American Economic Journal, Impacts of Private Prison Contracting on Inmate Time Served and Recividism, 2021
Download original document:
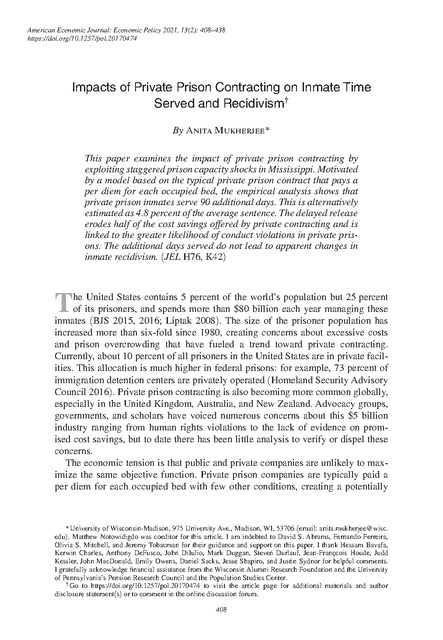
Document text
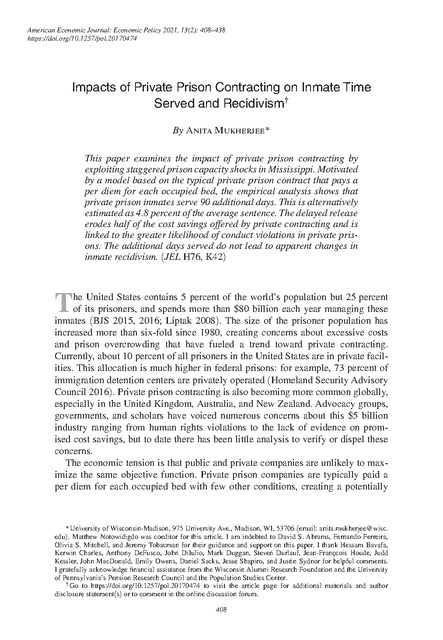
Document text
This text is machine-read, and may contain errors. Check the original document to verify accuracy.
American Economic Journal: Economic Policy 2021, 13(2): 408–438 https://doi.org/10.1257/pol.20170474 Impacts of Private Prison Contracting on Inmate Time Served and Recidivism† By Anita Mukherjee* This paper examines the impact of private prison contracting by exploiting staggered prison capacity shocks in Mississippi. Motivated by a model based on the typical private prison contract that pays a per diem for each occupied bed, the empirical analysis shows that private prison inmates serve 90 additional days. This is alternatively estimated as 4.8 percent of the average sentence. The delayed release erodes half of the cost savings offered by private contracting and is linked to the greater likelihood of conduct violations in private prisons. The additional days served do not lead to apparent changes in inmate recidivism. (JEL H76, K42) T he United States contains 5 percent of the world’s population but 25 percent of its prisoners, and spends more than $80 billion each year managing these inmates (BJS 2015, 2016; Liptak 2008). The size of the prisoner population has increased more than six-fold since 1980, creating concerns about excessive costs and prison overcrowding that have fueled a trend toward private contracting. Currently, about 10 percent of all prisoners in the United States are in private facilities. This allocation is much higher in federal prisons: for example, 73 percent of immigration detention centers are privately operated (Homeland Security Advisory Council 2016). Private prison contracting is also becoming more common globally, especially in the United Kingdom, Australia, and New Zealand. Advocacy groups, governments, and scholars have voiced numerous concerns about this $5 billion industry ranging from human rights violations to the lack of evidence on promised cost savings, but to date there has been little analysis to verify or dispel these concerns. The economic tension is that public and private companies are unlikely to maximize the same objective function. Private prison companies are typically paid a per diem for each occupied bed with few other conditions, creating a potentially * University of Wisconsin-Madison, 975 University Ave., Madison, WI, 53706 (email: anita.mukherjee@wisc. edu). Matthew Notowidigdo was coeditor for this article. I am indebted to David S. Abrams, Fernando Ferreira, Olivia S. Mitchell, and Jeremy Tobacman for their guidance and support on this paper. I thank Hessam Bavafa, Kerwin Charles, Anthony DeFusco, John DiIulio, Mark Duggan, Steven Durlauf, Jean-Françcois Houde, Judd Kessler, John MacDonald, Emily Owens, Daniel Sacks, Jesse Shapiro, and Justin Sydnor for helpful comments. I gratefully acknowledge financial assistance from the Wisconsin Alumni Research Foundation and the University of Pennsylvania’s Pension Research Council and the Population Studies Center. † Go to https://doi.org/10.1257/pol.20170474 to visit the article page for additional materials and author disclosure statement(s) or to comment in the online discussion forum. 408 VOL. 13 NO. 2 MUKHERJEE: IMPACTS OF PRIVATE PRISON CONTRACTING 409 perverse incentive for them to maximize the number of occupied beds. The extent to which these incentives can be powerful is highlighted by cases of corruption: for example, a private prison company paid two judges over $2.6 million to inflate offender sentences and assign them to its juvenile facility (Chen 2009). In another case relevant to the current study, the Commissioner of the Mississippi Department of Corrections and a h igh-ranking colleague recently had to step down from their posts and were sentenced to nearly 20 years in prison for accepting bribes to promote private contracting (Amy 2017). Despite evidence that private prison operators respond to contract incentives, little empirical work to date has examined whether inmates in private prison serve more time than those in public prison. Time served in prison is an important outcome because it is the primary punishment that society imposes on offenders. This punishment is carried out unfairly, however, if it varies systematically with whether an inmate is assigned to a private or public prison. Beyond the fairness aspect, the number of days a prisoner is incarcerated directly erodes the cost savings offered by private contracting. Yet, private contracting may still be appealing if competition improves quality, for example, through reductions in recidivism. Hence, I also study this outcome. This paper exploits the staggered entry and exit of private prisons in Mississippi between 1996 and 2004 to contribute instrumental variable (IV) estimates of the impact of private prison on inmate time served and recidivism. The rich set of inmate data available make it possible to control for a wide range of characteristics that are known to predict these outcomes. The striking differences across inmates in public and private prison raise concerns about selection on unobservable characteristics, however. Private prison inmates have longer sentences and have different racial, age, and marital status composition. They also serve a greater fraction of their sentences (73 percent versus 70 percent). If prison assignment is based on characteristics unobservable to the researcher, a credible empirical strategy requires a source of experimental or q uasi-experimental variation to draw valid conclusions about the effect of private prisons on inmate outcomes. The large capacity shocks from private prison openings, expansions, and closings provide this needed variation by serving as instruments for prison assignment. Figure 1 shows the daily inmate population across all private prisons: the state filled each private prison within two weeks of their opening or expanding, and then operated them at nearly full capacity. This pattern suggests that the probability with which an inmate was assigned to private prison is an increasing function of private prison bed capacity, a relationship that persists in a formal regression analysis. This finding enables an IV estimation in which the identifying assumption is that the sharp shocks to private prison bed capacity did not independently affect inmate time served or recidivism, an assumption that is plausible given that cost-cutting is typically the main motivation for private contracting. Prior research on comparing inmate outcomes in public versus private prisons has been limited to observational studies that do not address potentially nonrandom selection of inmates to private prison. They also focus mostly on recidivism, though an important exception is a working paper by Lindqvist (2008), which examines residential treatment centers for youth in Sweden. The paper finds that those in 410 AMERICAN ECONOMIC JOURNAL: ECONOMIC POLICY MAY 2021 Public prison 12,000 9,000 3,000 Private prison 6,000 2,000 3,000 1,000 0 Private prison daily population Public prison daily population 4,000 0 1996 1998 2000 2002 2004 2006 2008 2010 2012 Figure 1. Daily Prison Population by Facility, 1 996–2012 Notes: Daily inmate population in Mississippi for public and private prisons for all adult male inmates admitted between May 1, 1996 and May 1, 2012. The spikes (indicated by the vertical lines) correspond to shocks in the private prison capacity either through private prison entry, closure, or bed expansion. The dip in the private prison population in March 2001 corresponds to the opening of a juvenile private prison facility, where many inmates aged 18 to 20 were transferred upon its opening. The shaded region indicates data post July 31, 2004, which is not used in estimation (except in robustness checks) and is reserved for observing the conclusion of sentences served and recidivism. privately owned centers spent more days in treatment compared to those in p ublic centers. Some previous studies have found increases in recidivism for private prison inmates (Bayer and Pozen 2005, Spivak and Sharp 2008), while others have found no differences in this outcome (Bales et al. 2005; L anza-Kaduce, Parker, and Thomas 1999).1 The empirical models in these papers do not attempt to deal with inmate selection to private prison. Additionally, these prior studies do not typically use information on an inmate’s location throughout his sentence and instead limit the definition of private prison exposure to whether the inmate began or finished his sentence in such a location. This simplification causes mismeasurement in the extent of private prison exposure, a limitation that the present study addresses. I begin by constructing a model to help motivate the empirical analysis. As noted, the standard private prison contract pays a per diem for each occupied bed with limited additional contingencies; similar contracts are common in health care.2 1 Bayer and Pozen (2005) find that juvenile offenders released from private prisons have 5 to 8 percent higher rates of o ne-year recidivism; they study the role of nonprofit private prisons, which are more prevalent in the juvenile prison system. Bales et al. (2005) and Lanza-Kaduce, Parker, and Thomas (1999), however, use similar data and find no effect of private prison assignment on recidivism rates for male, female, or juvenile offenders in Florida. Spivak and Sharp (2008) estimate a 16 percent greater recidivism rate using data on adult male offenders in Oklahoma. 2 Per diem reimbursement is a key form of prospective payment method in health care (Casto and Forrestal 2013). These contracts are common in settings ranging from Medicaid nursing home reimbursements in the United States (Intrator et al. 2007) to hospital reimbursements in Asian countries (Jian and Guo 2009, Rodwin and Okamoto 2000). VOL. 13 NO. 2 MUKHERJEE: IMPACTS OF PRIVATE PRISON CONTRACTING 411 The theoretical prediction given this type of contract is that private operators may increase recidivism because they ignore the benefits of n oncontractible quality, for example, in the form of rehabilitation programs (Hart, Shleifer, and Vishny 1997). In the model, the private operator selects whether to distort release decisions based on the marginal profit and the level of government monitoring. The model also yields implications for recidivism based on the assumption that recidivism risk declines with time since offense, as in Kuziemko (2013). I study inmate time served using primarily a set of IV regressions. The instrument measures the capacity shock in private prisons experienced by each inmate; it equals the net number of private prison bed openings over the assigned sentence. The IV estimate shows that private prison inmates serve about 90 extra days, alternatively measured as 4.8 additional percent of their sentences. The OLS estimates are similar to the IV results, which suggests that the main controls used in these models are sufficient to address the most important sources of selection bias. I then explore a mechanism to explain the observed difference in inmate time served and establish that the widespread use of infractions (prison conduct violations) in private prisons is the likely reason for delayed release. Baseline infraction rates in public and private prisons are 18 and 46 percent, respectively. Even after controlling for all covariates, I find that an inmate in private prison is 9 to 14 percent more likely to be cited with an infraction over the course of his sentence. The final step in the analysis examines recidivism, defined as an inmate’s probability of r e-offending with a new felony within three years of release. The IV estimate of the impact of private prison on this outcome is not statistically significant; the 95 percent confidence interval includes effects ranging from −5.7 to 9.1 percent. The literature examines mostly the impact of time served on recidivism and offers a range of possible effects. For