given by 𝑉𝑉𝑉𝑉𝑉𝑉(𝑅𝑅) = �) 𝑉𝑉𝑉𝑉𝑉𝑉(𝑁𝑁 𝑛𝑛2 Classify cases as matchable or unmatchable For the purposes of record linkage, cases are separated into two groups determined by whether or not it is possible to match them between sources, within characteristic classifications that must be the same: originating agency identifier (ORI) code, sex, and year. Cases from either source 7 By incorporating agency-level characteristics into a stratified LP estimate, we may reduce bias induced by the assumption that agencies are observed with constant probability across agency characteristics. Page 41 that cannot be matched on these three characteristics are considered unmatchable and are excluded from subsequent probabilistic linking. Probabilistic linking between ARD and SHR For cases considered matchable across sources, probabilistic linking is used to determine whether a case in the Arrest-Related Deaths (ARD) program is the same as a case in the Supplementary Homicide Reports (SHRs). Within each distinct combination of ORI, sex, and year, probabilistic linking proceeds according to the following steps: a. Randomly sort the ARD cases b. For each ARD case i, calculate a similarity score, 𝑆𝑆𝑖𝑖,𝑗𝑗 , corresponding to each SHR case j c. Create a 0/1 indicator, 𝑢𝑢𝑖𝑖,𝑗𝑗 , indicating whether SHR case j has already been linked to another ARD record ′ d. Define 𝑆𝑆𝑖𝑖,𝑗𝑗 = 𝑆𝑆𝑖𝑖,𝑗𝑗 ∗ (1 − 𝑢𝑢𝑖𝑖,𝑗𝑗 ) e. Create a 0/1 indicator, 𝑡𝑡𝑖𝑖,𝑗𝑗 , for whether or not each SHR case is within acceptable threshold for being considered the same as the ARD case in question (an SHR case is considered within threshold if the difference in dates of incident and death is less than or equal to 30 days, the ages are less than or equal to 5 years apart, and the race/Hispanic origin matches exactly); Note that if 𝑢𝑢𝑖𝑖,𝑗𝑗 = 1 then 𝑡𝑡𝑖𝑖,𝑗𝑗 = 0 f. Treating the scores as unscaled probabilities, calculate the probability that the ARD case is not represented in SHR as follows 𝑘𝑘 ′ 𝑆𝑆𝑖𝑖,𝑛𝑛𝑛𝑛𝑛𝑛𝑛𝑛 = ��1 − 𝑆𝑆𝑖𝑖,𝑗𝑗 � (1 − 𝑡𝑡𝑖𝑖,𝑗𝑗 ) 𝑗𝑗=1 g. Determine the number of cases that are within threshold as 𝑘𝑘 𝑛𝑛𝑡𝑡 = � 𝑡𝑡𝑖𝑖,𝑗𝑗 𝑗𝑗=1 ′′ ′ h. If 𝑛𝑛𝑡𝑡 > 0 then define 𝑆𝑆𝑖𝑖,𝑗𝑗 = 𝑆𝑆𝑖𝑖,𝑗𝑗 ∗ 𝑡𝑡𝑖𝑖,𝑗𝑗 ′′ ′ i. Otherwise, if 𝑛𝑛𝑡𝑡 = 0, then define 𝑆𝑆𝑖𝑖,𝑗𝑗 = 𝑆𝑆𝑖𝑖,𝑗𝑗 𝑝𝑝𝑖𝑖,𝑗𝑗 = j. Define 𝑝𝑝𝑖𝑖,𝑗𝑗 , the probability that ARD case i is the same as SHR case j as ′′ 𝑆𝑆𝑖𝑖,𝑗𝑗 𝑗𝑗 ′′ 𝑆𝑆𝑖𝑖,𝑛𝑛𝑛𝑛𝑛𝑛𝑛𝑛 + ∑1 𝑆𝑆𝑖𝑖,𝑗𝑗 k. Define the probability of no match as Page 42 𝑝𝑝𝑖𝑖,𝑛𝑛𝑛𝑛𝑛𝑛𝑛𝑛 = 𝑆𝑆𝑖𝑖,𝑛𝑛𝑛𝑛𝑛𝑛𝑛𝑛 𝑗𝑗 ′′ 𝑆𝑆𝑖𝑖,𝑛𝑛𝑛𝑛𝑛𝑛𝑛𝑛 + ∑1 𝑆𝑆𝑖𝑖,𝑗𝑗 l. Define the cumulative probability of record linkage as 𝑗𝑗 𝑐𝑐𝑖𝑖,𝑗𝑗 = � 𝑝𝑝𝑖𝑖,𝑞𝑞 𝑞𝑞=1 m. Select a random variate 𝑥𝑥𝑖𝑖 , from 𝑋𝑋~𝑈𝑈𝑈𝑈𝑈𝑈𝑈𝑈𝑈𝑈𝑈𝑈𝑈𝑈(0,1) n. Link ARD case i with SHR case j if case j is the first case (going from 1 to k) such that 𝑐𝑐𝑖𝑖,𝑗𝑗 > 𝑥𝑥𝑖𝑖 o. If this condition