Adversariality, Plea Bargaining, and Prison Population Growth - Evidence From a Natural Experiment, 2019
Download original document:
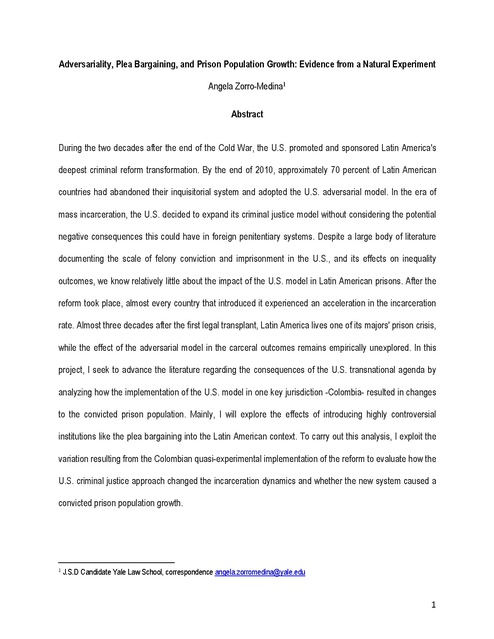
Document text
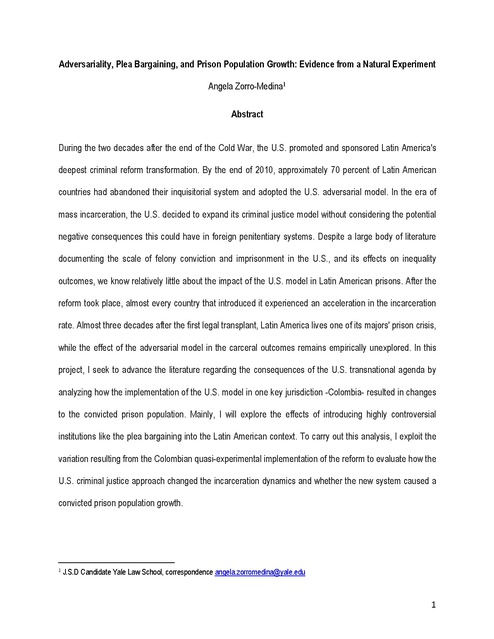
Document text
This text is machine-read, and may contain errors. Check the original document to verify accuracy.
Adversariality, Plea Bargaining, and Prison Population Growth: Evidence from a Natural Experiment Angela Zorro-Medina1 Abstract During the two decades after the end of the Cold War, the U.S. promoted and sponsored Latin America's deepest criminal reform transformation. By the end of 2010, approximately 70 percent of Latin American countries had abandoned their inquisitorial system and adopted the U.S. adversarial model. In the era of mass incarceration, the U.S. decided to expand its criminal justice model without considering the potential negative consequences this could have in foreign penitentiary systems. Despite a large body of literature documenting the scale of felony conviction and imprisonment in the U.S., and its effects on inequality outcomes, we know relatively little about the impact of the U.S. model in Latin American prisons. After the reform took place, almost every country that introduced it experienced an acceleration in the incarceration rate. Almost three decades after the first legal transplant, Latin America lives one of its majors' prison crisis, while the effect of the adversarial model in the carceral outcomes remains empirically unexplored. In this project, I seek to advance the literature regarding the consequences of the U.S. transnational agenda by analyzing how the implementation of the U.S. model in one key jurisdiction -Colombia- resulted in changes to the convicted prison population. Mainly, I will explore the effects of introducing highly controversial institutions like the plea bargaining into the Latin American context. To carry out this analysis, I exploit the variation resulting from the Colombian quasi-experimental implementation of the reform to evaluate how the U.S. criminal justice approach changed the incarceration dynamics and whether the new system caused a convicted prison population growth. 1 J.S.D Candidate Yale Law School, correspondence angela.zorromedina@yale.edu 1 I. INTRODUCTION During the early 1990s, the U.S. initiated a global campaign against transnational crime. In 1995, President Clinton spoke before the U.N. General Assembly calling for the establishment of an international network to combat drug trafficking, terrorism, and criminality. As part of these efforts to strengthen international cooperation, the U.S. created the "International Law Enforcement Academy" (ILEA), the "Office of Overseas Prosecutorial Development, Assistance and Training" (OPDAT), and the "Criminal Investigative Training Assistance Program" (ICITAP). Through the OPDAT and ICITAP, the U.S. Department of Justice (DOJ)Criminal Division has been able to influence police forces and criminal justice institutions overseas. To complement these institutions' work, the U.S. opened five ILEA's offices2 around the world to promote criminal justice techniques, criminal procedures, and transnational crime priorities (McLeod 2010). As part of this agenda, during the two decades following the Cold War, the U.S. promoted and sponsored Latin America's most profound criminal reform known as the Latin American Criminal Procedural Revolution (LACPR) (Langer 2007; McLeod 2010). The U.S. intervention varied across countries. In some cases, U.S. foreign aid programs financed the transformation without direct participation in the legislative discussion, while in others, the DOJ directly drafted procedural penal codes. As a result, by the end of 2010, approximately 70 percent of Latin America had adopted the U.S. adversarial model (see Figure 1).3 [FIGURE 1 HERE] 2 The U.S. divided the world into four relevant regions to allocate the ILEA’s offices: 1) Central-Eastern Europe and Central Asia (Budapest, Hungary); 2) South East Asia and China (Bangkok, Thailand); 3) South of the Sahel and West Africa (Gaborone, Botswana); and 4) Latin America and the Caribbean (San Salvador, El Salvador). In addition to those four offices, the ILEA established a central office in Roswell, New Mexico. This office is in charge of training officers from all countries around the globe. 3 After 2010 the transformations in the region continued. Uruguay adopted the new adversarial model in 2017. Four Latin American countries have not fully transitioned from an inquisitorial system to the U.S. model: Brazil, Belize, Suriname, and Guyana. However, this does not mean that their internal systems have not been influenced by the transnational project. For instance, between 1995 and 2008, Brazil has gone through two reformations moving toward the U.S. model without completing the transition. 2 In the mass incarceration era, the U.S. decided to expand its criminal justice model without considering the potential negative consequences this could have in foreign penitentiary systems. Despite a large body of literature documenting the scale of felony conviction and imprisonment in the U.S. (Dumont et al. 2012; David Garland 2001; Pettit and Western 2004) and its effects on inequality outcomes (Braman 2004; Comfort 2007; Geller et al. 2012; Hagan and Dinovitzer 1999; Haskins 2015, 2016; Pager 2003; Wakefield and Wildeman 2013; Western 2002, 2006; Western and Sirois 2017; Wildeman 2009), little is known about the U.S. model effects abroad. Figure 2 evidences the enormous variation across the Latin American prison population after the implementation of the U.S. model. The different patterns observed in figure 2 reveal the difficulties in understanding the effect of the reform in the region using comparative descriptive data. Despite these challenges, studies exploring the impact of the U.S. adversarial model in Latin American carceral outcomes have limited their analysis to these statistical comparisons. Almost three decades after the first wave of the LACPR, the region is experiencing a prison overcrowding crisis, and the lack of empirical research continues to obscure the causes behind it (Dudley and Bargent 2017; Marton Ramaciotti 2017). [FIGURE 2 HERE] This article seeks to advance the literature regarding the causes of prison population growth in the Americas by contributing to the ongoing mass-incarceration research from three different perspectives. First, I offer the first econometrical study evaluating the LACPR impact in incarceration rates. This analysis provides causal evidence of the effect of criminal procedural laws on imprisonment patters. To do this, I use Colombia as a case of study considering the reform quasi-experimental implementation and data availability. As I will show later in this paper, Colombia offers a perfect setting to develop a causal inference analysis.4 In addition 4 The clear-cut implementation of the reform makes Colombia one of the most successful countries transitioning from an inquisitorial system to the U.S.-style adversarial model. In 2008, only three years after introducing the new criminal system, Colombia extended the coverage of the adversarial model to its entire territory. Comparing the Colombian case with the Mexican case, the implementation success of the Colombian reform is undeniable. The Mexican government failed in the implementation of the 2005 3 to these empirical advantages, legal scholars argue that the DOJ's largest influence occurred in Colombia since OPDAT agents directly drafted sections of the approved legislation (Langer 2007). The DOJ's high involvement in the Colombian Procedural Penal Code allows directly exploring the effect of the U.S. intervention in foreign penitentiary systems. To develop this empirical study, I designed data treatment strategies to work with Latin American prison data. Understanding the differences between the U.S. and Latin American penitentiary systems is crucial to determine the cofounding institutional and socioeconomic factors that affect them. Here I propose particular guidelines about the treatment of Latin American data highlighting the need to develop empirical models design for the Global South, instead of directly applying Global North methodologies without understanding the differences among them. Second, I explored the effects of introducing plea bargaining into the Latin American context despite the U.S. mass-incarceration literature critiques of this figure (Heumann and Loftin 1979; Savitsky 2012). Before the introduction of the U.S. adversarial model, most Latin American countries only had one way to end criminal processes: trial. The new model increased the prosecutorial discretion by creating the possibility to negotiate agreements to terminate the process without a trial (plea bargaining) (Langer 2021). The lack of individual records to test changes in the risk of conviction or imprisonment before 2004 complicates the evaluation of plea negotiation effects in Latin America. To carry out this analysis, I combine event study and difference-in-difference estimations to assess how the U.S. adversarial model changed Latin American incarceration patterns. Using the variation resulting from the LACPR different timing in Colombia, I study the changes in entry and release rates, differences by gender, and disparities in first and second convictions. reform, which required the approval of second reform in 2008 to complete the transition. According to official sources, by 2016 the adversarial system only covered 74% of the country. (Riego 2007; Secretaría de Gobernación México 2016) 4 Finally, this paper aims to begin filling the literature gap between the U.S. and Latin American advancing empirical sociological research in the latter. Diverse scholars in the field have recognized the importance of comparative analysis to understand better the complex causal relationships that exist in national contexts (Munroe, Munroe, and Whiting 1981; Ruback and Weiner 1993; Sampson and Lauritsen 1997; Wildeman 2016); however, few empirical investigations outside the U.S. and Europe have been done in this area. By focusing on how changes in the prosecutor's discretion and the procedure length affected