example, M ueller-Smith (2017) finds that 90 additional days in prison (the results in the present paper) would translate to a 1 to 1.8 percent increase in quarterly recidivism. By contrast, estimates in Kuziemko (2013), Bhuller et al. (2018), and Zapryanova (2014) suggest a 3 percent reduction in recidivism risk for 90 additional days. (Part of the reason for the conflicting evidence is because the marginal impact of incarceration depends on the incarceration rate itself, as noted in Raphael and Stoll 2014.) The rest of this paper is organized as follows. Section I provides institutional background on private prison contracting and the parole system in Mississippi. Section II provides a model of release policies in private versus public prisons. Section III describes the data. Section IV details the empirical strategy. Section V discusses the results on time served and infractions, a mechanism for the delayed release. Section VI revisits the model and discusses the recidivism results. Section VII provides robustness checks, and Section VIII concludes. I. Institutional Background The correctional facilities in Mississippi include four private prisons and three state prisons, along with several county jails (all public) approved for holding long-term inmates. About 40 percent of all the state’s prison beds are private. The private and public prisons are comparable on most dimensions. For example, they 412 AMERICAN ECONOMIC JOURNAL: ECONOMIC POLICY MAY 2021 offer state-mandated resources including drug rehabilitation programs and are accredited by the American Correctional Association. Private companies exert control over a wide range of management decisions, however, ranging from meal choices to employee contracts. Over the time period studied, private prison guards in Mississippi earned $35,000 (compared to $50,000 for public prison guards) annually and had fewer employee benefits (MDOC 2012). A. Private Prison Contracts States contract with private prison operators to save costs and expand bed capacity.3 When selecting a contractor, the state solicits proposals for private prison beds and these “per diem” beds are required to provide at least a 10 percent cost savings compared to the public prison.4 The per diem payments depend only on the type of bed supplied (i.e., a high-security bed is provided a larger per diem than a medium-security bed), with some additional transfers for inmate health expenses. All private prisons in Mississippi were paid per bed occupied until May 2001. At that time, two private prisons were promised a guaranteed payment for 90 percent of the beds with a p er diem for the remaining beds. The guarantee was inoperative, however, since the prisons typically operated above 90 percent occupancy.5 B. Prison Assignment and Parole Processes Prisons in Mississippi are reserved for inmates who commit felonies and have sentences of at least one year. Once an offender is convicted of a felony, he is transferred to a public prison for classification. I detail the variables related to classification in Section III. The state then places the inmate in a private prison bed if one is available; because private prisons are mandated to be less expensive on a per-prisoner, per-day basis, the state mandates that these beds are filled first. If no private bed is available, the inmate goes to public prison but may later be moved. The data show that 90 percent of inmates who go to private prison remain there until release. Generally, inmates who are moved are done so by the state. Inmaterequested moves are rare because they must pay for the vehicle and security during the transport. Most inmates are released by a parole board prior to completion of their sentences. Factors influencing release are the amount of time already served (at least 25 percent 3 A quote from former Mississippi Department of Corrections Commissioner S.W. Pickett to Mississippi’s Governor and State Legislature in 1996 (the year the state began private prison contracting) illustrates these core goals: “The end of the Fiscal Year 1995 was essentially the middle of the largest expansion program in the Mississippi Department of Corrections’ history. Included in this expansion was the initiation of institutional privatization. This approach will minimize construction expenditure obligated by the state to relieve overcrowding, and must show at least a 10 percent cost savings in operational expenses. Our current expansion program will help ensure that Mississippi has an adequate number of prison beds to house those offenders sentenced to the Agency” (MDOC 1996, 2). 4 The Mississippi Senate Bill #2005 states: “No contract for private incarceration shall be entered into unless the cost of the private operation, including the state’s cost for monitoring the private operators, offers a cost savings of at least 10 percent to the Department of Corrections for at least the same level and quality of service offered by the Department of Corrections.” https://www.peer.ms.gov/Reports/reports/458.html. 5 I show that there is no heterogeneity by contract structure in online Appendix Table A.1. VOL. 13 NO. 2 MUKHERJEE: IMPACTS OF PRIVATE PRISON CONTRACTING 413 of the original sentence is required), severity of the main offense, community support or opposition to the inmate’s release, prior criminal records, crimes committed while incarcerated, behavior in prison, and participation in rehabilitative programs. Importantly, whether the inmate spent time in private or public prison is not a factor, and the parole process is the same for inmates regardless of their prison placement. Parole in Mississippi requires a unanimous vote from an appointed board, which always consists of five state employees who serve on a rotating basis.6 II. A Model of Prisoner Release Decisions I develop a model of prisoner release decisions to illustrate the distortion that can result from private contracting. The model borrows elements from Kuziemko (2013), which studied the costs and benefits of discretionary parole regimes. The setup also incorporates an important aspect of the incomplete contracting model in Hart, Shleifer, and Vishny (1997), which argued that private prison operators may undertake excessive cost cutting because they ignore the impact of these cost reductions on noncontractible quality. Both of these models, and hence the present model, also draw elements from Shavell (1987), which provides a framework for studying optimal prisoner release policies. A. Baseline Model without Private Contracting I posit that a state chooses an optimal release policy based on the trade-off between incarceration costs and the cost of severity-weighted recidivism risk. As in Kuziemko (2013), incarcerating a prisoner for an additional day costs the government some amount, but society benefits from a reduction in crime due to both an incapacitation effect (i.e., the prisoner cannot commit crime while incarcerated, and may even “age” out of crime while incarcerated) and a specific deterrence effect (i.e., a prisoner’s recidivism risk declines with time since the original offense as a result of punishment).7 Let prisoner ipose a severity-weighted cost of recidivism rithat is a function of his individual-specific risk, R i, and a parameter βi > 0that captures the rate at which his recidivism risk decreases with the number of days since his offense: gov, and the prisri(t) = Ri − βi t. If the daily cost of incarceration to the state is C oner time served is si, the state’s cost minimization problem is given by Recidivism ccsts Incarceration costs ∞ ⏞ (1) min C gov si + si ri(t)𝑑t . si ∫ 6 The full set of official parole guidelines for Mississippi is provided in online Appendix C. Prior research indicates that the incapacitation effect can be large: for example, Barbarino and Mastrobuoni (2014) estimates that the elasticity of total crime to incapacitation is between −17 and −30 percent, and related work finds that increases in time served can reduce subsequent recidivism (Maurin and Ouss 2009). Buonanno and Raphael (2013) also finds strong incapacitation effects using evidence from a large and collective Italian pardon. Owens (2009) finds that the incapacitation effect can be strong enough to justify longer sentences for at least juvenile offenders. There may also be a general deterrence effect as in Becker (1968), by which criminals decide to engage in less crime because of an increase in the expected incarceration length, but the empirical evidence for this channel is mixed. 7 414 AMERICAN ECONOMIC JOURNAL: ECONOMIC POLICY MAY 2021 In this cost minimization problem, the first-order condition is C gov − ri(s ⁎i ) = 0, ⁎ and the optimal policy for the state is to release prisoner i at s i , the point at which gov, the marginal the prisoner’s expected risk r i, or marginal social benefit, equals C social cost. Rewriting and rearranging this equality in terms of the components of r i yields Ri − C gov (2) s ⁎i = _ . βi Accordingly, the optimal time served in prison is an increasing function of the pris i. oner’s initial risk R iand a decreasing function of the rehabilitation rate β Figure 2 shows the recidivism cost and daily incarceration curves. The optimal number of days served, s ⁎i , has a legislative upper bound at the court-ordered sentence. In this setup, the state pays C gov s ⁎i in incarceration costs in exchange for ⁎ social benefit ∫0s i ri(t) dtfrom incapacitation. At this optimum, the state still faces an t) dtin s everity-weighted potential recidivism resulting from expected cost of ∫ s∞ ⁎i ri( prisoner i’s release. It is important to note that even without the private prison’s profit motive, there is a likely mismatch between the objective functions of private and public prisons that produce distortions in inmate outcomes. This is because the counterfactual to private prison is not a social planner, but a public prison operated by fallible humans with their own inefficient objective functions. For example, public prisons and their employees may also seek to maximize the number of beds filled each day as inmate populations are a primary determinant of prison budgets and thereby the basis of all prison employees’ job security. To the extent that profit motives and such other considerations are at play, both private and public prisons will keep inmates for a different amount of time compared to a social planner. B. Distortion of the Release Decision by the Private Operator As established in Section I, private prison operators must provide cost savings to be hired. Let the private operator charge a per diem P < C govfor each day that a bed is occupied. Friction arises because the private contractor faces Pas its marginal revenue; it does not internalize the social benefit of minimizing recidivism risk. Since P is the negotiated payment made by the state to the private operator for each bed occupied, the private operator incurs cost C priv < P, else it would not generate profit. The private operator’s marginal cost, C priv, need not be constant, although it is useful to think of C privas a fixed marginal cost for the first s ⁎i number of days, i.e., the case with no distortion in days served. When a private operator holds a prisoner beyond the number of days expected by the state, it must exert effort. This effort could take the form of distributing excessive infractions that delay an inmate’s release. This effort could also take the form of broader cost reductions that unintentionally affect infractions or delay release, such as hiring fewer guards than required or shirking on required prison conditions such as poor heating or cooling; these are examples of complaints lodged against VOL. 13 NO. 2 R MUKHERJEE: IMPACTS OF PRIVATE PRISON CONTRACTING Recidivism risk = 415 R − βt Cost Cpriv Private prison’s marginal cost of incarceration Cgov State’s marginal cost of incarceration P Per diem payment to private prison Expected cost saving s* + dˆ s* Days since offense (t) Distortion Figure 2. Theoretical Framework Notes: The figure shows the distortion in release policy arising from differences in objectives of the state and private