is not satisfied, ARD case i will be linked to no SHR cases with probability 𝑝𝑝𝑖𝑖,𝑛𝑛𝑛𝑛𝑛𝑛𝑛𝑛 Recombine cases Once matchable cases have gone through the probabilistic linking process, they are recombined with cases previously determined to be unmatchable due to a lack of overlap on key variables (ORI, sex, and year). Once recombined, the number of individual homicides in ARD, in SHR, and in both ARD and SHR are tabulated; these are denoted as 𝑚𝑚𝐴𝐴𝐴𝐴𝐴𝐴 , 𝑚𝑚𝑆𝑆𝑆𝑆𝑆𝑆 , and 𝑚𝑚𝐴𝐴𝐴𝐴𝐴𝐴||𝑆𝑆𝑆𝑆𝑆𝑆 , respectively. Stratify by individual-level characteristics Since homogeneity of capture probabilities cannot be assumed within or across lists, the LP estimator of population size may contain downward bias, leading to an overestimation of coverage (Wolter, 1986). A common method for amelioration of this bias—and the one we employ—is poststratification. Once record linkage has occurred, cases will be grouped into 𝑠𝑠 strata, defined by the cross-classification of demographic characteristics. If these characteristics are correlated with capture probability, bias incurred through use of the LP estimator will be reduced – the choice of characteristics is limited to information available in both sources (age, race/Hispanic origin, and sex). Estimate population size and variance by stratum Within each stratum 𝑙𝑙, with 1 ≤ 𝑙𝑙 ≤ 𝑠𝑠, obtain the LP estimate of population size and its associated variance as (3) �𝑙𝑙 = �𝑚𝑚𝑙𝑙,𝐴𝐴𝐴𝐴𝐷𝐷 +1�(𝑚𝑚𝑙𝑙,𝑆𝑆𝑆𝑆𝑆𝑆 +1) − 1 𝑀𝑀 𝑚𝑚 +1 𝑙𝑙,𝐴𝐴𝐴𝐴𝐴𝐴||𝑆𝑆𝑆𝑆𝑆𝑆 � 𝑙𝑙 is given by the following: The variance of M � 𝑙𝑙 � = (4) 𝑉𝑉𝑉𝑉𝑉𝑉�M �𝑚𝑚𝑙𝑙,𝐴𝐴𝐴𝐴𝐴𝐴 +1��𝑚𝑚𝑙𝑙,𝑆𝑆𝑆𝑆𝑆𝑆 +1��𝑚𝑚𝑙𝑙,𝐴𝐴𝐴𝐴𝐴𝐴 −𝑚𝑚𝑙𝑙,𝐴𝐴𝐴𝐴𝐴𝐴||𝑆𝑆𝑆𝑆𝑆𝑆 ��𝑚𝑚𝑙𝑙,𝑆𝑆𝑆𝑆𝑆𝑆 −𝑚𝑚𝑙𝑙,𝐴𝐴𝐴𝐴𝐴𝐴||𝑆𝑆𝑆𝑆𝑆𝑆 � 𝑚𝑚2𝑙𝑙,𝐴𝐴𝐴𝐴𝐴𝐴||𝑆𝑆𝐻𝐻𝑅𝑅 �𝑚𝑚𝑙𝑙,𝐴𝐴𝐴𝐴𝐴𝐴||𝑆𝑆𝑆𝑆𝑆𝑆 +2� Page 43 Estimate population size By summing the stratum-specific population sizes, we obtain the overall population size among observed agencies: 𝑠𝑠 � = � 𝑀𝑀 �𝑙𝑙 𝑀𝑀 𝑙𝑙=1 � is given by the following: The variance of 𝑀𝑀 𝑠𝑠 � � = � 𝑉𝑉𝑉𝑉𝑉𝑉(𝑀𝑀 �𝑙𝑙 ) 𝑉𝑉𝑉𝑉𝑉𝑉�𝑀𝑀 𝑙𝑙=1 Adjust population size for agency undercoverage To obtain an estimate of the overall population size, 𝑃𝑃�, among all agencies with LE homicides, including those unobserved across lists, we apply the ratio from 1 as follows: � 𝑃𝑃� = 𝑅𝑅 ∗ 𝑀𝑀 The variance of 𝑃𝑃� is given by the following: � 2 𝑉𝑉𝑉𝑉𝑉𝑉(𝑅𝑅) + 𝑅𝑅 2 𝑉𝑉𝑉𝑉𝑉𝑉�𝑀𝑀 �� 𝑉𝑉𝑉𝑉𝑉𝑉�𝑃𝑃�� = 𝑀𝑀 Estimate ARD coverage ARD coverage is estimated as the number of cases observed in ARD, divided by the estimated population size: (5) 𝐶𝐶 = 𝑚𝑚𝐴𝐴𝐴𝐴𝐷𝐷 𝑃𝑃� The variance of 𝐶𝐶 is given by the following: (6) 𝐶𝐶 2 𝑉𝑉𝑉𝑉𝑉𝑉(𝐶𝐶) = 𝑃𝑃�2 ∗ 𝑉𝑉𝑉𝑉𝑉𝑉(𝑃𝑃�) Replication To achieve final estimates of coverage and variance that take into account uncertainty inherent to the probabilistic record linkage, the process above is repeated 1,000 times. In each of