incarceration patterns, I intend to contribute to this empirical comparative conversation using different national settings in a causal inference framework. The paper proceeds as follows: In the second section, I present a literature review of the relationship between adversariality, plea bargaining, and mass-incarceration. In the following segment, I discuss the Colombian case and introduce my empirical hypotheses. In the fourth and fifth sections, I describe the data and explain the methodological strategy. And finally, in the last two segments, I present the results and conclusions of my estimates showing that the U.S. adversarial system caused an increase of 18% in Colombian incarceration rates. I will discuss how these changes affected men and women differently, connecting these disparities to the plea bargaining introduction. II. ADVESARIALITY, PLEA BARGAINING AND MASS-INCARCERATION During the 1960s, the research on the penal system explored the importance of procedural institutions on justice adjudication. Debates around the advantages and disadvantages of the Anglo-American adversarial model and European inquisitorial systems promoted a comparative analysis of criminal procedural institutions (Goldstein 1975; Goldstein and Marcus 1977; Langbein 1978; Langbein and Weinreb 1977). The transnational approach challenged the superiority of one model over the other, encouraging empirical evaluations of their effects in penal outcomes. However, the U.S. incarceration rise in the mid1970s stimulated the proliferation of national investigations, and comparative analysis fell out of favor in the U.S. criminological study. 5 Throughout the following decades, research on the criminal justice system widely focused on the U.S. prison population growth under an American exceptionalism approach. Empirical studies on this area explored the causal factors driving incarceration rates using U.S. historical data at the federal and state level (Marvell and Moody 1994; Pfaff 2017; Raphael 2010; Sorensen and Stemen 2002; Stolzenberg and D'Alessio 1996; Zimring, Hawkins, and Hawkins 1993). Some of the most important findings reveal that crime (Blumstein and Beck 1999; Blumstein and Moitra 1979; Listokin 2003a; Pfaff 2008, 2011; Reitz 1997; Zimring et al. 1993), economic conditions (Ignatieff 1978; Rothman 1990; Rusche and Kirchheimer 2003), demographic trends (Beckett 1999; Caplow and Simon 1999; Fryer et al. 2005; Marvell and Moody 1997; Sampson and Lauritsen 1997; Shannon et al. 2017; Tonry 1997; Western 2006), and political culture changes (David Garland 2001; D. Garland 2001; Mauer 1999; Shepherd 2002) are forces driving imprisonment growth. Although these research projects have been vital to understanding the U.S. mass-incarceration phenomenon, little is known about the role of adversariality in the prison population growth. Social science research has connected adversariality to the U.S. mass-incarceration through the increasing use of plea negotiations.5 The consolidation of plea bargaining as the modal form of criminal adjudication caused that between 1966 and 1983, over 90% of the U.S. sentences in criminal cases resulted from a guilty plea (Grossman and Katz 1983; Langbein 1978; Newman and Remington 1966). The central role of plea bargaining in the U.S. system motivated empirical studies evaluating its effects on crime rates, prison growth, and sentence length (Rubinstein and White 1979). The primary findings of this research suggest that inequality factors influence sentences length differences (Anderson, Kling, and Stith 1999; 5 Legal scholars have connected the appearance of plea bargaining with a natural development of adversariality. Using historical analysis, supporters of this view, claim that plea negotiations are not new form of adjudicating punishment, but a tool traceable to the nineteenth century highlighting the long tradition of this form of negotiation inside the adversarial culture. (Feeley 1978, 1982; Heumann 1975; Padgett 1985) 6 LaCasse and Payne 1999), and the likelihood to go to trial in the guilty plea cases (Ashenfelter, Eisenberg, and Schwab 1995; LaCasse and Payne 1999). Other empirical research has tested whether eliminating plea negotiations caused a change in incarceration rates exploiting state reforms (Bushway, Owens, and Piehl 2012; Church 1976; Weninger 1987). However, the results have been inconclusive. Moreover, in recent years, new studies discussing the role of plea negotiations on prison population growth have reappeared (Bibas 2012; Bushway, Redlich, and Norris 2014; Crespo 2018; Pfaff 2017). Yet, the existence of plea negotiations since the nineteen maintain the causal link unidentified in the absence of a proper counterfactual (Feeley 1978). In this paper, I intend to go back to the comparative analysis and transnational approach to assess the causal relationship between adversariality, guilty pleas, and incarceration rates. Specifically, I propose using the U.S. agenda in Latin America to advance both the understanding of the Latin American prison systems and the criminal procedural role in prison population growth. I exploit the Colombian quasiexperimental setting to perform this empirical evaluation. Since not all countries followed a quasiexperimental implementation of the reform, I focused on the Colombian case because it offers a unique setting by including for the first time the institution of plea bargaining and doing it in a natural experimental form. III. THE COLOMBIAN NATURAL EXPERIMENT The adoption of the U.S. adversarial model in Latin America created a quasi-experimental setting to evaluate the effects of adversariality and plea bargaining on incarceration rates. Specifically, Colombia, Perú, Mexico, and Chile followed a staggered implementation. However, only Chile and Colombia successfully executed the rolling-out process in the original dates. The Chilean case introduced the reform, between 2000 and 2005, in five waves across its territory, and reserved all metropolitan regions for the final stage (40.1% 7 of the Chilean population) (Gendarmeria de Chile 2000).6 The Chilean separation between rural and urban areas challenges the as-if-random identification assumption of a quasi-experimental analysis considering the relationship between incarceration rates and population type (rural or urban) (Clear 2007; Sampson 1983, 1985; Sampson and Loeffler 2010; Simes 2016, 2018; Travis, Western, and Redburn 2014). In contrast, Colombia divided its territory into four stages, covering approximately 25% of the population in each phase. The even distribution of the Colombian reform offers an arguable exogenous source of variation with distinguishable treatment and control groups. Figure 3 displays the distribution of the reform in the Colombian territory. [Figure 3 Here] Besides the advantages of the Colombian rolling-out reform, Latin American literature identified Colombia as one of the most successful cases transitioning towards the U.S. adversarial model (CEJA 2015; de Luca 2008) and the closest country to the U.S. in the region (Langer 2007; McLeod 2010). During the 1990s, Colombia strengthened the relationship with the U.S. with the creation of Plan Colombia, a major counternarcotics and criminal justice reform package. The Plan Colombia had bipartisan support in the U.S., which allowed it to survive from the Clinton Administration to the Obama Administration. As part of this cooperation program, the U.S. defined the Colombian justice reform priorities and monitored the progress benchmarks. Following the U.S. intervention, in 2000, Colombia started its penal transformation enacting a mixed inquisitorial procedural code with an adversarial lean tendency (Law 600/00). Despite this initial reform, the Colombian system continued to be highly inquisitorial. Under the law 600/00, prosecutors served a dual function as investigators and adjudicators in long written criminal processes that ended in private trials. Since all cases terminated in trials, the overloaded judicial system produced a low number of convictions every 6 First stage 9.7% population (16 December 2000); Second stage 10.9% population (16 October 2001); Third stage 4,4% (16 December 2002); Fourth stage 34.7% population (16 December 2003); Fifth stage metropolitan region 40,1% (16 June 2005). 8 year. After the first wave of the LACPR in 2005, the Colombian system only resolved 21% of new cases. However, this low rate changed after the LACPR third wave when the judicial system started processing almost 50% of incoming incidents (Martínez Cuéllar, Hernández Luna, and Parra González 2008). The small number of sentences created an impunity perception that motivated Colombian elites to advocate for a new procedural reform. This national movement amplified the U.S. economic and political pressures to complete the transition towards an adversarial model. Consequently, in August 2004, the Colombian Congress approved the new Adversarial Criminal Justice Code (ACJC). The reform transformed the written private processes into oral publicly available hearings7 and imposed temporal limits to reduce procedural length. After the change, between 2006 and 2007, decisions in homicide cases went from 493 days to 116 days (76% reduction), while property crimes cases went from 597 to 69 days (87% reduction) (Zorro-Medina, Acosta Mejia, and Mejia 2016). Additionally, the ACJC included alternative forms to terminate criminal cases. Mainly, the ACJC granted prosecutors the power to engage in plea bargains with the ultimate goal of increasing conviction rates. Before the ACJC, the law 600/00 regulated the agreement terms between the defense and the prosecutor, and it never substituted the trial. Those agreements offered the accused the possibility to accept charges to obtain a sentence reduction stipulated in the procedural code. In contrast, the ACJC incorporated fully discretional negotiations between the prosecutor and the defense in exchange for a guilty plea, and those agreements substituted trials. Between 2005 and 2007, trial sentences represented around 7% of total convictions (6% and 7% respectively). In contrast, during the same period, guilty pleas increased 9%, going from 7% to 14% of total convictions (Martínez Cuéllar et al. 2008). Moreover, from 2007 to 2016, the Colombian system 7 Despite the changes during the phases prior to the conviction, the Colombian criminal procedure continued to use the old inquisitorial written structure after a conviction happened. 