operators. The state minimizes severity-weighted recidivism subject to cost C gov, but the private operator maximizes profit given its p er diem payment Pand marginal cost C priv. The parameter Rrepresents prisoner i ’s recidivism risk, and βis the rate at which recidivism risk declines with days since offense. The state chooses to hold inmates for s ⁎ days and the private prison chooses to hold inmates for an additional dˆ days. the private prison operators in Mississippi (MDOC 2012). Formally, let the private prison’s daily cost of incarcerating an inmate be κ if di ≤ 0 (3) C priv = {κ + Md 2i if di > 0, is a scalar capturing the cost of where diis the amount of distortion (in days) and M distorting an inmate’s length of stay. This distortion allows the private prison operator to realize profit on each prisoner iin the amount (P − C priv) (s ⁎i + di) − Md 2i . Figure 2 illustrates how equation (3) affects the equilibrium outcomes in this framework. The optimal level of distortion based on the first-order condition is dˆi = (P − C priv) /(2M). As expected, this quantity decreases with the cost of distortion. The distortion in the number of prisoner days served is positive as long as the marginal revenue, P, exceeds the marginal cost to the private operator, C priv. C. Assessing Welfare Distortion of the release decision has direct implications regarding the fairness of the criminal justice system. Conditional on all available information, the state, acting as the social planner, does not seek differential punishment of inmates by assigning them to private prison. Therefore, the primary welfare loss from release policy distortion is unfair treatment, but society may also care about the eroded 416 AMERICAN ECONOMIC JOURNAL: ECONOMIC POLICY MAY 2021 cost savings—both in direct prison costs and future crime—and the prisoner’s value ˆi P in of freedom. Regarding the incarceration costs alone, the state pays an extra d incarceration costs for each prisoner i, and loses all the expected cost savings from gov ⁎ ˆi P > private contracting if d (C − P) s i . If the cost savings offered by private operators is (1 − γ) percent per occupied bed (by Mississippi state law, (1 − γ) ≥ 10 percent), then the inequality becomes d ˆi γ C gov > (1 − γ)C gov s ⁎i , which simpli⁎ ˆ ˆ fies to di / (di + s i ) > 1 − γ. Thus, any distortion in time served directly erodes the cost savings expected from private contracting.8 III. Mississippi Prisoner Data and Sample Definition The empirical analysis uses Mississippi inmates sentenced to prison between May 1, 1996 and July 31, 2013.9 Administrative records were obtained directly from the Mississippi Department of Corrections (MDOC), which manages an inmate data file that covers every inmate who served time in a state prison since 1981. Some variables, such as the dates of inmate transfers between facilities, are available only from May 1, 1996. The data contain standard criminal justice information on each offender’s demographics, current offense, offense history, and infractions while incarcerated. A special feature of this data is information on the movement of inmates between facilities over the course of their sentences, which permits measurement of whether an inmate ever served time in a private facility. This transfer information is difficult to obtain (and not available for current or recently released inmates) because the MDOC uses protected algorithms to move inmates between beds in the same facility, or between facilities, so that they do not develop excessive familiarity with guards or other inmates. Demographic variables available in the MDOC dataset include the offender’s age and gender, along with s elf-reported inmate information on race, education level, and marital status. While inmate hometown is not available, the MDOC records the county of conviction for each inmate. The classification data include information on the offender’s custody designation level (A to D, where A is minimum custody and D is maximum custody) as well as two medical designations that determine the extent to which the inmate will work while in prison: medical class, which focuses on physical health (and ranges from values A, excellent physical condition, to E, poor physical condition and severely limited physical capacity or stamina), and level of care, which focuses on mental health (and ranges from A, no mental health problems, to E, inpatient health treatment). I use the initial classification variables in all analyses. The MDOC Inmate Handbook (2011, 7) states that “all privileges, to include level of supervision within and outside of the institution, access to programs, activities, jobs, canteen, visits, and telephone, are based on the inmate’s custody [designation] level.” Additionally, the Handbook (p. 8) states that “the classification system is also used to determine which facility inmates will be housed in and places them in housing units which are appropriate for their custody assignment.” The 8 An extension to this model that allows the state to reoptimize release policies based on the cost savings offered by private contracting is in online Appendix B. 9 The data and analysis files are available in Mukherjee (2020). VOL. 13 NO. 2 MUKHERJEE: IMPACTS OF PRIVATE PRISON CONTRACTING 417 c lassification variables are determined by a classification hearing officer (always in a public prison) and are based on a variety of factors (e.g., a personal interview, expert recommendations, and prior escape history, in addition to factors such as the inmate’s age, education, and offense(s) committed), as well as medical and psychological evaluations of the inmate.10 The data also include information on the crime(s) committed, court-ordered sentence length, and the number of days served while the case was under trial. Using this information, I construct two key variables of interest: whether an inmate ever served time in a private prison, and whether he recidivated with a new felony within three years of release. Note that I only observe felonies in Mississippi, so prior or future crimes may be underestimated. This is a common censoring problem, but most offenders have convictions in only one state (Durose, Cooper, and Snyder 2014). I also use the available information to generate controls for criminal history. The primary analysis sample begins with 40,195 felonies committed by 34,571 adult male inmates between May 1, 1996 and July 31, 2004. Sentences that occur after July 31, 2004 were omitted to allow for the observation of time served and three-year recidivism for the analysis sample. Between August 1, 2004 and July 31, 2013, I observe an additional 39,059 sentences for 34,620 adult male inmates to examine recidivism. The sample is then restricted to bookings with sentence length between one and six years, bringing the number of inmate sentences to 32,614 (2,607 are dropped because of sentence lengths less than one year; 6,793 are dropped because of sentence lengths greater than six years). The sentences with less than one year are dropped because one year is the minimum sentence required to be eligible for prison (versus jail or county corrections) placement. Additionally, these sentences are anomalies because the MDOC states that all felonies must carry a minimum sentence of one year. In rare cases, however, the judge may award u p-front meritorious time to reduce the sentence. The sentences greater than six years are dropped to enable the observation of time served and three-year recidivism. Next, the sample is restricted to inmates who served at least 25 percent of their sentences for the given booking as this was the s tate-mandated minimum over the time period studied. This step removes 5,748 inmates. Finally, I drop 273 bookings due to missing covariates (239 for missing county and 34 for missing level of care). Together, these restrictions result in the primary sample of 26,593 bookings used in the analysis. Note that the robustness checks in Section VII present estimates of the main coefficients by relaxing some of the sampling restrictions. A. Summary Statistics Table 1 shows summary statistics for the sample of inmates by whether they served time in private prison. The sample consists of 26,593 inmates, about 19 percent of whom went to private prison over the time period examined. The descriptive statistics in Table 1 foreshadow the main results. As mentioned in the 10 I refer the reader to the MDOC’s Inmate Handbook (MDOC 2011) for further details on the classification process and the c lassification-related variables. 418 AMERICAN ECONOMIC JOURNAL: ECONOMIC POLICY MAY 2021 Table 1—Summary Statistics Sample: All (1) Public (2) Private (3) 1.98 0.71 0.25 0.24 1.82 0.70 0.25 0.18 2.65 0.73 0.26 0.47 0.68 0.31 0.57 0.54 0.67 0.32 0.55 0.53 0.71 0.28 0.67 0.56 0.04 0.18 0.20 0.14 0.09 0.06 0.04 0.10 0.16 0.03 0.17 0.22 0.13 0.10 0.06 0.03 0.10 0.16 0.07 0.21 0.14 0.15 0.03 0.04 0.09 0.10 0.18 2.93 1.17 0.27 2.75 1.15 0.27 3.68 1.23 0.27 Custody designation ( proportions) A B C D Unclassified 0.34 0.54 0.02 0.00 0.10 0.37 0.50 0.01 0.00 0.12 0.25 0.70 0.04 0.00 0.01 Medical class ( proportions) A B C D E 0.83 0.05 0.09 0.01 0.02 0.84 0.05 0.09 0.01 0.01 0.80 0.06 0.09 0.02 0.03 Level of care ( proportions) A B C D E 0.09 0.70 0.12 0.07 0.02 0.10 0.68 0.12 0.07 0.02 0.01 0.78 0.12 0.08 0.01 0.24 0.22 0.36 26,593 21,449 5,144 Outcomes Years served Fraction of sentence served Recidivism (36-month) Any infraction?a Demographics Black Age/100 Single Education < HS Offenses ( proportions) Aggravated assault Burglary Drug possession Drug selling Felony DUI Fraud Robbery Theft Other Offenses Sentence length Number of offenses Prior incarcerations (5 years) Instrument CapacityShock (/1,000) Observations Notes: Table shows summary statistics by whether the inmate ever went to private prison during his sentence. Each observation is an inmate-sentence between May 1, 1996 and July 31, 2004. The sample consists of male inmates with original sentences of 1 to 6 years that serve at least 25 percent of their sentences; see Section III in the text for further details. a Infractions data are available post-2000. VOL. 13 NO. 2 MUKHERJEE: IMPACTS OF PRIVATE PRISON CONTRACTING 419 introduction, private prison inmates serve a greater fraction of their sentences (73 versus 70 percent); this difference is statistically significant. Recidivism rates are similar for the two groups (25 versus 26 percent), and they mirror the national average of 24 percent over this time period (Langan and Levin 2013). The higher average sentence length among privately incarcerated inmates reflects both the state’s preferences in prison assignment and that inmates with longer sentences experience more private prison openings and bed expansions.11 Table 1 also reveals a considerable degree of difference along observed characteristics between inmates in public versus private prison. Echoing the anecdotal evidence of Spivak and Sharp (2008), I find that inmates in private prison are more likely to be Black (71 versus 67 percent), single (67 versus 55 percent), young (mean age of 28 versus 32), and less educated (56 versus 53 percent do not have a high school degree). Due to the detailed data available, much of the selection of inmates into private prison is observed and can be accounted for in the empirical analysis. The differences in these observables—all of which are statistically significant—raise concerns about selection on unobservables, which the IV analysis will address. The other variables shown in Table 1 relate to the breakdown of inmate offenses by private prison assignment. There are some differences by offense category in the types of inmates assigned to private prison; for example, fewer have drug possession and felony DUI charges, and more have robbery and assault charges. For each sentence, the average inmate in private prison had 1.23 offenses, versus 1.15 for those in public prison. The number of prior incarcerations (felonies) in the past five years is 0.27 for both groups. Table 1 also shows the distribution of the classification variables and the value of the instrument across these inmate groups. We observe that in terms of custody designation, a higher proportion of inmates in private prison (70 versus 50 percent) belong to the medium designation of B, with fewer being in the lowest designation of A (25 versus 37 percent). This difference helps explain the apparent negative selection of private prison inmates, for example, by sentence length and offense type. Both custody designations of C and D are rare, and about 12 percent of inmates in public prison remain unclassified in the data (unclassified inmates receive a custody designation of D until there is a change). In terms of medical class, slightly fewer private prison inmates belong to the lowest class of A (80 versus 84 percent), but all class levels are represented in both types of prison. Similarly, for the level of care, all levels are represented in both types of prison, but private prison inmates are more likely to have a level of B, indicating that some mental health interventions may be needed, versus a level of A, indicating no mental health concerns. It is worth noting that even though Mississippi has the fourth highest incarceration rate in the country (as of 2004), the state’s inmates are generally representative of state prison inmates in the United States based on nationwide summary statistics provided in Harrison and Beck (2005). The average sentence length and demographic characteristics of inmates are similar to other states, especially in the 11 Panel A of Figure A.1 in the online Appendix illustrates that inmates with longer sentence lengths are more likely to go to private prison. Panel B of the same figure shows that the instrument, explained in Section IVB, is similarly higher for inmates with greater sentence lengths. 