these replicates the linking procedure yields different results, and this variability is incorporated as follows: Let 𝑟𝑟 be the replicate index, where 1 ≤ 𝑟𝑟 ≤ 1,000, then the overall population size estimate is given by 1,000 1 𝑃𝑃� = � 𝑃𝑃�𝑟𝑟 1,000 𝑟𝑟=1 The variance of 𝑃𝑃� is given by the following: Page 44 1,000 1,000 𝑟𝑟=1 𝑟𝑟=1 1 1 𝑉𝑉𝑉𝑉𝑉𝑉�𝑃𝑃�� = � � 𝑉𝑉𝑉𝑉𝑉𝑉�𝑃𝑃�𝑟𝑟 �� + � � (𝑃𝑃�𝑟𝑟 − 𝑃𝑃�)2 � 1,000 999 The final coverage estimate and variance are obtained by replacing 𝑃𝑃� and 𝑉𝑉𝑉𝑉𝑉𝑉(𝑃𝑃�) with the above in equations (5) and (6), respectively. Page 45 Appendix C: Race/Hispanic origin coding Before matching and capture-recapture analyses may begin, RTI must recode race/Hispanic origin to be comparable between the Arrest-Related Deaths (ARD) program and Supplementary Homicide Report (SHR) data sources. This requirement presents a problem in that race/Hispanic origin is collected in a combined measure for the ARD and in two separate variables for the SHR. Since separating the combined ARD measure into two variables comparable to those that exist in the SHR would yield a missing race for all records currently coded as Hispanic, it is much more desirable to create a combined measure on the SHR file that is comparable to that in the ARD data. Given high levels of missingness in the SHR Hispanic origin measure, this approach is also not without problems. Hispanic origin in the SHR At the national level, Hispanic origin in the SHR is highly underreported, with a missingness rate of 53% in the years 2003–11. Cumulative V22 Frequency Percent Frequency 2051 53.44 (H) Hispanic origin 2051 600 (N) Not of Hispanic origin (U) Unknown or not reported 15.63 1101 86 2651 28.69 2.24 3752 3838 To examine whether SHR respondents may be using the Hispanic origin variable as an extra race category, when needed (i.e., when the deceased was obviously Hispanic), we explored the hypothesis that if the Hispanic origin responses that were not missing were mostly 1, it would be a strong indication that this was true. As shown in the frequency above, this is not the case. So, we dug a little deeper to see where the missing Hispanic origin cases were coming from, with the following result: State N Reported Hisp. CALIF 993 0.9073515 ƒƒƒƒƒƒƒƒƒƒƒƒƒƒƒƒƒƒƒƒƒƒƒƒƒƒƒƒƒƒƒƒƒ COLO 80 0 ALA CONN 0 10 0 ALASKA 18 DC 17 0 ARIZ 177 0.8983051 DEL 1 0 ARK FL 373 0 16 0 4 0 Page 46 GA 82 0.4512195 HAWAII 8 0 IDAHO 3 0 N MEX 46 NY 0 53 NEBR 0 11 0.1818182 ILL 137 0 NEV 79 0 IND 0 OHIO 44 0 50 IOWA 17 0 OKLA 92 0.5978261 KANS 0 OREG 33 0.6666667 14 KY 19 0 PA 185 0.9621622 LA 65 0 RI 1 SC 20 MAINE 18 MASS MD 0 11 0 169 0 0 0 S DAK 7 0 TENN 73 0 MICH 78 0.1153846 TEXAS 320 0.8937500 MINN 31 UTAH 23 MISS MO 2 0 VI 3 103 0.6116505 VA 45 VT 3 