9 acquitted one person for every nine convictions, and by 2016, almost 50% of sentences came from guilty pleas (Higuera Guío 2016). At first sight, Colombia introduced adversariality and plea bargaining following an experimental implementation as-good-as-if-random. Using this staggered rollout, I intend to estimate the effect of these two factors in prison population growth. Previous descriptive studies claim that the LACPR increased incarceration rates (Salinero Echeverría 2012; Zorro Medina 2014); however, this literature has been unable to identify a causal link. To address this, I separate the analysis into two stages. First, I evaluate whether the LACPR caused an increase in prison population growth. Since no-individual data is available, I estimate average treatment effects using aggregated data. By assessing the LACPR impact on prison population growth, I would be able to predict whether introducing an adversarial model, ceteris paribus, in a previously inquisitorial-country increases incarceration rates. Secondly, I explore the impact of introducing plea bargaining. As mentioned before, ideally, individual data would allow me to evaluate changes in conviction risk. However, due to data limitations, I calculate the average treatment effects. To separate the impact of procedural celerity and guilty-pleas, I classified conviction outcomes and their potential change as evidence supporting one of these two mechanisms. Figure 4 depicts the outcomes I have identified as evidence of procedural celerity (Mechanism A) or plea-bargaining (Mechanism B) effects. [Figure 4 Here] As observe in figure 4, I associate increases in all conviction types with procedural celerity (Mechanism A, Evidence 1A). Since the procedural length reduction occurred for all criminal cases, finding an increase in both first and second convictions would suggest that adversarial celerity contributes to prison growth. Furthermore, I would expect the relationship between first and second convictions rates to remain constant considering both processes followed the same procedural stages. Differentiated effects would suggest a different mechanism is driving these rates changes. The second evidence comes from admission and release 10 data. Since the ACJC maintained the post-sentencing old structure, an increase in admission rates but no effect on releases would support the idea that Mechanism A caused an expansion in the Colombian prison population. For Mechanism B, I perceive a change in release rates as evidence that plea-bargaining affected prison population growth, ceteris paribus. Because guilty pleas usually come with a sentence reduction, a rise in release rates could be signaling shorter prison sentences on average (Evidence 1B). In addition to release changes, I include estimations by sex associating gender difference to the discretional use of prison in guilty pleas (Evidence 2B). By maintaining the rest of the factors constant, a gender differential effect would be driven by plea bargaining negotiations since it is the only source of variation between men and women under the new code. I only explored gender differences since no other demographic characteristics are available.8 In the following sections, I explain the data used in this analysis, providing more empirical evidence of the quasi-experimental conditions of the Colombian case. IV. DATA To analyze the effect of the LACPR on the prison population growth, I constructed a municipality panel dataset using four sources of information. Data on prison population come from the Colombian National Penitentiary Institute (INPEC), the national agency responsible for all prison matters in the country, including collecting and maintaining data from all prisons across the national territory. Convicted population data is available every six months from June 2000 to December 2015 for all prisons in Colombia.9 Male and female incarceration rates are available from 2000 to 2015. However, gender-specific first and second conviction rates are only available after 2005; therefore, I only used sex-aggregated information during this period. Entry 8 Information on race, ethnicity, age, education level, among other variables is only available at national level. This aggregation level does not allow to exploit the quasi-experimental implementation of the reform. 9 Some prisons opened, closed, and re-opened during that period of time. 11 and release data10 are available every six months from June 2000 to December 2004, and from June 2008 to December 2015. Convicted admission and release rates are accessible only from June 2008 to December 2015. The data records 4,652 observations of prisons with convicted population over 15 years and 2,247 observations of sentenced population entries and releases over eight years. 11 Data on crime come from the National Police Reports on the System of Statistical Information on Crime, Violations, and Arrests (Sistema de Informacion Estadistico, Delincuencial, Contravencional y Operativo de la Policia Nacional-SIEDCO). The SIEDCO data uses incident-level information on criminal activity and arrests including the offense, date, and location.12 For this project, I aggregated crime and arrest data by municipality every six months. I only included high-impact crimes with lower measurement error (homicides, assaults, muggings, business robberies, home burglaries, and vehicle thefts), since the National Police data suffer from underreporting over the relevant period. To aggregate it, I constructed a crime index using a summation function weighting each crime by its average prison sentence.13 Because the dependent variables are convicted prison outcomes, the relevance of each crime depends on the average period a person spends in prison if convicted (see Table A2 for further detail about the weighting calculation). Finally, to control for socioeconomic and demographic municipality differences, I use a cross-sectional time-series data from the Center of Economic Studies from Universidad de Los Andes (CEDE) and the public demographic information of the Colombian Department of National Statistics (DANE). The CEDE panel 10 In Colombia prisons held pre-trial detainees and convicted individuals. In this article, I focus in the convicted individuals only. However, admission and release data between 2000 and 2004 includes both populations. Since this is the only data available, I use it as a proxy of convicted admission and release rates. Considering that convicted population is never larger than total prison population, a significant estimator would be underestimating the effect of the reform but never overestimating it. 11 According to the data, 160 prisons existed between 2000 and 2015 in Colombia, however, the number of prisons every year never surpass the 137. 12 Because of Colombia’s internal conflict, institutional data separates conflict-related crime information to violence connected to war. I only use non-conflict criminal data to estimate the models. 13 The Colombian penal code defines minimum and maximum sentencing guidelines for all criminal offenses. 12 includes yearly data about education, income, inequality, forced displacement, and rural index of each municipality from 2000 to 2012 (N=1122, T=13, NxT=14,586).14 I use controls traditionally identified by the literature as determinants of imprisonment rate: income per capita measured as municipality income per capita; institutional capacity included as a fiscal performance of the municipality; rural index; education, using as proxy municipal expenditure on education per capita; and population density (Jacobs 1961; Jacobs and Helms 1996, 2001; Kling 2006; Kowalski and Duffield 1990; Listokin 2003a; Pfaff 2007, 2008; Raphael 2010; Sampson 1983, 1985; Shichor, Decker, and O'Brien 1980; Wilson and Boland 1978). Because of Colombia's internal conflict, I controlled for structural differences related to war violence that could affect the incarceration rates using the number of persons that migrated from a municipality due to war, and the number of forced displaced persons that arrived at a municipality in a given year. The DANE provided municipality population density data from 2000 to 2015 (N=1122, T=16, NxT=17,952).15 V. EMPIRICAL STRATEGY For this analysis, I estimate the effect of introducing an adversarial model and plea bargaining on prison population growth by exploiting the natural variation resulting from the LACPR implementation timing in Colombia. Considering the lack of individual data, I use prison information to calculate the average treatment effects. As figure 3 shows, only 11% of Colombian municipalities have prisons.16 Consequently, I use prisonmunicipality-based panel estimations, including both prison-municipality and time fixed effects in all regressions.17 14 For the education variable, I only have information between 2000 and 2010 (NxT=12,342). 15 The methodological appendix includes more details about the data construction process. 16 Colombia has 1101 municipalities, and only 126 of them have prison in their territories. since Colombia is a unitary country, all municipalities follow the same substantive penal code, and those 126 municipalities hold all the prison population. 17 Because of the number of observations, it was not possible to include fixed effects of time-municipality. However, I address the potential time-varying municipalities effects in our event study analysis. Moreover, as I will show the parallel trends assumption holds without including time-varying municipality fixed effects, which allow me to continue with the specified model. 