420 AMERICAN ECONOMIC JOURNAL: ECONOMIC POLICY MAY 2021 southern United States. The percent of inmates in private prison is also not atypical: the state prison systems in Vermont, Oklahoma, Tennessee, and Idaho also held about 20 percent of inmates in private prison over this time period. IV. Empirical Strategy I begin with OLS analysis to show association between serving any time in private prison on inmate outcomes, and then introduce an IV strategy to deal with potential n onrandom selection into private prison. A. OLS Analysis I estimate the impact of private prison on inmate outcome Yi using (4) Yi = βPrivatei + θXi + ϵi, where P rivateiis a binary variable indicating whether the inmate served any time in private prison.12 I estimate two outcomes for time served: the number of days served and the fraction of sentence served. The vector X i captures demographic, offense (including criminal history), classification-related variables, admission time, and geographic information. Demographic information includes prisoner age at admission date, race, marital status, and education level; these variables are included because prior literature indicates they may be correlated with the outcomes studied. (For example, Lochner and Moretti 2004 finds that education reduces the probabilities of both incarceration and arrest.) For o ffense-related information, the controls include sentence length incorporated as dummies for each rounded sentence year, offense type for up to three offenses related to each inmate-sentence (included separately as primary, second, and third offenses), and criminal history.13 This latter set of variables includes controls for the number of felonies in the five years prior to the admission date, along with controls for the types of prior offenses when relevant. Classification variables include dummies for the inmate’s custody designation, medical class, and level of care. Admission time trends are linear (calculated as days since January 1, 1990) and interacted with the sentence length dummies to control for policies that may be changing over time. Finally, the geographic information contains dummies for the county of conviction related to the inmate-sentence. B. Instrumental Variable Analysis The IV approach uses capacity shocks in private prisons to generate variation in prison assignment. The openings of private prisons over the timespan studied 12 Online Appendix Figure A.2 shows the extent of treatment, i.e., the distributions of time served in private prison. 13 Note that 92.15 percent of sentences are within 30 days of an exact round year. The rounding process is standard: e.g., if an inmate’s sentence length is 2.49 years, his rounded sentence length is 2 years; if the sentence length is 2.51 years, it is rounded to 3 years. VOL. 13 NO. 2 MUKHERJEE: IMPACTS OF PRIVATE PRISON CONTRACTING 421 p rovide most of the variation, and similar instruments have been used in prior work. For example, Atkin (2016) uses the openings of manufacturing plants in Mexico to instrument for the returns to education in the study of high school dropout rates. In a more closely related setting, Chirakijja (2018) uses prison openings over a long timespan to examine their impact on local labor market conditions. The capacity shocks in Figure 1 are converted into an instrument by calculating the net number of private bed openings over the inmate’s assigned sentence. There is a small adjustment made to ensure that the capacity shock could have affected the inmate’s probability of going to private prison: it had to occur at least 90 days after his admission and at least 90 days before the sentence end date. The reason for the first cutoff is because the MDOC does not move inmates to private prison until inmate classification (which takes up to 45 days) and orientation (which could take up to 90 days) are complete. Consistent with this policy, I do not observe any inmate transfers to private prison until at least 90 days have been served (the minimum is 109 days). The MDOC also has a policy of not transferring inmates to a new prison if they have less than 90 days left to serve, which I observe to be true in the data. The instrumental variable CapacityShocki = ∑Jj=1 capacityshockij, where Cj if ai ≤ tj − 90 and vi ≥ tj + 90 (5) capacityshockij = {0 otherwise. The variable a iis the prisoner’s admission date, viis his court-ordered release date (i.e., the prisoner’s admission date plus his assigned sentence), tjis the date of the private prison bed capacity shock, and Cjis the number of private prison beds opening or closing. Estimating the first-stage equation requires care because the endogenous variable is binary. I adopt the two-stage least squares (2SLS) with probit correction method outlined in Wooldridge (2002) and discussed in Angrist and Krueger (2001).14 This method uses a probit model to estimate the probability of treatment, and the predicted probabilities are used as instruments in a standard 2SLS framework. Intuitively, the method works because any nonlinear function of an instrument is also a valid instrument. The key advantage is efficiency; while both the traditional 2SLS and 2SLS with probit correction methods yield estimates that are asymptotically unbiased, the latter method produces estimates more tightly centered around the true coefficient when the first stage is better approximated by a nonlinear function. Additional advantages of the procedure are that it is robust to misspecification of the probit model, and the standard errors are estimated in the same manner as in a traditional 2SLS framework. A disadvantage is potential identification from nonlinearity, but this is overcome by the use of a valid instrument—in this setting, C apacityShock— in estimating the “0th” stage probit model from which the fitted values are obtained as instruments.15 14 Prior studies using this 2SLS with probit correction method in a variety of settings include Dubin and McFadden (1984) (one of the first applications); Norton and Staiger (1994); Cameron et al. (1988); Adams, Almeida, and Ferreira (2009); Berger and Roman (2017); and Allen, Chandrasekaran, and Basuroy (2018). 15 In online Appendix Section D, I show the probit versus linear model fits of the first stage, and demonstrate via Monte Carlo simulation the relative efficiency of the 2SLS with probit correction method in the presence of 422 AMERICAN ECONOMIC JOURNAL: ECONOMIC POLICY MAY 2021 The probit model is given by P i = Φ(γ CapacityShocki + θXi), where Xi is the same as in the OLS specification. The predicted probabilities Pˆ are used as instruments in the first-stage equation, which is given by (6) Privatei = α + βPˆ i + δXi + ηi. The second-stage equation is given by + βIVˆ rivatei + δIV Xi + ϵi. P (7) Yi = αIV In estimation, the standard errors are clustered by admission m onth-year and sentence length (rounded to the nearest year) to account for parole guidelines over time that may have affected inmates differently depending on their sentence length. For example, Mississippi overhauled its parole guidelines by sentence length in 1995, before the start of the sample analyzed in this paper.16 Identification in the IV analysis requires three assumptions. First, CapacityShock must be a good predictor of prison assignment, and I show this in the regression analysis. Figure 3 also shows a visual representation of this first-stage relationship. Second, there should be instrument monotonicity. Following Dobbie, Goldin, and Yang (2018), I demonstrate this by showing that the first-stage relationships are strongly statistically significant and stable across subsegments of the inmate population by race, marital status, education, and age.17 Note that monotonicity in this setting means that the capacity shock can only increase an inmate’s likelihood of being assigned to private prison—in other words, no inmate can become more likely to go to public prison as a result of the increase private prison bed capacity (these would be defiers). Defined in this way, the estimation yields a local average treatment effect (LATE) interpretation, where the causal effect of private prison is estimated for the compliers who are assigned to private prison because of the capacity shock, but who would otherwise have been assigned to public prison in the absence of the capacity shock. Third, the exclusion restriction should be satisfied—the instrument should be otherwise unrelated to prisoner outcomes. In Section VII, I show a test of instrument exogeneity following Altonji, Elder, and Taber (2005). I also show that the instrument is not strongly correlated with any variable used in the analysis.18 While one can never “prove” the exclusion restriction, the sharp changes in private prison capacity are plausibly unrelated to patterns in the variables studied. This is not surprising given research showing that judges do not adjust sentences even in light varying levels of treatment and endogeneity. The results for all key outcomes using a traditional 2SLS method are in online Appendix Table A.2. 16 The results that follow are robust to clustering by admission month-year, as shown in online Appendix Table A.3. Their direction and statistical significance are also robust to more flexible time trends in the form of year fixed effects interacted with sentence length dummies. These results are in online Appendix Table A.4. 17 These results are in online Appendix Table A.5. The implied F-statistic of the instrument in each subsample exceeds 300. 