MONT NC 0 7 0 42 0.7619048 N DAK 1 NH 2 NJ 102 0 0 0 W VA 0 0 0 0 2 0 WASH 98 0.2346939 WIS WYO Page 47 47 0.4255319 3 0 Figure 7: ACS 5-year Hispanic origin, by county Highlighted in the table at left are the states where we would expect to find the largest Hispanic populations. Of the 2,068 cases occurring in these states, 72% come from states with high Hispanic origin coverage. This implies that the situation may not be as bad at the national level as it might seem based only on the frequency above. Assuming we were to create a combined race/Hispanic origin variable for the SHR, the distribution of white, black, and Hispanic nationally in 2003–09 would be 45.89%, 33.89%, and 16.28%, respectively. In the ARD, the corresponding breakdown is 42.06%, 31.03%, and 20.36%. If we subset to states with reporting rates for Hispanic origin greater than 75% (Arizona, California, North Carolina, Pennsylvania, and Texas), the SHR distribution of white, black, and Hispanic becomes 35.54%, 25.20%, and 34.01%, respectively. The corresponding breakdown in the ARD, when subset to the same states, is 32.38%, 26.39%, and 34.70%. Given these results, we follow an approach in which we create a combined race/Hispanic origin measure in the SHR and conduct both a national analysis as well as one subset to the states with high Hispanic origin reporting. Using only these five states would cover half of our national sample and would give us some insight into how sensitive the capture-recapture results are to the issue of data regarding Hispanic origin. Page 48 The Bureau of Justice Statistics of the U.S. Department of Justice is the principal federal agency responsible for measuring crime, criminal victimization, criminal offenders, victims of crime, correlates of crime, and the operation of criminal and civil justice systems at the federal, state, tribal, and local levels. BJS collects, analyzes, and disseminates reliable and valid statistics on crime and justice systems in the United States, supports improvements to state and local criminal justice information systems, and participates with national and international organizations to develop and recommend national standards for justice statistics. William J. Sabol is acting director. This report was written by Duren Banks, Lance Couzens, Caroline Blanton, and Devon Cribb, RTI International. Erin Kennedy, Crystal Daye, Lance G. Couzens, Devon S. Cribb, Caroline Blanton, and Duren Banks, RTI International provided statistical review and verification of the report. Lynne McConnell and Jill Thomas edited the report. Barbara Quinn produced the report. March 2015, NCJ 248543 Office of Justice Programs Innovation • Partnerships • Safer Neighborhoods www.ojp.usdoj.gov