13 I constructed the prison-municipality unit (N=126) based on the municipality administrative division and the prisons' locations across the territory. To match these 126 prison-municipalities with the relevant socioeconomic and crime information, I paired the data of municipalities without prisons to the nearest prisonmunicipality.18 Because all sentenced individuals go to the nearest center in the same Judicial District where the conviction occurred, I restricted the matching process to municipalities under the same jurisdiction.19 Considering the available data differences, I use three empirical approaches using normalize variables by 100,000 inhabitants and logarithmic transformations to allow non-linear relationships.20 First, I estimated an event study model following the Granger-Type Causality test structure to examine whether treatment and control groups had parallel trends21 before the LACPR (2000-2004), and to rule-out potential anticipation effects.22 Additionally, I captured phase-in-effects by extending the model to the post-treatment period. Equation 1 represents this leads-and-lags model: * !!,#$ = #% + ∑& &(') #& &!,#'& + ∑*(% #* &!,#+* + '! + (# + )!,# (1) where !!,#$ represents s for each prison-municipality i and period t: (i) convicted prison population; (ii) firstconviction prison population; (iii) second-conviction prison population; (iv) prison admission rates; (v) prison 18 Section I of the Methodological Appendix contains a detailed explanation of the matching distance algorithm. 19 The uneven distribution of prisons across the Colombian territory exemplifies the difficulties of implementing the methods traditionally used by the empirical literature of mass incarceration in the United States directly into Latin American contexts. Advancing the empirical literature on the causes and consequences of incarceration across the Americas must take into consideration the heterogeneity across different countries and develop new techniques that address these particularities. 20 For zero values, I used the transformation ln(y+1) =0. 21 I tested conditional and unconditional parallel trends. As I will show in the result section, the parallel trends assumption holds to both models. Since the unconditional parallel trend test provides stronger evidence of the comparability between treatment and control groups, I included these results. 22 The difference-in-difference models with time varying treatment the arguable exogeneity of the reform requires an exogeneity condition besides the tradition parallel trend assumption. This means that the implementation process must be independent of the outcome variables, and therefore, municipalities did not change their behavior anticipating the reform before it was implemented. This test allows me to see whether an anticipation effect occurred violating the exogeneity condition. 14 release rates; and (vi) the ratio of admissions over releases. I estimate the outcomes (i) to (iii) by sex. The term ∑& &() #& &!,#'& denotes the sequence of lagged treatment variables (m=-60 months), or in other words, captures the potential differences in the pre-treatment period between treatment and control groups. On the other hand, ∑**(% #* &!,#+* denotes the present and future treatment sequence (p=60 months), capturing the LACPR effect in the outcome variables. This leads-and-lags structure includes prisonmunicipality ('! ) fixed effects, year-month ((# ) fixed effects, and prison-municipality cluster standard errors. During the pre-treatment period, the parameter #& captures anticipation effects by testing an association between the reform and current outcomes before the implementation. Additionally, it estimates differences in pre-treatment trends (parallel trends assumption). Under strict exogeneity and parallel trends, #& = 0 for , = −6, … , −60. In the post-treatment phase (1 = 6, … ,60), ∑**() #* &!,#+* represents the phase-in effects of the reform, therefore parameter #* represents the immediate effect of the policy when 1 = 6, and the additional effects that occur after the LACPR adoption (1 > 0). Secondly, I exploited the timing-variation using a difference-in-difference model. I separate prisonmunicipalities into treatment (under the ACJC) and control groups (under Law 600/00) for each period. Formally, I estimate model 2: !!,#$ = 3% + 3, 45678!,# +3- 9!,# +3. ;!,#'/ + '! + (# + )!,# (2) where !!,#$ represents s prison population outcomes for each prison-municipality i and period t: (i) convicted prison population; (ii) first-conviction prison population; and (iii) second-conviction prison population. I also estimated model 2 by sex. Variable 45678!,# is a binary variable indicating whether the reform has been implemented in a prison-municipality. Vector 9!,# contains the already mentioned economic, demographic, and institutional variables to control for time-varying municipality characteristics. The term ;!,#'/ controls for 15 crime rates and police arrests. For ;!,#'/ , I used a lag-structure23 to avoid endogeneity problems (Listokin, 2003a; Pfaff, 2008, 2011).24 Since the lag-structure imposes restrictions on placebo tests, I incorporated an alternative estimation excluding ;!,#'/ to compare the results with the robustness check evaluations. The results show that excluding ;!,#'/ does not affect the results significantly. Furthermore, model 2 includes time fixed effects ((# ) to control for national tendencies and cyclicality in incarceration rates. Likewise, it contains prison-municipality ('! ) fixed effects to control for nonobservable and time-invariant prison-municipality characteristics, and for differences in the propensity to report data.25 Lastly, the last term of the model )!,# represents a prison-municipality clustered error. Although the implementation uses judicial districts for treatment assignment, the small number does not allow for clustering by them (Cameron and Miller 2015). I report the statistically significant coefficients as unweighted elasticities interpreted as the percentual change in the applicable rate.26 23 I used the National Prosecutor Office information about the length of the criminal process to design the lag structure of the model. On average, the process length since the incident report to trial is between 365 and 730 days. Based on this length of the process, I used a one-year lag measure of arrests and a two-year lag for the crime index 24 Previous literature has identified the problems to evaluate the impact of crime on imprisonment rates since the number of prisoners affects the amount of crime. Particularly, Pfaff (2011) and Listokin (2003) have pointed out, the relationship between crime and incarceration rates is complex and dynamic, the number of individuals in prison in a year depends "not only on the crime rate for that year but also on many lags of the crime rate" (Listokin, 2003, p. 183). 25 I attempted including municipality-time fixed effects or judicial districts-time fixed effects, but the number of observations is insufficient. Considering the parallel trends and exogeneity assumption hold without conditioning by them, I argue my estimators are unbiased. 26 The equitable distribution of the reform across the Colombian population approximates the population-weighted (WLS) and unweighted (OLS) estimates, reducing the presence of unmodeled heterogeneity (Deaton 1997; Goodman-Bacon 2018; Solon, Haider, and Wooldridge 2015; Wooldridge 2001). 16 Finally, in model 3, I estimated a fixed-effect regression controlling for economic, demographic, and institutional cofounding variables, as well as time-trends, for admission and release rates. I used this firstdifference model because of the lack of reliable data between 2005-2007. 27 Model 3 follows this specification: !!,#$ = 3% + 3, 45678!,# + 3- =! ∗ 45678!,# + +3. 9!,# +30 ;!,#'/ + '! + )!,# (3) where !!,#$ represents s prison population outcomes for each prison-municipality i and period t: (i) prison admission rates, (ii) prison release rates, and (iii) the ratio of entries over liberations. 45678!,# , is a binary variable that takes the value of one for all municipalities in the post-reform period (= ≥ 2008) and zero before the ACJC implementation (= ≤ 2004).28 The interaction term =! ∗ 45678!,# captures any cofounding time-trend effect in the first difference model with time-gaps. Additionally, I controlled for socioeconomic, crime, and arrest factors by including vectors 9!,# and ;!,#'/ . Lastly, model 3 contains prison municipality ('! ) fixed effects and cluster standard errors at the prison-municipality level.29 VI. RESULTS The leads-and-lags (model 1) findings suggest that all treatment and control groups had parallel trends and no-anticipation effects occurred before the reform implementation. Figure 5 displays the results for all 27 As mentioned previously, information on entries and releases from prison exists for the pre-reform period and post-reform period. For the pre-reform period between 2000 to 2004, excluding 2001; and after the reform from 2008 to 2015. This eliminates the possibility of exploiting the rolling-out implementation variation. 28 Although specifications (2) and (3) seem similar, the estimations differ substantially. While on the specification (2) the coefficient of interest represents a double difference, in model 3 the estimator characterizes one difference. The double difference comes from the comparison of the average outcome of the treatment and control group separately before and after the reform, and &"#$% − Y &"&'#& |D = 1] − posteriorly the subtraction of the initial difference in the control group from the treatment group (β"! = [Y &"#$% − & [Y Y"&'#& |D = 0]). Using two differences guarantees that the estimator β"! captures the effect of the reform instead of confounding time-trends of the outcome variable. In specification (3), the single difference does not exclude potential time-trends &"#$% )*+", |D = 1] − [Y &"&'#& )*+", |D = 1]). of the entry and release data (β"! = [Y 29 Because of the existence of potential structural differences between the municipalities in the pre-and-post treatment period, the results should not be considered as causal effects of the LACPR on the outcome variables, rather measures of correlation between the different variables and the reform. 