18 These results are in online Appendix Table A.6. VOL. 13 NO. 2 MUKHERJEE: IMPACTS OF PRIVATE PRISON CONTRACTING 423 Coefficient on private 1 0.5 0 −0.5 −500 0 500 1,000 1,500 2,000 2,500 Instrument (CapacityShock) Figure 3. Private Prison Treatment by Instrument Value Notes: The figure shows coefficients from a single probit regression on categories of the C apacityShock instrument (the reference category is CapacityShock = −1,000 beds). The dependent variable in the regression is Private, i.e., whether the inmate ever went to private prison, and the other covariates include the controls in Table 2. of policies such as truth-in-sentencing that increase time served more sharply and directly (Owens 2011).19 V. Results A. Time Served Table 2 presents results on the effect of private prison assignment on inmate time served. Columns 1 and 3 report the saturated OLS regression estimates on the number of days and fraction of sentence served, respectively, with all the controls discussed in Section IV. These results show that private prison inmates serve 85 more days, alternatively estimated as 6.2 percent of their sentences, than inmates in public prison. These estimates are consistent with each other, because 6.2 percent translates to about 83 additional days in prison based on the average sentence length for inmates in private prison.20 The other covariates shown in Table 2 have the expected sign across specifications: for example, each prior incarceration increases the fraction of sentence served by about 1 percent. Even after controlling for all covariates, I find that older, Black, and 19 Note that Dippel and Poyker (2019) finds that private prisons appear to increase sentence length for all inmates through a channel in which the state reoptimizes release policies as discussed in online Appendix Section B. The time served outcome in this analysis controls flexibly for sentence length and accounts for this possibility. 20 Table 1 shows that the average sentence length for inmates in private prison is 3.68 years. Thus, a 6.2 percent increase in fraction of sentence served translates to 3.68 × 365 × 0.062 = 83.28additional days. 424 AMERICAN ECONOMIC JOURNAL: ECONOMIC POLICY MAY 2021 Table 2—Impact of Private Prison on Time Served Dependent variable: Private Prior incarcerations Age/100 Black Single Education < HS CapacityShock (/1,000) Days served OLS (1) 85.250 (4.375) 11.907 (6.756) 198.121 (17.969) 16.585 (3.377) 29.741 (3.015) −4.823 (2.796) IV (2) 89.627 (26.414) 11.921 (6.732) 199.981 (19.129) 16.601 (3.355) 29.612 (3.119) −4.852 (2.780) Fraction served OLS (3) 0.062 (0.003) 0.009 (0.005) 0.170 (0.014) 0.015 (0.003) 0.025 (0.002) −0.001 (0.002) IV (4) 0.048 (0.019) 0.009 (0.005) 0.164 (0.015) 0.014 (0.003) 0.025 (0.002) −0.001 (0.002) Instrument (predicted probit) Dependent variable mean 2 R F-statistic Observations Offense variables Classification Time trends County fixed effects 722.7 0.737 — 26,593 Yes Yes Yes Yes 722.7 0.737 — 26,593 Yes Yes Yes Yes 0.71 0.280 — 26,593 Yes Yes Yes Yes 0.71 0.279 — 26,593 Yes Yes Yes Yes Private Private Probit eqn. (5) First stage (6) −0.002 (0.013) −0.456 (0.029) −0.007 (0.006) 0.023 (0.005) 0.008 (0.005) 0.034 (0.003) −0.002 (0.013) 0.059 (0.029) 0.002 (0.005) −0.005 (0.004) −0.001 (0.005) 0.19 — — 26,593 Yes Yes Yes Yes 0.19 0.177 887 26,593 Yes Yes Yes Yes 1.155 (0.039) Notes: The table shows regression estimates of the impact of private prison (Private) on inmate outcomes. “Fraction served” is the fraction of the inmate’s sentenced days that were served. “Private” is a binary variable for whether the inmate served any time in a private prison. Offense variables include sentence length dummies (rounded to the nearest year) and dummies for offense type for up to three offenses related to each inmate-sentence. They also include controls for the number of prior incarcerations in the five years before the admission date, along with controls for the offense type(s) of prior incarceration(s). Classification variables include the custody designation level, medical class, and level of care. Time trends include a linear time trend and its interaction with sentence length dummies. County fixed effects are for the county of conviction. Column 5 shows the mean marginal effects of the “0th” stage probit equation; the instrument CapacityShockhas a t-statistic of 11.22. Column 6 shows the first-stage estimates using the predicted probit probabilities from column 5 as an instrument. Standard errors in parentheses are robust and clustered by admission month-year and sentence length. single inmates each serve significantly larger fractions of their sentences. For example, column 3 shows that Black and single inmates serve 1.5 and 2.5 percent larger fractions of their sentences, respectively. Some of these differences may be due to in-prison behavior, which I explore in the next section. Figure 4 provides a visual of these results: it plots the time until release for inmates in private versus public prison. We observe that inmates in private prison have a lower likelihood of release (as estimated by Kaplan-Meier failure estimates) at every level of time served. There are spikes in the probability of release for inmates in both prison types at exact years, which are both common sentence lengths and common levels of time served.21 21 For improved comparison of inmates in public and private prison, this figure uses the matched and restricted sample of inmates (described in Section VIIC) eligible for private prison assignment during the capacity shocks. VOL. 13 NO. 2 425 MUKHERJEE: IMPACTS OF PRIVATE PRISON CONTRACTING 1 Proportion released 0.75 0.5 0.25 0 0 1 2 3 4 5 6 Time served (years) Figure 4. Proportion of Inmates Released by Time Served and Prison Type Notes: The figure shows the K aplan-Meier failure estimates of time (years) until release for the sample of inmates eligible for private prison assignment during the capacity shocks; this is the restricted sample used in the matching analysis described in Section VIIC. The solid line shows the proportion of private prison inmates released at each level of time served. The dashed line shows the analog for public prison inmates. The IV estimates are in columns 2 and 4 of Table 2. The estimates indicate that private prison inmates serve 90 additional days, alternatively estimated as an additional 4.8 percent in fraction of sentence served. The standard errors on the IV estimates contain the OLS estimates for both measures of time served. All covariates have similar magnitudes and signs as in the OLS regressions. Column 5 reports the marginal effects of the “0th” stage probit regressions discussed in Section IV. The coefficient of 0.034 on the instrument in column 5 indicates a 3.4 percent higher likelihood of private prison assignment for every 1,000 net private prison beds that opened over the inmate’s sentence. The CapacityShock instrument is strongly statistically significant; its t-statistic is 11.22. In implementing the two-step procedure, I use the predicted probit instrument shown in column 6; the coefficient of 1.155 implies that a 1 percentage point change in the inmate’s predicted probability leads to a change in likelihood that he goes to private prison by 1.155 percentage points. The t-statistic on this instrument is 29.62, translating to an F-statistic of 877.06 as shown in column 6. B. Infractions as a Mechanism for Delayed Release Having established that private prison assignment increases time served, I explore a mechanism that explains these results. Infractions are prison conduct violations given to inmates for behaviors ranging from disobeying a guard to possessing 426 AMERICAN ECONOMIC JOURNAL: ECONOMIC POLICY MAY 2021 Table 3—Infractions by Inmate Characteristics Public (1) Private (2) Demographics Black White Age 18–24 Age 25–34 Age 35–49 Age 50+ 0.19 0.16 0.22 0.19 0.15 0.11 0.51 0.38 0.54 0.46 0.33 0.22 Offenses Aggravated assault Burglary Drug possession Drug selling Felony DUI Fraud Robbery Theft Other 0.20 0.25 0.14 0.22 0.09 0.16 0.30 0.18 0.19 0.45 0.53 0.40 0.51 0.16 0.33 0.60 0.47 0.46 Sentence length 1 2 3 4 5 6 0.05 0.11 0.18 0.31 0.39 0.43 0.14 0.24 0.37 0.55 0.68 0.76 Overall 0.18 0.46 Notes: The table shows the proportion of inmates receiving an infraction by prison type and selected characteristics. For example, 19 percent of Black inmates received an infraction in public prison versus 51 percent in private prison. All differences are statistically significant ( p < 0.05). The sample contains 12,551 inmates in public prison and 3,203 in private prison. The sample includes inmates with admission dates between January 1, 2000 and July 31, 2004, because the infractions data are available post-2000. c ontraband.22 They are a critical component of release decisions by the parole board. Infractions are imperfect measures of behavior, however, because they can be affected by factors such as harsher prison conditions in private prisons—a problem relevant in Mississippi (Williams 2016). On the other hand, private prisons may have a better technology for monitoring infractions, or may be more likely to report them due to contract renewal incentives. A difference in infraction rates between inmates in public and private prison could also stem from shirking by public prison employees who underreport such violations. Table 3 shows summary statistics on infractions given to inmates by whether they were in private prison. They provide a leading explanation for why inmates serve more time in private prison: 46 percent of private prison inmates receive at least one infraction, versus 18 percent for those in public prison. Private prison 22 A new crime such as an assault on a fellow inmate is also processed as a crime with a sentence to be served concurrently or consecutively, and is accounted for in the calculation of sentence length. VOL. 13 NO. 2 427 MUKHERJEE: IMPACTS OF PRIVATE PRISON CONTRACTING Table 4—Impact of Private Prison on Infractions Dependent variable: Any infraction? Private Prior incarcerations Age/100 Black Single Education < HS Dependent variable mean R2 Observations Offense variables Classification Time trends County fixed effects Probit (1) OLS (2) 0.090 (0.006) 0.040 (0.012) −0.431 (0.036) 0.023 (0.007) 0.030 (0.006) 0.009 (0.006) 0.142 (0.010) 0.051 (0.015) −0.415 (0.040) 0.027 (0.007) 0.032 (0.006) 0.016 (0.006) 0.24 — 15,754 Yes Yes Yes Yes 0.24 0.317 15,754 Yes Yes Yes Yes Notes: The table shows regression estimates of the impact of private prison (Private) on whether the inmate had any infraction during his sentence. Sample includes inmates with admission dates between January 1, 2000 and July 31, 2004 since the infractions data are available p ost-2000. See notes for Table 2 for a list of the detailed controls. Mean marginal effects are reported for the probit model in column 1. Standard errors in parentheses are robust and clustered by admission month-year and sentence length. inmates in every demographic, offense, and sentence length category accumulate more infractions. Table 4 shows the difference in the probability of receiving an infraction by whether a prisoner is assigned to private prison after controlling for the available covariates, using both probit and linear probability specifications. (The IV approach is unavailable for the infractions analysis since these data are available post-2000, which is after the period in which most of the private prison bed capacity shocks occurred.) The estimating equation used to generate column 1 is a probit model with Infractionsias a binary variable indicating whether the prisoner received any infractions over the course of his sentence: Infractionsi = Φ(β Privatei + δXi).The estimating equation for column 2 is the analogous linear probability model: Infractions i = βPrivatei + δXi + ϵi. The probit and linear probability model estimates suggest that a private prison inmate is 9 or 14.2 percent more likely to obtain an infraction over the course of his sentence, respectively. These effects are high given that the baseline rates of any infraction. In both specifications, inmates with more prior incarcerations and less education are more likely to obtain an infraction. Inmates who are young, Black, or single all also show higher infraction rates. 428 AMERICAN ECONOMIC JOURNAL: ECONOMIC POLICY MAY 2021 Panel A R Cost Recidivism risk = R − βt Cpriv Private prison’s marginal cost of incarceration Cgov State’s marginal cost of incarceration Expected recidivism benefit from extra days Per diem payment to private prison Expected loss from extra days P s* + dˆ s* Distortion Days since offense (t) Panel B R Recidivism risk = R − β govt Recidivism risk = R − β privt Cpriv Private prison’s marginal cost of incarceration Cgov State’s marginal cost of incarceration P Per diem payment to private prison Cost s* s* + dˆ Distortion Days since offense (t) Figure 5. Theoretical Framework with Recidivism Notes: Figure illustrates hypotheses related to the effect of private prison exposure on inmate recidivism. See Figure 2 notes for notation. Panel A shows that recidivism risk is expected to decrease for private prison inmates due to the additional time served. Panel B shows that this effect could be undone if private prisons alter the rate at which recidivism risk falls with time served, i.e., if βis indexed by whether the inmate is in private prison. VI. Recidivism Analysis A. Assessing Welfare Impacts Considering Recidivism The results so far establish that private prison inmates serve more time. If these additional days decrease recidivism, then private prisons may not harm social welfare. Panel A of Figure 5 depicts this trade-off: distortions of increased time served are socially beneficial if they are sufficiently small and the recidivism risk curve is sufficiently flat. VOL. 13 NO. 2 MUKHERJEE: IMPACTS OF PRIVATE PRISON CONTRACTING 429 To consider the welfare impacts of private contracting, we calculate the social costs of incarceration with and without it. Without private contracting, the social costs of incarcerating an individual are (C gov − Ri)s ⁎i + , where Δ = Ri t − (βi/2) t 2 t=∞. With private contracting, (βi/2)(s ⁎i ) 2 + Δ ˆi , the amount of distortion, and P, the per diem payment: these costs depend on d 2 ⁎ ˆ (P − Ri)(s i + di ) + (βi/2)(s ⁎i + dˆi ) + Δ. A few observations are in order. First, absent distortion (i.e., dˆi = 0), social welfare is guaranteed to improve under private contracting by ( C gov − P)s ⁎i due to the cost savings. Second, if recidivism risk does not respond to time elapsed since offense (i.e., βi = 0), the increase in social welfare from private prison contracting is ( C gov − P) s ⁎i − (P − Ri)dˆi . In this case, social welfare is an increasing function of distortions, assuming that the criminal justice system incarcerates individuals with recidivism risk exceeding the marginal cost of incarceration. Based on these assumptions, private prisons increase social welfare by holding inmates longer if | ___________________________ gov 2 (C gov − P) 2 + 2(C gov − P)(Ri − C gov) ( C − P) + √ ˆi ≤ ______________________________________________ . (8) d βi The intuition from equation (8) is as follows. If the distortion from private prison contracting is sufficiently small, then social welfare improves. If β i = 0, the condition requires only that d ˆi < ∞, which is always the case since the private prison cannot hold a prisoner beyond his court-ordered sentence length. If private prisons offer no cost saving, i.e., if C gov = P, then equation (8) shows that social welfare is unchanged only if there is no distortion. Two testable implications emerge from this framework given the time served results. First, if recidivism risk falls with time since offense, and if private prisons have no other impact on recidivism risk, then recidivism risk should be lower for inmates who go to private prison. Using prisoner data from Georgia and an IV analysis based on parole guidelines, along with the same definition of recidivism used in this paper, Kuziemko (2013) estimated that each additional month in prison was associated with a 1.4 percent reduction in recidivism. Applied to Mississippi, this estimate implies a 2.8 to 4.2 reduction in recidivism rates for inmates who go to private prison. (As noted in the introduction, however, there is conflicting evidence on the impact of time served on recidivism.) Second, if there is no reduction in recidivism for inmates that go to private prison (as is true in the empirical analysis that follows), this result could be consistent with two interpretations. Either the marginal social benefit of incarceration, β, is close to zero (Abrams 2012), or, as illustrated in panel B of Figure 5, private prisons affect the slope of the recidivism risk curve. In the model, this implies that βis indexed by whether the inmate is in private or public prison, with β priv < β gov (recall that we assume β > 0). This latter effect could occur through several channels. Harsher conditions in private prison, which might result from cost-cutting incentives, could increase recidivism risk as evidenced in prior literature (Chen and Shapiro 2007; Drago, Galbiati, and Vertova 2011). Or, worse peer effects in private prison could lead them to be a “school of crime” (Bayer, Hjalmarsson, and Pozen 2009). 430 AMERICAN ECONOMIC JOURNAL: ECONOMIC POLICY MAY 2021 Table 5—Impact of Private Prison on Recidivism Dependent variable: Recidivism (36-month) Private Prior incarcerations Age/100 Black Single Education < HS Dependent variable mean R2 Observations Offense variables Classification Time trends County fixed effects OLS (1) Probit (2) IV (3) 0.014 (0.007) 0.062 (0.016) −0.311 (0.031) 0.023 (0.006) 0.067 (0.006) −0.011 (0.005) 0.015 (0.007) 0.052 (0.013) −0.336 (0.033) 0.024 (0.006) 0.067 (0.006) −0.010 (0.005) 0.017 (0.038) 0.062 (0.016) −0.310 (0.035) 0.023 (0.006) 0.067 (0.006) −0.011 (0.005) 0.25 0.084 26,593 Yes Yes Yes Yes 0.25 — 26,593 Yes Yes Yes Yes 0.25 0.084 26,593 Yes Yes Yes Yes Notes: Table shows regression estimates of the impact of private prison (Private) on 36-month recidivism (binary). See notes for Table 2 for a list of the detailed controls and the first-stage estimates related to column 3. Mean marginal effects are reported for the probit model in column 2. Standard errors in parentheses are robust and clustered by admission month-year and sentence length. B. Recidivism Results The present analysis does not provide consistent evidence that private prison assignment impacts recidivism. The OLS effect of private prison assignment on recidivism in column 1 of Table 5 indicates a 1.4 percent increase in reoffending with a felony within three years, and the probit model estimate in column 2 is similar at 1.5 percent. Both these estimates are statistically significant ( p < 0.05 ). The IV estimate in column 3, however, has a similar point estimate of 1.7 percent but is not statistically significant. The base rate of recidivism in this sample is about 25 percent, so the OLS and probit estimates imply effect sizes of about 6 percent. The coefficients on the covariates are similar across columns and mostly behave as shown in the literature. Inmates with a felony history recidivate at greater rates, and each prior incarceration is associated with a 5 to 6 percent increase in recidivism. Older inmates recidivate less, and each additional year of age lowers recidivism by 3 to 4 percent (close to the 5 percent estimate in Ganong 2012). Third, compared to married inmates, single inmates recidivate at about a 7 percent higher rate. The coefficient on education is the least expected, as it suggests that inmates with less than high school education recidivate at a 1 percent lower rate. It is not clear why this would be the case, though possibly such inmates receive their General VOL. 13 NO. 2 MUKHERJEE: IMPACTS OF PRIVATE PRISON CONTRACTING 431 Educational Development degrees (GEDs) in prison which reduces their propensity for future crime. Given the imprecision of the IV estimate, it is difficult to rule out the potential theories raised in the previous section. The 95 percent confidence interval on the IV estimate is wide and includes effects from −5.7 to 9.1 percent. The OLS and probit estimates both indicate increases in recidivism for private prison inmates, suggesting that private prisons may undo the reduction in reoffending rates we would expect from the additional time served. I refrain from interpretation of these results, however, as they are not robust to the IV or other specifications. They also do not change with different model specifications, including the addition of r elease-period controls.23 VII. Robustness The main results are robust to a variety of sampling methods, variable definitions, and estimation strategies. Here, I present a selection of tests that deal with potential concerns. A. Instrument Exogeneity: Sensitivity to Controls I show in Table 6 that the estimated impacts of private prison on inmate days served, fraction served, and recidivism are stable to the addition of layered controls.24 The controls are added in eight steps. The first column contains no controls and is included for consistency with prior papers; the second column only controls for sentence length and the custody designation component of the classification variables. Given that there are other strong predictors of the outcomes studied (e.g., time trends), it is not surprising that these first two columns produce estimates that are too large or of incorrect sign. The coefficients stabilize in columns 4 through 8, even as informative covariates related to the inmate’s offense, criminal history, medical class and level of care, demographics, and prior incarcerations are added in a stepwise fashion. (Note that the coefficients in column 8 replicate columns 2 and 4 of Table 2, and column 3 of Table 5.) The stability of the coefficients across these columns provides evidence for the exogeneity of the CapacityShock instrument. 23 I do not include release-period controls in the main analysis because the number of days served (and, hence, the release date) is endogenous to whether an inmate is in private prison. In a separate analysis, I include Mississippi’s unemployment and crime rates (violent crime, murder/manslaughter, rape, robbery, aggravated assault, property crime, burglary, larceny/theft, motor vehicle theft) in the year of the inmate’s release. These results are in online Appendix Table A.7. The OLS result is similar to that in Table 5; there is a 1.3 percent increase in recidivism for private prison inmates ( p < 0.10). The probit and IV specifications yield similar magnitudes but are not statistically significant. 24 This test of instrument exogeneity was proposed in Altonji, Elder, and Taber (2005). Pettersson-Lidbom (2010, 170), which also uses this test, writes: “For this test to be useful in practice, the number of controls must be sufficiently large, they must have significant explanatory power, and they must be representative of the full range of factors that determine the outcome as discussed by Altonji, Elder, and Taber (2005).” These conditions are met in my setting, as there are a large number of controls known to be predictive of inmate outcomes from prior literature; also, the F-statistics show that each layer of controls has substantial explanatory power. 432 AMERICAN ECONOMIC JOURNAL: ECONOMIC POLICY MAY 2021 Table 6—Sensitivity to Controls: IV Estimates of Private Prison Impact on Inmate Outcomes (1) (2) (3) (4) (5) (6) (7) (8) Panel A. Dependent variable: Days served (mean: 722.7) Private 953.144 226.369 85.423 100.033 (340.162) (62.172) (34.062) (31.400) 116.592 81.809 90.322 89.627 (30.091) (26.170) (26.167) (26.414) Wald test of joint significance of the control variables ( p-value in parentheses) R2 −0.288 0.687 0.715 0.717 0.732 0.734 10,591 (0.000) 725 (0.000) 149 (0.000) 582 (0.000) 118 (0.000) 137 91 (0.000) (0.000) Panel B. Dependent variable: Fraction served (mean: 0.71) Private 0.115 −0.724 (0.109) (0.040) 0.069 (0.024) 0.078 (0.022) 0.055 (0.021) 0.043 (0.019) 0.053 0.048 (0.019) (0.019) Wald test of joint significance of the control variables ( p-value in parentheses) 944 (0.000) 219 (0.000) 818 (0.000) 135 (0.000) 121 109 (0.000) (0.000) Panel C. Dependent variable: Recidivism (36-month) (mean: 0.25) Private 0.068 −0.186 −0.022 (0.098) (0.065) (0.044) 0.051 (0.040) 0.077 (0.040) 0.034 (0.038) 0.044 0.017 (0.038) (0.038) Wald test of joint significance of the control variables ( p-value in parentheses) R2 R2 First stage F -statistic (cluster robust) Observations Sentenced years Custody designation County fixed effects Time trends Medical class and level of care Offense Age and race Prior incarcerations Single and education — — −2.228 — — −0.030 0.175 3,272 (0.000) 0.216 0.223 0.055 0.268 0.064 0.272 0.073 0.736 0.276 0.078 0.737 0.279 0.020 0.043 — — 455 (0.000) 933 (0.000) 250 (0.000) 256 (0.000) 165 (0.000) 138 133 (0.000) (0.000) 0.084 57 26,593 202 26,593 565 26,593 666 26,593 706 26,593 902 26,593 900 26,593 887 26,593 No No No No No No No No No Yes Yes No No No No No No No Yes Yes Yes Yes No No No No No Yes Yes Yes Yes Yes No No No No Yes Yes Yes Yes Yes Yes No No No Yes Yes Yes Yes Yes Yes Yes No No Yes Yes Yes Yes Yes Yes Yes Yes No Yes Yes Yes Yes Yes Yes Yes Yes Yes Notes: The table shows regression estimates of the impact of private prison (Private) on inmate time served in panels A (days served) and B (fraction of sentence served) and 36-month recidivism (binary) in panel C. Column 1 includes no controls; column 2 adds controls for sentence length dummies and custody designation; column 3 adds controls for the county of conviction, linear time trends, and the linear time trend interacted with sentence length dummies; column 4 adds dummies for medical class and level of care; column 5 adds indicators (if any) for the primary offense type and up to two additional offenses related to the inmate-sentence; column 6 adds controls for the inmate’s age at admission and his race (whether Black); column 7 adds a set of detailed controls for criminal history, i.e., the number of felonies in the five years prior to admission as well as binary indicators for the offense types of any such felonies; and column 8 adds controls for inmate marital status (whether single) and education (whether less than high school). The Wald tests of joint significance and their respective p -values show that the control variables added at each step have significant explanatory power. The first stage F-statistics indicate the strength of the CapacityShockinstrument in each column. Standard errors in parentheses are robust and clustered by admission month-year and sentence length. B. Alternate Variable Definitions and Sampling Strategies Fraction Served in Private Prison.