17 incarceration rates with information during the rolling-out, and figure 6 shows the findings for entry and release rates, excluding the years 2005-2007 as this data is not available. These figures confirm that for , = −6, … , −60, the confidence interval includes #& = 0,30 offering evidence that no significant difference between the groups existed prior to the LACPR. In the post-treatment period, figure 5 reveals that the LACPR increased the incarceration rate and particularly the second-time rate. In contrast, these results suggest that the reform reduced the female incarceration rate for first-time and second-time convicted women. These findings provide evidence supporting a causal connection between the LACPR and incarceration rates growth. Moreover, the results show a differential effect between men and women associated with Mechanism B (plea bargaining). For females, figure 5 displays a consistent decrease in their incarceration rates. In contrast, for male rates no significant effect is observed using the unrestricted model 1. [FIGURE 5 HERE] Additionally, figure 6 reveals that an increase in admission and release rates occurred after the implementation of the LACPR. Figure 6 illustrates an expansion in admission rates after 12 months of the reform implementation and a rise in release rates after 36 months of this transformation. These findings support the hypothesis that celerity (mechanism A) and a plea-bargaining (mechanism B) caused an increase in incarceration rates, as explained in figure 3. [FIGURE 6 HERE] After finding evidence supporting the difference-in-difference identification assumptions for the outcome variables (parallel trends and treatment exogeneity), I tested these conditions in the cofounding 30 In this model, I use a confidence level of 90% since it increases the probability of rejecting γ- = 0 for m = −6, … , −60. Therefore, since I cannot reject γ- = 0 this suggest that parallel trends existed in the pre-treatment period. 18 variables to confirm the treatment and control groups' comparability. Figure 7 depicts the results of model 1 using the socioeconomic and crime variables. In this figure, I show that over the five years prior to the LACPR treatment and control groups had parallel trends in all socioeconomic, crime, and arrest factors. These findings confirm the quasi-experimental conditions of the Colombian case and support the use of a differencein-difference specification (model 2). [FIGURE 7 HERE] After providing robust evidence of parallel trends and treatment exogeneity, I estimated models 2 and 3. These models' results suggest the LACPR increased the incarceration rate between 18% and 30%, particularly causing a rise of 19%-22% in first-time and 28%-31% in second-time incarceration rates (see table 1). These findings confirm that the introduction of adversariality, ceteris paribus, affects prison growth. Moreover, table 1 shows that no statistical difference between first-time and second-time incarceration rates occurred (see column 4, table 1), supporting the hypothesis that procedural celerity (mechanism A) could have driven this increase. Furthermore, table 1 ratifies the growth in admission and liberations rates (columns 5 and 6, table 1). These findings from model 3 suggest that after the LACPR an increase of 75%-81% in entries and 48%58% in releases happened. As explained previously, the first-difference specification (model 3) does not eliminate previous structural differences as model 2 does, therefore, these findings should be interpreted as strong correlations and not causal links. Nevertheless, the consistent results strongly suggest a potential causal effect. [TABLE 1 HERE] Finally, table 2 displays the difference-in-difference test on female and male incarceration rates. These results confirm the decrease of 25%-27% in the female incarceration rates after the LACPR. Specifically, the female first-time incarceration rate declined by 29%, and second-time decreased by approximately 36%. On the contrary, male incarceration rates grew around 32%-40%, with first-time rates 19 increasing between 35%-42% and second-time rates rising 25%-26%. These gender disparities suggest that plea-bargaining (Mechanism B) affected prison population growth.31 These findings are consistent with previous empirical literature in this area. For instance, social scientists argue that male defenders are more likely to be incarcerated than their female counterparts (Berdejó 2018; Steffensmeier and Allan 1996; Steffensmeier, Kramer, and Streifel 1993). Moreover, scholars studying gender disparities in plea-bargaining negotiations suggest that men tend to receive longer sentences than women in criminal cases (Albonetti 2002; Mustard 2001; Shermer and Johnson 2010; Stacey and Spohn 2006; Starr 2015; Steffensmeier and Allan 1996; Steffensmeier et al. 1993; Ward, Hartley, and Tillyer 2016). [TABLE 2 HERE] VII. CONCLUSION For the last decades, the literature on prison growth has widely documented the increase in incarceration rates in different contexts in the Global North. Still, little is known about the factors driving changes in the Global South. Notably, quantitative researchers have overlooked the Latin American case. This paper proposed to use the quasi-experimental implementation of the U.S. adversarial model in Colombia to evaluate empirically untested hypotheses about the factors that influence prison population growth while advancing the knowledge of Latin American carceral state. The findings suggest that adversariality increases incarceration rates maintaining all other factors constants. For the Colombian case, the change caused a prison population growth of nearly 302-504 inmates per 100,000 inhabitants every year, most of them convicted for a second time. However, the effects of the reform had gender variation. For male incarceration rates, adversariality caused an increase of 192-240 men 31 Since data for the pre-treatment period is not available these estimates could be underestimating the effects for males and overestimated it for females. Still, the effect contrary direction cannot be explained by the data imputation process, strongly suggesting both groups were affected differently. 20 per 100,000 inhabitants yearly, while it decreased female rates in 102-110 women per 100,000 inhabitants annually. These results suggest that two mechanisms of the U.S. adversarial system influenced the prison growth in Colombia. First, the celerity increase reduced drastically procedural terms affecting admission rates 12 months after the reform. And secondly, the introduction of plea bargaining caused a rise in male conviction rates. This evidence is consistent with previous literature connecting plea-bargaining with incarceration growth and gender disparities (Berdejó 2018; Steffensmeier and Allan 1996; Steffensmeier, Kramer, and Streifel 1993). This study contributes to this literature by providing a causal link between these two while showing that expanding the research of prison beyond the U.S. borders can contribute to advance the criminological field. Moreover, it provides one of the few empirical studies on the Latin American prison system expanding the research of the U.S. foreign aid programs in the region. To continue advancing the Global understanding of the carceral state more comparative research is necessary. This paper proposes to use the quasi-experimental implementation of the U.S. wars on crime and drugs in other countries to evaluate untestable hypotheses existing in the literature. As this research shows, international cases contribute to advancing the empirical criminological scholarship in the U.S. and the Global South simultaneously. 21 Figure 1. Latin American Criminal Procedural Revolution, 1990-2020 First Wave Second Wave Third Wave Fourth Wave No ACJS 450 350 - 250 - 150 - 50 •• • - 450 Argentina - - 350 250 150 50 - •••• Panama I •• ~- • - ••• •I . 1 I I I I • • ' 2000 2010 1980 1990 I I I •• • I I I I I I I • • I ••••• •• i- ' ' 1980 1990 2000 2010 I I Costa Rica Dominican Republic I I I I I I I •• I••• I • I • I• I I I I • • • • ·r. • I .,. ••••• •• Honduras I I • •• I Mexico •• •• • I ••• I Nicaragua I I I I I I I .• .....• I •j• •• I Uruguay l I I I I • • • • •••r ' 1990 2000 2010 1980 I I I •• • • ·r••·· Venezuela I 1• • I 1 • 1980 1990 2000 2010 Peru •• •• • •• • J••• • I • • • ; •••••• Paraguay • I I I • I ••• I•••• .1 I Guatemala I I • I • I • I• • · 1· I Colombia I El Salvador I I I I I I •• r·I •••• - • •• •• •·1••·· Ecuador 450 350 . :· r - Chile I I I I I I ...... 250 150 Bolivia I I I I I I I • 50 Incarceration Rate per 100,000 Inhabitants Figure 2. Incarceration Rates Pre-LACPR and Post-LACPR in Latin America (1980-2015) • •• ••➔I • •• • I • I ' 2010 1980 1990 2000 Year I I I I • • • I I I I I ••• .... .1 1• ' ' 1990 2000 2010 1980 I I Figure 3. Distribution of the LACPR and Prison in Colombian Territory, 2005-2008 • □ □ □ January 2005- 22% Population January 2006- 26% Population January 2007- 27% Population January 2008- 25% Population • □ Municipalities with Prisons Muncipalities without Prisons Figure 4. Cases Explaining the Decrease in Pre-Trial Detention by the LACPR Evidence 1A: ↑ First = ↑ Second Conviction Population Mechanism A: Increase Procedural Celerity • Evidence 2A:↑ Admissions & Releases Increase Prison Population Evidence 1B: ↑ Releases Mechanism B: Introduction Plea Bargaining Evidence 2B: ∆ Female≠ ∆ Male Convicted Population Male Incarceration Rate .5 0 -.5 -1 1 0 -1 -2 1 1TTTT_ T I I I I I 1TT TtT~- T---- 0 -1 -2 1I I1111111 1 111 2nd-Time Incarceration Rate -60-48-36-24-12 0 12 24 36 48 60 72 Months relative to Criminal Reform ' 4 2 I - TTT- 0 -2 I 1111 ------ I -60-48-36-24-12 0 12 24 36 48 60 72 Months relative to Criminal Reform 1st-Time Male Incarceration Rate 2 2 0 -2 -4 -6 -36 -24 -12 0 12 24 36 48 60 72 Months relative to Criminal Reform 2nd-Time Male Incarceration Rate 1st-Time Incarceration Rate -60-48-36-24-12 0 12 24 36 48 60 72 Months relative to Criminal Reform ' 1 1-TTT ...... TTTT 0 -1 I I I -2 -3 111 l -36 -24 -12 0 12 24 36 48 60 72 Months relative to Criminal Reform 3 rTTTT TT 111 I I I I +TTT 11 ~~ 1 I 2 1 0 -_ - TT IIIIII 1-i-f-Ja _µ Wl-1141{ - -1 -36 -24 -12 0 12 24 36 48 60 72 Months relative to Criminal Reform -36 -24 -12 0 12 24 36 48 60 72 Months relative to Criminal Reform 1st-Time Female Incarceration Rate 1 2 2nd-Time Female Incarceration Rate Incarceration Rate 1.5 Female Incarceration Rate Figure 5. Event Study Estimates of the Impact of the LACPR on Incarceration Rates 1 - 0 ~~ I -_r r--=- ...T ff -1 -2 -3 -4 I I 11 1 1I I l 111 -36 -24 -12 0 12 24 36 48 60 72 Months relative to Criminal Reform 2 0 -2 -4 -6 -36 -24 -12 0 12 24 36 48 60 72 Months relative to Criminal Reform Figure 6. Event Study Estimates of the Impact of the LACPR on Prison Entries and Releases - Prison Entry Rate 1 I I - .5 I I T T T I I I I 1 - - 1- - - 0 I 1 _L - - - - I I I l __!:. - T I I l l l l I -.5 l -1 -36 -24 -12 0 12 24 Months relative to Criminal Reform 36 1 T Prison Release Rate T T T I I I .5 I I I I l -24 T T I I I I I 1 _ :__ I _ I l r t- - --1 - - - 0 -36 I I I I I -.5 - 48 I 1 T 60 72 - T I I I I I I I I l l l l I l 1 l -12 0 12 24 Months relative to Criminal Reform 36 48 60 72 Figure 7. Pre-Trends of Socio-Economic, Institutional and Crime Controls prior to the LACPR T 0 1 -.5 .6 1 - 1- 1 -1 0 I -.4 1 -.1 -.2 .05 0 -.05 -.05 I T .5 0 -.5 T I - I T T - T T .... T T 1 I 1 1 1 1 -.1 Forced Displacement Expulsion 1 T T I _ l _ l_ -L -60 -48 -36 -24 -12 Months relative to Criminal Reform - I 1 1 l 1 1 1 1 -1 1 T .... .5 I 0 T I I 1 1 1 1 l -.5 T l - T - 1 -1 -60 -48 -36 -24 -12 Months relative to Criminal Reform -60 -48 -36 -24 -12 Months relative to Criminal Reform -60 -48 -36 -24 -12 Months relative to Criminal Reform Percentage of Rural Population Education Investment Industry Tax Revenue T T I I T I , -- T I T T T - -T- ,=- 1 1 1 1 1 1 1 -60 -48 -36 -24 -12 Months relative to Criminal Reform 1 .5 0 -.5 I I - I T - 1- r+ T 1 1 1 1 1 1 1 1 -60 -48 -36 -24 -12 Months relative to Criminal Reform Percentage Change .1 I 1 1 1 1 0 T Forced Displacement Reception Percentage Change 0 T .05 -60 -48 -36 -24 -12 Months relative to Criminal Reform Percentage Change Percentage Change Percentage Change T I I --- -.2 Population Density .1 I .2 -60 -48 -36 -24 -12 Months relative to Criminal Reform T T - .4 Percentage Change T Fiscal Performance Percentage Change .5 Crime Index per 100,000 Inhabitants Percentage Change Percentage Change Arrest per 100,000 Inhabitants .02 T - I T .01 0 -.01 -.02 I ~ T T T T - I 1 1 1 1 1 1 I -60 -48 -36 -24 -12 Months relative to Criminal Reform Table 1. Aggregated Difference-in-Difference and First-Difference Model Results (1) (2) (3) (4) (5) (6) Convicted First Second Ratio First Admissions Releases Prison Conviction Conviction Conviction/Second Convicted Convicted Population Population Population Conviction Population Population Panel A: Without Crime and Arrest Controls LACPR 0.260*** 0.199** 0.271** -0.595 0.563*** 0.460*** (0.0880) (0.0988) (0.117) (1.047) (0.163) (0.153) (7) Convicted Entries/Releases 0.196 (0.214) Estimated Effect 30% 22% 31% 0% 75% 58% 0% Observations R-square 3,619 0.767 2,869 0.643 2,838 0.712 2,509 0.301 2,598 0.667 2,598 0.725 2,558 0.108 0.161** (0.072) 0.170* (0.0919) 0.243** (0.111) -0.663 (1.010) 0.595*** (0.185) 0.393** (0.166) 0.225 (0.233) Estimated Effect 18% 19% 28% 0% 81% 48% 0% Observations R-square 2,310 0.814 2,009 0.668 1,981 0.742 1,737 0.352 1,614 0.784 1,614 0.867 1,610 0.128 23 YES YES 23 YES YES 1.2 YES YES Panel B: Including Crime and Arrest Controls LACPR Mean T=0 140 87 67 4 YES Month-Year FE YES YES YES Prison-Municipality FE YES YES YES YES Cluster robust standard errors in parentheses *** Significant at the 1 percent level; ** Significant at the 5 percent level; * Significant at the 10 percent level Table 2. Difference-in-Difference and First-Difference Model Results by Sex (1) (2) (3) (4) (5) (6) Convicted Female Population Convicted Male Population Female First Conviction Population Male First Conviction Population Female Second Conviction Population Male Second Conviction Population -0.316*** (0.103) 0.337*** (0.114) -0.460*** (0.144) 0.297** (0.126) -0.447*** (0.0989) 0.234* (0.119) Estimated Effect -27% 40% -29% 35% -36% 26% Observations R-square 2,020 0.852 2,020 0.808 2,873 0.698 2,869 0.740 2,838 0.732 2,838 0.729 -0.242** (0.109) 0.276*** (0.092) -0.340** (0.137) 0.347*** (0.112) -0.113 (0.071) 0.224** (0.102) Estimated Effect -25% 32% -29% 42% 0% 25% Observations R-square 1,795 0.868 1,795 0.812 2,013 0.746 2,009 0.799 1,981 0.707 1,981 0.770 YES YES YES YES YES YES Panel A: Without Crime and Arrest Controls LACPR Panel B: Including Crime and Arrest Controls LACPR Mean T=0 34 50 YES Month-Year FE YES YES Prison-Municipality FE YES YES YES Cluster robust standard errors in parentheses *** Significant at the 1 percent level; ** Significant at the 5 percent level; * Significant at the 10 percent level METHODOLOGICAL APPENDIX Data notes The data used in this article came from different sources at different unit levels, periodicity, and identification numbers. The National Penitentiary Institution (INPEC) provided all dependent variables (stock of prison population, entries, and releases from prison). The raw-data contained information at the prison-level reported in June and December of every year between 2000 and 2015. For the admission and release variables, the INPEC did not have reliable data between 2005 and 2008 for the total prison population. For the convicted population the only information available was starting from 2008. To estimate the models using the convicted entry-release information, I created a "proxy" variable of convicted entries and releases prior to 2005. I used the total population entries and releases prior to 2005 and inputted those values to the convicted set. To have an identifier or "key" that allowed me to merge the different datasets, I created a unique prison id to input the Statistical-Administrative National Department (DANE). The DANE provided a unique municipality id to facilitate merging datasets.32 Therefore, I aggregated the prison-level information at the municipality level and maintained the periodicity to pair all datasets. Table A1 displays descriptive statistics of the dependent variables by stage. [TABLE A1 HERE] I use data on reported crimes and arrests from the Colombian National Police. For both arrest and crime data, the National Police reported the exact month and type of crime, as well as the municipality where the offense occurred. Because of the internal conflict, the data excludes crimes committed as part of the war going on in the country. To minimize systematic recording errors and misreporting, I only included homicides, 32 The DANE code eliminates the problem of differences in the way the municipalities are written, and avoids errors due to similar names between two or more municipalities. assaults, and thefts (muggings, to residences, to business, and to vehicles)33 (Martin and Legault 2005; Pepper, Petrie, and Sullivan 2010). To construct the Crime Index, I aggregated crime by using a weighted sum depending on the average sentence of each offense (Mejía, Ortega, and Ortiz 2015): % $ ' !"#$% '()%*!,# = ∑ -&! ∗ "/0%!,# 1 " $ ' where !"#$% '()%*!,# represents aggregated crime in municipality # for period 0; "/0%!,# is the number of offenses 2 per 100,000 inhabitants that occur in municipality # for period 0; 3' is the average sentence length in years for individuals convicted for offense 2; 3$ is the sum of 3' , which is 80 in this case. Table A2 shows the construction of 3' and 3$ . [TABLE A2 HERE] The Center of Economic Studies (CEDE) from Universidad de Los Andes provided the socioeconomic controls used. The public demographic information came from the DANE public datasets. The DANE estimates the population-based in the 2005 Census (the latest by this time). Although a new census took place in 2018, the data collected is not available yet. Both datasets contained municipality-level data yearly. To control for these socioeconomic variables without sacrificing observations, I duplicated the annual information in this set and matched it with the semesterly prison data. Table A3 presents the descriptive statistics of the socioeconomic controls included in the models. [TABLE A3 HERE] To create the final dataset, I merged data from the different sources at the prison-municipality level with a periodicity of six months (June and December) from 2000 to 2015. I grouped prisons located in the same municipality, and crimes and arrests that happened from January to June and July to December in 33 The Colombian National Police refers to these crimes as high-impact crimes: those offenses that have the greater negative social impact/cost. Other high-impact crimes for the Colombian case are terrorism, subversive acts and kidnappings. each municipality.34 I used the annual socioeconomic and demographic variables for the observations in June and December and merged it with the crime and arrest data using unique municipality identifiers. I focused my analysis on 126 municipalities with prisons, and I matched crime, arrests, socioeconomic and demographic variables to the closest "prison-municipality." For those cases in which one observation falls within the intersection of two "prison-municipalities," I matched it with the municipality from the same judicial district, if both "prison-municipalities" belong to the same judicial district I match it randomly to one of those municipalities. The following equation represents the algorithm: ) 4#( 567( − 7% 9 + (<( − <% )) , ∀ ( = 1, … ,997; 3 = 1, … ,126 (1.A) Placebo Tests To provide further evidence that the effects shown in the regressions from tables 1 to 4 are indeed the result of the implementation of the LACPR, I performed two falsification tests or placebo test.35 First, I assigned a random date of entry to the reform to every judicial district. Using these new data and its respective exposure time, I tested the effect of this artificial treatment on the convicted prison rates of interest. The results are presented in table 5. As expected, the artificial treatment does not appear to have consistent significance and similarities with the results shown above. The exposure time coefficient is significant and positive; however, this does not raise any concern regarding the validity of the results since the main estimations did not find a robust effect of this variable. [TABLE A4 HERE] 34 &'() !#,%,&'() = $*+&,(',-. !#,%,* , where !#,%,&'() is the number of offenses s that happened in municipality % recording under June; !#,%,* is the number of offenses s that occur in municipality % in the month &. 