—The main analysis treats private prison exposure as binary. I also estimate models using the fraction of sentence served in private prison.25 I find that the coefficient on FractionPrivate is 292 for the number of days served and to 0.21 for the fraction of sentence served. Since the mean fraction of sentence served in private prison is about 30 percent, these estimates corroborate the main results, which are roughly o ne-third the size of these estimates. This result 25 These results are in online Appendix Table A.8. VOL. 13 NO. 2 MUKHERJEE: IMPACTS OF PRIVATE PRISON CONTRACTING 433 suggests that the amount of delayed release increases linearly with time served in private prison. The recidivism results remain imprecise. Expanding the Inmate Sample.—The main analysis makes two key sample restrictions. It includes only inmates with sentences less than or equal to six years, so that there are sufficient windows to observe time served and three-year recidivism. It also includes only inmates who served at least 25 percent of their sentences because this is the c ourt-ordered minimum amount, and deviations occur for extenuating circumstances, e.g., compassionate release due to poor health. I demonstrate that these restrictions do not affect the main results. First, I include inmates with sentence lengths between 1 and 11 years and show that the time served results are stable for this group.26 Next, I include those inmates who served less than 25 percent of their sentences. The sample increases to 32,275 and mostly adds inmates with shorter sentence lengths and in public prison. Since these inmates are likely to be of lower recidivism risk—else they would not have received an early release exception—it is not surprising their inclusion increases the effect of private prison exposure on all outcomes.27 C. Alternate Estimation Strategies Leave-One-Out IV Analysis.—I estimate the main results using an alternative leave-one-out instrument that equals the fraction of other inmates with the same admission month and year who are assigned to private prison.28 This instrument obtains predictive power from admission date, as inmates who enter in the same month-year have correlated exposure to the shocks to private prison capacity. (By contrast, CapacityShockuses variation from both admission date and sentence length.) L eave-one-out instruments are generally reserved for random examiner settings such as those involving judging leniency as in Dobbie and Song (2015), but is also strong in this setting: the first-stage F-statistic is 935 after implementing the 2SLS with probit correction method. The estimates on both measures of time served are consistent with the capacity-based IV estimates (90 more days and 4.4 percent more fraction served for private prison inmates), and the recidivism estimate remains imprecise. Matching Analysis on a Restricted Sample.—The shocks in private prison capacity lead to private prison assignment because the new beds are filled quickly, so eligible inmates have a higher likelihood of transfer. I restrict to those inmates who were eligible for each private prison opening or expansion by keeping only those inmates admitted within 90 days of a private prison opening or expansion (the closing is not used in this analysis). Then, I match each one inmate who went to private 26 OLS and IV results are presented in online Appendix Figure A.3. I do not observe the three-year recidivism follow-up window for all of these inmates, so I do not examine that outcome. 27 OLS results in online Appendix Table A.9. Since the IV estimate may be unreliable with the inclusion of these inmates (they are not observed being sent to private prison), I estimate OLS regressions and find that the effect of private prison increases to 242 for the number of days served and to 16.3 percent for the fraction of sentence served. The OLS and probit estimates of private prison on recidivism produce statistically significant coefficients of about 2 percent, similar to the results in Table 5. 28 These results are in online Appendix Table A.10. 434 AMERICAN ECONOMIC JOURNAL: ECONOMIC POLICY MAY 2021 prison with an inmate who stayed in public prison for improved estimates of the impact of private prison on the outcomes studied.29 In total, 1,009 inmates go to private prison during the opening or expansion periods, and the matching method achieves its goal of generating comparable inmates among this restricted sample: the matched pairs are balanced on nearly all covariates. The estimates show that private prison inmates serve 71.2 more days, alternatively estimated as 5.2 more percent of fraction served. There is no statistically significant result on recidivism.30 VIII. Conclusion Despite the continued increase in private prison contracting, there has been little research on how they impact inmates and whether they provide cost savings. This paper contributes a key set of initial evidence on this question, taking care to address concerns about unobservable selection of inmates to private prison. Evaluating private prisons is challenging because prison assignment may depend on unobservable prisoner traits. I address this problem by leveraging prison capacity shocks to generate quasi-random assignment. The analysis shows that inmates in private prison serve about 4 to 7 percent larger fractions of their sentences, or 85 to 90 extra days for the average prisoner. The increased time served for inmates in private prison constitutes a distortion in the delivery of justice. Broadly, these bed capacity shocks also illustrate how c apacity-based constraints can have large impacts within criminal justice. For example, Yang (2016) finds that judge vacancies led to 1.5 percent fewer inmates in the prison system due to increased plea bargaining and reduced prison sentences. The estimates on the impact of private prisons on recidivism are not consistently statistically significant, so I focus my attention on the results related to time served. Based on this metric, the analysis shows that private prisons are indeed cost-saving but may not be as attractive a choice as claimed by their proponents. The results imply that about 48 percent of the cost savings are eroded by the additional time served, leaving about 52 percent in cost savings. To put a number on this in 2016 dollars, consider an average inmate in the sample studied, with sentence length of about three years. Assuming that each year in private prison costs about $45,000 (versus about $50,000 for public prison), the cost savings offered by private prison would be approximately $7,800 (52 percent of the upper bound of three years × $5,000/year = $15,000 in savings) over that inmate’s sentence.31 There are, of course, other costs that are difficult to quantify—e.g., the cost of injustice to society (if private prison inmates systematically serve more time), the inmate’s individual value of freedom, and impacts of the additional incarceration on future employment. Abrams and Rohlfs (2011) estimates a prisoner’s value of 29 The matching analysis requires inmates to be exactly matched on sentence length (rounded to the nearest year), primary offense type, number of offenses, and classification variables. Additionally, inmates are matched on the second and third offense types, if any; number and offense type(s) of prior felonies in the past five years; age; race (whether Black); marital status (whether single); and education (whether less than high school). 30 The summary statistics for the matched sample pairs are in online Appendix Table A.11. The matching on restricted sample results are in online Appendix Table A.12. 31 The cost estimates are broadly taken from Hyland (2019). VOL. 13 NO. 2 MUKHERJEE: IMPACTS OF PRIVATE PRISON CONTRACTING 435 freedom for 90 days at about $1,100 using experimental variation in bail setting. Mueller-Smith (2017) estimates that 90 days of marginal incarceration costs about $15,000 in reduced wages and increased reliance on welfare. If these social costs were to exceed $7,800 in the example stated, private prisons would no longer offer a bargain in terms of welfare-adjusted cost savings. Another contribution of this paper is to show that the mechanism by which private prison inmates experience delayed release is via the greater accumulation of infractions or prison conduct violations. Infractions are used by the state parole board to assess whether prisoners should be granted early release, and inmates in private prison are 9 to 14 percent more likely to receive an infraction over the course of their sentences. This finding suggests that release policy distortions could be curtailed by greater monitoring. For example, states could appoint committees to evaluate whether infractions are correctly imposed. States could also establish tighter guidelines regarding infractions to address the widespread differences between public and private prisons. These findings point to several avenues for future work. Quantifying specific deterrence—the effect of time served in prison on future reoffending—has been a longstanding question to which researchers have uncovered conflicting evidence. This paper provides a unique experiment in which certain inmates receive two treatments: both additional time in prison and serving time in a private rather than public prison. The point estimate of these combined effects in my analysis is about 1.5 percent, which is about a 6 percent effect size. It is possible that all of this effect comes from the additional time served in prison. Or, it could be that private prisons increase criminal reoffending; separating the effects in future work would be valuable in assessing the policy impacts of private contracting. E x ante, the answer is not clear: on the one hand, factors such as the harsher conditions that have been documented in private prisons may increase future criminal behavior as in Chen and Shapiro (2007). On the other hand, factors such as more disciplining in private prison could reduce future criminal behavior. Future related research on private contracting may also find inspiration from health care, a setting that has been studied more thoroughly in this context (e.g., Duggan, Gruber, and Vabson 2017). For example, states worry that private prison contractors may “cream skim” the lowest cost inmates in the same way that insurers can attempt to select lowest risk patients (Brown et al. 2014; Kuziemko, Meckel, and Rossin-Slater 2013). As another example, the Hospital Readmissions Reduction Program was introduced in 2013 by the Center for Medicare and Medicaid Services to penalize hospitals for excessive readmission rates, which are akin to recidivism rates; Gupta (2017) and Batt, Bavafa, and Soltani (2020) analyze this program, focusing on contract incentives. Similar studies in the prison context would be useful. REFERENCES Abrams, David S. 2012. “Estimating the Deterrent Effect of Incarceration Using Sentencing Enhance- ments.” American Economic Journal: Applied Economics 4 (4): 32–56. Abrams, David S., and Chris Rohlfs. 