35 In econometrics a placebo test is described as a “conceptual placebo”. It consists in repeating the analysis on a dataset where no intervention took place. Besides the randomization of the groups, I estimated an additional regression using the actual order the LACPR implementation but for the pre-treatment period. The third and fourth columns of table 6 confirm that this artificial treatment did not have any significant effect either. These results suggest that the effects found in the main estimations are capturing the LACPR impact in incarceration rates, in lieu of a spurious or concurrent phenomenon. [TABLE A5 HERE] Table A1. Descriptive Statistics of the Dependent Variables by Stages (2000-2015) * Variable Stage N Mean Std. Dev. Min Stage 1 524 241 328.73 Stage 2 1032 317 759.04 Stage 3 1867 181 437.93 Conviction Rate Stage 4 703 61 63.12 Total 4126 202 502.24 Stage 1 385 44 40.28 Stage 2 780 55 144.62 Stage 3 1408 43 87.86 Entry Rate Stage 4 530 16 18.78 Total 3103 41 95.76 Stage 1 Stage 2 Release Rate Stage 3 Stage 4 Total Stage 1 Stage 2 Stage 3 Male Conviction Rate Stage 4 Total Stage 1 Stage 2 Stage 3 Female Conviction Rate Stage 4 Total *Rates calculated by 100,000 residents 385 780 1408 530 3103 263 505 913 343 2024 263 505 913 343 2024 40 45 37 15 36 266 283 167 61 191 30 31 22 16 24 Table A2. Crime Index Weight Parameters Min. Max Offenses p* Sentence* Sentence* Homicide 17 37 27 Assaults 1 15 8 Muggings 1 16 9 Business Robberies 2 28 15 Home Burglaries 6 14 10 Vehicle Thefts 7 15 11 *Calculated using the Colombian Penal Code by June 2017 30.33 87.63 66.92 14.25 64.84 384.53 642.94 418.12 59.41 455.57 56.88 96.40 42.15 29.15 60.91 p* P+ 0.34 0.10 0.11 0.19 0.12 0.14 0 0 0 0 0 0 0 0 0 0 Max 2410 5493 4040 356 5493 228 2573 985 256 2573 0 0 0 0 0 0 0 0 0 0 0 0 0 0 0 160 1253 846 86 1253 2410 4007 4040 300 4040 370 976 279 160 976 Table A3. Descriptive Statistics of the Cofounding and Control Variables by Stages (2000-2015)* Variable Stage N Mean Std. Dev. Min Max Variable Stage N Mean Std. Dev Min Max Stage 1 411 121.93 109.97 9.59 628.28 Stage 1 524 388.03 862.26 29.64 4064.94 Stage 2 858 102.74 88.65 0.00 740.86 Stage 2 1061 234.70 567.40 19.18 3453.85 Population Arrest Rate Stage 3 1375 96.00 86.83 2.93 711.14 Stage 3 1771 75.28 75.30 0.57 401.44 Density Stage 4 600 73.51 58.75 9.74 339.70 Stage 4 770 209.11 504.60 1.86 2298.32 Total 3244 96.91 87.33 0.00 740.86 Total 4126 180.97 487.88 0.57 4064.94 Stage 1 411 30.84 15.72 5.59 72.85 Stage 1 524 706.68 1736.18 3.00 21457 Stage 2 858 29.69 16.76 0.00 88.68 Displaced Stage 2 1061 1290.64 3809.75 0.00 49615 Crime Index Stage 3 1375 22.08 16.61 0.00 100.05 Population Stage 3 1771 2970.33 4770.19 0.00 34519 Rate Expelled Stage 4 600 22.03 14.12 2.83 92.37 Stage 4 770 6854.12 9117.77 0.00 74989 Total 3244 25.19 16.57 0.00 100.05 Total 4126 2975.72 5793.63 0.00 74989 Stage 1 524 0.89 0.83 0.00 3.08 Stage 1 524 2771.51 8034.25 0.00 52304 Stage 2 1061 0.99 1.01 0.03 4.82 Displaced Stage 2 1061 2137.46 5605.11 0.00 45928 Rural Index Stage 3 1771 1.42 1.17 0.09 6.91 Population Stage 3 1771 2296.12 3396.49 9.00 26193 Arrival Stage 4 770 0.69 0.44 0.02 2.28 Stage 4 770 6252.08 7340.77 0.00 45219 Total 4126 1.11 1.03 0.00 6.91 Total 4126 2975.72 5793.63 0.00 74989 Stage 1 524 0.03 0.06 0.00 0.35 Stage 1 440 0.73 0.06 0.59 0.85 Investment Stage 2 1061 0.04 0.09 0.00 0.88 Stage 2 787 0.72 0.08 0.58 0.89 in Education Stage 3 Land Gini 1771 0.02 0.03 0.00 0.26 Stage 3 1206 0.71 0.07 0.42 0.85 Per Capita Stage 4 770 0.02 0.03 0.00 0.29 Stage 4 627 0.67 0.06 0.44 0.85 Total 4126 0.03 0.06 0.00 0.88 Total 3060 0.71 0.07 0.42 0.89 Stage 1 311 0.03 0.06 0.00 0.34 Stage 1 468 61.87 7.32 46.02 84.02 Tax 623 0.05 0.09 0.00 0.83 Stage 2 925 62.67 7.41 27.08 86.65 Revenues Stage 2 Fiscal from Industry Stage 3 992 0.03 0.03 0.00 0.24 Stage 3 1553 60.47 6.72 35.75 80.93 Performance and Stage 4 426 0.02 0.03 0.00 0.22 Stage 4 673 58.90 7.47 23.70 80.70 Business Total 2352 0.03 0.06 0.00 0.83 Total 3619 60.92 7.24 23.70 86.65 *Rates calculated by 100,000 residents REFERENCES Albonetti, Celesta A. 2002. "The Joint Conditioning Effect of Defendant's Gender and Ethnicity on Length of Imprisonment under the Federal Sentencing Guidelines for Drug Trafficking/Manufacturing Offenders." J. Gender Race & Just. 6:39. Anderson, James M., Jeffrey R. Kling, and Kate Stith. 1999. "Measuring Interjudge Sentencing Disparity: Before and after the Federal Sentencing Guidelines." The Journal of Law and Economics 42(S1):271–308. Ashenfelter, Orley, Theodore Eisenberg, and Stewart J. Schwab. 1995. "Politics and the Judiciary: The Influence of Judicial Background on Case Outcomes." The Journal of Legal Studies 24(2):257–81. Beckett, Katherine. 1999. Making Crime Pay: Law and Order in Contemporary American Politics. Oxford University Press. Berdejó, Carlos. 2018. "Criminalizing Race: Racial Disparities in Plea-Bargaining." BCL Rev. 59:1187. Bibas, Stephanos. 2012. "Incompetent Plea Bargaining and Extrajudicial Reforms." Harv. L. Rev. 126:150. Blumstein, Alfred, and Allen J. Beck. 1999. "Population Growth in U.S. Prisons, 1980-1996." Crime and Justice 26:17–61. Blumstein, Alfred, and Soumyo Moitra. 1979. "An Analysis of the Time Series of the Imprisonment Rate in the States of the United States: A Further Test of the Stability of Punishment Hypothesis." The Journal of Criminal Law and Criminology (1973-) 70(3):376–90. Braman, Donald. 2004. "Families and the Moral Economy of Incarceration." Journal of Religion & Spirituality in Social Work: Social Thought 23(1–2):27–50. Bushway, Shawn D., Emily G. Owens, and Anne Morrison Piehl. 2012. "Sentencing Guidelines and Judicial Discretion: Quasi-experimental Evidence from Human Calculation Errors." Journal of Empirical Legal Studies 9(2):291–319. Bushway, Shawn D., Allison D. Redlich, and Robert J. Norris. 2014. "An Explicit Test of Plea Bargaining in the 'Shadow of the Trial.'" Criminology 52(4):723–54. Cameron, A. Colin, and Douglas L. Miller. 2015. "A Practitioner's Guide to Cluster-Robust Inference." Journal of Human Resources 50(2):317–72. Caplow, Theodore, and Jonathan Simon. 1999. "Understanding Prison Policy and Population Trends." Crime and Justice 26:63–120. CEJA. 2015. Prisión Preventiva y Reforma Procesal Penal En América Latina. Centro de Estudios Para la Justicia. Church, Thomas. 1976. "Plea Bargains, Concessions and the Courts: Analysis of a Quasi-Experiment." Law & Society Review 10(3):377–401. Clear, Todd R. 2007. "The Impacts of Incarceration on Public Safety." Social Research: An International Quarterly 74(2):613–30. Comfort, Megan. 2007. "Punishment beyond the Legal Offender." Annu. Rev. Law Soc. Sci. 3:271–96. Crespo, Andrew Manuel. 2018. "The Hidden Law of Plea Bargaining." Columbia Law Review 118(5):1303– 1424. Deaton, Angus. 1997. The Analysis of Household Surveys: A Microeconometric Approach to Development Policy. The World Bank. Dudley, Steven, and James Bargent. 2017. The Prison Dilemma: Latin America's Incubators of Organized Crime. InSight Crime. Dumont, Dora M., Brad Brockmann, Samuel Dickman, Nicole Alexander, and Josiah D. Rich. 2012. "Public Health and the Epidemic of Incarceration." Annual Review of Public Health 33:325–39. Feeley, Malcolm M. 1978. "Perspectives on Plea Bargaining." Law & Soc'y Rev. 13:199. Feeley, Malcolm M. 1982. "Plea Bargaining and the Structure of the Criminal Process." The Justice System Journal 338–54. Fryer, Roland G., Paul S. Heaton, Steven D. Levitt, and Kevin M. Murphy. 2005. Measuring the Impact of Crack Cocaine. National Bureau of Economic Research. Garland, D. 2001. The Culture of Control: Crime and Social Order in Contemporary Society. OUP Oxford. Garland, David. 2001. Mass Imprisonment: Social Causes and Consequences. Sage. Geller, Amanda, Carey E. Cooper, Irwin Garfinkel, Ofira Schwartz-Soicher, and Ronald B. Mincy. 2012. "Beyond Absenteeism: Father Incarceration and Child Development." Demography 49(1):49–76. Gendarmeria de Chile. 2000. Compendio Estadístico. Goldstein, Abraham S., and Martin Marcus. 1977. "The Myth of Judicial Supervision in Three" Inquisitorial" Systems: France, Italy, and Germany." The Yale Law Journal 87(2):240–83. Goldstein, Joseph. 1975. "For Harold Lasswell: Some Reflections on Human Dignity, Entrapment, Informed Consent, and the Plea Bargain." The Yale Law Journal 84(4):683–703. Goodman-Bacon, Andrew. 2018. Difference-in-Differences with Variation in Treatment Timing. National Bureau of Economic Research. Grossman, Gene M., and Michael L. Katz. 1983. "Plea Bargaining and Social Welfare." The American Economic Review 73(4):749–57. Hagan, John, and Ronit Dinovitzer. 1999. "Collateral Consequences of Imprisonment for Children, Communities, and Prisoners." Crime and Justice 26:121–62. Haskins, Anna R. 2015. "Paternal Incarceration and Child-Reported Behavioral Functioning at Age 9." Social Science Research 52:18–33. Haskins, Anna R. 2016. "Beyond Boys' Bad Behavior: Paternal Incarceration and Cognitive Development in Middle Childhood." Social Forces 95(2):861–92. Heumann, Milton. 1975. "A Note on Plea Bargaining and Case Pressure." Law & Society Review 9(3):515– 28. Heumann, Milton, and Colin Loftin. 1979. "Mandatory Sentencing and the Abolition of Plea Bargaining: The Michigan Felony Firearm Statute." Law and Society Review 393–430. Higuera Guío, Clara Milena. 2016. Boletín Estadístico Sistema Penal Acusatorio en Colombia 11 Años. Bogota, Colombia: Consejo Superior de la Judicatura. Ignatieff, Michael. 1978. A Just Measure of Pain: The Penitentiary in the Industrial Revolution, 1750-1850. Pantheon. Jacobs, David, and Ronald Helms. 2001. "Toward a Political Sociology of Punishment: Politics and Changes in the Incarcerated Population." Social Science Research 30(2):171–94. Jacobs, David, and Ronald E. Helms. 1996. "Toward a Political Model of Incarceration: A Time-Series Examination of Multiple Explanations for Prison Admission Rates." American Journal of Sociology 102(2):323–57. Jacobs, Jane. 1961. "The Life and Death of Great American Cities: The Failure of Town Planning." Penguin, Harmondsworth. by Guest on May 23:2011. Kling, Jeffrey R. 2006. "Incarceration Length, Employment, and Earnings." American Economic Review 96(3):863–76. Kowalski, Gregory S., and Don Duffield. 