2011. “Optimal Bail and the Value of Freedom: Evidence from the Philadelphia Bail Experiment.” Economic Inquiry 49 (3): 750–70. 436 AMERICAN ECONOMIC JOURNAL: ECONOMIC POLICY MAY 2021 Adams, Renée, Heitor Almeida, and Daniel Ferreira. 2009. “Understanding the Relationship between Founder-CEOs and Firm Performance.” Journal of Empirical Finance 16 (1): 136–50. Allen, B.J., Deepa Chandrasekaran, and Suman Basuroy. 2018. “Design Crowdsourcing: The Impact on New Product Performance of Sourcing Design Solutions from the ‘Crowd.’” Journal of Marketing 82 (2): 106–23. Altonji, Joseph G., Todd E. Elder, and Christopher R. Taber. 2005. “Selection on Observed and Unobserved Variables: Assessing the Effectiveness of Catholic Schools.” Journal of Political Economy 113 (1): 151–84. Amy, Jeff. 2017. “Former Mississippi Prison Chief Sentenced to Nearly 20 Years.” Washington Post, May 24. https://www.washingtontimes.com/news/2017/may/24/mississippi-ex-prison-chief-facessentencing-for-c/. Angrist, Joshua D., and Alan B. Krueger. 2001. “Instrumental Variables and the Search for Identification: From Supply and Demand to Natural Experiments.” Journal of Economic Perspectives 15 (4): 69–85. Atkin, David. 2016. “Endogenous Skill Acquisition and Export Manufacturing in Mexico.” American Economic Review 106 (8): 2046–85. Bales, William D., Laura E. Bedard, Susan T. Quinn, David T. Ensley, and Glen P. Holley. 2005. “Recidivism of Public and Private State Prison Inmates in Florida.” Criminology and Public Policy 4 (1): 57–82. Barbarino, Alessandro, and Giovanni Mastrobuoni. 2014. “The Incapacitation Effect of Incarceration: Evidence from Several Italian Collective Pardons.” American Economic Journal: Economic Policy 6 (1): 1–37. Batt, Robert, Hessam Bavafa, and Mohamad Soltani. 2020. “Quality Improvement Spillovers: Evidence from the Hospital Readmissions Reduction Program.” https://pdfs.semanticscholar.org/8 65c/337ca1b20abc12c1dafd7e556143e3b8f6bd.pdf. Bayer, Patrick, Randi Hjalmarsson, and David Pozen. 2009. “Building Criminal Capital behind Bars: Peer Effects in Juvenile Corrections.” Quarterly Journal of Economics 124 (1): 105–47. Bayer, Patrick, and David E. Pozen. 2005. “The Effectiveness of Juvenile Correctional Facilities: Public versus Private Management.” Journal of Law and Economics 48 (2): 549–89. Becker, Gary S. 1968. “Crime and Punishment: An Economic Approach.” Journal of Political Economy 76 (2): 169–217. Berger, Allen N., and Raluca A. Roman. 2017. “Did Saving Wall Street Really Save Main Street? The Real Effects of TARP on Local Economic Conditions.” Journal of Financial and Quantitative Analysis 52 (5): 1827–67. Bhuller, Manudeep, Gordon B. Dahl, Katrine V. Loken, and Magne Mogstad. 2018. “Intergenerational Effects of Incarceration.” AEA Papers and Proceedings 108: 234–40. Brown, Jason, Mark Duggan, Ilyana Kuziemko, and William Woolston. 2014. “How Does Risk Selection Respond to Risk Adjustment? New Evidence from the Medicare Advantage Program.” American Economic Review 104 (10): 3335–64. Buonanno, Paolo, and Steven Raphael. 2013. “Incarceration and Incapacitation: Evidence from the 2006 Italian Collective Pardon.” American Economic Review 103 (6): 2437–65. Cameron, A.C., P.K. Trivedi, Frank Milne, and J. Piggott. 1988. “A Microeconometric Model of the Demand for Health Care and Health Insurance in Australia.” Review of Economic Studies 55 (1): 85–106. Carson, E. Ann, and E. Anderson. 2016. “Prisoners in 2015.” Bureau of Justice Statistics Bulletin. US Department of Justice, NCJ-250229. https://www.bjs.gov/content/pub/pdf/p15.pdf. Carson, E. Ann. 2018. “Prisoners in 2016.” Bureau of Justice Statistics, NCJ251149). US Department of Justice. https://www. bjs. gov/content/pub/pdf/p16. pdf. Casto, Anne B., and Elizabeth Forrestal. 2013. Principles of Healthcare Reimbursement. 3rd ed. Chicago: American Health Information Management Association. Chen, M. Keith, and Jesse M. Shapiro. 2007. “Do Harsher Prison Conditions Reduce Recidivism? A Discontinuity-Based Approach.” American Law and Economics Review 9 (1): 1–29. Chen, Stephanie. 2009. “Pennsylvania Rocked by ‘Jailing Kids for Cash’ Scandal.” CNN, February 23. http://www.cnn.com/2009/CRIME/02/23/pennsylvania.corrupt.judges. Chirakijja, Janjala. 2018. “The Local Economic Impacts of Prisons.” https://economics.smu.edu.sg/ sites/economics.smu.edu.sg/files/economics/pdf/Seminar/2019/20190410.pdf. Dippel, Christian, and Michael Poyker. 2019. “Do Private Prisons Affect Criminal Sentencing?” NBER Working Paper 25715. VOL. 13 NO. 2 MUKHERJEE: IMPACTS OF PRIVATE PRISON CONTRACTING 437 Dobbie, Will, Jacob Goldin, and Crystal S. Yang. 2018. “The Effects of Pretrial Detention on Con- viction, Future Crime, and Employment: Evidence from Randomly Assigned Judges.” American Economic Review 108 (2): 201–40. Dobbie, Will, and Jae Song. 2015. “Debt Relief and Debtor Outcomes: Measuring the Effects of Consumer Bankruptcy Protection.” American Economic Review 105 (3): 1272–1311. Drago, Francesco, Roberto Galbiati, and Pietro Vertova. 2011. “Prison Conditions and Recidivism.” American Law and Economics Review 13 (1): 103–30. Dubin, Jeffrey A., and Daniel McFadden. 1984. “An Econometric Analysis of Residential Electric Appliance Holdings and Consumption.” Econometrica 52 (2): 345–62. Duggan, Mark, Jonathan Gruber, and Boris Vabson. 2017. “The Consequences of Health Care Privatization: Evidence from Medicare Advantage Exits.” American Economic Journal: Economic Policy 10 (1): 153–86. Durose, Matthew R., Alexia D. Cooper, and Howard N. Snyder. 2014. Recidivism of Prisoners Released in 30 States in 2005: Patterns from 2005 to 2010 (Vol. 28). Washington, DC: US Department of Justice, Office of Justice Programs, Bureau of Justice Statistics. Ganong, Peter N. 2012. “Criminal Rehabilitation, Incapacitation, and Aging.” American Law and Economics Review 14 (2): 391–424. Gupta, Atul. 2017. “Impacts of Performance Pay for Hospitals: The Readmissions Reduction Program.” Becker Friedman Institute for Research in Economics, Health Economics Working Paper 2017-07. Harrison, Paige M., and Allen J. Beck. 2005. Prison and Jail Inmates at Midyear 2004. Washington, DC: Bureau of Justice Statistics. Hart, Oliver, Andrei Shleifer, and Robert W. Vishny. 1997. “The Proper Scope of Government: Theory and an Application to Prisons.” Quarterly Journal of Economics 112 (4): 1127–61. Homeland Security Advisory Council. 2016. Report of the Subcommittee on Privatized Immigration Detention Facilities. Washington, DC: US Department of Homeland Security. Hyland, Shelley S. 2019. “Justice Expenditure and Employment Extracts, 2016.” Bureau of Justice Statistics. https://www.bjs.gov/index.cfm?ty=pbdetail&iid=6728 (accessed June 1, 2019). Intrator, Orna, David C. Grabowski, Jacqueline Zinn, Mark Schleinitz, Zhanlian Feng, Susan Miller, and Vince Mor. 2007. “Hospitalization of Nursing Home Residents: The Effects of States’ Medic- aid Payment and Bed-Hold Policies.” Health Services Research 42 (4): 1651–71. Jian, Weiyan, and Yan Guo. 2009. “Does Per-Diem Reimbursement Necessarily Increase Length of Stay? The Case of a Public Psychiatric Hospital.” Health Economics 18 (S2): S97–S106. Kuziemko, Ilyana. 2013. “How Should Inmates be Released from Prison? An Assessment of Parole versus Fixed-Sentence Regimes.” Quarterly Journal of Economics 128 (1): 371–424. Kuziemko, Ilyana, Katherine Meckel, and Maya Rossin-Slater. 2013. “Do Insurers Risk-Select Against Each Other? Evidence from Medicaid and Implications for Health Reform.” NBER Working Paper 19198. Kyckelhahn, Tracey. 2015. “Justice Expenditure and Employment Extracts, 2012.” Bureau of Justice Statistics. https://www.bjs.gov/index.cfm?ty=pbdetail&iid=5239 (accessed May 15, 2019). Langan, Patrick A., and David J. Levin. 2013. “Recidivism of Prisoners Released in 1994.” Washington, DC: Bureau of Justice Statistics. Lanza-Kaduce, Lonn, Karen F. Parker, and Charles W. Thomas. 1999. “A Comparative Recidivism Analysis of Releasees from Private and Public Prisons.” Crime and Delinquency 45 (1): 28–47. Lindqvist, Erik. 2008. “Privatization of Credence Goods: Theory and an Application to Residential Youth Care.” Research Institute of Industrial Economics Working Paper 750. Liptak, Adam. 2008. “U.S. Prison Population Dwarfs that of Other Nations.” April 23. The New York Times. https://www.nytimes.com/2008/04/23/world/americas/23iht-23prison.12253738.html Lochner, Lance, and Enrico Moretti. 2004. “The Effect of Education on Crime: Evidence from Prison Inmates, Arrests, and Self-Reports.” American Economic Review 94 (1): 155–89. Maurin, Eric, and Aurelie Ouss. 2009. “Sentence Reductions and Recidivism: Lessons from the Bastille Day Quasi Experiment.” IZA Institute of Labor Economics Discussion Paper 3990. Mississippi Department of Corrections (MDOC). 1996. Mississippi Department of Corrections Fiscal Year 1995 Annual Report. Jackson, MS: MDOC. Mississippi Department of Corrections (MDOC). 2011. Mississippi Department of Corrections Inmate Handbook. Jackson, MS: MDOC. Mississippi Department of Corrections (MDOC). 2012. Mississippi Department of Corrections Fiscal Year 2011 Annual Report. Jackson, MS: MDOC. 438 AMERICAN ECONOMIC JOURNAL: ECONOMIC POLICY MAY 2021 Mueller-Smith, Michael. 2017. “The Criminal and Labor Market Impacts of Incarceration.” Unpublished. Mukherjee, Anita. 2021. “Replication data for: Impacts of Private Prison Contracting on Inmate Time Served and Recidivism.” American Economic Association [publisher], Inter-university Consortium for Political and Social Research [distributor]. https://doi.org/10.3886/E119726V1. Norton, Edward C., and Douglas O. Staiger. 1994. “How Hospital Ownership Affects Access to Care for the Uninsured.” RAND Journal of Economics 21 (1): 171–85. Owens, Emily G. 2009. “More Time, Less Crime? Estimating the Incapacitative Effect of Sentence Enhancements.” Journal of Law and Economics 52 (3): 551–79. Owens, Emily G. 2011. “Truthiness in Punishment: The Far Reach of Truth-in-Sentencing Laws in State Courts.” Journal of Empirical Legal Studies 8 (S1): 239–61. Pettersson-Lidbom, Per. 2010. “Dynamic Commitment and the Soft Budget Constraint: An Empirical Test.” American Economic Journal: Economic Policy 2 (3): 154–79. Raphael, Steven, and Michael A. Stoll. 2014. “A New Approach to Reducing Incarceration while Maintaining Low Rates of Crime.” http://www.hamiltonproject.org/assets/legacy/files/downloads_and_ links/v5_THP_RaphaelStoll_DiscPaper.pdf. Rodwin, M.A., and A. Okamoto. 2000. “Physicians’ Conflicts of Interest in Japan and the United States: Lessons for the United States.” Journal of HealthPolitics, Policy and Law 25 (2): 343–75. Shavell, Steven. 1987. “A Model of Optimal Incapacitation.” American Economic Review 77 (2): 107–10. Spivak, Andrew L., and Susan F. Sharp. 2008. “Inmate Recidivism as a Measure of Private Prison Performance.” Crime and Delinquency 54 (3): 482–508. Williams, Timothy. 2016. “Privately Run Mississippi Prison, Called a Scene of Horror, Is Shut Down.” New York Times, September 15. https://www.nytimes.com/2016/09/16/us/mississippi-closesprivate-prison-walnut-grove.html. Wooldridge, Jeffrey M. 2002. Econometric Analysis of Cross Section and Panel Data. Cambridge, MA: MIT Press. Yang, Crystal S. 2016. “Resource Constraints and the Criminal Justice System: Evidence from Judicial Vacancies.” American Economic Journal: Economic Policy 8 (4): 289–332. Zapryanova, Mariyana. 2014. “The Effects of Time in Prison and Time on Parole on Recidivism.” https://www.k-state.edu/economics/seminars/papers/Zapryanova%20JMP.pdf.