1990. "The Impact of the Rural Population Component on Homicide Rates in the United States: A County-Level Analysis." Rural Sociology 55(1):76–90. LaCasse, Chantale, and A. Abigail Payne. 1999. "Federal Sentencing Guidelines and Mandatory Minimum Sentences: Do Defendants Bargain in the Shadow of the Judge?" The Journal of Law and Economics 42(S1):245–70. Langbein, John H. 1978. "Torture and Plea Bargaining." The University of Chicago Law Review 46(1):3–22. Langbein, John H., and Lloyd L. Weinreb. 1977. “Continental Criminal Procedure: Myth and Reality.” Yale LJ 87:1549. Langer, Máximo. 2007. "Revolution in Latin American Criminal Procedure: Diffusion of Legal Ideas from the Periphery." The American Journal of Comparative Law 55(4):617–76. Langer, Máximo. 2021. "Plea Bargaining, Conviction Without Trial, and the Global Administratization of Criminal Convictions." Annual Review of Criminology 4(1):null. Listokin, Yair. 2003a. "Does More Crime Mean More Prisoners? An Instrumental Variables Approach." The Journal of Law and Economics 46(1):181–206. Listokin, Yair. 2003b. “Does More Crime Mean More Prisoners? An Instrumental Variables Approach.” The Journal of Law and Economics 46(1):181–206. de Luca, Javier Augusto. 2008. Jornadas Iberoamericanas. Oralidad En El Proceso y Justicia Penal Alternativa. INACIPE. Martin, Robert A., and Richard L. Legault. 2005. "Systematic Measurement Error with State-Level Crime Data: Evidence from the 'More Guns, Less Crime' Debate." Journal of Research in Crime and Delinquency 42(2):187–210. Martínez Cuéllar, Mariana, Yezid Hernández Luna, and Adela del Pilar Parra González. 2008. Boletín Dirección de Justicia y Seguridad: Cifras de Justicia, Jurisdicción Penal 1996-2007. Bogota, Colombia: DNP. Marton Ramaciotti, Amanda. 2017. “Cárceles en Latinoamérica y la crisis de seguridad regional.” El Tiempo, October 13. Marvell, Thomas B., and Carlisle E. Moody. 1994. "Prison Population Growth and Crime Reduction." Journal of Quantitative Criminology 10(2):109–40. Marvell, Thomas B., and Carlisle E. Moody. 1997. "Age-Structure Trends and Prison Populations." Journal of Criminal Justice 25(2):115–24. Mauer, Marc. 1999. The Crisis of the Young African American Male and the Criminal Justice System. April. McLeod, Allegra M. 2010. "Exporting U.S. Criminal Justice." Yale L. & Pol' y Rev. 29:83. Mejía, Daniel, Daniel Ortega, and Karen Ortiz. 2015. “Un Análisis de La Criminalidad Urbana En Colombia.” Consultado En Www. Caf. Com. Munroe, Robert L., Ruth H. Munroe, and Beatrice Blyth Whiting. 1981. Handbook of Cross-Cultural Human Development. Garland STPM Press. Mustard, David B. 2001. "Racial, Ethnic, and Gender Disparities in Sentencing: Evidence from the U.S. Federal Courts." The Journal of Law and Economics 44(1):285–314. Newman, Donald J., and Frank J. Remington. 1966. Conviction: The Determination of Guilt or Innocence without Trial. Vol. 278. Little, Brown Boston. Padgett, John F. 1985. "The Emergent Organization of Plea Bargaining." American Journal of Sociology 90(4):753–800. Pager, Devah. 2003. "The Mark of a Criminal Record." American Journal of Sociology 108(5):937–75. Pepper, John, Carol Petrie, and Sean Sullivan. 2010. "Measurement Error in Criminal Justice Data." Pp. 353– 74 in Handbook of quantitative criminology. Springer. Pettit, Becky, and Bruce Western. 2004. "Mass Imprisonment and the Life Course: Race and Class Inequality in U.S. Incarceration." American Sociological Review 69(2):151–69. Pfaff, John. 2017. Locked in: The True Causes of Mass Incarceration-and How to Achieve Real Reform. Basic Books. Pfaff, John F. 2007. "The Empirics of Prison Growth: A Critical Review and Path Forward." J. Crim. L. & Criminology 98:547. Pfaff, John F. 2008. "Criminology: The Empirics of Prison Growth: A Critical Review and Path Forward, 98 J. Crim." L. & Criminology 547. Pfaff, John F. 2011. "The Micro and Macro Causes of Prison Growth." Ga. St. U.L. Rev. 28:1239. Raphael, Steven. 2010. Improving Employment Prospects for Former Prison Inmates: Challenges and Policy. Reitz, Kevin R. 1997. Zimring, Hawkins, and the Macro Problems of Imprisonment. JSTOR. Riego, Cristián. 2007. “Nuevas Tendencias de Las Reformas Procesales Penales En América Latina.” Reformas Procesales Penales En América Latina: Resultados Del Proyecto de Seguimiento IV Etapa 3. Rothman, DJ. 1990. THE REGULATION OF MADNESS-THE ORIGINS OF INCARCERATION IN FRANCECASTEL, R. AMER SOCIOLOGICAL ASSOC 1722 N S.T. N.W., WASHINGTON, DC 20036-2981. Ruback, Richard Barry, and Neil Alan Weiner. 1993" “Social and Cultural Aspects of Interpersonal Violent Behaviors. Introductio".” Violence and Victims 8(3):193. Rubinstein, Michael L., and Teresa J. White. 1979"Alaska's’s Ban on Plea Bargainin".” Law and Society Review 367–83. Rusche, Georg, and Otto Kirchheimer. 2003. Punishment and Social Structure. Transaction publishers. Salinero Echeverría, Sebastián. 2012. “¿ Por Qué Aumenta La Población Penal En Chile?: Un Estudio Criminológico Longitudinal.” Ius et Praxis 18(1):113–50. Sampson, Robert J. 1983" “Structural Density and Criminal Victimizatio".” Criminology 21(2):276–93. Sampson, Robert J. 1985" “Neighborhood and Crime: The Structural Determinants of Personal Victimizatio".” Journal of Research in Crime and Delinquency 22(1):7–40. Sampson, Robert J., and Janet L. Lauritsen. 1997" “Racial and Ethnic Disparities in Crime and Criminal Justice in the United State".” Crime and Justice 21:311–74. Sampson, Robert J., and Charles Loeffler. 2010"Punishment's’s Place: The Local Concentration of Mass Incarceratio".” Daedalus 139(3):20–31. Savitsky, Douglas. 2012" “Is Plea Bargaining a Rational Choice? Plea Bargaining as an Engine of Racial Stratification and Overcrowding in the United States Prison Syste".” Rationality and Society 24(2):131–67. Secretaría de Gobernación México. 2016. Porcentaje de Cobertura Nuevo Sistema Penal Acusatorio. Prensa. 106. Shannon, Sarah K. S., Christopher Uggen, Jason Schnittker, Melissa Thompson, Sara Wakefield, and Michael Massoglia. 2017" “The Growth, Scope, and Spatial Distribution of People With Felony Records in the United States, 1948–201".” Demography 54(5):1795–1818. Shepherd, Joanna M. 2002" “Fear of the First Strike: The Full Deterrent Effect oCalifornia's’s Two-and ThreeStrikes Legislatio".” The Journal of Legal Studies 31(1):159–201. Shermer, LaureO'Neillll, and Brian D. Johnson. 2010" “Criminal Prosecutions: Examining Prosecutorial Discretion and Charge Reductions in U.S. Federal District Court".” Justice Quarterly 27(3):394–430. Shichor, David, David L. Decker, and Robert MO'Brienen. 1980" “The Relationship of Criminal Victimization, Police per Capita and Population Density in Twenty-Six Citie".” Journal of Criminal Justice 8(5):309–16. Simes, Jessica T. 2016" “Neighborhood Attainment After Priso".” Simes, Jessica T. 2018" “Place and Punishment: The Spatial Context of Mass Incarceratio".” Journal of Quantitative Criminology 34(2):513–33. Solon, Gary, Steven J. Haider, and Jeffrey M. Wooldridge. 2015" “What Are We Weighting Fo"?” Journal of Human Resources 50(2):301–16. Sorensen, Jon, and Don Stemen. 2002" “The Effect of State Sentencing Policies on Incarceration Rate".” Crime & Delinquency 48(3):456–75. Stacey, Ann Martin, and Cassia Spohn. 2006" “Gender and the Social Costs of Sentencing: An Analysis of Sentences Imposed on Male and Female Offenders in Three U.S. District Court".” Berkeley J. Crim. L. 11:43. Starr, Sonja B. 2015" “Estimating Gender Disparities in Federal Criminal Case".” American Law and Economics Review 17(1):127–59. Steffensmeier, Darrell, and Emilie Allan. 1996" “Gender and Crime: Toward a Gendered Theory of Female Offendin".” Annual Review of Sociology 22(1):459–87. Steffensmeier, Darrell, John Kramer, and Cathy Streifel. 1993" “Gender and Imprisonment Decision".” Criminology 31(3):411–46. Stolzenberg, Lisa, and Stewart JD'Alessioio. 1996" “The Unintended Consequences of Linking Sentencing Guidelines to Prison Populations-A Reply to Moody and Marvel".” Criminology 34:269. Tonry, Michael. 1997" “Ethnicity, Crime, and Immigratio".” Crime and Justice 21:1–29. Travis, Jeremy, Bruce Western, and F. Stevens Redburn. 2014" “The Growth of Incarceration in the United States: Exploring Causes and Consequence".” Wakefield, Sara, and Christopher Wildeman. 2013. Children of the Prison Boom: Mass Incarceration and the Future of American Inequality. Oxford University Press. Ward, Jeffrey T., Richard D. Hartley, and Rob Tillyer. 2016" “Unpacking Gender and Racial/Ethnic Biases in the Federal Sentencing of Drug Offenders: A Causal Mediation Approac".” Journal of Criminal Justice 46:196–206. Weninger, Robert A. 1987" “The Abolition of Plea Bargaining: A Case Study of El Paso County, Texa".” UCLA L. Rev. 35:265. Western, Bruce. 2002" “The Impact of Incarceration on Wage Mobility and Inequalit".” American Sociological Review 526–46. Western, Bruce. 2006" “Incarceration, Unemployment, and Inequalit".” Pp. 191–212 in The Inequality Reader: Contemporary and Foundational Readings in race, Class and Gender. Westview Press Boulder, CO. Western, Bruce, and Catherine Sirois. 2017. Racial Inequality in Employment and Earnings after Incarceration. working paper, Harvard University, Cambridge, MA. Wildeman, Christopher. 2009" “Parental Imprisonment, the Prison Boom, and the Concentration of Childhood Disadvantag".” Demography 46(2):265–80. Wildeman, Christopher. 2016" “Incarceration and Population Health in Wealthy Democracie".” Criminology 54(2):360–82. Wilson, James Q., and Barbara Boland. 1978" “The Effect of the Police on Crim".” Law and Society Review 367–90. Wooldridge, J. M. 2001. Unobserved Heterogeneity and Estimation of Average Partial Effects, Michigan State University, Department of Economics. mimeo. Zimring, Franklin E., Gordon J. Hawkins, and Gordon Hawkins. 1993. The Scale of Imprisonment. University of Chicago Press. Zorro Medina, Ángela. 2014. “El Impacto Del Sistema Penal Acusatorio En El Crecimiento de La Población Reclusa Condenada y Sin Condena En El Corto Plazo En Colombia.” Uniandes. Zorro-Medina, Angela, Camilo Acosta Mejia, and Daniel Mejia. 2016. “Certainty vs. Severity Revisited: Evidence for Colombia.” Documento CEDE (2016–21).